Genome-wide epigenetic and mRNA-expression profiling followed by CRISPR/Cas9-mediated gene-disruptions corroborate the MIR141/MIR200C-ZEB1/ZEB2-FGFR1 axis in acquired EMT-associated EGFR TKI-resistance in NSCLC cells
Introduction
Lung cancer is the leading cause of cancer-related deaths worldwide. Approximately 80% of cases are non-small cell lung cancer (NSCLC) and 10–20% of these have activating mutations in the gene encoding the receptor tyrosine kinase (RTK) epidermal growth factor receptor (EGFR) (1). Hence, EGFR has been a target of treatment with the use of 1st, 2nd, and 3rd generation tyrosine kinase inhibitors (TKIs) such as erlotinib, afatinib, and osimertinib (2-4). EGFR TKI-resistance (E-TKI-R) in consequence of secondary mutations in EGFR, such as T790M, can be circumvented by using 3rd generation TKIs (2,4). However, despite initial efficacy, patients will develop E-TKI-R over time (1,5). TKI-R is a consequence of genetic and epigenetic alterations supporting EGFR bypass signaling and an epigenetic mechanism of acquired E-TKI-R receiving increased attention is epithelial-mesenchymal-transition (EMT) (6-9). In vitro studies have shown strong association between EMT and development of E-TKI-R and in vivo studies have identified EMT in 20–40% of E-TKI-R cases (6,7,9,10).
The EMT program involves the disruption of cell-cell adherence and tight junctions as well as loss of cell polarity of the epithelial cancer cells for the acquisition of a mesenchymal-like phenotype (9). Molecularly, EMT is characterized by the loss of the epithelial markers such as ESRP1, ESRP2, EPCAM, GRHL1, GRHL2, OVOL1, OVOL2, and E-cadherin (CDH1), and a subsequent up-regulation of the mesenchymal markers Vimentin (VIM) and N-cadherin (CDH2) (9). Being a transcriptional differentiation program, EMT is governed by core EMT transcription factors (EMT-TFs) ZEB1, ZEB2, SNAI1, SNAI2, TWIST1, and TWIST2 which acts as both transcriptional repressors and activators and together with other transcriptional regulating factors form an intricate regulatory network of transcriptional circuitry (9-11). On top of this, EMT-TF expression levels are through auto-regulatory loops mediated by miRNAs exemplified by ZEB1 and ZEB2 expression being regulated by the EMT suppressing MIR200-family (12,13). The exact mechanisms by which EMT contributes to E-TKI-R has been suggested to be related to increased anti-apoptotic abilities and activation of alternative EMT-associated RTKs providing bypass signaling to TKI-inhibited EGFR (6,7). Such alternative RTKs support EMT by signaling through the PI3K and MAPK pathways, which are central regulators of cell proliferation, survival, migration, and differentiation. Examples of alternative RTKs are IGF1R, AXL, and FGFR1 [reviewed in (6,7)]. Activation of transforming growth factor-beta (TGF-β) receptor and bone morphogenetic protein (BMP) receptor mediated signaling by TGF-β and BMPs facilitate the transcriptional regulatory function of suppressor of mothers against decapentaplegic (SMAD) complexes that induces EMT through up-regulation of EMT-TFs (9-11).
EMT-TFs are closely interconnected to a range of epigenetic modifiers acting on the DNA and histone levels that allows for gene specific changes in epigenetic modifications (10). This is exemplified by the interactions of the transcription factors ZEB1 and SNAI1 with histone modifiers such as LSD1, EZH2, SUV39H1, and G9a and their capability to recruit DNA methyl transferases (DNMTs) (10). The down-regulation of the CDH1 gene encoding E-cadherin is considered a hallmark of EMT, and SNAI1 and ZEB1 both recruit histone deacetylases (HDACs) as well DNMTs to the CDH1 promoter in EMT (10). On the genomic scale, NSCLC cells, including cells with acquired E-TKI-R, can be categorized into epithelial-like or mesenchymal-like based on DNA-methylation patterns (14). The histone modification H3K36me3 exemplifies a histone modification displaying genome-wide redistribution during EMT (15). H3K36me3 is primarily deposited at the 3'-end of transcriptional active genes by the SETD2 methyl transferase which is recruited by the elongating RNA polymerase (16,17). This prevents cryptic transcriptional initiation through a mechanism which also involves DNA-methylation of intragenic regions by DNMT3A and DNMT3B (18). Moreover, SETD2 and accompanied H3K36me3 is in NSCLC adenocarcinoma described to inhibit STAT1-IL8-mediated EMT and thereby tumor growth and metastasis (19).
The NSCLC HCC827 cell line harbors the recurrently observed adenocarcinoma exon 19del driver mutation in EGFR. We previously established HCC827 cell clones with EMT-associated E-TKI-R (EMT-E-TKI-R) (20). Such mesenchymal HCC827 cell clones (HCC827EMT cells) were raised in parallel with epithelial HCC827 cell clones (HCC827MET cells) with E-TKI-R caused by EGFR bypass signaling due to over-expression of the RTK c-MET (MET) in consequence of MET-amplification (MET-E-TKI-R) (20). In our previous analyses of the HCC827EMT cells, we showed that FGFR1 up-regulation supports EGFR bypass signaling and pinpointed ZEB1 to be a candidate core EMT-TF to govern EMT-E-TKI-R (20-22). This is in line with other studies of NSCLC EMT-E-TKI-R showing that FGFR1 is a potential bypass RTK (23,24), ZEB1 is a driver (25,26), and MIR200C and MIR141 to be inhibitors through an auto-regulatory loop with ZEB1 (12,27,28). To consolidate the knowledge of NSCLC EMT-E-TKI-R, we here present analyses for EMT-E-TKI-R-mediated genome-wide changes in mRNA-expression, DNA-methylation, and H3K36me3, as well as functional analyses of candidate genes. We present the following article in accordance with the MDAR reporting checklist (available at https://tlcr.amegroups.com/article/view/10.21037/tlcr-22-507/rc).
Methods
Cell lines
HCC827 cells (RRID:CVCL_2063) were grown at 37 °C and 5% CO2 in RPMI supplemented with 10% fetal bovine serum and 1% penicillin-streptomycin (Gibco, Thermo Fisher Scientific, Waltham, MA, USA). We previously established the HCC827 erlotinib EMT-E-TKI-R cell clones, HCC827EMT clones 4 and 10, used in this study (20). HCC827EMT cell clones 4 and 10 have a well-characterized EMT morphological status (20). Summarized, HCC827EMT cell clones 4 and 10 display an EMT-mediated mesenchymal phenotype relative to the parental HCC827 cells (HCC827PAR cells) displaying an epithelial phenotype (20). mRNA-expression analyses, western blot analyses, and immunological staining’s, e.g., E-cadherin (CDH1) and Vimentin (VIM) verified decreased and increased expression, respectively, in HCC827EMT cell clones 4 and 10 relative to HCC827PAR cells (20). The HCC827EMT cells were grown in the presence of 5 µM erlotinib (Selleckchem, Houston, TX, USA, Cat#S1023) in solvent dimethyl sulfoxide (DMSO). HCC827Cas9 cells with stable expression of Cas9 were generated by transduction of pLentiCas9-Blast (Addgene, Watertown, MA, USA, Cat#52962) into HCC827PAR cells as previously described (29). HCC827Cas9 cells with CRISPR/Cas9-mediated genetic modification of ZEB1, MIR200C, MIR141, and FGFR1 resulting from transduction of sgRNA expression vectors were grown as HCC827PAR cells after finalization of hygromycin or puromycin resistance selection. E-TKI-R HCC827Cas9 cells with CRISPR/Cas9-mediated ZEB1 depletion were established by a continuous high-dose erlotinib approach as described (30). For every passage (P1 to P15) half of the cells in a T75 flask were processed for RNA, DNA, and protein extraction, one fourth of the cells frozen, and the remaining cells used for continued growth. Erlotinib resistance was verified by cell viability analyses. Erlotinib treated HCC827Cas9 cells harboring CRISPR/Cas9-mediated FGFR1 depletion were generated by adding 1 µM erlotinib and cells grown until P2. For control, HCC827Cas9 cells harboring control sgRNA, were supplemented with DMSO solvent to match the added volume of erlotinib. Figure S1 summarize the HCC827-derived cell lines analyzed in this study.
RNA-sequencing, differential mRNA-expression analysis and gene specific mRNA-expression analyses
RNA extraction was performed using TRI-reagent (Sigma-Aldrich, Saint Louis, MO, USA). RNA-sequencing (seq) was performed with RNA extracted from HCC827EMT clone 4, HCC827EMT clone 10 and two samples of HCC827PAR cells, using Truseq (Illuminia, San Diego, CA, USA) RNA library construction, 100 bp paired-end Illumina HiSeq, post-seq adaptor removal, as well as initial filtering steps were performed by BGI Genomics (Shenzhen, China). Approximately 40 million reads were produced per sample. Transcript quantification from reads were performed using SALMON (RRID:SCR_017036). The two HCC827EMT cell clones 4 and 10 and the two samples of HCC827PAR cells were sequenced separately to account for variability. The paired samples were following analyzed as biological replicates, and hereafter we referred to the collective datasets HCC827EMT and HCC827PAR. The differential expression analysis comparing HCC827EMT to HCC827PAR cells was based on quantified transcript counts using DEseq2 (RRID:SCR_015687). Prior to differential analysis by DEseq2, genes with combined transcript counts lower than 10 were removed from analysis. An exact negative binomial test was used for differential analysis and resulting p values were adjusted for multiple testing using Benjamini-Hochberg correction, as part of the DESeq2 analysis, to generate adjusted P values. Genes with a fold change (FC) in expression >2 and adjusted P values <0.05 were considered differentially expressed genes (DEGs).
For quantitative reverse transcription polymerase chain reaction (RT-qPCR) mRNA was extracted using TRI-Reagent (Sigma-Aldrich). One µg of RNA was converted to cDNA using iScript cDNA Synthesis Kit (Bio-Rad, Hercules, CA, USA). For qPCR 1 µL of cDNA, 0.125 µL of each primer (10 µM), SYBR Green I master mix (Sigma-Aldrich) and water were mixed in a 96-well PCR plate and analysed on a LightCycler 480 (Roche, Basel, Switzerland). Normalisation to the reference genes, ACTB and IPO8, was performed using the X0 method (31). miRNA was extracted using miRNeasy Mini Kit (Qiagen, Venlo, Netherlands) and converted to cDNA using miRCURY LNA RT Kit (Qiagen). For qPCR 3 µL of 60× diluted cDNA, 1 µL of specific Locked Nucleid Acids (LNA) PCR primer mix, water and 2× miRCURY SYBR Green Master Mix (Qiagen) were mixed in a 96 well PCR plate and analysed with LightCycler 480 (Roche). Normalisation to MIR16 expression was done using the X0 method (31). The illustrated RT-qPCR data represents one biological sample analysed in technical triplicates. Independent replicates showed similar results. RT-qPCR primer sequences are available upon request.
H3K36me3 chromatin immunoprecipitation (ChIP)
ChIPs were performed using chromatin prepared from HCC827EMT cell clones 4 and 10 and two samples of HCC827PAR. Chromatin was prepared by crosslinking in media containing 1% formaldehyde for 10 minutes at room temperature. The crosslinking was quenched by the addition of 125 mM glycine and 5 minutes incubation at room temperature. Following washing with ice-cold PBS, cells were collected by spinning at 1,000 ×g for 5 min at 4 °C and lysed in 50 µL ChIP lysis buffer per million cells. Lysates were fragmented by sonication (Diagnode, Liege, Belgium) (5 min cycles of pulses for 30 s on, 30 s off), to an average fragment length of 200–500 bp, adding ice to the water bath between each round. Cellular debris were pelleted and removed by 10 min centrifugation at 20,000 ×g. For immunoprecipitation, 25 µL pre-washed protein A/G magnetic beads (Thermo Fisher Scientific, Waltham, MA, USA) were incubated with anti-H3K36me3 (Abcam, Cambridge, UK, Cat#ab9050, RRID:AB_306966) or rabbit IgG (Thermo Fisher Scientific Cat# 02-6102, RRID:AB_2532938) and incubated for 1 hour at 4 °C with rotation. Antibody-bead complexes were blocked in RIPA buffer containing 1% BSA and incubated for 30 min at 4 °C. Blocked antibody-bead complexes were added to 12 µg of chromatin and incubated overnight with rotation at 4 °C. Bead-bound antigen and antibody complexes were collected and washed followed by elution in TE buffer containing 1% SDS for 1 hour at 65 °C. Following bead removal samples were treated with 40 µg Proteinase K for additional 2 hours at 65 °C. Eluted DNA was extracted using phenol and chloroform followed by EtOH precipitation.
ChIP-seq and differential binding analysis
Input and H3K36me3 ChIP DNA samples from HCC827EMT cell clones 4 and 10 and two samples of HCC827PAR cells were progressed for library construction, 50 bp single end sequencing, post-sequencing adaptor removal, and initial sequence filtering at BGI Genomics (Shenzhen, China). Sequencing was performed with BGISEQ-500 (RRID:SCR_017979) to produce a minimum of 40 million clean reads per sample. Reads were mapped to the genome (hg19) using STAR (RRID:SCR_004463) and PCR duplicates removed using Picard Toolkit (RRID:SCR_006525). Peak calling for quality control analysis was performed using MACS (RRID:SCR_013291) and enrichment quality was evaluated using the ChIPQC package in Bioconductor (RRID:SCR_006442). BAM files were converted using BEDTools (RRID:SCR_006646). HCC827EMT cell clones 4 and 10 and the two samples of HCC827PAR cells were considered biological replicates, and hereafter we referred to the collective datasets HCC827EMT and HCC827PAR.
Differential binding analysis and annotation was performed using DiffReps (RRID:SCR_010873) using a window size of 1,000 bp with a step size of 100 bp. Normalization was carried out using the read count for a particular window over read count across all samples. An exact negative binomial test was used for differential analysis. P values were Benjamini-Hochberg adjusted to correct for multiple testing. Significant differential peaks (adjusted P value <0.05) were annotated to genes using region analysis in DiffReps (RRID:SCR_010873). Kendall rank correlation coefficient analysis for DEGs and H3K36me3 peaks was based on genetic overlaps allowing for 2 kb flanking regions as identified with the matchDatasets function of the shiftR package (RRID:SCR_003005). Each overlap was then internally ranked by their P value and FC using the formula −Log10 (P value) × Log2 (FC).
DNA-methylation profiling and differential methylation analyses
DNA extraction for DNA-methylation analyses of HCC827EMT cell clones 4 and 10, and two samples of HCC827PAR cells, was done with MasterPure DNA purification kit (Epicentre, Madison, WI, USA). Bisulfite treated DNA samples were progressed DNA-methylation EPIC array BeadChip analysis at the German Cancer Research Center (DKFZ). The imported RGChannelSet was normalized using Minfi (RRID:SCR_012830) including the first four principle components, NOOB background correction, and dye normalization to regress out unwanted technical variation. M-values were transformed to beta-values and by employing the champ.filter function from the ChAMP package (RRID:SCR_012891) probes with a detection P>0.01 as well as probes with <3 beads were removed. Probes lacking the DNA sequence cytosine (C) connected by a phosphodiester bond with guanine (G) (CpG) were removed whereas SNP-probes as well as sex chromosome located probes were kept as all samples have the same genetic background. Cross-reactive probes were removed leaving 821,948 probes for downstream analysis. HCC827EMT cell clones 4 and 10 and the two samples of HCC827PAR cells were considered biological replicates, and hereafter we referred to the collective datasets HCC827EMT and HCC827PAR. To identify differentially methylated positions (DMPs), M-values (transformed back from beta-values) were used for linear regression applying the lmFit function from the LIMMA package (RRID:SCR_010943) and P values corrected for multiple testing using the Benjamini-Hochberg algorithm to generate adjusted P values. Differentially methylated regions (DMRs) were identified applying the DMRcate package in Bioconductor (RRID:SCR_006442) with lambda (Gaussian kernel bandwidth) set to 500 and C (scaling factor) set to 5. Significant regions should include a minimum of three probes and have a false discovery rate (FDR) <0.05. Kendall rank correlation coefficient analysis for DEGs and DMPs or DEGs and DMRs depended on genetic overlaps (allowing for 2 kb flanking regions when using the full dataset and 0 kb if assigned to specific annotated genomic regions) identified with the matchDatasets function of the shiftR package (RRID:SCR_003005). Each overlap was then internally ranked by P value and FC using the formula −Log10 (P value) × Log2 (FC).
DNA-methylation analysis of MIR200-family loci with pyrosequencing (Qiagen) of bisulfite treated DNA was performed as previously described (32). Illustrated data represents one biological replicate examined in technical triplicates. Independent biological replicates showed similar results.
CRISPR/Cas9 procedures
sgRNAs targeting ZEB1 (sgRNAs Z1 and Z2), MIR200C and MIR141 (sgRNAs M2 and M1), and FGFR1 (sgRNAs F1 and F2) were designed using the UCSC Genome Browser (33) and the CHOPCHOP tool (34) (RRID:SCR_015723). For control was used a scrambled sgRNA, sgRNA C, with no target homology in the human genome. sgRNA sequences are shown in Table S1. Potential sgRNA off-targets were determined using the CHOP-CHOP tool as well as the CRISPR Targets tool on the UCSC Genome Browser (33,34). Only sgRNAs with potential off-targets containing a minimum of 2 mismatches were used. Furthermore, the Massachusetts Institute of Technology (MIT) specificity score and the Cutting Frequency Determination (CFD) score were used to select sgRNAs generating few potential off-targets and with low probability of being cleaved (35,36). Notably, the potential off-targets with the highest CFD scores needed to be located in intergenic or intron regions for a sgRNA to be selected.
The protocol to obtain CRISPR/Cas9-mediated genetic modifications was previously described (29). Briefly, sgRNA oligonucleotides were cloned in pLentiGuide-Puromycin (Addgene, Cat#52963) (control, FGFR1, ZEB1, and MIR200C sgRNAs) or pLentiGuide-Hygromycin (Addgene, Cat#139462) (control and MIR141 sgRNAs). Correct insertion of the sgRNA oligonucleotides was verified by sequencing using a primer pairs targeting the U6 promoter. Genetic modifications in ZEB1, MIR200C, MIR141, and FGFR1 in HCC827Cas9 cells was performed using lentiviral transduction of sgRNA expressing vectors and cells allowed growth for minimum 14 days with hygromycin or puromycin resistance selection. For analysis of indel and knock out efficiency, genomic DNA was extracted using EZNA tissue DNA kit (Omega Biotek, Norcross, GA, USA). Genomic regions of interest were amplified using 50 ng DNA and HotStarTaq polymerase (Qiagen) or Taq DNA Polymerase Master Mix RED (Ampliqon, Odense, Denmark). DNA sequences of PCR products were analysed for indel and knock out scores using the Synthego ICE analysis tool (https://labs.synthego.com/). Given that high indel and knock-out percentages were obtained for all presented sgRNAs, we subsequently analysed cell populations instead of cell clones to minimize phenotype effects arising from the outgrowth of colonies from single cells. For western blot analysis of CRISPR/Cas9-mediated depletions the following antibodies were used: rabbit anti-ZEB1 (diluted 1/750) (Bethyl Laboratories, Montgomery, TX, USA, Cat# A301-922A, RRID:AB_1524126), rabbit anti-FGFR1 (diluted 1/500) (Cell Signaling Technology, Danvers, MA, USA, Cat# 9740, RRID:AB_11178519), rabbit anti-histone-H3 (diluted 1/10,000) (Abcam Cat# ab1791, RRID:AB_302613), mouse anti-βActin (diluted 1/5,000) (Sigma-Aldrich Cat# A5316, RRID:AB_476743), goat anti-mouse HRP conjugated (diluted 1/10,000) (Agilent, Santa Clara, CA, USA, Cat# P0447, RRID:AB_2617137), and goat anti-rabbit HRP conjugated (diluted 1/10,000) (Agilent Cat# P0448, RRID:AB_2617138).
Cell viability assays
Cell viability as a response to varying concentrations of erlotinib dissolved in DMSO was measured with a colorimetric MTS Cell Proliferation Assay Kit (Abcam, Cat#ab197010) and a Synergy HTX Multi-Mode Reader (Agilent). 5000 cells/well were seeded in 96-Well Plates (Thermo Fisher Scientific, Cat#249935) in 200 µL medium. The two outermost wells of the plate were filled with PBS to minimize evaporation of medium during incubation. After 24 h cells were exposed in duplicates to 0, 0.1, 1 or 5 µM erlotinib diluted in medium containing equal amounts of DMSO, and three control wells without cells containing 200 µL medium was prepared. After 72 h 20 µL MTS reagent was added each well and the cells were incubated for 1 h. The plate was shaken briefly prior to absorbance measurements at 490 nm (MTS formazan product) and 690 nm (background). The mean background corrected 490 nm absorbance for control wells was subtracted from the mean background corrected 490 nm absorbance for duplicate sample wells. The results are presented as change in mean number of viable cells with erlotinib relative to cells not treated with erlotinib. Differences in viability for given concentrations of erlotinib were considered significant if P<0.05 in unpaired two-sample t-tests.
Correlation and Gene Ontology analyses
The Gene Ontology analysis of omics data was performed using the clusterProfiler package (RRID:SCR_016884) to identify enriched ontologies surviving a Benjamini-Hochberg correction (adjusted P values <0.05). Enriched ontologies were reduced to non-redundant ontologies using a semantic similarity cutoff of 0.6.
For retrospective gene-expression analyses we used The Cancer Cell Line Encyclopedia (CCLE, RRID:SCR_013836) subset, Lung_Non-Small-Cell (Lung_NSC), which contains gene-expression data from NSCLC cell lines and The Cancer Genome Atlas (TCGA, RRID:SCR_003193) subset, Lung Adenocarcinoma (LUAD), which contains gene-expression data from dissected NSCLC tumors. Cell line HCC827GR5 (RRID:CVCL_V622) with E-TKI-R due to MET-amplification (37) was RNA-seq analyzed relative to HCC827 cells using available data in the Depmap portal (RRID:SCR_017655). For the Depmap analyses transcript per million (TPM) 0.02 was assigned the minimal detectable RNA-seq derived expression level and for genes with TPM below that a TPM =0.02 imputed to support downstream FC calculations. Expression correlation analyses and gene set enrichment analysis were done as described (38) using the molecular signatures database (MSigDB, RRID:SCR_016863).
Ethical considerations
This study does not involve human subjects. Therefore, the authors have not obtained ethical approval. The study was conducted in accordance with the Declaration of Helsinki (as revised in 2013).
Results
mRNA-seq analysis revealed massive mRNA-expression changes in EMT-E-TKI-R
We previously showed that HCC827 cells can serve as a model for EMT-E-TKI-R not depending on acquisition of secondary EGFR mutations and thereby development of EGFR resistance towards all generations of TKIs (20). In this study, we examined E-TKI-R HCC827 cells with a well-described EMT-mediated mesenchymal phenotype (HCC827EMT cells) relative to parental HCC827 cells displaying an epithelial phenotype (HCC827PAR cells) (20). To reveal genome-wide mRNA expression changes associated with EMT-E-TKI-R, paired-end whole transcriptome sequencing was performed on extracted RNA from the two HCC827EMT cell clones 4 and 10 and two samples of HCC827PAR cells. The two HCC827 EMT cell clones, as well as the two HCC827PAR samples, were sequenced separately to account for biological variability. Differential expression analysis considered the paired samples as biological replicates, and hereafter we referred to the collective datasets HCC827EMT and HCC827PAR. Comparing the RNA-seq derived transcriptomic profile of HCC827EMT relative to HCC827PAR cells, a total of 27,521 unique ensemble genes were identified. Following statistical (P<0.05) and effect (FC >2) filtering, a total of 4,205 DEGs were identified (Figure 1A). Of these 1,972 genes were down-regulated and 2,233 genes were up-regulated in HCC827EMT relative to HCC827PAR cells. The EMT-E-TKI-R down-regulated DEGs included the well characterized epithelial markers CDH1, EPCAM, ESRP1, ESRP2, OVOL1, OVOL2, GRHL1, and GRHL2, and the up-regulated DEGs included mesenchymal markers such as CDH2 (the gene encoding N-Cadherin) and VIM (Figure 1B). HCC827GR5 cells have E-TKI-R as result of MET-amplification (MET-E-TKI-R). None of the EMT marker genes displayed similar expression changes (FC >2) in HCC827GR5 cells supporting the notion that these mRNA expression changes are EMT-E-TKI-R specific (Figure 1B). GO-term enrichment analysis of the EMT-E-TKI-R DEGs, revealed functional annotations related to adhesion, proliferation, and cell differentiation and development to be among the most significantly enriched annotations, and which are functions expected to be associated with EMT (Figure 1C). Collectively, the RNA-seq findings substantiate HCC827EMT cells as a model for EMT-E-TKI-R and illustrates a list of potential EMT driver and marker genes.
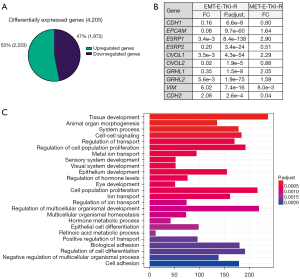
Genome-wide DNA-methylation changes in EMT-E-TKI-R
We determined genome-wide DNA-methylation in HCC827EMT cell clones 4 and 10 and two samples of HCC827PAR cells with 850 k EPIC arrays. Differential DNA-methylation analysis considered the paired samples as biological replicates, and hereafter we referred to the collective datasets HCC827EMT and HCC827PAR. By linear regression a total of 22,162 experiment-wide significant (FDR <0.05) DMPs were identified resulting from EMT-E-TKI-R. Of these, 14,163 were hypermethylated and 7,999 were hypomethylated (Figure 2A upper panel). The DMPs could be clustered into 2,062 significantly (FDR <0.05) DMRs containing a minimum of three CpGs. 1,447 of the DMRs were hypermethylated (Figure 2A lower panel). Stratifying DMPs according to genomic CpG-island context showed tendency of increased association of hypermethylated DMPs with CpG-islands (Figure 2B); 12,364 DMPs could be annotated to genes, with 3,474 DMPs being in the upstream promoter region 0–200 (TSS200) and 200–1,500 (TSS1500) bases upstream the transcription start site (Figure 2C). Distribution of gene-annotated hypermethylated and hypomethylated DMPs were relatively similar (Figure 2C).
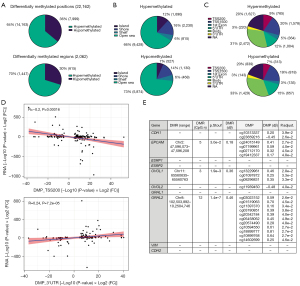
Next, the correlation between DMPs and changes in mRNA-expression was investigated. We matched the significant DMPs to the associated gene allowing for a flanking region of 2 kb. The matched loci were then ranked to produce a composite value of significance and direction of change. In general, correlation between changes in gene annotated DNA-methylation and RNA-expression was weak (Figure S2A,S2B). No significant correlation was present between mRNA expression and DMPs located within the distal promoter (TSS1500) (Figure S2C). However, in agreement with the traditional dogma, hyper-methylation at the core promoter region (TSS200) and the 1st exon negatively correlated with gene-expression (Figure 2D and Figure S2D). For the 5' UTR and gene body no correlation was observed (Figure S2E,S2F). A positive correlation with mRNA-expression changes was observed for DMPs located in the 3' UTR in agreement with previous observations (Figure 2D) (39). We note that all the observed DMP and RNA-expression correlations are perceived as weak to moderate according to standard interpretations (40). Inspection of the epithelial markers CDH1, EPCAM, ESRP1, ESRP2, OVOL1, OVOL2, GRHL1, and GRHL2 revealed DMRs for EPCAM (5 CpGs), OVOL1 (3 CpGs) and GRHL2 (12 CpGs) (Figure 2E). DMPs were present for CDH1, EPCAM, OVOL1, OVOL2, and GRHL2 (Figure 2E). For mesenchymal markers CDH2 and VIM neither DMRs nor DMPs were present (Figure 2E). Collectively, although some gene-specific changes in DNA-methylation correlated with mRNA-expression, EMT-E-TKI-R changes in the genome-wide mRNA-expression profile appears to be mirrored only to minor degree in genome-wide DNA-methylation changes.
Genome-wide H3K36me3-modification changes in EMT-E-TKI-R
Whereas RNA-seq informs concerning the genome-wide mRNA expression level, describes H3K36me3 ChIP-seq genome-wide transcriptional activity (41). H3K36me3 and the corresponding SETD2 methyltransferase were previously described impacting NSCLC EMT through modification of the promoter of CXCL8-gene encoding interleukin-8 (19). To the best of our knowledge, H3K36me3 has not been examined genome-wide in relation to EMT-E-TKI-R. We performed H3K36me3 ChIP-seq using HCC827EMT cell clones 4 and 10 and two samples of HCC827PAR cells. Single-end DNA sequencing of both input and enriched DNA extracted after H3K36me3 antibody ChIP was undertaken. Differential binding analysis considered the paired samples as biological replicates, and hereafter we referred to the collective datasets HCC827EMT and HCC827PAR. Differential binding analysis comparing HCC827EMT to HCC827PAR revealed 41,855 genomic regions significant (adjusted P<0.05). The majority of regions (66%) were located within gene bodies (Figure 3A). The differential regions located within gene bodies were distributed across 6,288 unique genes, of which 2,873 genes contained regions enriched in H3K36me3 and 3,836 genes contained regions depleted for H3K36me3 (Figure 3B); 421 genes contained both H3K36me3 enriched and depleted regions in result of EMT-E-TKI-R (Figure 3B).
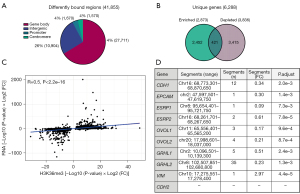
Kendall rank correlation analysis showed positive correlation (R=0.50) between changes in H3K36me3 and mRNA-expression (Figure 3C). This is in agreement with previous studies addressing such correlation (40). In addition, this observation indicates that EMT-E-TKI-R-mediated changes in H3K36me3 has superior correlation with mRNA-expression compared to what was observed for the correlation between DNA-methylation and mRNA-expression. Gene-specific inspection revealed that all the down-regulated epithelial markers CDH1, EPCAM, ESRP1, ESRP2, OVOL1, OVOL2, GRHL1, and GRHL2 contained H3K36me3 depleted regions (Figure 3D). In addition, the up-regulated mesenchymal marker VIM contained an H3K36me3 enriched region (Figure 3D). We note that a described NSCLC EMT-associated decrease in H3K36me3 at the CXCL8 promoter was not identified in HCC827EMT relative to HCC827PAR cells. Moreover, instead of the described increase in CXCL8 mRNA expression with EMT, we observed a 0.49-fold decrease in expression by EMT-E-TKI-R (19). Collectively, the presented ChIP-seq results suggest H3K36me3 to be a broad and highly plastic event better correlated with mRNA-expression relative to what was observed for DNA-methylation in HCC827 EMT-T-TKI-R.
Molecular dissection of gene-expression and epigenetic changes in EMT-E-TKI-R
We aimed using functional genomics data to reveal single genes and pathways taking part in the development of EMT-E-TKI-R. First, we examined how the genes for the six core EMT-TFs ZEB1, ZEB2, SNAI1, SNAI2, TWIST1 and TWIST2 changed in mRNA-expression and epigenetically status in HCC827EMT relative to HCC827PAR cells. We observed increased mRNA-expression levels for both ZEB1 (3.4-fold) and ZEB2 (15.8-fold) (Figure 4A). Despite the higher fold up-regulation for ZEB2, base count analysis of RNA-seq data, semi-quantitative RT-qPCR analyses, and examination of available HCC827 mRNA-expression data from CCLE Lung_NSC, invariably supported a higher level of ZEB1 relative to ZEB2 mRNA-expression in both HCC827PAR and HCC827EMT cells. SNAI1, SNAI2, and TWIST2 displayed a decrease in mRNA-expression levels in HCC827EMT relative to HCC827PAR cells (Figure 4A). TWIST1 had mRNA-expression below the inclusion criteria for DEG analysis and manual data inspection revealed no significant alteration in expression (Figure 4A). The RNA-seq results were in alignment with our previous described RT-qPCR-based analyses of EMT-E-TKI-R in HCC827 cells (20,22). In HCC827GR5 cells with MET-E-TKI-R a concordant decrease in SNAI1 expression was present (Figure 4A). However, in HCC827GR5 cells ZEB1, ZEB2, and TWIST1 mRNA expression was decreased (Figure 4A). We next examined how DNA-methylation and H3K36me3 distributed over the six core EMT-TF genes in HCC827EMT relative to HCC827PAR cells (Figure 4B). For ZEB1 and ZEB2 an increase in H3K36me3 at gene-bodies was present, whereas it was decreased at few positions for SNAI1 and TWIST2 (Figure 4B). The ZEB1 and ZEB2 H3K36me3 results support that the up-regulation at mRNA level, at least partially, is a consequence of increased transcription. For DNA-methylation, the significant changes were in the promoter regions for ZEB2 with three DMPs and two DMRs, and for TWIST1 with three DMPs and one DMR (Figure 4B).
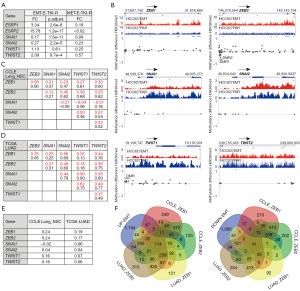
We addressed if the observed up-regulation of both ZEB1 and ZEB2 mRNA reflected a general co-expression pattern in NSCLC cells more evident than other pairwise combinations of EMT-TFs. For this, we performed a Spearman correlation analysis using RNA-seq data existing for 138 NSCLC cell lines in CCLE Lung_NSC. This revealed that the most pronounced expression correlation was between ZEB1 and ZEB2 mRNA (r=0.66) if core EMT-TFs were pairwise examined (Figure 4C). Next, we questioned how genome-wide determined mRNA-expression-correlation coefficients to the six EMT-TFs correlated pairwise. This showed that genes whose mRNA-expression correlated with ZEB1 in general also showed a correlation of expression with ZEB2 (r=0.90) (Figure 4C). We also examined RNA-seq data from NSCLC adenocarcinoma tumors available in TCGA_LUAD (n=517). Correlation analyses again showed highest correlation between ZEB1 and ZEB2 mRNA-expression (r=0.76) (Figure 4D). Analysis of the genome-wide determined mRNA-expression-correlation coefficients to the six EMT-TFs again revealed that genes whose expression correlated to ZEB1 also correlated to expression of ZEB2 (r=0.95) (Figure 4D). We finally performed regression analysis of genome-wide determined EMT-TF mRNA correlation coefficients in NSCLC cells with the RNA-seq determined expression changes in HCC827EMT relative to HCC827PAR cells. This revealed that up-regulated and down-regulated gene-expression profiles correlated among the six core EMT-TFs best with ZEB1 and ZEB2 (Figure 4E). Together, these results support that the observed concomitant mRNA up-regulation of ZEB1 and ZEB2 in HCC827EMT relative to HCC827PAR cells, is due to the two ZEB-genes being in the same regulatory pathway and in general co-regulated in NSCLC cells and tumors.
To identify gene-sets mirroring a ZEB1 and ZEB2 regulatory pathway we identified genes up-regulated in HCC827EMT relative to HCC827PAR cells and simultaneous have positively correlated expression with both ZEB1 and ZEB2 in TCGA_LUAD and CCLE_Lung_NSC datasets. Similar, we identified genes down-regulated in HCC827EMT relative to HCC827PAR cells, which at the same time have negatively correlated expression with both ZEB1 and ZEB2. This revealed gene-sets containing 114 up-regulated and 41 down-regulated genes (Figure 4F and Tables S2,S3). Only 11 and 5 of these genes, respectively, have the same direction of change in mRNA expression in HCC827GR5 cells (FC >2) indicating that most of the genes could have EMT-specific importance (Tables S2,S3). Ontology analyses showed enrichment among the genes in the two gene-sets for pathways related to EMT (Table S4). Moreover, there was enrichment of defined transcriptional regulatory cis-elements (Table S4). We note that among the up-regulated genes, 16 genes possess MIR200-family binding sites in accordance with the described negative regulatory loop between the expression of ZEB1 and ZEB2 EMT-TFs and the MIR200-family (Table S2) (12). For the gene-sets with up-regulated and down-regulated genes, 65 genes and 22 genes, respectively, displayed significant changes in H3K36me3 supporting that the observed alterations in mRNA levels, at least to some degree, reflects a transcriptional alteration (Tables S2,S3). We also notice that DMPs were present for 62 and 17, respectively, of the genes (Tables S2,S3). We anticipate the two identified gene-sets could possess EMT-E-TKI-R diagnostic potential.
ZEB1 depletion impacts both EMT and MET-activation in E-TKI-R
Previous analyses have highlighted the importance of the ZEB-proteins, and in particular ZEB1, for NSCLC EMT including EMT-E-TKI-R (20,25,26,42,43). To substantiate this line of existing evidence, we addressed how CRISPR/Cas9-mediated ZEB1 depletion impacts on EMT-E-TKI-R in HCC827 cells. For CRISPR/Cas9-depletion, two ZEB1 sgRNAs were designed which targets exon 5 encoding the first zinc finger cluster of the ZEB1 protein (Figure S3). The ZEB1 sgRNAs Z1 and Z2 and a scrambled control sgRNA (sgRNA C) were transduced into HCC827Cas9 cells. After puromycin selection the efficiency of ZEB1 depletion was determined (Figure S3). In sgRNA C and ZEB1 sgRNA Z1 and Z2 transduced HCC827Cas9 cells we measured expression of EMT markers and this showed that ZEB1 depletion resulted in decreased VIM and ZEB2 mRNA expression (Figure 5). For ZEB2, the mRNA expression reached background level following ZEB1 depletion. For other examined EMT-markers, we observed no pronounced mRNA expression changes (Figure S3).
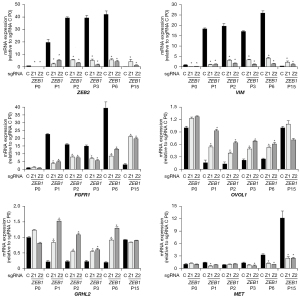
We previously showed that 48 h treatment of HCC827 cells with erlotinib was insufficient to drive ZEB1 up-regulation (30). In accordance, ZEB1 depletion in HCC827Cas9 cells had no effect on proliferation and viability in presence of erlotinib for 72 h (data not shown). We next addressed the involvement of ZEB1 in EMT-E-TKI-R development within a longer timeframe of erlotinib treatment. HCC827Cas9 cells harboring either sgRNA C or sgRNAs targeting ZEB1 were grown in presence of erlotinib. At each cellular passages (P), similar amounts of control and ZEB1-depleted cells surviving erlotinib treatment were progressed for growth in erlotinib containing medium. This procedure was continued until P15 reached after approximately 3 months. By RT-qPCR we examined mRNA-expression of EMT-markers and a candidate set of previously described EGFR bypassing receptor kinases at selected cell passages. Summarized, HCC827Cas9 sgRNA C cells rapidly displayed gene-expression characteristics of EMT similar to what we described for the HCC827EMT cells, e.g., increased VIM, FGFR1, ZEB1, and ZEB2 expression and decreased GRHL2 and OVOL1 expression (Figure 5 and Figure S3) (20). This EMT gene-expression phenotype reached maximum around P3 and P6 and then declined at P15 (Figure 5 and Figure S3). Instead, at P15 there was an increase in MET mRNA expression. This is in accordance with MET-activation at later cell passages being a preferential bypass mechanism for EGFR-signaling-inhibition and that cells with acquired MET-activation, and the associated epithelial gene-expression phenotype, could outgrow the first wave of surviving cells with acquired EMT-characteristics (Figure 5). For HCC827Cas9 cells with ZEB1 depletion, appearance of the EMT-marker mRNA expression profile at early cell passages were less pronounced (Figure 5 and Figure S3). Hence, there was less mRNA expression of mesenchymal markers ZEB2, VIM, and FGFR1 and more mRNA expression of epithelial-markers OVOL1 and GRHL2 (Figure 5). At P15 ZEB1 depletion resulted in relative high FGFR1 mRNA expression but concomitant high VIM and ZEB2 mRNA expression was absent (Figure 5 and Figure S3). Moreover, the high FGFR1 mRNA expression in ZEB1 depleted cells at P15 is associated with less pronounced MET-activation (Figure 5). In conclusion, ZEB1 depletion modulates the acquirement of an EMT-E-TKI-R gene-expression profile in HCC827 cells.
Interaction between MIR200-family, ZEB1 and ZEB2 expression in EMT-E-TKI-R
Previous results have extensively shown an association between MIR200-family expression and NSCLC EMT and the existence of an auto-regulatory loop in NSCLC EMT between expression of the MIR200-family, ZEB1 and ZEB2 (12,27,28). We next addressed if EMT-E-TKI-R in the HCC827 cells also associated with alterations in MIR200-family expression. Both the MIR200B-MIR200A-MIR429 precursor locus at chromosome 1 and the MIR200C-MIR141 precursor locus at chromosome 12 can be transcriptionally down-regulated during EMT in a process involving increased DNA-methylation (12,32). Inspection of the EPIC array DNA-methylation data for MIR200C-MIR141 precursor locus revealed DMPs and a DMR with gain in DNA-methylation in HCC827EMT relative to HCC827PAR cells (Figure 6A). DNA-methylation changes were not evident for the MIR200B-MIR200A-MIR429 precursor locus (Figure 6B). To verify the EPIC array DNA-methylation data which showed preferential gain in DNA-methylation at the MIR200C-MIR141 precursor locus, we performed pyrosequencing analyses. The pyrosequencing results for DNA-methylation were in alignment with the EPIC array DNA-methylation data and as expected according to previous results for acquired MIR200C-MIR141 precursor locus DNA-methylation in NSCLC EMT (Figure 6C) (28,32). We notice an EMT-associated decrease in H3K36me3 for one region in the MIR200C-MIR141 precursor locus in the ChIP-seq dataset following EMT-E-TKI-R, and in alignment, the RNA-seq dataset showed decreased expression of the MIR200C-MIR141 precursor RNA (Figure 6A and data not shown). Quantitative detection of the MIR200-family showed that EMT-E-TKI-R resulted in decreased expression of MIR200C and MIR141 whereas expression of MIR200B and MIR429 was not significantly changed (Figure 6D). We could neither detect expression of MIR200A before nor after EMT-E-TKI-R. We note that MIR200C appeared more expressed than MIR141 in the LNA qPCR assay (Figure 6D). Base count analysis of HCC827 MIR expression data available in CCLE supported more expression of MIR200C compared to MIR141 (data not shown). The expression analyses also supported that DNA-methylation status of the MIR200C-MIR141 precursor locus is inversely correlated with the expression of MIR200C and MIR141 and accordingly is a proxy for expression (27). ZEB1 has a well-described capacity to transcriptional repress the MIR200C-MIR141 precursor locus in a process also involving recruitment of DNMTs (12). This is reflected in the increased DNA-methylation of the MIR200C-MIR141 precursor locus in NSCLC EMT (Figure 6C) (27,32). Since ZEB1 depletion by CRISPR/Cas9 in HCC827Cas9 cells resulted in a delay in mRNA expression of EMT-markers following erlotinib treatment (Figure 5), we questioned if ZEB1 depletion also resulted in less acquirement of DNA-methylation at the MIR200C-MIR141 precursor locus. As expected, less DNA-methylation was present in support for ZEB1 expression being inversely correlated with MIR200C-MIR141 precursor locus DNA-methylation (Figure 6E). Neither MIR200C-MIR141 precursor locus DNA-methylation nor expression changes were observed following MET-E-TKI-R in HCC827 cells, supporting that the acquirement of MIR200C-MIR141 precursor locus DNA-methylation is specifically linked to the EMT (data not shown).
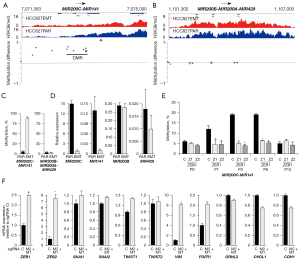
To address if a decrease in functional MIR200C and MIR141 affected EMT-marker gene-expression we performed a CRISPR/Cas9-mediated disruption of MIR200C and MIR141 seed-sequences. We designed sgRNAs targeting genomic cleavage to positions corresponding to the seed-sequences of MIR200C (sgRNA M2) and MIR141 (sgRNA M1) (Figure S4). Subsequently, we performed a simultaneous seed-sequence depletion with sgRNAs M1 and M2 in HCC827Cas9 cells. This resulted in seed-sequence indel scores of 88% for MIR200C, 98% for MIR141, and a combined indel score of 86% (Figure S4). MTS-assays showed that the MIR200C and MIR141 seed-sequence disruptions did not change intrinsic erlotinib sensitivity (data not shown). MIR200C and MIR141 seed-sequence disruption resulted in up-regulation of ZEB1 (2.5-fold) and ZEB2 (7.5-fold), in accordance with both ZEB-genes being targets (Figure 6F) (12). Moreover, the mesenchymal markers VIM and FGFR1 were up-regulated 8-fold and 2-fold, respectively (Figure 6F). For epithelial markers, mRNA down-regulation was not observed (Figure 6F). Altogether, the data are in alignment with previous results for NSCLC showing that MIR200C and MIR141 inhibits expression of mesenchymal markers including ZEB1 and ZEB2 (12,27,28), but also that MIR200C and MIR141 seed-sequence disruption is insufficient to drive the mRNA expression profile present in HCC827EMT relative to HCC827PAR cells.
Receptor kinase expression in EMT-E-TKI-R
We next used the generated omics data to further address potential EGFR-bypassing receptor kinases with altered expression in EMT-E-TKI-R. We included in the analysis 77 genes assigned to be receptor kinases [group 320, The Human Genome Organisation Gene Nomenclature Committee (HGNC)]. Of these, we could extract valid mRNA-expression results for 56 genes which showed that 12 genes were more than 2-fold up-regulated and 13 genes more than 2-fold down-regulated (Figure 7A and Table S5). Among the genes not having significantly altered mRNA-expression we note AXL encoding a well-characterized mediator of EMT (1.8-fold up-regulation) and that EGFR and MET mRNAs were 1.3-fold and 1.9-fold down-regulated (Table S5). However, the modest AXL mRNA up-regulation and EGFR and MET mRNA down-regulation was associated with increased and decreased H3K36me3 for these genes, respectively. For 12 of the 13 receptor kinases having mRNA down-regulated more than 2-fold, a decrease in H3K36me3 was present. Among the 12-up-regulated genes, six genes displayed increase in H3K36me3. DMPs were present in most of the receptor kinase genes displaying changes in mRNA-expression (Table S5). Erythropoietin producing hepatoma (EPH) receptor genes represented three of the up-regulated genes, EPHA5, EPHA6, EPHA4 and four of the down-regulated genes, EPHB2, EPHB3, EPHA2 and EPHA1. The mRNA-expression level for the up-regulated EPH receptors was modest (column base count, Table S5). Up-regulated receptor kinase genes with relative high mRNA-expression level included INSR, FGFR3, and FGFR1, which is in line with previously described EGFR bypass signaling pathways in HCC827 cells (6,20,30). Activation of INSR and the related IGF1R (1.3-fold up-regulated in the RNA-seq dataset) through insulin and IGF1 can confer TKI-R in EGFR-signaling depending cancer cells (30,44). The 3.7-fold up-regulation of INSR mRNA expression was associated with an increase in H3K36me3. The up-regulated RTKs (FC >2) in HCC827EMT relative to HCC827PAR cells were not up-regulated in consequence of the MET-E-TKI-R in HCC827GR5 cells (Table S5).
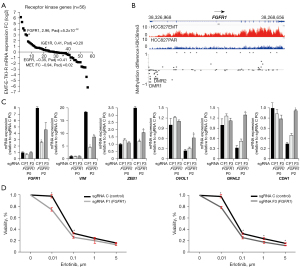
We previously showed FGFR1 mRNA and FGFR1 protein up-regulation in HCC827EMT cells (20-22). A functional involvement of FGFR1 signaling to confer intrinsic and acquired E-TKI-R was shown with pharmacological inhibition, ectopic expression assays, and in a CRISPR-screen (21,23,45,46). We observed a 7.6-fold up-regulation of FGFR1 in HCC827EMT relative to HCC827PAR cells and this was associated with an increase in H3K36me3 (Figure 7B). The H3K36me3 data supports that FGFR1 mRNA up-regulation at least in part is a transcriptional mediated process. Nine DMPs and two DMRs with a decrease in methylation in HCC827EMT relative to HCC827 cells could be detected in the 5'-end of the FGFR1 gene (Figure 7B). For mRNA of the other FGF receptors, we observed an up-regulation at 14-fold of FGFR3 and 1.7-fold of FGFR4 whereas FGFR2 was down-regulated 74-fold (Table S5). None of these mRNA-expression changes associated with significant alterations in H3K36me3 whereas DMPs were present for FGFR2 and FGFR4 (Table S5). Note that despite the more pronounced fold up-regulation of FGFR3 versus FGFR1, the absolute mRNA level was higher for FGFR1 in the HCC827EMT relative to HCC827PAR cells.
To further delineate the function of FGFR1 for EMT-E-TKI-R we performed CRISPR/Cas9-mediated FGFR1 depletion in HCC827 cells with two sgRNAs, F1 and F3, targeting FGFR1 exon 5 (Figure S5). sgRNAs F1 and F3 targets the part of the FGFR1 gene encoding the N-terminal part of the second immunoglobulin (Ig) domain. In HCC827Cas9 cells harboring FGFR1 sgRNAs F1 and F3, the FGFR1 protein levels were reduced but not to an extend reflecting the knock-out efficiency (Figure S5). We note that whereas CRISPR/Cas9 processing with sgRNA F1 resulted in similar knock-out and indel percentages of 94%, sgRNA F3 resulted in a knock-out percentage (71%) outnumbering the indel percentage (96%) (Figure S5). In consequence, sgRNA F3 could in principle result in production of FGFR1 with a dominant-negative function. We observed no dramatic change in EMT-marker gene-expression in absence of erlotinib upon FGFR1 depletion with sgRNA F1 and F3 (Figure 7C). MTS-assays showed tendency of an increased erlotinib sensitivity by FGFR1 depletion with sgRNA F1 and F3 (Figure 7D). Growing HCC827Cas9 cells harboring either control sgRNA C or FGFR1 sgRNA F1 and F3 for two passages in presence of erlotinib, showed that for HCC827Cas9 cells harboring FGFR1 sgRNA F3, expression of mesenchymal markers VIM and ZEB1 was decreased and expression of epithelial markers OVOL1, GRHL2, and CDH1 was increased (Figure 7C). For FGFR1 sgRNA F1 the same effect for mesenchymal marker expression was present, whereas for the epithelial markers only tendency of up-regulation was detected (Figure 7C). This discrepancy could reflect different FGFR1 depletion profiles, as well as off-target profiles, achieved with sgRNAs F1 and F3. However, collectively the presented data are in alignment with at least a supportive function of FGFR1 for mesenchymal cell survival in EMT-E-TKI-R (21-24,43,45,46).
Discussion
In this study, we characterized genome-wide changes in mRNA-expression, H3K36me3 and DNA-methylation in the context of EMT-E-TKI-R in EGFR-mutated HCC827 NSCLC cells. We succeeded identify epigenetic changes associated with changes in mRNA-expression levels. Our analyses revealed only minor correlation between changes in DNA-methylation and mRNA-expression whereas strong correlation existed between changes in H3K36me3 and mRNA-expression. A functional relationship between changes in DNA-methylation and mRNA-expression levels is often assumed, but experimental studies have showed that this is not a general dogma (47,48). This is exemplified by Lin et al. showing that differences in DNA-methylated regions associated with mRNA-expression changes in EMT only constitute a minority of all regions differentially DNA-methylated in EMT (49). In this line, genome-wide analysis showed that methylation status of only 16.6% of promoter located CpGs correlate with the mRNA-expression level (47). Thus, although we do not dispute DNA-methylation changes as being highly correlated to expression of specific genes, we find from our data that the general correlation of all genome-wide DNA-methylation and mRNA-expression changes to be weak to moderate. However, by inspecting a genomic-subset, namely the well-established markers of EMT defined to possess both differential mRNA-expression and DNA-methylation, we find correlation. Beyond the changes in selected EMT markers, we observed massive DNA-methylation changes following EMT-E-TKI-R in HCC827 cells. This is in agreement with other comparisons of DNA-methylation in epithelial-like and mesenchymal-like NSCLC cells (10,14). Addressing DNA-methylation changes in models of induced EMT and accordingly encountering intermediate EMT states revealed that following a short time frame for EMT induction, e.g., 36 hours of TGF-β treatment, no substantial genome-wide changes were present (15). In contrast, a short time-frame of EMT-induction resulted in genome-wide redistribution of H3K9me2, H3K4me3 and H3K36me3 (15). Thus, DNA-methylation changes seem to represent a later epigenetic event than changes in histone modifications, and DNA-methylation may therefore primarily be implicated in the maintenance of the EMT-mediated mesenchymal transcriptional profile rather than the onset of EMT (50). The impact of the time in EMT-induction to allow manifestation of changes in DNA-methylation is underlined by our analyses of EMT-induction in HCC827 cells with StemXvivo [(22); data not shown]. Following 5 days of EMT-induction, mRNA expression changes mirroring EMT are present whereas EPIC array analyses did not reveal massive genome-wide changes in DNA-methylation [(22); data not shown]. Despite the known association between H3K36me3 and mRNA-expression, this is to the best of our knowledge the first NSCLC EMT-E-TKI-R study directly comparing the correlation of changes in DNA-methylation and H3K36me3 to changes in mRNA-expression. McDonald et al. carefully investigated the genome-wide distribution of H3K36me3 in the context of EMT and in agreement with our findings, found massive genome-wide H3K36me3 redistribution (15). This suggests H3K36me3 to be a broad and highly plastic event compared to DNA-methylation, and in agreement with H3K36me3 being directly deposited with the elongating RNA polymerase complex and thus a direct consequence of transcription (51). On top of this, H3K36me3 actively recruits DNMTs to facilitate methylation of intragenic CpGs and thereby can precede DNA-methylation (18,52). In alignment, for EMT markers we find more systematic changes in H3K36me3, than changes in DNA-methylation, that correlate with changes in mRNA expression. We find it an observation of potential diagnostic importance that change in H3K36me3 relative to DNA-methylation correlates better with change in mRNA expression. This could have impact for future delineation of epigenetic-based molecular markers for gene-expression changes associated with EMT-E-TKI-R in NSCLC. For one example, in the era of molecular cancer diagnostics based on liquid biopsies, achieving circulating cell-free (cf) tumor RNA can be problematic. A proxy for achieving tumor gene-expression information is analysis of epigenetic markers present at the cf DNA with origin from the tumor cells and the corresponding cf-nucleosomes. Whereas cfDNA-methylation has been widely addressed, recent published results have also revealed that quantitative analyses of histone modifications using cfChIP possess a cancer diagnostic potential (53-55). The hereby-presented data points that quantifying H3K36me3 at cf nucleosomes from the basis of liquid biopsies could extend the number of genes for which it will be possible to derive tumor gene-expression information beyond what will be possible with DNA-methylation analyses. For lung cancer patients, this principally could allow improved determination of onset of EMT-E-TKI-R and accordingly a more timely treatment protocol.
E-TKI-R in NSCLC patients is often observed being a result of genetic changes (1). EGFR secondary mutations is the primarily cause and MET bypass signaling caused by amplification of the MET-gene second leading. Novel generation TKI’s change the resistance pattern through their action also on EGFR with secondary resistance mutations, but resistance will inevitably develop. With the persisting obstacle of acquired resistance, there is a need to understand the resistance mechanism and dynamics of development to improve treatment strategies. EMT is a known mechanism of E-TKI-R in NSCLC, but EMT is primarily documented in studies exploring E-TKI-R in cell lines (7,20,43,56,57). The cell line-based studies of EMT-E-TKI-R have pointed to that resistant cells present large variation in the expression of EMT markers. The EMT marker expression seems to depend on both the resistance development protocol in terms of TKI concentration and time of treatment, as well as the genetic background of cell lines. This variability can be explained by differences in cell utilization of core EMT-TFs and accordingly potential developing a spectrum of intermediary and endpoint EMT stages with varying expression of epithelial and mesenchymal markers (58,59). In HCC827 cells, MET-amplification and EMT are the best described mechanisms to confer E-TKI-R (20,30). FGFR1 bypass signaling is described to be involved in EMT-E-TKI-R (21-24,43,45,46). FGFR1 up-regulation does not appear in advance of EMT and does not drive EMT, but is a concomitant event in EMT (22). MTS-assays only showed tendency of increased erlotinib sensitivity upon FGFR1 depletion. This could support that FGFR1 bypass signaling only has minor impact for cell viability at an immediate EGFR TKI exposure and that the relatively low FGFR1 expression is insufficient to bypass EGFR-signaling. Following two cell passages in presence of the TKI erlotinib, FGFR1 expression is induced, and under these conditions FGFR1 depletion resulted in presence of cell populations with less expression of mesenchymal markers. This suggests that FGFR1 bypass signaling, at this later stage of erlotinib exposure, contributes survival benefit to cells undergoing EMT. In alignment, we note that Raoof et al. in a whole-genome CRISPR-screening, identified FGFR1 as the top target to have impact for EMT-E-TKI-R in NSCLC cells harboring EGFR-mutations (23). Changes in receptor kinase mRNA-expression upon EMT-E-TKI-R was not restricted to FGFR1 in HCC827 cells. We find it in particular interesting that upon EMT-E-TKI-R three of the up-regulated genes, EPHA5, EPHA6, EPHA4 and four of the down-regulated genes, EPHB2, EPHB3, EPHA2 and EPHA1 were EPH receptor genes. EPH receptors were previously assigned important functions in EMT, e.g., up-regulation of EPHA2 in HCC827 cells for bypass signaling in E-TKI-R (60,61). Whereas such EPHA2 up-regulation was observed in HCC827GR5 cells (61), we here observed EPHA2 down-regulation in HCC827EMT relative to HCC827PAR cells. We note that a previous examination of ZEB1-driven EMT-associated gene-expression in NSCLC cells showed that EPHA1 was a candidate ZEB1-repressed gene (25). This is in alignment with our observations for EPHA1 mRNA expression in HCC827EMT relative to HCC827PAR cells. The function in EMT-E-TKI-R for EPH receptors either alone or through their interaction with RTKs needs further clarification.
An important question to be raised is whether EMT-E-TKI-R reflects a drug-tolerant state during resistance development which confers sufficient cell survival to allow later development of more stable genetically based resistance mechanism such as MET-activation through amplification or EGFR secondary mutations (62). Our data supports that ZEB1 in HCC827 cells is functionally involved in mediating EMT-E-TKI-R, but on top of this, more surprisingly, ZEB1 appears important for allowing generation of HCC827 cells with acquired MET-activation. Despite not formally shown, our data supports the model that ZEB1-conferred EMT-E-TKI-R is a transient state allowing cell survival in sufficient time under the selective pressure by TKI for superior resistance properties to arise, such as MET-E-TKI-R (62). Such a model could also explain the discrepancy between the commonly observed EMT-E-TKI-R in cell culture models and E-TKI-R through genetic alterations in patient samples. In patients, an increased selective pressure and/or later sampling will preferentially allow identification of the population of cancer cells that have obtained most optimal resistance properties, e.g., MET-activation or EGFR secondary mutations. At P15 following erlotinib-treatment, HCC827Cas9 cells with ZEB1 depletion possesses relative high FGFR1, without concomitant high ZEB2 and VIM, mRNA expression level, but instead presented with increased TWIST1 mRNA expression (Figure 5 and Figure S3). If such cells display an EMT-morphology awaits elucidation.
Our data did not reveal core EMT-TFs SNAI1, SNAI2, TWIST1 and TWIST2 directly have impact for the gene-expression and epigenetic profiles observed in HCC827EMT cells. This does not rule out that these EMT-TFs have functional importance for intermediary EMT stages, a notion that is supported by the observed change in expression of the EMT-TFs during the progression of EMT in HCC827 cells (20,30). To this end, it should be noted that DNA-methylation changes were observed for TWIST1 in HCC827EMT cells which could mirror a previous change in expression along the EMT process. TWIST1 was previously described as a mediator of EMT-E-TKI-R in EGFR-mutated NSCLC cells (63) and TWIST1 can functionally cooperate with ZEB1 (11). However, the presented omics and CRISPR/Cas9 derived data supports an in particular important function for ZEB1 among the EMT-TFs to confer EMT-E-TKI-R. That ZEB1 depletion resulted in a mRNA-expression phenotype related to EMT-E-TKI-R, corroborates that ZEB2 per se cannot functional substitute for ZEB1 (11). However, our data show that ZEB1 and ZEB2 invariably have a correlated mRNA-expression pattern, exemplified with the used HCC827 cells, as well across TCGA tumor samples and NSCLC cell lines. Moreover, we previously identified ZEB2 up-regulation in consequence of forced up-regulation of ZEB1 and here show ZEB2 down-regulation in consequence of ZEB1 depletion (Figure 5, Figure S3) (22). Since both ZEB1 and ZEB2 genes have gain of H3K36me3 in HCC827EMT relative to HCC827PAR cells, their mRNA up-regulation can reflect concordant transcriptional regulation. On top of this, depletion of MIR200C and MIR141 functionality by indel introduction in the seed-sequences with CRISPR/Cas9 resulted in increased mRNA levels for both ZEB1 and ZEB2 indicating concordant regulation also at the post-transcriptional level. Thus, our data further supports a connected regulation of ZEB1 and ZEB2 mRNA levels. It could be of importance that we hereby confirmed a more pronounced change in DNA-methylation for ZEB2 compared to ZEB1 upon EMT-E-TKI-R in NSCLC cells (14). Thus, measurement of ZEB2 DNA-methylation status is a potential diagnostic proxy for both tumor cell ZEB1 and ZEB2 mRNA expression in relation to EMT-E-TKI-R. We focused our analyses on ZEB1 given that inspection of RNA-seq data indicated a higher mRNA-expression level than ZEB2 and the similar expression results of the two genes. Further analyses are required to elucidate if ZEB1 and ZEB2 have common targets and if they have hierarchical or parallel functions as EMT-TFs to mediate EMT-E-TKI-R in NSCLC cells (11). From the basis of the observations of ZEB1 and ZEB2 co-regulation, we identified lists of genes mRNA expression correlated with both ZEB1 and ZEB2 in NSCLC cell lines and dissected tumor samples, and in addition having mRNA expression changes upon EMT-E-TKI-R in HCC827 cells. These genes could be potential direct regulatory targets for the ZEB1 and ZEB2 transcription factors or be regulated in similar pathways as ZEB1 and ZEB2. The gene-lists included 114 up-regulated and 41 down-regulated genes and the majority also have epigenetic changes. However, we note several examples of genes where changed mRNA-expression is only associated with either H3K36me3 or DNA-methylation changes, as well as examples of genes without associated epigenetic changes. For the latter it could be interesting, e.g., LOX, OSTM1, SERINC1, and FHL1 possess MIR200-family binding sites making these genes candidates for regulation through a post-transcriptional miRNA-based mechanism. The list for down-regulated genes included several well-described epithelial marker genes such as ESRP1, GRHL2, OVOL2, and EPCAM, but in addition also not well characterized candidates. The list of up-regulated genes included the well-established mesenchymal markers FGFR1 and VIM. We find the latter list of genes to have particular importance, given that whereas the list of well-characterized epithelial markers is long, it is sparser with well-accepted mesenchymal markers for EMT in NSCLC. In a CRISPR/Cas9-based study addressing the importance of ZEB1 in NSCLC EMT related to immune escape the CD70 gene was identified being activated by ZEB1 and as result of EMT in NSCLC cells (42). However, our RNA-seq data showed a 0.52-fold decrease in CD70 expression in HCC827EMT relative to HCC827PAR cells. Along with the above described discrepancy in EMT expression profile of CXCL8, this importantly exemplifies that EMT-associated gene-expression changes in NSCLC not necessarily are coherent, but depends on the exact EMT-model analyzed (19,25). We are confident that the continuous identification of gene-lists associated with EMT-E-TKI-R in NSCLC using well-defined cellular models will act supportive to already present multi-omics identified gene-sets for future improved understanding and diagnosis of EMT-E-TKI-R (64-66).
Conclusions
The results from the genome-wide epigenetic and RNA-expression changes in HCC827EMT relative to HCC827PAR cells raise awareness to investigate H3K36me3 for being an EMT-marker in clinical samples. Additionally, this study further underlines the role of the MIR200C/MIR141-ZEB1/ZEB2-FGFR1 axis in acquired EMT-E-TKI-R. These findings provide increased insight into acquirement of EMT-E-TKI-R and raise attention of diagnostic and therapeutic strategies to prevent EMT-E-TKI-R.
Acknowledgments
Funding: The project was supported by Kræftfonden, Dagmar Marshalls Mindelegat, Fabrikant Einar Willumsens Mindelegat, Marie og Børge Kroghs Fond, P. A. Messerschmidt og Hustrus Fond, Thora og Viggo Grove’s Mindelegat, Familien Erichsens Familiefond, Axel Muusfeldts Fond, Direktør Emil C. Hertz og Hustru Inger Hertz’ Fond, Købmand Sven Hansen og Hustru Ina Hansens Fond, Peetz legat, Frimodt-Heineke Fonden, Else og Mogens Wedell-Wedellsborgs Fond, and Harboefonden.
Footnote
Reporting Checklist: The authors have completed the MDAR reporting checklist. Available at https://tlcr.amegroups.com/article/view/10.21037/tlcr-22-507/rc
Data Sharing Statement: Available at https://tlcr.amegroups.com/article/view/10.21037/tlcr-22-507/dss
Peer Review File: Available at https://tlcr.amegroups.com/article/view/10.21037/tlcr-22-507/prf
Conflicts of Interest: All authors have completed the ICMJE uniform disclosure form (available at https://tlcr.amegroups.com/article/view/10.21037/tlcr-22-507/coif). The authors have no conflicts of interest to declare.
Ethical Statement: The authors are accountable for all aspects of the work in ensuring that questions related to the accuracy or integrity of any part of the work are appropriately investigated and resolved. This study does not involve human subjects. Therefore, the authors have not obtained ethical approval. The study was conducted in accordance with the Declaration of Helsinki (as revised in 2013).
Open Access Statement: This is an Open Access article distributed in accordance with the Creative Commons Attribution-NonCommercial-NoDerivs 4.0 International License (CC BY-NC-ND 4.0), which permits the non-commercial replication and distribution of the article with the strict proviso that no changes or edits are made and the original work is properly cited (including links to both the formal publication through the relevant DOI and the license). See: https://creativecommons.org/licenses/by-nc-nd/4.0/.
References
- Thai AA, Solomon BJ, Sequist LV, et al. Lung cancer. Lancet 2021;398:535-54. [Crossref] [PubMed]
- Franceschini D, Rossi S, Loi M, et al. Lung cancer management: monitoring and treating resistance development in third-generation EGFR TKIs. Expert Rev Anticancer Ther 2020;20:743-53. [Crossref] [PubMed]
- Westover D, Zugazagoitia J, Cho BC, et al. Mechanisms of acquired resistance to first- and second-generation EGFR tyrosine kinase inhibitors. Ann Oncol 2018;29:i10-9. [Crossref] [PubMed]
- Wu L, Ke L, Zhang Z, et al. Development of EGFR TKIs and Options to Manage Resistance of Third-Generation EGFR TKI Osimertinib: Conventional Ways and Immune Checkpoint Inhibitors. Front Oncol 2020;10:602762. [Crossref] [PubMed]
- Lim SM, Syn NL, Cho BC, et al. Acquired resistance to EGFR targeted therapy in non-small cell lung cancer: Mechanisms and therapeutic strategies. Cancer Treat Rev 2018;65:1-10. [Crossref] [PubMed]
- Jakobsen KR, Demuth C, Sorensen BS, et al. The role of epithelial to mesenchymal transition in resistance to epidermal growth factor receptor tyrosine kinase inhibitors in non-small cell lung cancer. Transl Lung Cancer Res 2016;5:172-82. [Crossref] [PubMed]
- Zhu X, Chen L, Liu L, et al. EMT-Mediated Acquired EGFR-TKI Resistance in NSCLC: Mechanisms and Strategies. Front Oncol 2019;9:1044. [Crossref] [PubMed]
- Bakir B, Chiarella AM, Pitarresi JR, et al. EMT, MET, Plasticity, and Tumor Metastasis. Trends Cell Biol Trends Cell Biol 2020;30:764-76. [Crossref] [PubMed]
- Brabletz S, Schuhwerk H, Brabletz T, et al. Dynamic EMT: a multi-tool for tumor progression. EMBO J 2021;40:e108647. [Crossref] [PubMed]
- O'Leary K, Shia A, Schmid P. Epigenetic Regulation of EMT in Non-Small Cell Lung Cancer. Curr Cancer Drug Targets 2018;18:89-96. [Crossref] [PubMed]
- Stemmler MP, Eccles RL, Brabletz S, et al. Non-redundant functions of EMT transcription factors. Nat Cell Biol 2019;21:102-12. [Crossref] [PubMed]
- Liu C, Hu W, Li LL, et al. Roles of miR-200 family members in lung cancer: more than tumor suppressors. Future Oncol 2018;14:2875-86. [Crossref] [PubMed]
- Cavallari I, Ciccarese F, Sharova E, et al. The miR-200 Family of microRNAs: Fine Tuners of Epithelial-Mesenchymal Transition and Circulating Cancer Biomarkers. Cancers (Basel) 2021;13:5874. [Crossref] [PubMed]
- Walter K, Holcomb T, Januario T, et al. DNA methylation profiling defines clinically relevant biological subsets of non-small cell lung cancer. Clin Cancer Res 2012;18:2360-73. [Crossref] [PubMed]
- McDonald OG, Wu H, Timp W, et al. Genome-scale epigenetic reprogramming during epithelial-to-mesenchymal transition. Nat Struct Mol Biol 2011;18:867-74. [Crossref] [PubMed]
- Huang C, Zhu B. Roles of H3K36-specific histone methyltransferases in transcription: antagonizing silencing and safeguarding transcription fidelity. Biophys Rep 2018;4:170-7. [Crossref] [PubMed]
- Chen R, Zhao WQ, Fang C, et al. Histone methyltransferase SETD2: a potential tumor suppressor in solid cancers. J Cancer 2020;11:3349-56. [Crossref] [PubMed]
- Neri F, Rapelli S, Krepelova A, et al. Intragenic DNA methylation prevents spurious transcription initiation. Nature 2017;543:72-7. [Crossref] [PubMed]
- Yang X, Chen R, Chen Y, et al. Methyltransferase SETD2 inhibits tumor growth and metastasis via STAT1-IL-8 signaling-mediated epithelial-mesenchymal transition in lung adenocarcinoma. Cancer Sci 2022;113:1195-207. [Crossref] [PubMed]
- Jakobsen KR, Demuth C, Madsen AT, et al. MET amplification and epithelial-to-mesenchymal transition exist as parallel resistance mechanisms in erlotinib-resistant, EGFR-mutated, NSCLC HCC827 cells. Oncogenesis 2017;6:e307. [Crossref] [PubMed]
- Gammelgaard KR, Vad-Nielsen J, Clement MS, et al. Up-Regulated FGFR1 Expression as a Mediator of Intrinsic TKI Resistance in EGFR-Mutated NSCLC. Transl Oncol 2019;12:432-40. [Crossref] [PubMed]
- Vad-Nielsen J, Gammelgaard KR, Daugaard TF, et al. Cause-and-Effect relationship between FGFR1 expression and epithelial-mesenchymal transition in EGFR-mutated non-small cell lung cancer cells. Lung Cancer 2019;132:132-40. [Crossref] [PubMed]
- Raoof S, Mulford IJ, Frisco-Cabanos H, et al. Targeting FGFR overcomes EMT-mediated resistance in EGFR mutant non-small cell lung cancer. Oncogene 2019;38:6399-413. [Crossref] [PubMed]
- Terp MG, Jacobsen K, Molina MA, et al. Combined FGFR and Akt pathway inhibition abrogates growth of FGFR1 overexpressing EGFR-TKI-resistant NSCLC cells. NPJ Precis Oncol 2021;5:65. [Crossref] [PubMed]
- Larsen JE, Nathan V, Osborne JK, et al. ZEB1 drives epithelial-to-mesenchymal transition in lung cancer. J Clin Invest 2016;126:3219-35. [Crossref] [PubMed]
- Yoshida T, Song L, Bai Y, et al. ZEB1 Mediates Acquired Resistance to the Epidermal Growth Factor Receptor-Tyrosine Kinase Inhibitors in Non-Small Cell Lung Cancer. PLoS One 2016;11:e0147344. [Crossref] [PubMed]
- Liu PL, Liu WL, Chang JM, et al. MicroRNA-200c inhibits epithelial-mesenchymal transition, invasion, and migration of lung cancer by targeting HMGB1. PLoS One 2017;12:e0180844. [Crossref] [PubMed]
- Sato H, Shien K, Tomida S, et al. Targeting the miR-200c/LIN28B axis in acquired EGFR-TKI resistance non-small cell lung cancer cells harboring EMT features. Sci Rep 2017;7:40847. [Crossref] [PubMed]
- Dietz LL, Furman NT, Larsen TV, et al. An Extended PD-L2 Cytoplasmic Domain Results From Alternative Splicing in NSCLC Cells. J Immunother 2022;45:379-88. [Crossref] [PubMed]
- Hussmann D, Madsen AT, Jakobsen KR, et al. IGF1R depletion facilitates MET-amplification as mechanism of acquired resistance to erlotinib in HCC827 NSCLC cells. Oncotarget 2017;8:33300-15. [Crossref] [PubMed]
- Thomsen R, Sølvsten CA, Linnet TE, et al. Analysis of qPCR data by converting exponentially related Ct values into linearly related X0 values. J Bioinform Comput Biol 2010;8:885-900. [Crossref] [PubMed]
- Hur K, Toiyama Y, Takahashi M, et al. MicroRNA-200c modulates epithelial-to-mesenchymal transition (EMT) in human colorectal cancer metastasis. Gut 2013;62:1315-26. [Crossref] [PubMed]
- Haeussler M, Zweig AS, Tyner C, et al. The UCSC Genome Browser database: 2019 update. Nucleic Acids Res 2019;47:D853-8. [Crossref] [PubMed]
- Labun K, Krause M, Torres Cleuren Y, et al. CRISPR Genome Editing Made Easy Through the CHOPCHOP Website. Curr Protoc 2021;1:e46. [Crossref] [PubMed]
- Doench JG, Fusi N, Sullender M, et al. Optimized sgRNA design to maximize activity and minimize off-target effects of CRISPR-Cas9. Nat Biotechnol 2016;34:184-91. [Crossref] [PubMed]
- Haeussler M, Schönig K, Eckert H, et al. Evaluation of off-target and on-target scoring algorithms and integration into the guide RNA selection tool CRISPOR. Genome Biol 2016;17:148. [Crossref] [PubMed]
- Engelman JA, Zejnullahu K, Mitsudomi T, et al. MET amplification leads to gefitinib resistance in lung cancer by activating ERBB3 signaling. Science 2007;316:1039-43. [Crossref] [PubMed]
- Larsen TV, Hussmann D, Nielsen AL. PD-L1 and PD-L2 expression correlated genes in non-small-cell lung cancer. Cancer Commun (Lond) 2019;39:30. [Crossref] [PubMed]
- Luo R, Bai C, Yang L, et al. DNA methylation subpatterns at distinct regulatory regions in human early embryos. Open Biol 2018;8:180131. [Crossref] [PubMed]
- Mukaka MM. Statistics corner: A guide to appropriate use of correlation coefficient in medical research. Malawi Med J 2012;24:69-71. [PubMed]
- Xiao C, Fan T, Tian H, et al. H3K36 trimethylation-mediated biological functions in cancer. Clin Epigenetics 2021;13:199. [Crossref] [PubMed]
- Ortiz-Cuaran S, Swalduz A, Foy JP, et al. Epithelial-to-mesenchymal transition promotes immune escape by inducing CD70 in non-small cell lung cancer. Eur J Cancer 2022;169:106-22. [Crossref] [PubMed]
- Ware KE, Hinz TK, Kleczko E, et al. A mechanism of resistance to gefitinib mediated by cellular reprogramming and the acquisition of an FGF2-FGFR1 autocrine growth loop. Oncogenesis 2013;2:e39. [Crossref] [PubMed]
- Zhou J, Wang J, Zeng Y, et al. Implication of epithelial-mesenchymal transition in IGF1R-induced resistance to EGFR-TKIs in advanced non-small cell lung cancer. Oncotarget 2015;6:44332-45. [Crossref] [PubMed]
- Azuma K, Kawahara A, Sonoda K, et al. FGFR1 activation is an escape mechanism in human lung cancer cells resistant to afatinib, a pan-EGFR family kinase inhibitor. Oncotarget 2014;5:5908-19. [Crossref] [PubMed]
- Terai H, Soejima K, Yasuda H, et al. Activation of the FGF2-FGFR1 autocrine pathway: a novel mechanism of acquired resistance to gefitinib in NSCLC. Mol Cancer Res 2013;11:759-67. [Crossref] [PubMed]
- Medvedeva YA, Khamis AM, Kulakovskiy IV, et al. Effects of cytosine methylation on transcription factor binding sites. BMC Genomics 2014;15:119. [Crossref] [PubMed]
- Maeder ML, Angstman JF, Richardson ME, et al. Targeted DNA demethylation and activation of endogenous genes using programmable TALE-TET1 fusion proteins. Nat Biotechnol 2013;31:1137-42. [Crossref] [PubMed]
- Lin SH, Wang J, Saintigny P, et al. Genes suppressed by DNA methylation in non-small cell lung cancer reveal the epigenetics of epithelial-mesenchymal transition. BMC Genomics 2014;15:1079. [Crossref] [PubMed]
- Rose NR, Klose RJ. Understanding the relationship between DNA methylation and histone lysine methylation. Biochim Biophys Acta 2014;1839:1362-72. [Crossref] [PubMed]
- Venkatesh S, Workman JL. Set2 mediated H3 lysine 36 methylation: regulation of transcription elongation and implications in organismal development. Wiley Interdiscip Rev Dev Biol 2013;2:685-700. [Crossref] [PubMed]
- Rondelet G, Dal Maso T, Willems L, et al. Structural basis for recognition of histone H3K36me3 nucleosome by human de novo DNA methyltransferases 3A and 3B. J Struct Biol 2016;194:357-67. [Crossref] [PubMed]
- Vad-Nielsen J, Meldgaard P, Sorensen BS, et al. Cell-free Chromatin Immunoprecipitation (cfChIP) from blood plasma can determine gene-expression in tumors from non-small-cell lung cancer patients. Lung Cancer 2020;147:244-51. [Crossref] [PubMed]
- Månsson CT, Vad-Nielsen J, Meldgaard P, et al. EGFR transcription in non-small-cell lung cancer tumours can be revealed in ctDNA by cell-free chromatin immunoprecipitation (cfChIP). Mol Oncol 2021;15:2868-76. [Crossref] [PubMed]
- Sadeh R, Sharkia I, Fialkoff G, et al. ChIP-seq of plasma cell-free nucleosomes identifies gene expression programs of the cells of origin. Nat Biotechnol 2021;39:586-98. [Crossref] [PubMed]
- Thomson S, Buck E, Petti F, et al. Epithelial to mesenchymal transition is a determinant of sensitivity of non-small-cell lung carcinoma cell lines and xenografts to epidermal growth factor receptor inhibition. Cancer Res 2005;65:9455-62. [Crossref] [PubMed]
- Rastogi I, Rajanna S, Webb A, et al. Mechanism of c-Met and EGFR tyrosine kinase inhibitor resistance through epithelial mesenchymal transition in non-small cell lung cancer. Biochem Biophys Res Commun 2016;477:937-44. [Crossref] [PubMed]
- Nieto MA, Huang RY, Jackson RA, et al. EMT: 2016. Cell 2016;166:21-45. [Crossref] [PubMed]
- Karacosta LG, Anchang B, Ignatiadis N, et al. Mapping lung cancer epithelial-mesenchymal transition states and trajectories with single-cell resolution. Nat Commun 2019;10:5587. [Crossref] [PubMed]
- Li RX, Chen ZH, Chen ZK. The role of EPH receptors in cancer-related epithelial-mesenchymal transition. Chin J Cancer 2014;33:231-40. [Crossref] [PubMed]
- Koch H, Busto ME, Kramer K, et al. Chemical Proteomics Uncovers EPHA2 as a Mechanism of Acquired Resistance to Small Molecule EGFR Kinase Inhibition. J Proteome Res 2015;14:2617-25. [Crossref] [PubMed]
- Clement MS, Gammelgaard KR, Nielsen AL, et al. Epithelial-to-mesenchymal transition is a resistance mechanism to sequential MET-TKI treatment of MET-amplified EGFR-TKI resistant non-small cell lung cancer cells. Transl Lung Cancer Res 2020;9:1904-14. [Crossref] [PubMed]
- Yochum ZA, Cades J, Wang H, et al. Targeting the EMT transcription factor TWIST1 overcomes resistance to EGFR inhibitors in EGFR-mutant non-small-cell lung cancer. Oncogene 2019;38:656-70. [Crossref] [PubMed]
- Sitthideatphaiboon P, Teerapakpinyo C, Korphaisarn K, et al. Co-occurrence CDK4/6 amplification serves as biomarkers of de novo EGFR TKI resistance in sensitizing EGFR mutation non-small cell lung cancer. Sci Rep 2022;12:2167. [Crossref] [PubMed]
- Wang TH, Wu CC, Huang KY, et al. Integrated Omics Analysis of Non-Small-Cell Lung Cancer Cells Harboring the EGFR C797S Mutation Reveals the Potential of AXL as a Novel Therapeutic Target in TKI-Resistant Lung Cancer. Cancers (Basel) 2020;13:111. [Crossref] [PubMed]
- Wang Z, Zhang L, Xu W, et al. The Multi-Omics Analysis of Key Genes Regulating EGFR-TKI Resistance, Immune Infiltration, SCLC Transformation in EGFR-Mutant NSCLC. J Inflamm Res 2022;15:649-67. [Crossref] [PubMed]