The development and validation of a circulating tumor cells-based integrated model for improving the indeterminate lung solid nodules diagnosis
Highlight box
Key findings
• The combination of CTCs with radiomics could complement conventional clinical models in improving the individual management for patients with pulmonary pure-solid nodules.
What is known and what is new?
• The CTCs-based integrated model provided an effective and robust performance in pulmonary nodules diagnosis.
• Our study provides a new non-invasive and accurate method for early-stage lung cancer detection.
What is the implication, and what should change now?
• Multi-dimensional features were of great necessity for early-stage lung cancer detection.
Introduction
Increasing evidence demonstrates that compared with lung cancer manifesting as radiological subsolid nodules, those presenting as pure solid nodules have a higher rate of occult lymph node metastasis, more malignant behaviors, and poorer prognosis, even after surgical resection (1,2). The majority of pure solid lung cancers have reached an advanced stage at the time of diagnosis; hence, early detection is critical to effective treatment and long-term survival of these patients (3,4). However, early-stage lung cancer always manifests as solitary lung nodules without any typical symptoms, and the management of indeterminate pulmonary solid nodules (IPSNs) always involves an unacceptable rate of invasive diagnostic procedures for patients with benign disease, and associated morbidities of delayed therapies for those with malignant disease (5-8). Thus, accurate and timely diagnosis of IPSNs is important to reduce patient mortality and overtreatment.
Circulating tumor cells (CTCs) in the blood of patients with cancer, a subclass of tumor cells able to migrate from the primary site to nearby blood and/or lymphatic vessels and survive in the challenging micro-environment of the blood-stream, have gained increasing interest (9). The levels of CTCs could reflect biological aggressiveness (10,11); hence, it they are often isolated from the peripheral blood of patients with cancer for research in the diagnosis and prognosis of malignant tumors, with promising results (12-15). Nevertheless, in previous research, the potential role of CTCs testing for the diagnosis of nodules has been limited, with an area under the receiver operating characteristic (ROC) curve (AUC) no higher than 0.8, and with low sensitivity (30–89%) (16-18). Several recent studies have reported that the combination of CTCs and additional dimensional features (e.g., nodules radiological size, serum tumor marker) could significantly improve the differentiation ability of small lung nodules with high sensitivity and specificity (13,17,19). Besides, the combination of CTCs and artificial intelligence imaging was also shown to be an independent indicator for lung adenocarcinoma invasiveness in our previously published study (20). However, compared with traditional radiological features, radiomics has been shown to be a more effective clinical application tool for differentiating lung nodules in the early screening for lung cancer (21), since it can quickly extract a larger number of quantitative features from radiological images using high-throughput calculations (22-24). To date, there has been no study assessing the potential of integrated model constructed by incorporating quantitative radiomic signatures with CTCs in classifying IPSNs.
Here, we are aimed to develop a radio-biological model by combining clinical variables and radiomics features with the level of CTCs, and hypothesized that this integrated risk model might provide a novel insight into risk probability of IPSNs, complementing CTCs testing to form an effective platform for the early-stage lung cancer detection. We present the following article in accordance with the TRIPOD reporting checklist (available at https://tlcr.amegroups.com/article/view/10.21037/tlcr-23-145/rc).
Methods
Ethical statement
This study was approved by the Ethics Committees of Shanghai Pulmonary Hospital (No. L21-022) and The First Hospital of Lanzhou University (No. LDYYLL2023-40). The study conformed to the provisions of the Declaration of Helsinki (as revised in 2013). The requirement for written informed consent was waived for the retrospective cohort. The informed consent process is shown in (Appendix 1).
Patient selection
Figure 1 shows the flowchart of patient selection. Patients with indeterminate lung lesions at chest computed tomography (CT), who underwent pathological examination in Shanghai Pulmonary Hospital (SPH cohort) between January 2021 and April 2021 were retrospectively included in this study. All patients included in our study are solid nodules at chest CT, and the histopathological report. Patients with multiple lung lesions (n=970), subsolid lesions (n=1,754), and receiving any treatment before CTCs testing (n=31) were excluded; patients with radiological nodules larger than 30 mm (n=68) and no definitely pathological report (n=32) were also excluded. To develop an independent validation set, patients who underwent CTCs testing and pathological examination from August 2019 to July 2021 in the First Hospital of Lanzhou University were retrospectively enrolled. The entire inclusion and exclusion criteria are detailed in Figure 1. Clinical characteristics were retrospectively collected from medical records. The maximum value of standardized uptake value (SUVmax) of patients who underwent positron emission tomography/CT (PET-CT) examination one month before pathological examination was also collected.
Nodules segmentation and radiomics model construction
The detailed procedures of chest CT scanning and image acquisition are detailed in Supplementary Material (Appendix 2). Images of IPSNs were subjected to manual segmentation by 3 researchers (S.S., X.M., and H.H.) at the lung window setting [level, −450 Hounsfield unit (HU); width, 1,500 HU] using 3D-Slicer software, version 4.10.1 (www.slicer.org), and then verified and corrected by 2 senior researchers (M.Z. and Y.S.). Feature extraction was performed using the PyRadiomics package in Python, version 3.7 (www.python.org). Feature selection was conducted by sequentially applying minimum redundancy maximum relevance (mRMR) and least absolute shrinkage and selection operator (LASSO) techniques due to the high dimensionality and multicollinearity, which are described in Supplementary Material (Appendix 3). Finally, 12 radiomics features were selected successfully through the LASSO analysis (Figure S1A,S1B & Table S1), and a radiomics score was calculated for each patient. The relationship of radiomics score with nodules classification was illustrated in a waterfall plot (Figure S1C-S1E).
CTCs detection test
CTC testing was performed when patients were admitted to hospital for preoperative examination (about 2–3 days before surgery). The standard protocols of CTCs detection testing were employed as previously published (17,25,26), and the same method for CTCs detection was applied in the First Hospital of Lanzhou University. The peripheral venous blood sample (3 mL) of all patients enrolled in this study was collected via an EDTA-containing anti-coagulant tube (Becton, Dickinson and Company, NJ, USA) before surgical operation. The blood sample were temporarily stored at 4 °C and processed within 4 hours. CTCs were enriched and detected using CytoploRare® Detection Kit (GenoSaber Biotech, Shanghai, China). the enrichment of CTC was initially achieved by lysis of erythrocytes and remove of leukocytes, and then the enriched cells were incubated with detection probes at room temperature for forty minutes. After repeated washing to elute redundant detection probes, the remnant samples were amplified and quantified by fluorescent quantitative polymerase chain reaction (PCR) using ABI 7300 Real-Time PCR System (ThermoFisher, MA, USA). The same detection kit was adopted for the external validation set, and the results of detection test were retrospectively collected from medical records; 8.7 folate unit (FU)/3 mL was considered the optimal cutoff threshold for differential diagnosis of lung cancer based on kit instruction.
Study design
In this study, patients’ age, smoking history, prior extra-thoracic cancer, location of the nodule, speculation, and the nodule size were used to calculate a Mayo risk score (27). The integrated model incorporated estimated radiomics score as a single variable, Mayo risk score, and the levels of CTCs to yield a probability of malignancy by logistic regression analysis. The predictive efficacy of this integrated model was constructed on a basis of the training set, and evaluated in internal and independent external validation sets. The proportions of benign and malignant nodules that reside at different probability deciles from the integrated model and other 3 models alone were first compared, since the proportion of malignant and benign nodules in a specific malignancy probability decile provides information that may be helpful in clinical decision-making (28). The improvement value of the integrated model was then investigated by comparison with clinical assessment procedures. We also tested the performance of this integrated model in patients with different nodule sizes and intermediate risks. The net reclassification index (NRI) was applied to quantify the additional benefit for nodules risk classifications derived from the novel model.
Statistical analysis
Categorical variables were compared using Pearson’s chi-squared test or Fisher’s exact test, and continuous variables were analyzed using the independent t-test. The integrated model was developed by logistic regression analysis based on radiomics score, Mayo risk score, and the levels of CTCs and to calculate the malignant probability of each patient. Youden index (sensitivity + specificity − 1) was used to identify the optimal cut-off values in the Training set. During the internal and external validation of the model, the malignant probability for each patient in these two sets was calculated according to the established integrated model and performed logistic regression using the malignant probability as factor. The AUC was calculated from the regression analysis, and compared by the DeLong’s test. The specific performance metrics, including sensitivity, specificity, positive predictive value (PPV), negative predictive value (NPV), and accuracy at the threshold of 0.5 or higher, of each approach were calculated. An NRI >0 indicated significant improvement in malignant risk prediction of IPSNs.
All analyses were implemented by SPSS software (version 23.0; IBM Corp., Armonk, NY, USA), MedCalc software, (version 16.4; MedCalc, Ostend, Belgium), and R software (version 3.6.2, www.R-project.org) with the following R packages: mRMRe, glmnet, waterfalls, vioplot, pROC. A P value <0.05 was considered statistically significant.
Results
Baseline characteristics
A total of 519 patients with IPSNs from the SPH cohort were yielded for analysis, consisting of 291 males (56.1%) and 228 females (43.9%), with a median age of 61 years [interquartile range (IQR), 53–67 years]. Among them, 364 patients (70%) were randomly selected acting as a training set, including 81 benign nodules (22.3%) and 283 malignant nodules (77.7%); the remaining 155 patients (30%) were developed as an internal validation set, including 46 benign nodules (29.7%) and 109 malignant nodules (70.3%). The external test set included 52 males and 30 females, with a median age of 57 years (IQR, 52–63 years). Benign lesions were detected in 36 patients (43.9%) and malignant lesions were detected in 46 patients (56.1%). Of 215 patients undergoing PET-CT examination, 152 cases were in training set, and 63 in internal validation set.
In the training and internal validation set, the patients with malignant nodules seemed to be older {55 [50–64] vs. 63 [55–69] years, P<0.001 for the training test; 54 [47–65] vs. 62 [55–67] years, P=0.002 for the validation set}, have larger nodules size [15.6 (10.9–18.3) vs. 19.5 (14.3–24.4) mm, P<0.001 for the training test; 15.8 (10.8–19.8) vs. 19.5 (13.3–25.8) mm, P=0.002 for the internal validation set], and were more frequently diagnosed with a higher level of CTCs [9.0 (6.6–13.6) vs. 14.0 (9.9–18.9), P<0.001 for the training test; 10.7 (7.9–12.3) vs. 14.0 (9.7–16.8), P<0.001 for the internal validation set]. In the external validation set, the patients with malignant nodules were older, and were more frequently diagnosed with a higher level of CTCs. The clinical characteristics and radiological parameters are detailed in Table 1.
Table 1
Characteristics | SPH cohort (n=519) | External validation set (n=82) | |||||||||
---|---|---|---|---|---|---|---|---|---|---|---|
Training set (n=364) | Internal validation set (n=155) | ||||||||||
Benign (n=80) | Malignant (n=284) | P value | Benign (n=46) | Malignant (n=109) | P value | Benign (n=36) | Malignant (n=46) | P value | |||
Age at CT scans | 55 [50–64] | 63 [55–69] | <0.001 | 54 [47–65] | 62 [55–67] | 0.002 | 53 [49–58] | 60 [53–66] | 0.003 | ||
Gender (male) | 45 (55.6) | 165 (58.3) | 0.659 | 22 (47.8) | 59 (54.1) | 0.473 | 23 (63.9) | 29 (63.0) | 0.937 | ||
Current/former smoker | 6 (7.4) | 25 (8.8) | 0.685 | 1 (2.2) | 7 (6.4) | 0.275 | 18 (50.0) | 23 (50.0) | 1.000 | ||
History of malignant cancer | 2 (2.5) | 16 (5.7) | 0.244 | 4 (8.7) | 5 (4.6) | 0.318 | 0 | 0 | NA | ||
Radiological size | <0.001 | 0.007 | 0.957 | ||||||||
5≤ size ≤20 mm | 63 (78.8) | 147 (51.8) | 36 (78.3) | 56 (51.4) | 17 (47.2) | 22 (47.8) | |||||
20< size ≤30 mm | 17 (21.3) | 137 (48.2) | 10 (21.7) | 52 (47.7) | 19 (52.8) | 24 (52.2) | |||||
Median [IQR], mm | 15.6 [10.9–18.3] | 19.5 [14.3–24.4] | <0.001 | 15.8 [10.8–19.8] | 19.5 [13.3–25.8] | 0.002 | 19.3 [13.1–25.1] | 20.7 [15.2–24.0] | 0.991 | ||
Located in upper lobe | 39 (48.1) | 143 (50.5) | 0.705 | 18 (39.1) | 57 (52.3) | 0.134 | 20 (55.6) | 21 (45.7) | 0.373 | ||
CTCs (FU/3 mL) | 9.0 [6.6–13.6] | 14.0 [9.9–18.9] | <0.001 | 10.7 [7.9–12.3] | 14.0 [9.7–16.8] | <0.001 | 9.1 [6.9–12.6] | 10.5 [8.3–14.2] | 0.013 | ||
PET-CT examination | 26 (32.5) | 126 (44.4) | 0.057 | 15 (32.6) | 48 (44.0) | 0.186 | – | – | – | ||
Histology | <0.001 | <0.001 | <0.001 | ||||||||
Adenocarcinoma | – | 195 (68.9) | – | 74 (67.9) | – | 39 (84.8) | |||||
Squamous cell carcinoma | – | 38 (13.4) | – | 17 (15.6) | – | 4 (8.7) | |||||
Others malignant tumors | – | 50 (17.7) | – | 18 (16.5) | – | 3 (6.5) | |||||
Lung cancer probability, % | |||||||||||
Integrated risk model | 53.0 [33.8–74.0] | 81.8 [73.4–95.3] | <0.001 | 59.4 [43.0–75.1] | 83.5 [78.1–93.0] | <0.001 | 45.3 [29.5–56.8] | 64.6 [47.3–77.7] | <0.001 | ||
CTCs test prediction | 64.8 [52.3–80.2] | 79.1 [67.0–91.6] | <0.001 | 67.2 [58.2–76.1] | 78.5 [66.1–88.0] | <0.001 | 51.1 [41.3–60.4] | 60.0 [49.4–68.7] | 0.006 | ||
Mayo clinical model | 15.9 [5.0–19.1] | 28.9 [10.5–42.0] | <0.001 | 16.0 [4.4–22.6] | 28.9 [9.1–42.4] | 0.001 | 39.2 [15.2–63.0] | 43.1 [25.7–58.6] | 0.467 | ||
Radiomics model | 61.7 [44.1–79.5] | 79.2 [74.2–88.2] | <0.001 | 68.0 [54.7–83.6] | 80.3 [75.8–88.7] | <0.001 | 50.7 [37.5–64.6] | 60.3 [55.7–68.3] | 0.005 |
Values are numbers of patients with percentages in parentheses for categorical variables, and median with interquartile range [IQR] for continuous variable. P represents the statistical difference between training and test sets. SPH, Shanghai Pulmonary Hospital; CT, computed tomography; IQR, interquartile range; CTCs, circulating tumor cells; FU, functional unit; PET, positron emission tomography; NA, not applicable.
Development and validation of the integrated model
The distribution of malignant risk estimated by the models included in this study for each set are illustrated in Table 1. The integrated model correctly classified 32.5% of benign nodules and 97.4% of malignant nodules (Figure 2A) in the internal validation set, which was more specific than the CTCs test (15.2% vs. 99.1%), the radiomics model (17.4% vs. 96.4%), and the Mayo clinical model (93.4% vs. 19.3%, Figure S2A) at a probability threshold of 0.5. Similar trends were obtained for external validation set at the same threshold (Figure 2B and Figure S2B).
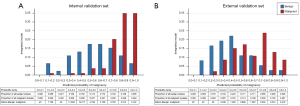
The integrated model achieved an AUC of 0.83 (0.78–0.88), 0.83 (0.75–0.91), and 0.76 (0.66–0.87) in the training, internal validation, and external validation sets, respectively (Figure 3A-3C). It outperformed the CTCs test [0.70 (0.62–0.79), P=0.001 and 0.68 (0.56–0.80), P=0.129 for the internal and external validation set], Mayo clinical model [0.68 (0.59–0.77), P<0.001; 0.55 (0.42–0.68), P=0.007], and radiomics model [0.72 (0.63–0.81), P=0.002; 0.67 (0.55–0.79), P=0.050], with a higher AUC (Figure 3A-3C). Comparison to PET-CT was restricted to 215 cases who had undergone PET-CT, and comparable performance with PET-CT examination was obtained by the integrated model (P=0.379, P=0.308 for training and internal validation set, respectively; Figure 3D,3E). The sensitivity, specificity, PPV, NPV, and accuracy achieved by the integrated model was 0.92 (0.88–0.95), 0.47 (0.36–0.59), 0.86 (0.82–0.90), 0.62 (0.49–0.74), and 0.82 (0.78–0.86) in the training set (Figure 3F), 0.97 (0.92–0.99), 0.33 (0.20–0.48), 0.77 (0.69–0.84), 0.83 (0.59–0.96), and 0.78 (0.71–0.84) in the internal validation set (Figure 3G), and 0.74 (0.59–0.86), 0.67 (0.49–0.81), 0.74 (0.59–0.86), 0.67 (0.49–0.81), and 0.71 (0.60–0.80) in the external test set at the threshold of 0.5 (Figure 3H), with detailed performance metrics for each model listed in Table S2. NRI analysis demonstrated that the integrated model provided net benefit for differentiation of IPSNs (Table S3).
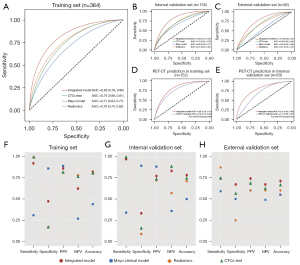
Performance of the integrated model for nodules with different radiological size
For IPSNs with size ≥5 and <20 mm, the integrated model showed a significant improvement in AUC of 0.09 (0.01–0.17; P=0.044, Figure 4A), 0.23 (0.03–0.42; P=0.026, Figure 4B) over CTCs testing, as well as the radiomics and Mayo clinical models, with a highest accuracy of 0.73 (0.63–0.82) and 0.62 (0.45–0.77) for internal and external validation set (Table 2). For IPSNs with a size ≥10 and <20 mm (Figure 4C,4D) and ≥20 and <30 mm (Figure 4E,4F), the performance of the integrated model was also superior to the other 3 models, with performance metrics detailed in Table S2. Net improvement for the diagnostic accuracy of IPSNs was found to be achieved by the integrated model across different subsets of nodules size in regard to NRI analysis (Table S3).
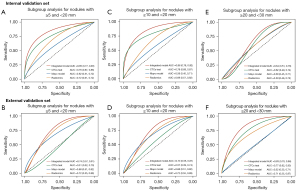
Table 2
Performance | Integrated model | Mayo clinical model | Radiomics | CTCs test |
---|---|---|---|---|
Nodules of size ≥5 and <20 mm | ||||
Internal validation set (n=92) (95% CI) | ||||
Sensitivity | 0.95 (0.85–0.99) | 0.02 (0.00–0.10) | 0.91 (0.80–0.97) | 0.98 (0.91–1.00) |
Specificity | 0.39 (0.23–0.57) | 1.00 (0.90–1.00) | 0.33 (0.19–0.51) | 0.11 (0.03–0.26) |
PPV | 0.71 (0.60–0.81) | 1.00 (0.03–1.00) | 0.68 (0.56–0.78) | 0.64 (0.53–0.74) |
NPV | 0.82 (0.57–0.96) | 0.40 (0.29–0.50) | 0.71 (0.44–0.90) | 0.80 (0.28–0.99) |
Accuracy | 0.73 (0.63–0.82) | 0.40 (0.30–0.51) | 0.68 (0.58–0.78) | 0.65 (0.54–0.74) |
External validation set (n=39) (95% CI) | ||||
Sensitivity | 0.55 (0.32–0.76) | 0.05 (0.00–0.23) | 0.68 (0.45–0.86) | 0.73 (0.50–0.89) |
Specificity | 0.71 (0.44–0.90) | 0.94 (0.71–1.00) | 0.65 (0.38–0.86) | 0.35 (0.14–0.62) |
PPV | 0.71 (0.44–0.90) | 0.50 (0.01–0.99) | 0.71 (0.48–0.89) | 0.59 (0.39–0.78) |
NPV | 0.55 (0.32–0.76) | 0.43 (0.27–0.61) | 0.61 (0.36–0.83) | 0.50 (0.21–0.79) |
Accuracy | 0.62 (0.45–0.77) | 0.56 (0.40–0.72) | 0.67 (0.50–0.81) | 0.56 (0.40–0.72) |
Intermediate risk nodules identified by Mayo clinical model (5%≤ risk probability <65%) | ||||
Internal validation set (n=115) (95% CI) | ||||
Sensitivity | 0.98 (0.92–1.00) | 0.13 (0.07–0.22) | 0.98 (0.92–1.00) | 0.99 (0.93–1.00) |
Specificity | 0.28 (0.14–0.47) | 0.94 (0.79–0.99) | 0.12 (0.04–0.29) | 0.19 (0.07–0.36) |
PPV | 0.78 (0.69–0.85) | 0.85 (0.55–0.98) | 0.74 (0.65–0.82) | 0.76 (0.67–0.84) |
NPV | 0.82 (0.48–0.98) | 0.29 (0.21–0.39) | 0.67 (0.22–0.96) | 0.86 (0.42–1.00) |
Accuracy | 0.78 (0.70–0.85) | 0.36 (0.27–0.45) | 0.74 (0.65–0.82) | 0.77 (0.66–0.84) |
External validation set (n=64) (95% CI) | ||||
Sensitivity | 0.72 (0.55–0.86) | 0.25 (0.12–0.42) | 0.83 (0.67–0.94) | 0.75 (0.58–0.88) |
Specificity | 0.64 (0.44–0.81) | 0.75 (0.55–0.89) | 0.39 (0.22–0.59) | 0.50 (0.31–0.69) |
PPV | 0.72 (0.55–0.86) | 0.56 (0.30–0.80) | 0.64 (0.49–0.77) | 0.66 (0.49–0.80) |
NPV | 0.64 (0.44–0.81) | 0.44 (0.29–0.59) | 0.65 (0.38–0.86) | 0.61 (0.39–0.80) |
Accuracy | 0.69 (0.56–0.80) | 0.47 (0.34–0.60) | 0.64 (0.51–0.76) | 0.64 (0.51–0.76) |
CTCs, circulating tumor cells; CI, confidence interval; PPV, positive predictive value; NPV, negative predictive value.
Performance of the integrated model for intermediate-risk nodules
Using the thresholds of 5% and 65%, the Mayo clinical model classified 115 cases (74.2%) from the internal validation set and 64 cases (78.0%) from the external validation set as intermediate risk nodules. Of 115 cases, 70.4% (81/115) were correctly classified as malignant nodules by the integrated model with an AUC of 0.80 (0.71–0.87; Figure 5A), a sensitivity of 0.98 (0.92–1.00) and an accuracy of 0.78 (0.70–0.85), showing superior performance to CTCs testing [AUC: 0.67 (0.58–0.76), P=0.010] and the radiomics model [AUC: 0.67 (0.58–0.76), P=0.008]. Similarly, in the external validation set, 56.3% (36/64) were correctly classified as malignant nodules with an AUC of 0.77 (0.64–0.86; Figure 5B), a sensitivity of 0.72 (0.55–0.86), and an accuracy of 0.69 (0.56–0.80), which maintained better performance than CTCs testing [AUC: 0.66 (0.53–0.77), P=0.098] and the radiomics model [AUC: 0.67 (0.54–0.78), P=0.069], even though no significantly statistical difference. The performance metrics was detailed in Table 2. The benefit of the integrated model in reclassifying nodules compared with the other 3 models is illustrated in Figure 5C-5H. NRI analysis demonstrated that the integrated model provided net benefit for classifying benign nodules form malignant nodules in intermediate risk subgroup (Table S3).
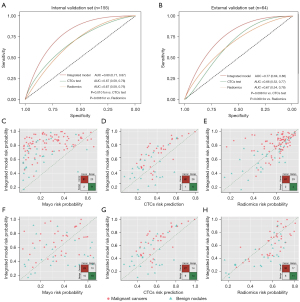
Discussion
In this study, the derivation and validation of a radio-biological integrated model constructed by combining radiomics features, Mayo risk score, and blood-based CTCs were reported for classifying and risk stratifying IPSNs in 601 patients from 2 Chinese hospitals. The integrated model showed a promising reclassification performance that was significantly better than CTCs testing prediction alone, and superior to that of the existing risk model (net reclassification of at least 17% on the external validation set compared with the Mayo clinical model). Further, the integrated model achieved performance not inferior to PET-CT for those who had undergone PET-CT. Robust performance with high sensitivity were also found across different solid sizes and malignant risk probabilities. To our best knowledge, this is the first study to integrate the level of CTCs, radiomics features, and clinical variables into a single risk model for malignant risk stratification of IPSNs in a large Chinese population, and to validate it in an independent external set.
Lung cancer remains the leading cause of cancer-related death worldwide. Early diagnosis can markedly improve the prognostic outcomes, and currently, 2 principal routes are widely used for the early lung cancer diagnosis. The first route is screening using low-dose computed tomography (LDCT), which was shown to reduce lung cancer-related deaths by 26% in the European NELSON trial (29). However, substantial pitfalls of LDCT have been discovered in clinical practice, despite the excellent sensitivity in detecting lung nodules. A major bottleneck is that LDCT generated exceptionally high false-positive results followed by biopsies to confirm the diagnostic findings, thereby leading to invasive biopsy-related complications, patient anxiety, and economic burden, preventing it from being an efficient tool for lung cancer screening (8). Another example is that the radiation risk related with annual LDCT screening is yet to be sufficiently resolved (30). The second route is the detection of a tumor as an incidental discovery in patients receiving chest CT examination for an unrelated reason. Multiple clinical risk models, such as the widely used Mayo clinical model (31), and Brock model (32), have been recommended to assist clinicians in the assessment of patients with incidentally diagnosed IPNs in management guidelines (33,34). However, the predictive value of these logistical regression-based methods is limited at least partly due to their reliance on qualitative, and hence inconsistent, human interpretation of radiological variable such as nodules size and morphology. Moreover, there was no specific recommended strategy provided for nodules with intermediate risk, which produce the highest rate of diagnostic errors and invasive procedures. Hence, a more efficient, and non-invasive or minimally invasive approach is critical to complement the existing routes for assessing cancer probability of IPNs, especially for those with intermediate risk.
A recently proposed method for the classifications of IPNs is liquid biopsy-based biomarker, and it has been considered an easier, safer, more-effective, and non-invasive tool for cancer diagnosis and treatment (10). CTCs, a liquid biopsy-based biomarker, have been increasingly studied as promising diagnostic or screening indicators for many types of malignant cancers, and have allowed researchers to reevaluate the inclusion of CTCs in the screening and diagnostic workflows due to the advancements in detection technology (35). Zhang et al. revealed that the detection of CTCs in peripheral blood was a reliable method to differentiate malignancy of indeterminate solitary lung nodules (13). Our previous study indicated that CTCs showed good performance in distinguishing benign from malignant of indeterminate solitary pulmonary nodules with AUC achieved 0.792 in validation set (17). Different from the previous study, our study distinguished between benign and malignant pulmonary nodules especially for solid nodules and combined with radiomics features and clinical variables. Consistent with previous studies, the CTCs test was found to achieve a better performance than the traditional Mayo clinical model with a higher AUC, sensitivity, and accuracy in the current study, indicating the significant value of CTCs in risk stratification for IPSNs. Nevertheless, using CTCs as a liquid biopsy biomarker for early detection of lung cancer was not found to be sufficient for researchers accounting for its limited AUC, low sensitivity, but high specificity (36). Kammer et al. (27) integrated CYFRA21-1, clinical variables, and radiomics features of IPNs as a single risk, and reported that the combined model provided improved diagnostic accuracy over the Mayo clinical model, and strategy guided by this model reduced invasive procedures of at least 10% in the intermediate risk nodules, showing the importance and clinical utility of incorporating image and clinical signatures on the basis of blood-based biomarkers. Hence, to enhance the diagnostic performance of CTCs test, we hypothesized that incorporating quantitative image features and clinical variables with the results of CTCs testing could provide a novel insight into classifications of lung nodules, and improve the noninvasive diagnostic accuracy, compensating for low sensitivity of CTCs to form an effective platform for the early detection of lung cancer in high-risk populations and enabling physicians to design a tailored treatment approach.
To verify our hypothesis, the integrated model was derived by the aforementioned markers, and validated on a group of pathologically-confirmed IPSNs mostly at early-stage lung cancer (72.9%) from the department of thoracic surgery in this retrospective evaluation design study. The model achieved a higher AUC (0.83), sensitivity (0.95), and NPV (0.82) than CTCs testing alone in the internal validation set, and similar performance was obtained for the external validation set, indicating the potential value of integration of biological markers and quantitative image features for classifying IPSNs. Diagnosis of IPSNs with size ranging from 5 to 20 mm, or intermediate risk probability is still challenging for clinicians due to the lack of well-specified optimal action strategies. The integrated model showed a robust performance for IPSNs with different nodule sizes. Moreover, the integrated model achieved an AUC of 0.77 and NRI of 0.12 at the threshold of 0.5 or higher, and was shown to correctly classify more than half of the intermediate risk nodules into the malignant group in the external validation set, showing the great clinical benefit for IPSNs’ diagnosis.
PET-CT has been known to be a more accurate and effective tool than CT alone for distinguishing solitary lung nodules, resulting in fewer equivocal findings (37). To further evaluate the predictive efficacy of the integrated model, the comparison with PET-CT on the participants with established PET-CT records in the SPH cohort was performed. As a result, the AUC of the integrated model was found to be higher than that of PET-CT in the internal validation set with no significant statistical difference (P=0.308), demonstrating their comparable performances.
In the clinical application of the model, we should recognize that the models show the best performance in populations similar to those in which they were developed that the reason why the model shows good performance in its internal validation set but less than satisfactory performance in the external validation set. The ratio of benign and malignant nodules was inconsistent between the training set and the external verification set (1:3.6 in the training set and 1:1.3 in the external verification set). We calculate the proportion of malignant and benign nodules that reside at different probability from our integrated model in the external set (Figure 2A). The integrated model classified 73.9% of malignant nodules at a probability threshold of 0.5 or higher. Besides, this model classified 66.6% of benign nodules at a probability threshold of 0.5 or lower. The results indicate that this integrated model exhibits satisfactory discriminative performance even when the ratio of benign to malignant nodules is inconsistent. However, to be cautious, it is better to apply this model to the populations who were similar to those who were developed.
The work presented here has limitations. Firstly, this was a retrospective study, making selection bias inevitable. Secondly, specific approaches were not applied to handle the parameter variations derived from different scanners at multiple institutions, which might have led to some inherent bias. Extraction of radiomics features relied on manual segmentation, which was precise but time-consuming and labor-intensive. A user-friendly, fully automated segmentation for future application is of great necessity. Thirdly, our external validation included 82 IPSNs consisting of 36 benign and 46 malignant diseases, which may fail to reflect typical nodules sets as encountered in all clinical practice settings and was certainly not reflective of the disease prevalence encountered in a screening cohort. The feasibility and practicality of this integrated radio-biological model need to be validated in a large prospective cohort in the future.
Conclusions
In conclusion, we developed an integrated model by incorporating CTCs, clinical variables, and radiomics features for diagnosis IPSNs. It was shown to have robust and superior performance as compared with existing clinical assessment models, and no inferiority to the current PET-CT examination for IPSNs diagnosis, which may help to facilitate the accurate diagnosis of early-stage lung cancer and guide clinical decision-making.
Acknowledgments
The abstract has been presented at IASLC 2022 World Conference on Lung Cancer.
Funding: This study was supported by the Shanghai Municipal Health Commission, China (No. 201940192); Three-year Action Plan to Promote Clinical Skills and Clinical Innovation in Municipal Hospitals of Shanghai Shenkang Hospital Development Center (No. SHDC2020CR3032B); Shanghai Science and Technology Commission (No. 21YF1438200); and the National Natural Science Foundation of China (No. 82102126).
Footnote
Reporting Checklist: The authors have completed the TRIPOD reporting checklist. Available at https://tlcr.amegroups.com/article/view/10.21037/tlcr-23-145/rc
Data Sharing Statement: Available at https://tlcr.amegroups.com/article/view/10.21037/tlcr-23-145/dss
Peer Review File: Available at https://tlcr.amegroups.com/article/view/10.21037/tlcr-23-145/prf
Conflicts of Interest: All authors have completed the ICMJE uniform disclosure form (available at https://tlcr.amegroups.com/article/view/10.21037/tlcr-23-145/coif). The authors have no conflicts of interest to declare.
Ethical Statement: The authors are accountable for all aspects of the work in ensuring that questions related to the accuracy or integrity of any part of the work are appropriately investigated and resolved. This study was approved by the Ethics Committees of Shanghai Pulmonary Hospital (No. L21-022) and The First Hospital of Lanzhou University (No. LDYYLL2023-40). The study conformed to the provisions of the Declaration of Helsinki (as revised in 2013). The requirement for written informed consent was waived for the retrospective cohort.
Open Access Statement: This is an Open Access article distributed in accordance with the Creative Commons Attribution-NonCommercial-NoDerivs 4.0 International License (CC BY-NC-ND 4.0), which permits the non-commercial replication and distribution of the article with the strict proviso that no changes or edits are made and the original work is properly cited (including links to both the formal publication through the relevant DOI and the license). See: https://creativecommons.org/licenses/by-nc-nd/4.0/.
References
- Travis WD, Asamura H, Bankier AA, et al. The IASLC Lung Cancer Staging Project: Proposals for Coding T Categories for Subsolid Nodules and Assessment of Tumor Size in Part-Solid Tumors in the Forthcoming Eighth Edition of the TNM Classification of Lung Cancer. J Thorac Oncol 2016;11:1204-23.
- Hattori A, Hirayama S, Matsunaga T, et al. Distinct Clinicopathologic Characteristics and Prognosis Based on the Presence of Ground Glass Opacity Component in Clinical Stage IA Lung Adenocarcinoma. J Thorac Oncol 2019;14:265-75. [Crossref] [PubMed]
- Zwanenburg A, Vallières M, Abdalah MA, et al. The Image Biomarker Standardization Initiative: Standardized Quantitative Radiomics for High-Throughput Image-based Phenotyping. Radiology 2020;295:328-38. [Crossref] [PubMed]
- Hirsch FR, Scagliotti GV, Mulshine JL, et al. Lung cancer: current therapies and new targeted treatments. Lancet 2017;389:299-311. [Crossref] [PubMed]
- Gould MK, Tang T, Liu IL, et al. Recent Trends in the Identification of Incidental Pulmonary Nodules. Am J Respir Crit Care Med 2015;192:1208-14. [Crossref] [PubMed]
- Siegel RL, Miller KD, Jemal A. Cancer statistics, 2020. CA Cancer J Clin 2020;70:7-30. [Crossref] [PubMed]
- National Lung Screening Trial Research Team. Reduced lung-cancer mortality with low-dose computed tomographic screening. N Engl J Med 2011;365:395-409. [Crossref] [PubMed]
- Lokhandwala T, Bittoni MA, Dann RA, et al. Costs of Diagnostic Assessment for Lung Cancer: A Medicare Claims Analysis. Clin Lung Cancer 2017;18:e27-34. [Crossref] [PubMed]
- Bayarri-Lara CI, de Miguel Pérez D, Cueto Ladrón de Guevara A, et al. Association of circulating tumour cells with early relapse and 18F-fluorodeoxyglucose positron emission tomography uptake in resected non-small-cell lung cancers. Eur J Cardiothorac Surg 2017;52:55-62. [Crossref] [PubMed]
- Liu C, Xiang X, Han S, et al. Blood-based liquid biopsy: Insights into early detection and clinical management of lung cancer. Cancer Lett 2022;524:91-102. [Crossref] [PubMed]
- Alix-Panabières C, Pantel K. Clinical Applications of Circulating Tumor Cells and Circulating Tumor DNA as Liquid Biopsy. Cancer Discov 2016;6:479-91. [Crossref] [PubMed]
- Zhang W, Qin T, Yang Z, et al. Telomerase-positive circulating tumor cells are associated with poor prognosis via a neutrophil-mediated inflammatory immune environment in glioma. BMC Med 2021;19:277. [Crossref] [PubMed]
- Zhang W, Duan X, Zhang Z, et al. Combination of CT and telomerase+ circulating tumor cells improves diagnosis of small pulmonary nodules. JCI Insight 2021;6:e148182. [Crossref] [PubMed]
- Lei Y, Sun N, Zhang G, et al. Combined detection of aneuploid circulating tumor-derived endothelial cells and circulating tumor cells may improve diagnosis of early stage non-small-cell lung cancer. Clin Transl Med 2020;10:e128. [Crossref] [PubMed]
- Yang Z, Bai H, Hu L, et al. Improving the diagnosis of prostate cancer by telomerase-positive circulating tumor cells: A prospective pilot study. EClinicalMedicine 2022;43:101161. [Crossref] [PubMed]
- Marquette CH, Boutros J, Benzaquen J, et al. Circulating tumour cells as a potential biomarker for lung cancer screening: a prospective cohort study. Lancet Respir Med 2020;8:709-16. [Crossref] [PubMed]
- Zhou Q, Geng Q, Wang L, et al. Value of folate receptor-positive circulating tumour cells in the clinical management of indeterminate lung nodules: A non-invasive biomarker for predicting malignancy and tumour invasiveness. EBioMedicine 2019;41:236-43. [Crossref] [PubMed]
- Li Z, Cai J, Zhao Y, et al. Folate receptor-positive circulating tumor cells in the preoperative diagnosis of indeterminate pulmonary nodules. J Clin Lab Anal 2022;36:e24654. [Crossref] [PubMed]
- Ma G, Yang D, Li Y, et al. Combined measurement of circulating tumor cell counts and serum tumor marker levels enhances the screening efficiency for malignant versus benign pulmonary nodules. Thorac Cancer 2022;13:3393-401. [Crossref] [PubMed]
- Ma M, Xu S, Han B, et al. A retrospective diagnostic test study on circulating tumor cells and artificial intelligence imaging in patients with lung adenocarcinoma. Ann Transl Med 2022;10:1339. [Crossref] [PubMed]
- Liu A, Wang Z, Yang Y, et al. Preoperative diagnosis of malignant pulmonary nodules in lung cancer screening with a radiomics nomogram. Cancer Commun (Lond) 2020;40:16-24. [Crossref] [PubMed]
- Massion PP, Antic S, Ather S, et al. Assessing the Accuracy of a Deep Learning Method to Risk Stratify Indeterminate Pulmonary Nodules. Am J Respir Crit Care Med 2020;202:241-9. [Crossref] [PubMed]
- Lambin P, Rios-Velazquez E, Leijenaar R, et al. Radiomics: extracting more information from medical images using advanced feature analysis. Eur J Cancer 2012;48:441-6. [Crossref] [PubMed]
- Peikert T, Bartholmai BJ, Maldonado F. Radiomics-based Management of Indeterminate Lung Nodules? Are We There Yet? Am J Respir Crit Care Med 2020;202:165-7. [Crossref] [PubMed]
- Zhang F, Wu X, Zhu J, et al. 18F-FDG PET/CT and circulating tumor cells in treatment-naive patients with non-small-cell lung cancer. Eur J Nucl Med Mol Imaging 2021;48:3250-9. [Crossref] [PubMed]
- Yin W, Zhu J, Ma B, et al. Overcoming Obstacles in Pathological Diagnosis of Pulmonary Nodules through Circulating Tumor Cell Enrichment. Small 2020;16:e2001695. [Crossref] [PubMed]
- Kammer MN, Lakhani DA, Balar AB, et al. Integrated Biomarkers for the Management of Indeterminate Pulmonary Nodules. Am J Respir Crit Care Med 2021;204:1306-16. [Crossref] [PubMed]
- Reid M, Choi HK, Han X, et al. Development of a Risk Prediction Model to Estimate the Probability of Malignancy in Pulmonary Nodules Being Considered for Biopsy. Chest 2019;156:367-75. [Crossref] [PubMed]
- de Koning HJ, van der Aalst CM, de Jong PA, et al. Reduced Lung-Cancer Mortality with Volume CT Screening in a Randomized Trial. N Engl J Med 2020;382:503-13. [Crossref] [PubMed]
- Carozzi FM, Bisanzi S, Carrozzi L, et al. Multimodal lung cancer screening using the ITALUNG biomarker panel and low dose computed tomography. Results of the ITALUNG biomarker study. Int J Cancer 2017;141:94-101. [Crossref] [PubMed]
- Ost DE, Gould MK. Decision making in patients with pulmonary nodules. Am J Respir Crit Care Med 2012;185:363-72. [Crossref] [PubMed]
- Gould MK, Ananth L, Barnett PG, et al. A clinical model to estimate the pretest probability of lung cancer in patients with solitary pulmonary nodules. Chest 2007;131:383-8. [Crossref] [PubMed]
- Callister ME, Baldwin DR, Akram AR, et al. British Thoracic Society guidelines for the investigation and management of pulmonary nodules. Thorax 2015;70:ii1-ii54. [Crossref] [PubMed]
- Gould MK, Donington J, Lynch WR, et al. Evaluation of individuals with pulmonary nodules: when is it lung cancer? Diagnosis and management of lung cancer, 3rd ed: American College of Chest Physicians evidence-based clinical practice guidelines. Chest 2013;143:e93S-e120S.
- Li Z, Xu K, Tartarone A, et al. Circulating tumor cells can predict the prognosis of patients with non-small cell lung cancer after resection: a retrospective study. Transl Lung Cancer Res 2021;10:995-1006. [Crossref] [PubMed]
- Zheng H, Wu X, Yin J, et al. Clinical applications of liquid biopsies for early lung cancer detection. Am J Cancer Res 2019;9:2567-79. [PubMed]
- Spadafora M, Pace L, Mansi L. Segmental (18)F-FDG-PET/CT in a single pulmonary nodule: a better cost/effectiveness strategy. Eur J Nucl Med Mol Imaging 2017;44:1-4. [Crossref] [PubMed]
(English Language Editor: J. Jones)