Circulating immune response proteins predict the outcome following disease progression of osimertinib treated epidermal growth factor receptor-positive non-small cell lung cancer patients
Introduction
Lung cancer is one of the most frequent types of cancer worldwide and the type of cancer that causes most deaths (1,2).
Ten to 15 percent of non-small cell lung cancer (NSCLC) adenocarcinomas in Caucasians are caused by activating mutations (e.g., EGFR-L858R and EGFR-ex19 deletion) in the ATP-binding pocket of the epidermal growth factor receptor (EGFR) (3,4). These mutations drive the cancer development (5), making it sensitive to EGFR-targeting tyrosine kinase inhibitors (EGFR-TKI), which is a current standard of care for these patients with advanced stage cancer (6-8). Unfortunately, resistance toward the drugs will develop over time, in most patients this is seen as the T790M mutation for first/second generation EGFR-TKIs (9-11). This resistance mutation has been overcome by the new third generation EGFR-TKI, osimertinib, which is currently the first line of treatment in many countries (12-14). However, most patients eventually develop osimertinib resistance as well (15). Compared to first/second generation EGFR-TKI’s, in which EGFR mutations are the most common form of resistance, osimertinib resistance can be mediated by new EGFR mutations (e.g., EGFR-C797S) but more commonly through bypass mutations, such as ERBB2 and MET amplifications, as well as PIK3CA, APC, NF1, and BRAF mutations (16-18). Because of this, osimertinib-resistant patients represent a genetically heterogeneous group (19), highlighting the need for ways to monitor tumor development in patients during their treatments.
Biomarkers found in the bloodstream, which portray the molecular state of a specific cancer, have been used for many years. Using cell-free DNA in liquid biopsies to monitor known oncogenic drivers, such as EGFR, ALK, and KRAS mutations, with quantitative real time polymerase chain reaction (qPCR) and next-generation sequencing (NGS) is an effective and acknowledged method in clinical practice and is often used as a supplement to biopsies (20-22). However, other types of biomarkers, such as circulating proteins, could provide additional information and be easier and cheaper to use in clinical practice.
Protein biomarkers in lung cancer have been investigated for many years. Carcinoembryonic antigen (CEA) is a well-known biomarker in lung cancer (23). Neuron specific enolase (NSE) and pro gastrin releasing peptide (proGRP) are biomarkers that have been suggested for small cell lung cancer (SCLC) and could be important as biomarkers for the resistance mechanism toward osimertinib, in which EGFR-positive NSCLC transforms to SCLC (24-27).
More specific and precise biomarkers, such as NSE and proGRP that indicate specific resistance mechanisms, would be of great value in clinical practice. Therefore, we wanted to discover novel biomarkers by investigating circulating proteins in the bloodstream at different times during treatment of EGFR-mutated NSCLC patients with osimertinib. Two time points in the patient’s course of treatment were used for further analysis: the time of response to osimertinib and the time of progressive disease (PD). The response blood sample was chosen over the baseline blood sample as we wanted to compare the situation with and without effect of osimertinib. Plasma samples from response and PD were analyzed using a proximity extension assay (PEA), applying the Olink target 96 Oncology panel II. We present the following article in accordance with the MDAR reporting checklist (available at https://tlcr.amegroups.com/article/view/10.21037/tlcr-22-577/rc).
Methods
Patients
The patients included for this retrospective study’s cohort were all enrolled in a prospective, observational, multicenter study of advanced stage lung cancer patients, with a verified EGFR mutation, during which blood samples were taken consecutively during various treatment lines (ID NCT02284633). The study was conducted at the Department of Oncology, Aarhus University hospital, and included patients from four oncology departments in the western part of Denmark between August 2014 and December 2018. The study was conducted according to criteria’s set by the Declaration of Helsinki (as revised in 2013) and was approved by the National Committee on Health Ethics (No. 1-10-72-83-14) and the Danish Data Protection Agency (No. 1-16-02-431-14). All subjects provided written informed consent before inclusion.
Eighty-five patients from the prospective study received treatment with osimertinib, and, therefore, were available for inclusion in this retrospective study. Patients included were all treated with osimertinib as a first- or second-line of treatment. All patients receiving osimertinib as second-line of treatment received erlotinib as first-line of treatment. Inclusion required that the patient responded to treatment with osimertinib, followed by PD, and had blood samples available. Response to treatment was based on the response evaluation criteria in solid tumors (RECIST), and PD was either defined as RECIST, smaller than RECIST, and/or as a clinical judgement by the patient’s physician (28). The primary outcome of this study was to evaluate the OS following PD on osimertinib. Progression free survival (PFS) was defined as the time from osimertinib start until PD. OS was defined as the time from osimertinib start until death or censoring of data, whereas OS after PD was defined as the time from PD until death or censoring of data.
Data collection
Data regarding treatment and demographics before start of treatment were extracted from the Aarhus Lung Cancer database (AALCR). Data were updated from medical records to the lung cancer database on January 3, 2022.
Blood samples
Peripheral blood was drawn from each patient approximately every 4–6 weeks in 10 mL EDTA tubes and centrifuged within 6 h at 1,400 g for 15 min at room temperature. Plasma was aliquoted and stored at −80 ℃ (29).
Two blood samples from each patient were selected: a response blood sample and a progression blood sample. A response blood sample was defined as a blood sample taken from the patient after treatment with osimertinib was initiated, and the patient showed response to the treatment according to the RECIST criteria. More specifically, the patient should have stable disease (SD), partial response (PR) or complete response (CR) at the time of blood withdrawal or a maximum of two months before or after the scan. The progression sample was the blood sample taken at the first PD during osimertinib treatment, identified by the oncologist, or a blood sample taken a maximum of one month before or after. If the sample was taken after the scan, it was only applicable if no new treatment was initiated in the meantime.
PEA
Response and PD plasma samples were analyzed using PEAs at BioXpedia, Aarhus, Denmark, which is described in detail in previous studies (30,31). In this study, we applied the Target 96 Oncology II panel (Olink). This panel is a high-throughput, multiplex immunoassay targeting 92 oncology-associated proteins. In brief, the technology applies two oligonucleotide-conjugated antibodies for each protein, which, upon binding to the target, allow hybridization of the oligonucleotides. Addition of a DNA polymerase amplifies a unique PCR reporter sequence, which is detected using qPCR (Fluidigm Biomark HD system). Subsequently, the Cq-values are normalized to an interplate control and converted to Normalized Protein eXpression (NPX) units on a Log2 scale.
Gene Ontology (GO) enrichment analysis
GO enrichment analysis (32,33) was performed using the topGO package (v. 2.49.0, https://topgo.bioinf.mpi-inf.mpg.de/) (34). The analysis was performed in R version 4.2.1. Of the 92 proteins in the Target 96 Oncology II panel (Olink), 91 were analyzed using the biological process (BP) ontology database (SEZ6L was excluded because it was not associated with any BP GO terms). The gene universe was defined as the 91 proteins, and the significant genes were defined as differentially expressed proteins at PD compared to the response (two-tailed q<0.05). Significantly enriched GO terms were defined as having a P<0.05 based on the classic algorithm and Fisher exact test.
Patients were divided into groups based on their summarized NPX (sNPX) values for proteins associated with the GO terms “immune response” (GO:0006955) and “adaptive immune response” (GO:0002250). sNPX values are calculated by adding the NPX values for individual proteins related to a specific GO term together for each patient. Patients with sNPX values above the median for all patients were classified as “high”, whereas patients with sNPX below the median were classified as “low”.
Statistical analysis
Differences in protein levels at response compared to PD were analyzed using the Wilcoxon signed-rank test. To correct for multiple testing, false discovery rate (FDR) adjustment (35) was performed where a two-tailed q-value <0.05 were considered significant. The Kaplan-Meier method including a log-rank test was used to study the overall survival (OS) after PD. Data analyses were performed in R v. 4.2.1, as well as GraphPad Prism v. 9.3.1.
Results
Patients
Twenty-six patients were included in the study, and plasma samples were investigated with PEA analysis. The inclusion of patients for the study is described in Figure 1. Unfortunately, one patient’s blood sample was not of a sufficient quality to be analyzed; therefore, only 25 patients are included in the following results.
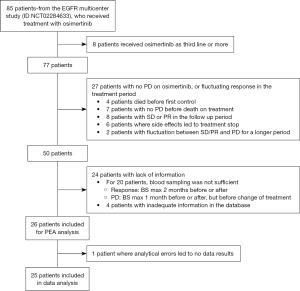
The patient’s demographics and characteristics at the start of osimertinib treatment are based on high or low sNPX values at PD for “Immune response” proteins (Table 1). There was a predominance of women in the cohort (68%). At the start of osimertinib treatment, the mean age was 64.1 years (range, 28–82 years); the majority had disseminated disease TxNxM1b (68%), no brain metastases (BM) (76%), were former or current smokers (56%), with a PS score at 0 or 1 (60%), and a baseline comorbidity score of 0 (72%), based on Charlson comorbidity index. Only one patient harbored a rare EGFR mutation (S768I), while the rest had a common EGFR mutation: del19 (18 patients) or L858R (6 patients). The T790M mutation was found in 76% of patients before osimertinib was initiated, either in blood samples using the Cobas EGFR V2 mutation test by Roche or in tumor biopsies. Two patients received osimertinib as first-line of therapy while the rest received osimertinib as second-line of treatment. The majority of the patients had PD based on RECIST (60%). A significant difference between smokers versus nonsmokers was found in the low versus high group. Patients in the high group had a higher proportion of smokers (former and current), compared to the low group who had a higher proportion of nonsmokers (never and passive). Apart from this, there was no significant difference in demographics between the groups.
Table 1
Demographics | All patients (n=25) | Low (n=12; 48%) | High (n=13; 52%) | P value |
---|---|---|---|---|
Gender, n [%] | ||||
Female | 17 [68] | 7 [58] | 10 [77] | |
Male | 8 [32] | 5 [42] | 3 [23] | 0.4110 |
Age (years) | ||||
Mean age | 64.1 | 62.9 | 65.1 | |
<64.1, n [%] | 11 [44] | 6 [50] | 5 [38] | |
≥64.1, n [%] | 14 [56] | 6 [50] | 8 [62] | 0.6951 |
TNM stage, n [%] | ||||
M0 and M1A | 8 [32] | 5 [42] | 3 [23] | |
M1B | 17 [68] | 7 [58] | 10 [77] | 0.4110 |
Smoking status, n [%] | ||||
Never | 11 [44] | 8 [67] | 3 [23] | |
Former/current | 14 [56] | 4 [33] | 10 [77] | 0.0472 |
PS, n [%] | ||||
0 or 1 | 15 [60] | 9 [75] | 6 [46] | |
2 or more | 7 [28] | 2 [17] | 5 [39] | 0.3358 |
Unknown | 3 [12] | 1 [8] | 2 [15] | |
Comorbidity score at BL, n [%] | ||||
0 | 18 [72] | 9 [75] | 9 [69] | |
1 or more | 7 [28] | 3 [25] | 4 [31] | >0.9999 |
EGFR mutation, n [%] | ||||
Common (del19, L858R) | 24 [96] | 12 [100] | 12 [92] | |
Rare | 1 [4] | 0 | 1 [8] | >0.9999 |
T790M status, n [%] | ||||
Yes | 19 [76] | 8 [67] | 11 [85] | |
No | 6 [24] | 4 [33] | 2 [15] | 0.3782 |
BM, n [%] | ||||
Yes | 6 [24] | 2 [17] | 4 [31] | |
No | 19 [76] | 10 [83] | 9 [69] | 0.6447 |
Line of therapy, n [%] | ||||
First-line | 2 [8] | 1 [8] | 1 [8] | |
Second-line | 23 [92] | 11 [92] | 12 [92] | >0.9999 |
PD, n [%] | ||||
RECIST | 15 [60] | 6 [50] | 9 [69] | |
Other | 10 [40] | 6 [50] | 4 [31] | 0.4283 |
“Immune response” proteins median sNPX: 41.49. Patients with sNPX values above the median for all patients were classified as “high”, whereas patients with sNPX below the median were classified as “low”. All demographics, except for PD and comorbidities, are defined at osimertinib start. Differences between groups are tested using Fisher’s exact test. TNM, tumor-node-metastasis; PS, performance status; BL, baseline; EGFR, epidermal growth factor receptor; BM, brain metastases; PD, progressive disease; RECIST, response evaluation criteria in solid tumors; sNPX, summarized Normalized Protein expression.
Discovering differences in circulating proteins
Plasma samples from 25 patients were investigated for circulating proteins using PEA. The plasma samples taken at the time of positive treatment response (called response sample, Table S1) were compared to the plasma samples taken at disease progression (called PD sample, Table S2), and the differences between the two samples were determined. Figure 2A displays the seven proteins that were differentially regulated at response compared to PD samples. Just one of the seven proteins were upregulated in progression samples, whereas six proteins were downregulated (Table 2). The patients were then grouped based on the median difference between the response and the PD blood sample for each of the seven significant proteins (Figure 2B). Patients with a difference in NECTIN4 levels below the group median (0.29) demonstrated a longer OS following PD than the patients above the median [hazard ratio (HR) =4.06; 95% confidence interval (CI): 1.57–10.45; P=0.00033]. For the remainder of the proteins, no difference was detected between the groups. None of the differentially regulated proteins had a significant impact on PFS (Figure S1).
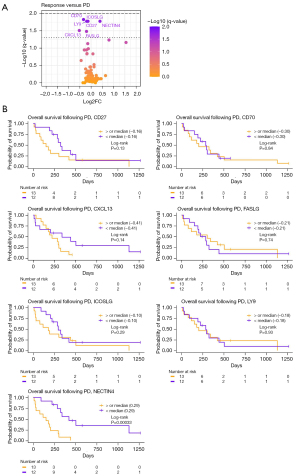
Table 2
Protein | Log2FC | Median at response (± SE) | Median at progression (± SE) | q-value |
---|---|---|---|---|
CD27 | −0.18 | 8.11 (±0.09) | 7.93 (±0.09) | 0.0167 |
CD70 | −0.34 | 4.63 (±0.08) | 4.29 (±0.09) | 0.0149 |
CXCL13 | −0.52 | 8.41 (±0.27) | 7.88 (±0.29) | 0.0310 |
FASLG | −0.20 | 9.93 (±0.11) | 9.73 (±0.09) | 0.0330 |
ICOSLG | −0.14 | 5.31 (±0.05) | 5.18 (±0.04) | 0.0167 |
LY9 | −0.19 | 6.46 (±0.09) | 6.26 (±0.09) | 0.0167 |
NECTIN4 | 0.40 | 5.20 (±0.13) | 5.60 (±0.19) | 0.0167 |
Log2FC, Log2 fold change; SE, standard error.
Although not statistically significant, CEACAM5 had the highest Log2 fold change (Log2FC) (Log2FC =1.55, equivalent to 2.93 increase in linear values). This is supported by previous studies describing CEA as a marker for tumor burden, associated with PFS and OS in lung cancer (23,36). In this study, we find that a high level of PEA measured CEACAM5 in the response sample was associated with a reduced PFS as well as OS (Figure S2). However, the dynamic changes of CEACAM5 did not predict the outcome following PD. These findings validate the PEA protocol as a way to study tumor dynamics in the blood.
GO enrichment analysis
To better understand which processes were differently expressed between response and progression samples, we applied GO enrichment analysis to the PEA-measured proteins. In total 3,423 BP GO terms were assigned to 91 of the 92 proteins in the Olink Target 96 Oncology II panel. Based on the significant proteins presented in Figure 2 and Table 2, we found 31 significantly enriched GO terms (P<0.05; Table S3), and the top 10 GO terms are displayed in Figure 3. The figure displays how most of the significant GO terms are associated with the immune system. Therefore, we conclude that, during osimertinib treatment, the patient’s immune system is altered from the time of response to progression. The six proteins associated with the GO term immune response all showed a decrease between the two samples (Table 2, Table S3). These results demonstrate that immune biomarkers are declining overall at the time of PD compared to response.
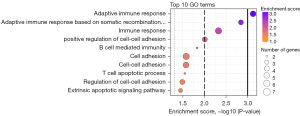
Immune proteins at progression predicts OS after PD
To investigate if the combined protein concentration of the immune proteins that were discovered in the GO enrichment analysis could predict the patients’ OS after PD, a survival analysis was performed between groups with low and high sNPX. Proteins associated to the GO term “Immune response” were chosen because this term was associated to most proteins (CD27, CD70, CXLC13, FASLG, ICOSLG, LY9, Table S3). A significant difference between the high (median survival =442 days) and low (median survival =193 days) groups was discovered (Figure 4A, HR =3.04; 95% CI: 1.24–7.45; P=0.0046). The course of the disease is displayed for each patient after initiation of osimertinib until the time of death or last follow-up (January 3, 2022) in Figure 4B. This demonstrates a similar blood sampling, treatment, and follow-up for the two groups. A significant difference was also found when only looking at proteins related to the “Adaptive immune response” (CD27, CD70, CXLC13, ICOSLG, LY9, Figure S3), which was the GO term most significant in the GO enrichment analysis (Figure 3). Patients with a low sNPX (median survival =324 days) in relation to the adaptive immune response had a significantly longer OS after PD, compared to patients with high sNPX (median survival =193 days) at PD (HR =2.72; 95% CI: 1.12–6.57; P=0.0146). This indicate that patients with a low amount of circulating immune response proteins in the blood at PD have a longer survival after PD, compared with patients with a high. To investigate the relevance of each protein in the “immune response” patients were divided based on the level of the individual proteins (Figure S4). These results demonstrated that only CXCL13 levels could significantly predict the patient’s outcome, however with an inferior HR of 2.55 (95% CI: 1.06–6.31; P=0.021), compared to the combined sNPX values (HR =3.04). This indicates that a combined evaluation of proteins related to the immune system is the strongest predictor of the patient’s outcome following PD.
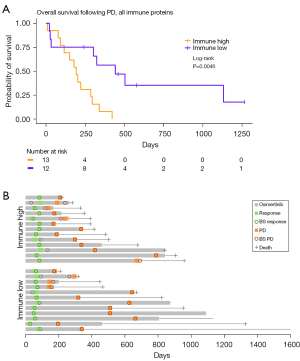
Discussion
In this study, we found that immune-related proteins are differentially regulated in the blood taken from patients at the time of osimertinib response compared to at PD. By defining two groups of patients based on sNPX values for immune response proteins, we found that patients with a lower amount of circulating immune response proteins at PD had a significantly longer OS after PD.
This study serves as a pilot study for the relation between the immune system and osimertinib resistance and lacks a suitable validation cohort to verify the findings. Future research with a more homogenous cohort consisting of first-line osimertinib treated patients could further strengthen the hypotheses presented in this study. Although this study identifies immune response-related proteins to be of importance during osimertinib treatment it is most likely that other protein pathways are also involved in osimertinib resistance which could be identified using a larger protein panel. Furthermore, future studies could address the causal link and temporal relationship between immune-related proteins in plasma and tumor progression on osimertinib.
Interestingly, this study demonstrates that patients with high amount of immune response-related proteins are more likely to have a smoking history compared to patients with a low amount of immune response-related proteins (Table 1). This supports the idea of smoking affecting the immune landscape of lung cancer patients (37) and future studies is needed to evaluate the involvement of smoking and the immune system at osimertinib progression.
Previous studies have found that the immune biomarkers are associated with the response to EGFR-TKI treatment (38,39). Recently, Gurule et al. used RNA extracted from tissue biopsies to demonstrate that, during the initial treatment, interferon-gamma related genes are upregulated (38). Furthermore, they found a positive correlation between the level of interferon-gamma upregulation and the time-to-progression, indicating that a strong innate immune response is associated with favorable clinical response to the treatment. Our findings add to this study by evaluating the tumor dynamics at PD, rather than at the beginning of TKI-treatment. In this study, we evaluate tumor dynamics by analyzing the circulating proteins representing the protein expression of all cells shedding to the bloodstream, including tumor and immune cells, rather than RNA-seq on tumor biopsies.
A previous study found that some patients have increased programmed death-ligand 1 (PD-L1) expression at PD, compared to baseline, when treated with osimertinib (39). In the study by Isomoto et al., 27 patients were treated with anti-programmed death 1 (anti-PD-1) antibodies following their initial TKI treatment after PD. The patients with a high PD-L1 expression at PD responded well to the treatment. These results demonstrate that, in some patients, PD could be the result of changes in the tumor microenvironment inhibiting the antitumor immune response. In this study, we found that patients with high levels of circulating adaptive immune proteins had a shorter OS following PD. Potentially, these patients represent patients with increased PD-L1 expression induced by EGFR-driven tumors (40,41) leading to PD, with an exhausted immune system reducing the survival after PD. This is supported by Dai et al. who demonstrated that clear cell renal cell carcinoma patients with high levels of intratumoral CD8+, CXCL13+ T cells had inferior outcome and had elevated CD8+ T cell exhaustion markers (PD-1, Tim-3, TIGIT) (42). Furthermore, exhausted CD39+, CD4+ T cells have also demonstrated increased CXCL13 expression (43). Unfortunately, we were not able to obtain information on the PD-L1 status, or other biomarkers in the tumor at PD for the patients in this study; however, future studies could give more insights into this hypothesis. Combined, these studies indicate that some patients with tumor progression on osimertinib could benefit from immune checkpoint inhibitor therapy. This is currently being investigated in combination with chemotherapy in the KEYNOTE-789 and CheckMate722 phase III clinical trials. Evaluating the immune-related proteins in plasma at PD on osimertinib, could potentially help to stratify which patients would benefit from immunotherapy.
One of the limitations of this study is the small number of patients used in the survival analysis, where the group median is necessary to distinguish the immune high and low groups. Another limitation is the definition of PD for the patients. It is a well-known problem that the RECIST criteria are difficult to translate onto a heterogenic group of patients, given that many factors in their course of disease can affect the choice for further treatment. Additionally, PD is a subjective decision made by the patient’s oncologist based on radiologic scans and clinical assessments, which can be different between oncologists (44,45). For most patients, the PD was based on RECIST, but in some cases, it was described as PD based on clinical assessment of the patient. This variation in describing RECIST could interfere with the results.
Furthermore, patients were often treated beyond progression (Figure 4B). This is not uncommon, and treatment beyond progression can be used to avoid a withdrawal tumor flair (46,47). Treatment beyond progression is also seen in cases where the patient has localized progression and receives local radiation therapy in combination with EGFR-TKI, which results in another durable period without progression on the EGFR-TKI (48,49).
In many studies, baseline blood samples are used for comparison of the patient’s oncological status with later blood samples in the patient’s course of treatment. In this study, we decided to introduce the use of a response blood sample instead. Baseline blood samples are taken prior to the first line of treatment, and most patients had already been treated with erlotinib before osimertinib. Therefore, we assumed other proteins not related to the ongoing treatment would disturb the analysis.
Conclusions
Based on blood samples taken during osimertinib treatment of EGFR-mutated NSCLC patients, we find that the level of circulating immune proteins at PD can predict OS after PD. These findings solidify the importance of the immune system in EGFR-TKI resistance and demonstrate the need for future research to understand the interplay between targeted therapies and the effectiveness of the immune response.
Acknowledgments
The authors are grateful for all the patients who participated in this study.
Funding: None.
Footnote
Reporting Checklist: The authors have completed the MDAR reporting checklist. Available at https://tlcr.amegroups.com/article/view/10.21037/tlcr-22-577/rc
Data Sharing Statement: Available at https://tlcr.amegroups.com/article/view/10.21037/tlcr-22-577/dss
Peer Review File: Available at https://tlcr.amegroups.com/article/view/10.21037/tlcr-22-577/prf
Conflicts of Interest: All authors have completed the ICMJE uniform disclosure form (available at https://tlcr.amegroups.com/article/view/10.21037/tlcr-22-577/coif). The authors have no conflicts of interest to declare.
Ethical Statement: The authors are accountable for all aspects of the work in ensuring that questions related to the accuracy or integrity of any part of the work are appropriately investigated and resolved. The research was conducted in accordance with the Declaration of Helsinki (as revised in 2013) and approved by the National Committee on Health Ethics (No. 1-10-72-83-14) and the Danish Data Protection Agency (No. 1-16-02-431-14). Written informed consent was obtained from all participating individuals.
Open Access Statement: This is an Open Access article distributed in accordance with the Creative Commons Attribution-NonCommercial-NoDerivs 4.0 International License (CC BY-NC-ND 4.0), which permits the non-commercial replication and distribution of the article with the strict proviso that no changes or edits are made and the original work is properly cited (including links to both the formal publication through the relevant DOI and the license). See: https://creativecommons.org/licenses/by-nc-nd/4.0/.
References
- WHO. Cancer Fact Sheet - Tracea, broncus and lung: International Agency for Research on Cancer. 2019. Available online: http://gco.iarc.fr/today/data/factsheets/cancers/15-Lung-fact-sheet.pdf
- Herbst RS, Morgensztern D, Boshoff C. The biology and management of non-small cell lung cancer. Nature 2018;553:446-54. [Crossref] [PubMed]
- Weber B, Hager H, Sorensen BS, et al. EGFR mutation frequency and effectiveness of erlotinib: a prospective observational study in Danish patients with non-small cell lung cancer. Lung Cancer 2014;83:224-30. [Crossref] [PubMed]
- Rosell R, Moran T, Queralt C, et al. Screening for epidermal growth factor receptor mutations in lung cancer. N Engl J Med 2009;361:958-67. [Crossref] [PubMed]
- Gazdar AF. Activating and resistance mutations of EGFR in non-small-cell lung cancer: role in clinical response to EGFR tyrosine kinase inhibitors. Oncogene 2009;28:S24-31. [Crossref] [PubMed]
- Pao W, Miller V, Zakowski M, et al. EGF receptor gene mutations are common in lung cancers from "never smokers" and are associated with sensitivity of tumors to gefitinib and erlotinib. Proc Natl Acad Sci U S A 2004;101:13306-11. [Crossref] [PubMed]
- Lynch TJ, Bell DW, Sordella R, et al. Activating mutations in the epidermal growth factor receptor underlying responsiveness of non-small-cell lung cancer to gefitinib. N Engl J Med 2004;350:2129-39. [Crossref] [PubMed]
- Mok TS, Wu YL, Thongprasert S, et al. Gefitinib or carboplatin-paclitaxel in pulmonary adenocarcinoma. N Engl J Med 2009;361:947-57. [Crossref] [PubMed]
- Engelman JA, Jänne PA. Mechanisms of acquired resistance to epidermal growth factor receptor tyrosine kinase inhibitors in non-small cell lung cancer. Clin Cancer Res 2008;14:2895-9. [Crossref] [PubMed]
- Pao W, Miller VA, Politi KA, et al. Acquired resistance of lung adenocarcinomas to gefitinib or erlotinib is associated with a second mutation in the EGFR kinase domain. PLoS Med 2005;2:e73. [Crossref] [PubMed]
- Kobayashi S, Boggon TJ, Dayaram T, et al. EGFR mutation and resistance of non-small-cell lung cancer to gefitinib. N Engl J Med 2005;352:786-92. [Crossref] [PubMed]
- Cross DA, Ashton SE, Ghiorghiu S, et al. AZD9291, an irreversible EGFR TKI, overcomes T790M-mediated resistance to EGFR inhibitors in lung cancer. Cancer Discov 2014;4:1046-61. [Crossref] [PubMed]
- Ballard P, Yates JW, Yang Z, et al. Preclinical Comparison of Osimertinib with Other EGFR-TKIs in EGFR-Mutant NSCLC Brain Metastases Models, and Early Evidence of Clinical Brain Metastases Activity. Clin Cancer Res 2016;22:5130-40. [Crossref] [PubMed]
- Mok TS, Wu Y-L, Ahn M-J, et al. Osimertinib or Platinum-Pemetrexed in EGFR T790M-Positive Lung Cancer. N Engl J Med 2017;376:629-40. [Crossref] [PubMed]
- Michels S, Heydt C, van Veggel B, et al. Genomic Profiling Identifies Outcome-Relevant Mechanisms of Innate and Acquired Resistance to Third-Generation Epidermal Growth Factor Receptor Tyrosine Kinase Inhibitor Therapy in Lung Cancer. JCO Precis Oncol 2019;3:PO.18.00210.
- Blakely CM, Watkins TBK, Wu W, et al. Evolution and clinical impact of co-occurring genetic alterations in advanced-stage EGFR-mutant lung cancers. Nat Genet 2017;49:1693-704. [Crossref] [PubMed]
- Bordi P, Del Re M, Minari R, et al. From the beginning to resistance: Study of plasma monitoring and resistance mechanisms in a cohort of patients treated with osimertinib for advanced T790M-positive NSCLC. Lung Cancer 2019;131:78-85. [Crossref] [PubMed]
- Zhu C, Zhuang W, Chen L, et al. Frontiers of ctDNA, targeted therapies, and immunotherapy in non-small-cell lung cancer. Transl Lung Cancer Res 2020;9:111-38. [Crossref] [PubMed]
- Leonetti A, Sharma S, Minari R, et al. Resistance mechanisms to osimertinib in EGFR-mutated non-small cell lung cancer. Br J Cancer 2019;121:725-37. [Crossref] [PubMed]
- Lindeman NI, Cagle PT, Aisner DL, et al. Updated Molecular Testing Guideline for the Selection of Lung Cancer Patients for Treatment With Targeted Tyrosine Kinase Inhibitors: Guideline From the College of American Pathologists, the International Association for the Study of Lung Cancer, and the Association for Molecular Pathology. J Mol Diagn 2018;20:129-59. [Crossref] [PubMed]
- Winther-Larsen A, Demuth C, Fledelius J, et al. Correlation between circulating mutant DNA and metabolic tumour burden in advanced non-small cell lung cancer patients. Br J Cancer 2017;117:704-9. [Crossref] [PubMed]
- Rolfo C, Mack PC, Scagliotti GV, et al. Liquid Biopsy for Advanced Non-Small Cell Lung Cancer (NSCLC): A Statement Paper from the IASLC. J Thorac Oncol 2018;13:1248-68. [Crossref] [PubMed]
- Grunnet M, Sorensen JB. Carcinoembryonic antigen (CEA) as tumor marker in lung cancer. Lung Cancer 2012;76:138-43. [Crossref] [PubMed]
- Burghuber OC, Worofka B, Schernthaner G, et al. Serum neuron-specific enolase is a useful tumor marker for small cell lung cancer. Cancer 1990;65:1386-90. [Crossref] [PubMed]
- Huang Z, Xu D, Zhang F, et al. Pro-gastrin-releasing peptide and neuron-specific enolase: useful predictors of response to chemotherapy and survival in patients with small cell lung cancer. Clin Transl Oncol 2016;18:1019-25. [Crossref] [PubMed]
- Kato Y, Tanaka Y, Hino M, et al. ProGRP as early predictive marker of non-small-cell lung cancer to small-cell lung cancer transformation after EGFR-TKI treatment. Respir Med Case Rep 2019;27:100837. [Crossref] [PubMed]
- Lamy PJ, Grenier J, Kramar A, et al. Pro-gastrin-releasing peptide, neuron specific enolase and chromogranin A as serum markers of small cell lung cancer. Lung Cancer 2000;29:197-203. [Crossref] [PubMed]
- Eisenhauer EA, Therasse P, Bogaerts J, et al. New response evaluation criteria in solid tumours: revised RECIST guideline (version 1.1). Eur J Cancer 2009;45:228-47. [Crossref] [PubMed]
- Ebert EBF, McCulloch T, Hansen KH, et al. Clearing of circulating tumour DNA predicts clinical response to first line tyrosine kinase inhibitors in advanced epidermal growth factor receptor mutated non-small cell lung cancer. Lung Cancer 2020;141:37-43. [Crossref] [PubMed]
- Assarsson E, Lundberg M, Holmquist G, et al. Homogenous 96-plex PEA immunoassay exhibiting high sensitivity, specificity, and excellent scalability. PLoS One 2014;9:e95192. [Crossref] [PubMed]
- Lundberg M, Eriksson A, Tran B, et al. Homogeneous antibody-based proximity extension assays provide sensitive and specific detection of low-abundant proteins in human blood. Nucleic Acids Res 2011;39:e102. [Crossref] [PubMed]
- Ashburner M, Ball CA, Blake JA, et al. Gene ontology: tool for the unification of biology. The Gene Ontology Consortium. Nat Genet 2000;25:25-9. [Crossref] [PubMed]
- The Gene Ontology resource: enriching a GOld mine. Nucleic Acids Res 2021;49:D325-34. [Crossref] [PubMed]
- Alexa A, Rahnenführer J, Lengauer T. Improved scoring of functional groups from gene expression data by decorrelating GO graph structure. Bioinformatics 2006;22:1600-7. [Crossref] [PubMed]
- Benjamini Y, Hochberg Y. Controlling the False Discovery Rate: A Practical and Powerful Approach to Multiple Testing. Journal of the Royal Statistical Society Series B (Methodological) 1995;57:289-300. [Crossref]
- Arrieta O, Saavedra-Perez D, Kuri R, et al. Brain metastasis development and poor survival associated with carcinoembryonic antigen (CEA) level in advanced non-small cell lung cancer: a prospective analysis. BMC Cancer 2009;9:119. [Crossref] [PubMed]
- Corke LK, Li JJN, Leighl NB, et al. Tobacco Use and Response to Immune Checkpoint Inhibitor Therapy in Non-Small Cell Lung Cancer. Curr Oncol 2022;29:6260-76. [Crossref] [PubMed]
- Gurule NJ, McCoach CE, Hinz TK, et al. A tyrosine kinase inhibitor-induced interferon response positively associates with clinical response in EGFR-mutant lung cancer. NPJ Precis Oncol 2021;5:41. [Crossref] [PubMed]
- Isomoto K, Haratani K, Hayashi H, et al. Impact of EGFR-TKI Treatment on the Tumor Immune Microenvironment in EGFR Mutation-Positive Non-Small Cell Lung Cancer. Clin Cancer Res 2020;26:2037-46. [Crossref] [PubMed]
- Chen N, Fang W, Zhan J, et al. Upregulation of PD-L1 by EGFR Activation Mediates the Immune Escape in EGFR-Driven NSCLC: Implication for Optional Immune Targeted Therapy for NSCLC Patients with EGFR Mutation. J Thorac Oncol 2015;10:910-23. [Crossref] [PubMed]
- Akbay EA, Koyama S, Carretero J, et al. Activation of the PD-1 pathway contributes to immune escape in EGFR-driven lung tumors. Cancer Discov 2013;3:1355-63. [Crossref] [PubMed]
- Dai S, Zeng H, Liu Z, et al. Intratumoral CXCL13(+)CD8(+)T cell infiltration determines poor clinical outcomes and immunoevasive contexture in patients with clear cell renal cell carcinoma. J Immunother Cancer 2021;9:e001823. [Crossref] [PubMed]
- Balança CC, Salvioni A, Scarlata CM, et al. PD-1 blockade restores helper activity of tumor-infiltrating, exhausted PD-1hiCD39+ CD4 T cells. JCI Insight 2021;6:142513. [Crossref] [PubMed]
- Beaumont H, Iannessi A, Wang Y, et al. Blinded Independent Central Review (BICR) in New Therapeutic Lung Cancer Trials. Cancers (Basel) 2021;13:4533. [Crossref] [PubMed]
- Abramson RG, McGhee CR, Lakomkin N, et al. Pitfalls in RECIST Data Extraction for Clinical Trials: Beyond the Basics. Acad Radiol 2015;22:779-86. [Crossref] [PubMed]
- Riely GJ, Kris MG, Zhao B, et al. Prospective assessment of discontinuation and reinitiation of erlotinib or gefitinib in patients with acquired resistance to erlotinib or gefitinib followed by the addition of everolimus. Clin Cancer Res 2007;13:5150-5. [Crossref] [PubMed]
- Chaft JE, Oxnard GR, Sima CS, et al. Disease flare after tyrosine kinase inhibitor discontinuation in patients with EGFR-mutant lung cancer and acquired resistance to erlotinib or gefitinib: implications for clinical trial design. Clin Cancer Res 2011;17:6298-303. [Crossref] [PubMed]
- Yu HA, Sima CS, Huang J, et al. Local therapy with continued EGFR tyrosine kinase inhibitor therapy as a treatment strategy in EGFR-mutant advanced lung cancers that have developed acquired resistance to EGFR tyrosine kinase inhibitors. J Thorac Oncol 2013;8:346-51. [Crossref] [PubMed]
- Chan OSH, Lam KC, Li JYC, et al. ATOM: A phase II study to assess efficacy of preemptive local ablative therapy to residual oligometastases of NSCLC after EGFR TKI. Lung Cancer 2020;142:41-6. [Crossref] [PubMed]