LRP1B mutation is associated with tumor immune microenvironment and progression-free survival in lung adenocarcinoma treated with immune checkpoint inhibitors
Highlight box
Key findings
• LRP1B mutation is associated with higher immune cell infiltration and elevated immune gene expression in TIME and potentially serves as a prognostic biomarker for LUAD patients treated with ICIs.
What is known and what is new?
• LRP1B mutation is associated with higher tumor mutation burden (TMB), and patients with LRP1B mutation have prolonged survival after immunotherapy.
• LRP1B mutation can affect the immune microenvironment, thus improving the efficacy of ICIs treatment and prolonging the PFS of LUAD patients.
What is the implication, and what should change now?
• We can be more aggressive in selecting ICIs for LUAD patients with LRP1B mutation, rather than just assessing PD-L1 expression or TMB to determine whether they should receive immunotherapy.
Introduction
Lung cancer has been recognized as one of the world’s most lethal cancers (1). Lung adenocarcinoma (LUAD) accounts for more than 40% of lung cancer cases (2,3). Despite the development of therapeutic modalities such as targeted therapy, survival rates for patients with LUAD remain below 20% over 5 years (4,5). Surgery is currently the primary treatment, but even in stage I LUAD, 30% of patients still develop recurrence or metastasis within five years after undergoing radical surgery (6). Adjuvant chemotherapy has been shown to improve patient survival after surgery, but for some patients the toxicity associated with chemotherapy outweighs its potential benefits (7).
Immunotherapy has altered the prognosis of LUAD, of which immune checkpoint inhibitors (ICIs) have attracted considerable attention due to durable responsiveness and significant improvement in patients’ prognosis. ICIs mainly act by preventing the binding of inhibitory receptors (checkpoint block) to their ligands. Tumor mutational burden (TMB) and programmed death ligand-1 (PD-L1) are widely clinically used as predictive biomarkers. However, ICIs are effective in only a fraction of patients, possibly due to the spatial heterogeneity within the tumor (8), subjective analysis of PD-L1 (9), and lack of standardized thresholds for TMB determination (10). The positive response of LUAD to ICIs often relies on the interaction between tumor cells and immune cells and immune-related molecules in the tumor immune microenvironment (TIME), while major cellular components of the TIME also play an anti-tumor role during the pre-progression phase (11). It may be regulated by the involvement of T cell activation and CD4 memory T cell pathways (12). The TIME is a highly complex and interconnected spatial structure, in which the spatial structure of various cell types is determined by the vascular, lymphatic vessel and fibroblast matrix in the microenvironment. It contains a variety of functionally distinct immune cells and non-immune stromal cells and is important in immunotherapy (13-15). Growing evidence demonstrates that both immune and stromal cells influence tumor response to ICIs (16).
A low-density lipoprotein receptor-related protein, LRP1B, is implicated as strongly associated with cancer development and due to its frequent inactivation in non-small cell lung cancer (NSCLC), and was initially postulated to be a tumor suppressor gene (17). Studies have shown that specific genetic mutations affect the effects of ICIs, and EMT amplification has previously been found to be associated with prolonged progression-free survival (PFS) after receiving immunotherapy (18). Recently, LRP1B mutation was discovered to be associated with improved outcomes in multiple cancers receiving immunotherapy (19,20). However, the molecular mechanism of how LRP1B mutations are related to better prognosis in immunotherapy patients is unclear, and the most common explanation is that LRP1B mutation is associated with TMB (21). Here, we hypothesize that LRP1B mutation in LUAD may positively affect the TIME, ultimately affecting the outcome of patients treated with ICIs. We present the following article in accordance with the REMARK reporting checklist (available at https://tlcr.amegroups.com/article/view/10.21037/tlcr-23-39/rc).
Methods
The Cancer Genome Atlas (TCGA)-LUAD and ICIs cohorts
To assess the association of LPR1B mutation with the outcomes of patients receiving ICIs, we collected an LUAD cohort treated with programmed cell death protein 1 (PD-1) plus CTLA-4 blockade from a previous study (ICIs cohort) (22). A total of 59 LUAD patients with complete sequencing and clinical data were included. The clinical information and sequencing data of all patients were collected, including age, sex, treatment response, neoantigen loads (NALs), TMB, and gene mutation types and sites. PFS was defined as starting on the day of the first dose and ending on the date of disease progression, patient death, or study completion. The best overall response was assessed according to RECIST 1.1 criteria. We classified patients in this cohort into the LRP1B-mutated type (MT) group (n=15) and LRP1B-wild type (WT) group (n=44) according to the mutation status of LRP1B. Furthermore, TCGAbiolinks (23) was used to download data on the transcriptome, somatic mutations, and overall survival (OS) for the TCGA-LUAD cohort. We used cBioPortal (24) to obtain data on PFS for the TCGA-LUAD cohort. After excluding patients with missing data, a total of 507 LUAD patients were included. Differences in the survival of TCGA-LUAD patients according to LRP1B mutation status were analyzed.
Tumor mutation characteristics and tumor immunogenicity assessment
The somatic mutations of 59 LUAD patients from the ICIs cohort were obtained by whole exome sequencing (WES). In accordance with previous studies, TMB was defined as the overall number of non-synonymous mutations per megabase (Mb) in the coding region of the genes (25). NALs and immune scores were obtained from the previous study (26). LRP1B mutation frequencies, mutation types, and mutant structural domains were obtained from cBioPortal (24). Maftools was used to display the top 20 mutations and clinical features of the ICIs and TCGA-LUAD cohorts, as well as the LRP1B mutations in both cohorts (27). The correlation of the mutated genes was displayed by ComplexHeatmap in the R package (28).
Analysis of copy number variation
After quality control, data from the TCGA-LUAD cohort was retrieved from Affymetrix SNP 6.0 microarray (hg19) by Broad GDAC Firehose (http://gdac.broadinstitute.org/). GISTIC 2.0 (29) was conducted to analyze copy number variants (CNVs) and significantly amplified or deleted regions. The confidence interval (CI) was set to 0.99 (X chromosome excluded), and default parameters were set for others.
Immune characteristics analysis
The transcriptome of the TCGA-LUAD cohort was obtained via TCGAbiolinks. We performed Cell-type Identification by Estimating Relative Subsets of RNA Transcripts (CIBERSORT) analysis (30) on the transcriptome and estimated the content of the 22 immune cells. We made a comparison of the immune genes between the LRP1B-MT and LRP1B-WT groups (26). The level of gene expression was assessed uniformly as log2 [transcripts per million (TPM) +1].
Analysis of pathway enrichment and DNA damage response and repair pathway
The limma package in R (31) was used to analyze differential gene expression between the LRP1B-MT group and LRP1B-WT groups in the TCGA-LUAD cohort. Any gene with an absolute value of log2foldchange (FC) greater than 0.5 and P value less than 0.05 was identified as significantly different. We used the gene set enrichment analysis (GSEA) to analyze the differential genes for gene annotation enrichment analysis in terms of Gene Ontology (GO) and Kyoto Encyclopedia of Genes and Genomes (KEGG). A P value <0.05 was considered statistically significant. We evaluated non-synonymous mutations in the DNA damage response and repair (DDR) pathways in the ICIs and TCGA-LUAD cohorts. Non-synonymous mutations in the LRP1B-MT and LRP1B-WT groups were analyzed. The GSEA and DDR pathway were referenced in the MSigDB database (Broad Institute, Cambridge, MA, USA). The DDR pathway gene set is available in Table S1 (32).
Analysis of TIME in clinical specimens
We retrospectively collected 20 LUAD patient samples which confirmed to be clinical stage IA according to the eighth edition of tumor-node-metastasis (TNM) staging from January 2020 to January 2021 who underwent partial pneumonectomy or radical lobectomy at the Department of Thoracic Surgery of the First Affiliated Hospital of Soochow University; all samples were sequenced using a gene panel. The inclusion criteria were as follows: (I) postoperative pathological diagnosis of lung adenocarcinoma; (II) no preoperative oncology-related treatment; (III) no distant metastases were detected during preoperative examination. The study was conducted in accordance with the Declaration of Helsinki (as revised in 2013). The study was approved by institutional ethics board of the First Affiliated Hospital of Soochow University (No. 20202372). Individual consent for this retrospective analysis was waived. A total of 10 primary LUAD cases were identified as containing LRP1B mutation, and the other 10 cases as LRP1B WT. Sections were cut from formalin-fixed and paraffin-embedded LUAD tissue (4-µm thickness). Anti-CD4, anti-CD8, anti-PD-L1, and anti-CD68 staining were performed on tissue sections by multiplex immunohistochemistry (mIHC). The following antibodies were used: rabbit anti-CD4 (ZA0519, dilution 1:100), rabbit anti-CD8 (ZA0508, dilution 1:100), rabbit anti-PD-L1 (ZA0629, dilution 1:25), and mouse anti-CD68 (ZM0060, dilution 1:500). Antibodies were purchased from ZSGB-BIO (Beijing, China). Briefly, the sections were dewaxed with xylene, hydrated in 100%, 95%, 90%, 85%, 80%, and 75% ethanol for 5 minutes, and subsequently hydrated with distilled water. The antigen repair solution in the kit was used for microwave antigen repair, followed by incubation with primary antibody at 37 ℃ for 1 hour, washing, and incubation with secondary antibody at 37 ℃ for 10 minutes. Finally, 4',6-diamidino-2-phenylindole (DAPI) staining was performed at 25 ℃ for 5–10 minutes before sealing the sections. Table S2 provides information on the antibodies and their dilution concentrations. The mIHC staining was quantified digitally using HALO image analysis software (Indica Labs, Albuquerque, NM, USA).
Construction of a nomogram for predicting PFS
In the ICIs cohort, univariate and multivariate Cox regression analysis of PFS was performed using the survival package in R. A nomogram was constructed using rms in R to predict the probability of PFS at a specific time point; survROC and calibrate were used to determine the predictive power.
Statistical analysis
All analyses and statistical tests were conducted using the R software (version 4.1.1; The R Foundation for Statistical Computing, Vienna, Austria). Differences in the continuous variables between the 2 groups were evaluated by the Wilcoxon test, including TMB, NAL, immune gene expression, the scores of homologous recombination (HR) defects, and cytolytic activity. Differences in mutation frequency and clinical response to immunotherapy between the LRP1B-MT and LRP1B-WT groups were assessed by chi-squared test. The Kaplan-Meier (KM) and log-rank test were used to analyze survival-related data. All tests were set with P<0.05 indicating statistical significance.
Results
LRP1B is highly mutated in LUAD patients and is associated with multiple immune-related pathways
In this study, we found a high mutation frequency of LRP1B in the ICIs and TCGA-LUAD cohorts, 25% and 36%, respectively, and most of them were missense mutations. In the TCGA-LUAD cohort, the lollipop plot showed a higher number of splice mutations at the X470 splice/V470F mutation site (Figure 1A,1B). To further explore the functional impact caused by the LRP1B mutation, we analyzed the genes that were differentially expressed genes (DEGs) between the LRP1B-MT group and LRP1B-WT group in the TCGA-LUAD cohort, with 586 genes significantly up-regulated and 361 genes significantly down-regulated (Figure 1C). GO and KEGG enrichment analysis demonstrated that these DEGs were significantly enriched in 39 GO pathways and 1 KEGG pathway, including T cell differentiation and activation involved in immune response, T cell activation, immune response−activating cell surface receptor, which are closely related to the immune microenvironment (Figure 1D). We performed GSEA to further explore the pathways enriched by these DEGs. We found that DNA damage repair (DDR)-related pathways, such as cell cycle, DNA replication, HR, mismatch repair (MMR), and regulation of DNA repair, were significantly enriched in the LRP1B-MT group. In the TIME, the notch signaling pathway, PD-L1 expression, and the PD-1 checkpoint pathway in cancer were upregulated in the LRP1B-MT group. In contrast, some negative immune regulation pathways, including linoleic acid metabolism, arachidonic acid metabolism, fatty acid metabolism, and interleukin-6 production were enriched in the LRP1B-WT group. Additionally, we found that the activities of oncogenic signaling pathways such as sprouting angiogenesis were markedly increased in LRP1B-MT LUAD patients (Figure 1E,1F). These results indicate that LRP1B mutation may have a positive impact on antitumor immunity.
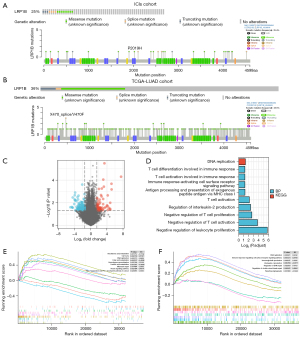
Mutation landscape in LRP1B-mutated LUAD patients
We explored the differences in mutation driver genes under different LRP1B mutation statuses. The top 20 genes are demonstrated in Figure 2A. Clinical information for the LRP1B-MT and LRP1B-WT groups in the ICIs and TCGA-LUAD cohorts is displayed in Figure 2B. In the ICIs cohort, the top 5 mutated genes were TP53 (46%), RYR2 (42%), TTN (41%), KRAS (39%), and MUC16 (36%). There was a significant increase in mutation frequency of RYR2 (93% vs. 25%, P<0.001) and ANK2 (60% vs. 6%, P<0.001) in the LRP1B-MT group compared with the LRP1B-WT group. In the TCGA-LUAD cohort, the top 20 mutated genes were similar to those in the ICIs cohort, except for CSMD1, COL11A1, ZNF536, and NAV3. Figure 2C,2D show the co-occurrence and mutual exclusion analysis of the top 20 mutated genes in the ICIs and TCGA-LUAD cohorts. Figure 2E,2F show that 1q21.3 and 14q13.1 amplifications were enriched in the LRP1B-MT group. Additionally, the LRP1B-WT group had more amplifications on chromosomes 8 and 12, such as 8q24.21 and 12q15. The detailed mutation frequencies in the ICIs cohort are provided in Table S3.
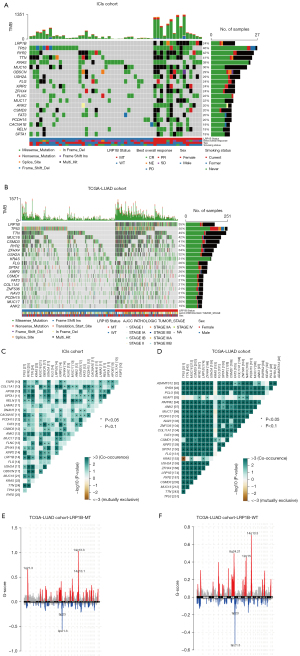
TIME in LRP1B-mutated LUAD patients
The outcomes of patients undergoing ICIs were correlated with TIME, and the components in the TIME can be influenced by the somatic mutation of specific genes. We compared the TIME with different LRP1B mutation statuses in the TCGA-LUAD cohort. Figure 3A shows the expression of some immune activation-related genes, such as TAP1 (antigen processing gene) and MICB (antigen presentation gene), RALGPS2 (B cell-related gene), CD8A and GZMB (cytolytic activity gene), CXCL9 and CXCL10 (chemokines), and IFN-γ, ICAM1, and TNF (proinflammatory regulators). Their expression markedly increased in the LRP1B-MT group. In addition, Figure 3B shows that immune checkpoint biomarkers, including CD274, LAG3, EDNRB, and PDCD1, displayed increased expression in the LRP1B-MT group than those in the LRP1B-WT group. Additionally, we compared the score of immune signatures. Compared with the LRP1B-WT group, the scores of HR defects and cytolytic activity of the LRP1B-MT group were significantly higher (Figure 3C). Investigation of 22 immune cells in the TCGA-LUAD cohort demonstrated that activated immune cells, including M0 macrophages, M1 macrophages, CD8+ T cells, and activated CD4+ memory T cells were significantly enriched in the TIME of LRP1B-MT group (Figure 3D). In contrast, there were more immune cells with a resting function: CD4+ memory T, dendritic, and mast cells were all static in the TIME of the LRP1B-WT group. This suggests that LRP1B mutation may have an impact on the TIME, enhancing the immune-related pathway gene expression and facilitating the infiltration of activated immune cells.
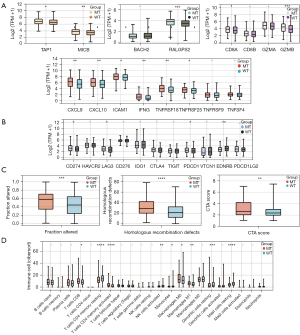
Analysis of immune infiltration and PD-L1 expression in LRP1B-mutated LUAD patients in clinical specimens
To validate the bioinformatic analysis of the TIME in LRP1B-mutated LUAD patients, we performed mIHC analysis on clinically collected LUAD specimens. Typical images of immunostaining for CD8 and CD4 in the LRP1B MT and WT groups of LUAD are shown in Figure 4A,4B. The results showed an elevated level of CD8+ T cell infiltration in LRP1B-MT patients than that in LRP1B-WT patients (Figure 4C, P<0.01). However, no marked differences were observed in the CD4+ T cell infiltration levels (Figure 4D, P=0.14). Furthermore, Figure 5A,5B show higher PD-L1 expression in tumor tissues with the LRP1B mutation (P<0.01), which is similar to the results of our bioinformatics analysis. Although there was no significant difference in the total number of macrophages (P=0.25), PD-L1+ CD68+ macrophages had a higher level of infiltration in LRP1B-MT patients (P<0.05).
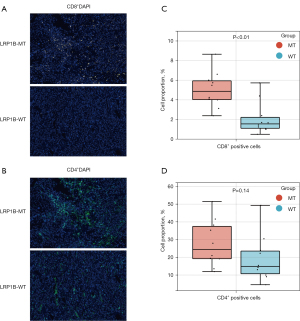
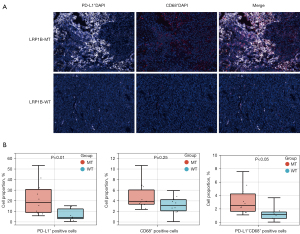
Analysis of immunogenicity and DDR mutations in LRP1B-mutated LUAD patients
High immunogenicity benefits patients receiving immunotherapy, and TMB is regarded as an independent predictive biomarker. First, we compared the differences in TMB under different LRP1B mutation statuses. A markedly higher TMB level was observed in the LRP1B-MT group in both the ICIs and TCGA-LUAD cohorts (Figure 6A,6B). Additionally, we investigated the NAL in the ICIs and TCGA-LUAD cohorts. Markedly higher NAL in the LRP1B-MT group was observed than in the LRP1B-WT group (Figure 6C,6D). The DDR pathway is closely associated with genomic instability. In the ICIs cohort, mutation counts in the DDR-related pathways [base excision repair (BER), double-strand break (DSB) repair, Fanconi anemia (FA), HR, MMR, nucleotide excision repair (NER), non-homologous end-joining (NHEJ), and single-stranded DNA binding (SSB)] were statistically higher in the LRP1B-MT group (both P<0.05), except for HR (P=0.09) (Figure 6E). Figure 6F suggests that in the TCGA-LUAD cohort, LUAD patients with LRP1B-MT had higher mutations in all 8 DDR-related pathways compared to those with LRP1B-WT (both P<0.05).
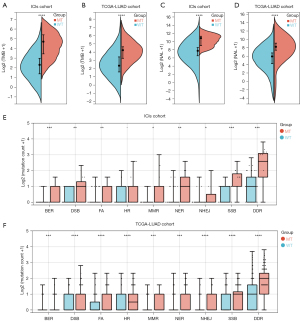
LRP1B mutation improves the prognosis of ICIs-treated LUAD patients
The baseline data for patients in the ICIs cohort are demonstrated in Table 1. We conducted KM analysis to investigate the association between LRP1B mutation and the outcome of LUAD patients treated with ICIs. PFS was significantly prolonged (P=0.0016) for LRP1B-MT patients in comparison to LRP1B-WT patients (Figure 7A). Nonetheless, no significant difference was observed in PFS (P=0.43; Figure 7B) or OS (P=0.16; Figure 7C) between the LRP1B-MT and LRP1B-WT groups in the TCGA-LUAD cohort in which no patients received ICIs treatment. We observed that LRP1B-MT patients had a significantly higher proportion of durable clinical benefits (P=0.03; Figure 7D) and best overall response (P=0.02; Figure 7E) compared with LRP1B-WT patients in the ICIs cohort. According to these results, LRP1B mutation can be instrumental in improving the prognosis of patients treated with ICIs by affecting the TIME.
Table 1
Characteristic | LRP1B-WT (n=44) | LRP1B-MT (n=15) | P value |
---|---|---|---|
Age, years, n (%) | 0.030 | ||
>65 | 25 (42.4) | 3 (5.1) | |
≤65 | 19 (32.2) | 12 (20.3) | |
Sex, n (%) | 0.499 | ||
Female | 26 (44.1) | 11 (18.6) | |
Male | 18 (30.5) | 4 (6.8) | |
Best overall response, n (%) | 0.018 | ||
CR | 2 (3.4) | 2 (3.4) | |
NE | 3 (5.1) | 0 (0) | |
PD | 14 (23.7) | 1 (1.7) | |
PR | 7 (11.9) | 8 (13.6) | |
SD | 18 (30.5) | 4 (5.1) | |
Durable clinical benefit, n (%) | 0.030 | ||
Durable clinical benefit | 19 (32.2) | 12 (20.3) | |
No durable benefit | 25 (42.4) | 3 (5.1) | |
NAL, n (%) | <0.001 | ||
High | 16 (27.1) | 14 (23.7) | |
Low | 28 (47.5) | 1 (1.7) | |
TMB, n (%) | <0.001 | ||
High | 16 (27.1) | 14 (23.7) | |
Low | 28 (47.5) | 1 (1.7) |
ICIs, immune checkpoint inhibitors; WT, wild type; MT, mutated type; CR, complete response; NE, not evaluated; PD, progressive disease; PR, partial response; SD, stable disease; NAL, neoantigen load; TMB, tumor mutational burden.
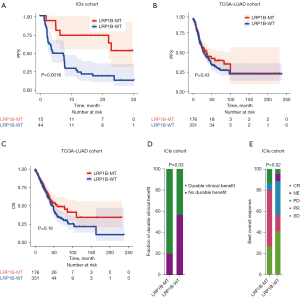
Nomogram predicts short-term PFS in LUAD patients treated with ICIs
We firstly performed a univariate regression Cox analysis to explore the association between clinical factors and PFS in patients with LUAD. Figure 8A shows that in the univariate analysis, NAL [hazard ratio (HR) =0.491, 95% CI: 0.261–0.925, P=0.028] and LRP1B status (HR =0.265, 95% CI: 0.110–0.638, P=0.003) can predict the prognosis of LUAD patients. After adjusting for age, sex, NAL, TMB, and LRP1B status by multivariate Cox regression analysis, we found that only the age (HR =0.208, 95% CI: 1.035–4.179, P=0.04) and LRP1B status (HR =0.232, 95% CI: 0.084–0.645, P=0.005) can be considered independent predictors in the ICIs cohort (Figure 8B). We constructed a nomogram to predict the probability of PFS at 1 and 2 years for LUAD patients treated with ICIs (Figure 8C). The receiver operating characteristic (ROC) curve illustrates the reliable predictive power of the nomogram with area under the curve (AUC) values of 0.823 and 0.840 for 1 and 2 years, respectively (Figure 8D). The 1- and 2-year calibration curve for this nomogram also performs well (Figure 8E). Based on the above results, we believe that the constructed nomogram can help predict the short-term PFS probability for LUAD patients treated with ICIs.
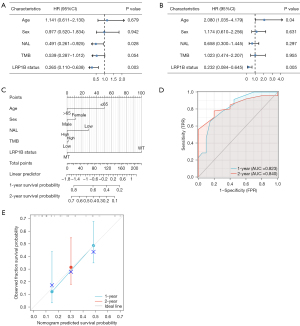
Discussion
The clinical application of immunotherapy in patients with LUAD has yielded remarkable results. It has been shown to improve overall survival in LUAD patients with few side effects and a long duration of action, even months or years after treatment has been stopped (33). In a retrospective cohort study of stage III melanoma, PD-1 immunotherapy significantly reduced regional recurrence and systemic metastasis rates compared to conventional therapy, suggesting that immunotherapy also plays an important role in reducing tumor micrometastasis. Nevertheless, many patients do not benefit from immunotherapy. It is noteworthy that neither PD-L1 expression nor TMB is an accurate predictor of whether a patient will benefit from immunotherapy (34). In our study, we discovered that LRP1B mutation was associated with known immunotherapy-related biomarkers, such as CD8+ T cell infiltration, pre-treatment expression of cytotoxic genes, and interferon-gamma (IFN-γ) (16). In addition, LRP1B mutations could enhance immunogenicity, including TMB, NAL, and mutations in DDR-related pathways, both in the ICIs and TCGA-LUAD cohorts.
Changes in the TIME have been demonstrated to influence the response to immunotherapy. Inflammatory TIME is associated with a high response rate to receive immunotherapy (35). The presence of a large number of tumor infiltrating lymphocytes, high density of CD8+ T cells and high expression of PD-L1 allows the patient to respond well to immunotherapy. Previous studies have suggested that immunotherapy works primarily by revitalizing the antitumor immune effect mediated by T cells (36). Among various cancer types, a higher density of CD8+ T cells usually indicates a better response to PD-1/PD-L1 blockade as well as longer survival, especially in the tumor core and the invasive margin (37,38). CD4+ T cells also play a key role in tumor immunotherapy. Several subpopulations of CD4+ Th1 cells have been shown to be more enriched in melanoma that responded to CTLA-4 blockade (39). In addition, activated M1 macrophages can promote CD8+ T cell activation via antigen presentation and secretion of cytokines (40). Our mIHC analysis revealed that higher levels of PD-L1+ macrophages (labeled by anti-CD68) infiltration could be observed in LUAD with LRP1B MT than that with LRP1B WT. The higher proportion of PD-L1+CD68+ macrophages was indicated for better immunotherapy outcomes in melanoma (41) and NSCLC (42). The pivotal position of T cells in immunotherapy depends on the interaction of IFN-γ in TIME components (16) secreted by cytotoxic T cells, Th1 cells, and natural killer (NK) cells; IFN-γ enhances the cytotoxicity of CD8+ T cells and NK cells, promotes antigen presentation and activates M1 macrophages (16). Also, it inhibits the growth of tumor cells and promotes their apoptosis (43). IFN-γ can upregulate PD-L1 expression on adjacent cells, which is related to a higher response rate in NSCLC patients who receive PD-1/PD-L1 blockade (44). Our study found that the LRP1B-MT group had more immune cell infiltration and higher INF-γ expression than the LRP1B-WT group, implying that the LRP1B mutation may be able to enhance LUAD patient response to immunotherapy by affecting the TIME in LUAD patients.
Moreover, CXCL9 and CXLC10 are secreted by T cells and tumor cells due to the positive feedback mechanism generated by INF-γ, thus increasing the infiltration of cytotoxic lymphocytes and NK cells in the TIME (45). Some studies have reported that the pre-treatment expression of cytotoxic genes (CD8A, GZMB) was associated with PD-1 blockade (46). Consistent with other studies, the expression of immune checkpoint genes (CD274 and PDCD1) was positively associated with the prognosis of immunotherapy (47). Although high expression of EDNRB and LAG3 is associated with poor prognosis in LUAD, but they are potential targets for the ICIs treatment (48-50). A study has also shown that the differential expression of immunotherapy-related genes in LUAD is related to the TMB and tumor immune cell infiltration (51). Therefore, LRP1B mutation can promote the secretion of inflammatory mediators and chemokines to recruit and enhance immune cytotoxicity while upregulating the expression of immune genes and ultimately enhancing the antitumor activity of the organism itself.
Genomic instability leads to the development of high immunogenicity, which is important for predicting clinical benefits for ICIs-treated patients (52). Studies have shown that high levels of TMB effectively predict the clinical benefit of ICIs, and LRP1B mutation status has also been reported to be closely associated with TMB levels (53). At the same time, certain somatic mutations in the genome can induce NAL to be expressed on the surface of tumor cells, thereby making NAL another important marker associated with the efficacy of immunotherapy (54). We found that LRP1B mutation was positively related to higher TMB and elevated NAL, which suggests that LRP1B mutation is a prognostic biomarker for ICIs-treated LUAD patients.
Mutations in LRP1B-MT patients were found to occur more frequently in genes related to DDR pathways. The normal activity of the DDR pathway can repair most DNA damage, but when genes in the pathway are altered, it will cause mis-repair or delayed repair, which will eventually lead to genomic instability (55). Genetic instability is associated with an activated immune microenvironment. In a study of colorectal cancer, it was found that MMR-deficient tumor tissues had a higher density of CD8+ lymph-like cells and a higher proportion of PD-L1 positive cells compared to MMR-normal tumor tissues (56). The accumulated defects in the DDR machinery can initiate the interferon gene (STING) pathway, which is associated with better response to ICIs (57,58). As expected, GSEA revealed that the major DDR pathway was significantly enriched in the LRP1B-MT group compared to in the LRP1B-WT group. Also, pathways related to NOTCH signaling, cell cycle, and immunoglobulin production were highly enriched in the LRP1B-MT group. Among them, the NOTCH signaling pathway not only regulates the functional state of immune cells but also determines their differentiation. The DLL1-NOTCH1/2 axis drives the differentiation of naïve CD8+ T cells to effector T cells by regulating the expression of granzyme B and perforin and can suppress tumor growth (59).
Recent reports have shown that lipid metabolism is associated with poorer immunotherapy outcomes. For example, cholesterol can inhibit T cell receptor signaling leading to T cell depletion (60). Our research examined the differences between the LRP1B-MT and LRP1B-WT group regarding pathways related to lipid metabolism. Among them, the fatty acid metabolic pathway was drastically downregulated in LRP1B-MT LUAD patients, predicting tumor progression and metastasis (61). In addition, interleukin-6, a pleiotropic pro-inflammatory factor, is considered a poor prognostic factor for T cell-mediated antitumor immunity ascribable to its role in inhibiting the conversion of CD4+ T cells into effector TH1 cells capable of secreting IFN-γ, and the interleukin-6-related pathway was significantly enriched in the LRP1B-WT group (62).
The common criteria used to evaluate immunotherapy include objective remission rates based on RECIST 1.1 criteria, progression-free survival, overall survival, and treatment-related adverse effects. By analyzing the ICIs and TCGA-LUAD cohorts, we noticed that the LUAD patients with LRP1B mutation had a better PFS and a higher proportion of durable clinical benefit after receiving ICIs treatment than LRP1B-WT patients in the ICIs cohort. However, we did not observe similar results in the TCGA-LUAD cohort. These results demonstrate that LRP1B mutation may enhance patients’ response to ICIs treatment by altering the TIME, providing evidence for LRP1B mutation as a prognostic biomarker in ICIs treatment. Our study provides a new clinical treatment option for LRP1B-mutated LUAD patients, but there are a few limitations. First, our mIHC cohort had a limited sample size and lacked RNA-seq data. To compensate for this limitation, we introduced the TCGA-LUAD cohort to compare the differences in TIME between the LRP1B-MT and LRP1B-WT groups. Second, although our preliminary results found that the LRP1B mutation may play a role in regulating the TIME, the molecular mechanism warrants further studies.
Conclusions
In conclusion, from a TIME perspective, our study explored the relationship between LRP1B mutation and clinical benefit of ICIs therapy in LUAD patients. Our results show that LRP1B mutation is a potential prognostic biomarker for ICIs treatment of LUAD. We believe that for patients with intermediate to advanced LUAD, the detection of LRP1B mutation should be included among the criteria for assessing the suitability of patients for ICIs treatment, in addition to testing for PD-L1 expression and TMB or NAL.
Acknowledgments
Funding: This work was supported by grants from the Major Project of Basic Science (Natural Science) Research in Jiangsu Higher Education Institutions in 2021 (No. 21KJA310003) and the Nurturing Project of Health Talents in Gusu district (No. GSWS2019027).
Footnote
Reporting Checklist: The authors have completed the REMARK reporting checklist. Available at https://tlcr.amegroups.com/article/view/10.21037/tlcr-23-39/rc
Data Sharing Statement: Available at https://tlcr.amegroups.com/article/view/10.21037/tlcr-23-39/dss
Peer Review File: Available at https://tlcr.amegroups.com/article/view/10.21037/tlcr-23-39/prf
Conflicts of Interest: All authors have completed the ICMJE uniform disclosure form (available at https://tlcr.amegroups.com/article/view/10.21037/tlcr-23-39/coif). The authors have no conflicts of interest to declare.
Ethical Statement: The authors are accountable for all aspects of the work in ensuring that questions related to the accuracy or integrity of any part of the work are appropriately investigated and resolved. The study was conducted in accordance with the Declaration of Helsinki (as revised in 2013). The study was approved by institutional ethics board of the First Affiliated Hospital of Soochow University (No. 20202372). Individual consent for this retrospective analysis was waived.
Open Access Statement: This is an Open Access article distributed in accordance with the Creative Commons Attribution-NonCommercial-NoDerivs 4.0 International License (CC BY-NC-ND 4.0), which permits the non-commercial replication and distribution of the article with the strict proviso that no changes or edits are made and the original work is properly cited (including links to both the formal publication through the relevant DOI and the license). See: https://creativecommons.org/licenses/by-nc-nd/4.0/.
References
- Nasim F, Sabath BF, Eapen GA. Lung Cancer. Med Clin North Am 2019;103:463-73. [Crossref] [PubMed]
- Bray F, Ferlay J, Soerjomataram I, et al. Global cancer statistics 2018: GLOBOCAN estimates of incidence and mortality worldwide for 36 cancers in 185 countries. CA Cancer J Clin 2018;68:394-424. [Crossref] [PubMed]
- Gridelli C, Rossi A, Carbone DP, et al. Non-small-cell lung cancer. Nat Rev Dis Primers 2015;1:15009. [Crossref] [PubMed]
- Hirsch FR, Scagliotti GV, Mulshine JL, et al. Lung cancer: current therapies and new targeted treatments. Lancet 2017;389:299-311. [Crossref] [PubMed]
- Siegel RL, Miller KD, Fuchs HE, et al. Cancer Statistics, 2021. CA Cancer J Clin 2021;71:7-33. [Crossref] [PubMed]
- Saynak M, Veeramachaneni NK, Hubbs JL, et al. Local failure after complete resection of N0-1 non-small cell lung cancer. Lung Cancer 2011;71:156-65. [Crossref] [PubMed]
- Sangha R, Price J, Butts CA. Adjuvant therapy in non-small cell lung cancer: current and future directions. Oncologist 2010;15:862-72. [Crossref] [PubMed]
- Ilie M, Long-Mira E, Bence C, et al. Comparative study of the PD-L1 status between surgically resected specimens and matched biopsies of NSCLC patients reveal major discordances: a potential issue for anti-PD-L1 therapeutic strategies. Ann Oncol 2016;27:147-53. [Crossref] [PubMed]
- Rimm DL, Han G, Taube JM, et al. A Prospective, Multi-institutional, Pathologist-Based Assessment of 4 Immunohistochemistry Assays for PD-L1 Expression in Non-Small Cell Lung Cancer. JAMA Oncol 2017;3:1051-8. [Crossref] [PubMed]
- Endris V, Buchhalter I, Allgäuer M, et al. Measurement of tumor mutational burden (TMB) in routine molecular diagnostics: in silico and real-life analysis of three larger gene panels. Int J Cancer 2019;144:2303-12. [Crossref] [PubMed]
- Fridman WH, Remark R, Goc J, et al. The immune microenvironment: a major player in human cancers. Int Arch Allergy Immunol 2014;164:13-26. [Crossref] [PubMed]
- Liu L, Xu S, Huang L, et al. Systemic immune microenvironment and regulatory network analysis in patients with lung adenocarcinoma. Transl Cancer Res 2021;10:2859-72. [Crossref] [PubMed]
- Fridman WH, Zitvogel L, Sautès-Fridman C, et al. The immune contexture in cancer prognosis and treatment. Nat Rev Clin Oncol 2017;14:717-34. [Crossref] [PubMed]
- Petitprez F, Vano YA, Becht E, et al. Transcriptomic analysis of the tumor microenvironment to guide prognosis and immunotherapies. Cancer Immunol Immunother 2018;67:981-8. [Crossref] [PubMed]
- Fridman WH, Miller I, Sautès-Fridman C, et al. Therapeutic Targeting of the Colorectal Tumor Stroma. Gastroenterology 2020;158:303-21. [Crossref] [PubMed]
- Petitprez F, Meylan M, de Reyniès A, et al. The Tumor Microenvironment in the Response to Immune Checkpoint Blockade Therapies. Front Immunol 2020;11:784. [Crossref] [PubMed]
- Liu CX, Musco S, Lisitsina NM, et al. LRP-DIT, a putative endocytic receptor gene, is frequently inactivated in non-small cell lung cancer cell lines. Cancer Res 2000;60:1961-7. [PubMed]
- Su S, Lin A, Luo P, et al. Effect of mesenchymal-epithelial transition amplification on immune microenvironment and efficacy of immune checkpoint inhibitors in patients with non-small cell lung cancer. Ann Transl Med 2021;9:1475. [Crossref] [PubMed]
- Brown LC, Tucker MD, Sedhom R, et al. LRP1B mutations are associated with favorable outcomes to immune checkpoint inhibitors across multiple cancer types. J Immunother Cancer 2021;9:e001792. [Crossref] [PubMed]
- Cheng Y, Tang R, Li X, et al. LRP1B is a Potential Biomarker for Tumor Immunogenicity and Prognosis of HCC Patients Receiving ICI Treatment. J Hepatocell Carcinoma 2022;9:203-20. [Crossref] [PubMed]
- Chen H, Chong W, Wu Q, et al. Association of LRP1B Mutation With Tumor Mutation Burden and Outcomes in Melanoma and Non-small Cell Lung Cancer Patients Treated With Immune Check-Point Blockades. Front Immunol 2019;10:1113. [Crossref] [PubMed]
- Hellmann MD, Nathanson T, Rizvi H, et al. Genomic Features of Response to Combination Immunotherapy in Patients with Advanced Non-Small-Cell Lung Cancer. Cancer Cell 2018;33:843-852.e4. [Crossref] [PubMed]
- Colaprico A, Silva TC, Olsen C, et al. TCGAbiolinks: an R/Bioconductor package for integrative analysis of TCGA data. Nucleic Acids Res 2016;44:e71. [Crossref] [PubMed]
- Cerami E, Gao J, Dogrusoz U, et al. The cBio cancer genomics portal: an open platform for exploring multidimensional cancer genomics data. Cancer Discov 2012;2:401-4. [Crossref] [PubMed]
- Asmann YW, Parikh K, Bergsagel PL, et al. Inflation of tumor mutation burden by tumor-only sequencing in under-represented groups. NPJ Precis Oncol 2021;5:22. [Crossref] [PubMed]
- Thorsson V, Gibbs DL, Brown SD, et al. The Immune Landscape of Cancer. Immunity 2018;48:812-830.e14. [Crossref] [PubMed]
- Mayakonda A, Lin DC, Assenov Y, et al. Maftools: efficient and comprehensive analysis of somatic variants in cancer. Genome Res 2018;28:1747-56. [Crossref] [PubMed]
- Gu Z, Eils R, Schlesner M. Complex heatmaps reveal patterns and correlations in multidimensional genomic data. Bioinformatics 2016;32:2847-9. [Crossref] [PubMed]
- Reich M, Liefeld T, Gould J, et al. GenePattern 2.0. Nat Genet 2006;38:500-1. [Crossref] [PubMed]
- Newman AM, Liu CL, Green MR, et al. Robust enumeration of cell subsets from tissue expression profiles. Nat Methods 2015;12:453-7. [Crossref] [PubMed]
- Ritchie ME, Phipson B, Wu D, et al. limma powers differential expression analyses for RNA-sequencing and microarray studies. Nucleic Acids Res 2015;43:e47. [Crossref] [PubMed]
- Subramanian A, Tamayo P, Mootha VK, et al. Gene set enrichment analysis: a knowledge-based approach for interpreting genome-wide expression profiles. Proc Natl Acad Sci U S A 2005;102:15545-50. [Crossref] [PubMed]
- Gettinger SN, Horn L, Gandhi L, et al. Overall Survival and Long-Term Safety of Nivolumab (Anti-Programmed Death 1 Antibody, BMS-936558, ONO-4538) in Patients With Previously Treated Advanced Non-Small-Cell Lung Cancer. J Clin Oncol 2015;33:2004-12. [Crossref] [PubMed]
- Rossi G, Russo A, Tagliamento M, et al. Precision Medicine for NSCLC in the Era of Immunotherapy: New Biomarkers to Select the Most Suitable Treatment or the Most Suitable Patient. Cancers (Basel) 2020;12:1125. [Crossref] [PubMed]
- Lin A, Wei T, Meng H, et al. Role of the dynamic tumor microenvironment in controversies regarding immune checkpoint inhibitors for the treatment of non-small cell lung cancer (NSCLC) with EGFR mutations. Mol Cancer 2019;18:139. [Crossref] [PubMed]
- Sharma P, Allison JP. The future of immune checkpoint therapy. Science 2015;348:56-61. [Crossref] [PubMed]
- Herbst RS, Soria JC, Kowanetz M, et al. Predictive correlates of response to the anti-PD-L1 antibody MPDL3280A in cancer patients. Nature 2014;515:563-7. [Crossref] [PubMed]
- Cheng X, Wang L, Zhang Z. Prognostic significance of PD-L1 expression and CD8(+) TILs density for disease-free survival in surgically resected lung squamous cell carcinoma: a retrospective study. J Thorac Dis 2022;14:2224-34. [Crossref] [PubMed]
- Wei SC, Levine JH, Cogdill AP, et al. Distinct Cellular Mechanisms Underlie Anti-CTLA-4 and Anti-PD-1 Checkpoint Blockade. Cell 2017;170:1120-1133.e17. [Crossref] [PubMed]
- Murray PJ, Wynn TA. Protective and pathogenic functions of macrophage subsets. Nat Rev Immunol 2011;11:723-37. [Crossref] [PubMed]
- Toki MI, Merritt CR, Wong PF, et al. High-Plex Predictive Marker Discovery for Melanoma Immunotherapy-Treated Patients Using Digital Spatial Profiling. Clin Cancer Res 2019;25:5503-12. [Crossref] [PubMed]
- Liu Y, Zugazagoitia J, Ahmed FS, et al. Immune Cell PD-L1 Colocalizes with Macrophages and Is Associated with Outcome in PD-1 Pathway Blockade Therapy. Clin Cancer Res 2020;26:970-7. [Crossref] [PubMed]
- Akdis M, Aab A, Altunbulakli C, et al. Interleukins (from IL-1 to IL-38), interferons, transforming growth factor β, and TNF-α: Receptors, functions, and roles in diseases. J Allergy Clin Immunol 2016;138:984-1010. [Crossref] [PubMed]
- Garcia-Diaz A, Shin DS, Moreno BH, et al. Interferon Receptor Signaling Pathways Regulating PD-L1 and PD-L2 Expression. Cell Rep 2017;19:1189-201. [Crossref] [PubMed]
- Tokunaga R, Zhang W, Naseem M, et al. CXCL9, CXCL10, CXCL11/CXCR3 axis for immune activation - A target for novel cancer therapy. Cancer Treat Rev 2018;63:40-7. [Crossref] [PubMed]
- Inoue H, Park JH, Kiyotani K, et al. Intratumoral expression levels of PD-L1, GZMA, and HLA-A along with oligoclonal T cell expansion associate with response to nivolumab in metastatic melanoma. Oncoimmunology 2016;5:e1204507. [Crossref] [PubMed]
- Tomlins SA, Khazanov NA, Bulen BJ, et al. Development and validation of an integrative pan-solid tumor predictor of PD-1/PD-L1 blockade benefit. Commun Med (Lond) 2023;3:14. [Crossref] [PubMed]
- He Y, Yu H, Rozeboom L, et al. LAG-3 Protein Expression in Non-Small Cell Lung Cancer and Its Relationship with PD-1/PD-L1 and Tumor-Infiltrating Lymphocytes. J Thorac Oncol 2017;12:814-23. [Crossref] [PubMed]
- Freitas JT, Lopez J, Llorian C, et al. The immunosuppressive role of Edn3 overexpression in the melanoma microenvironment. Pigment Cell Melanoma Res 2021;34:1084-93. [Crossref] [PubMed]
- Wei F, Ge Y, Li W, et al. Role of endothelin receptor type B (EDNRB) in lung adenocarcinoma. Thorac Cancer 2020;11:1885-90. [Crossref] [PubMed]
- Zhang P, Wang W, Liu L, et al. Analysis of prognostic model based on immunotherapy related genes in lung adenocarcinoma. Sci Rep 2022;12:22077. [Crossref] [PubMed]
- Wang S, He Z, Wang X, et al. Antigen presentation and tumor immunogenicity in cancer immunotherapy response prediction. Elife 2019;8:e49020. [Crossref] [PubMed]
- Lan S, Li H, Liu Y, et al. Somatic mutation of LRP1B is associated with tumor mutational burden in patients with lung cancer. Lung Cancer 2019;132:154-6. [Crossref] [PubMed]
- Snyder A, Makarov V, Merghoub T, et al. Genetic basis for clinical response to CTLA-4 blockade in melanoma. N Engl J Med 2014;371:2189-99. [Crossref] [PubMed]
- Dunn GP, Old LJ, Schreiber RD. The three Es of cancer immunoediting. Annu Rev Immunol 2004;22:329-60. [Crossref] [PubMed]
- Le DT, Uram JN, Wang H, et al. PD-1 Blockade in Tumors with Mismatch-Repair Deficiency. N Engl J Med 2015;372:2509-20. [Crossref] [PubMed]
- Mouw KW, Goldberg MS, Konstantinopoulos PA, et al. DNA Damage and Repair Biomarkers of Immunotherapy Response. Cancer Discov 2017;7:675-93. [Crossref] [PubMed]
- Teo MY, Seier K, Ostrovnaya I, et al. Alterations in DNA Damage Response and Repair Genes as Potential Marker of Clinical Benefit From PD-1/PD-L1 Blockade in Advanced Urothelial Cancers. J Clin Oncol 2018;36:1685-94. [Crossref] [PubMed]
- Huang Y, Lin L, Shanker A, et al. Resuscitating cancer immunosurveillance: selective stimulation of DLL1-Notch signaling in T cells rescues T-cell function and inhibits tumor growth. Cancer Res 2011;71:6122-31. [Crossref] [PubMed]
- Wang F, Beck-García K, Zorzin C, et al. Inhibition of T cell receptor signaling by cholesterol sulfate, a naturally occurring derivative of membrane cholesterol. Nat Immunol 2016;17:844-50. [Crossref] [PubMed]
- Kim YS, Jung J, Jeong H, et al. High Membranous Expression of Fatty Acid Transport Protein 4 Is Associated with Tumorigenesis and Tumor Progression in Clear Cell Renal Cell Carcinoma. Dis Markers 2019;2019:5702026. [Crossref] [PubMed]
- Tsukamoto H, Fujieda K, Senju S, et al. Immune-suppressive effects of interleukin-6 on T-cell-mediated anti-tumor immunity. Cancer Sci 2018;109:523-30. [Crossref] [PubMed]
(English Language Editor: J. Jones)