Identification of distinct tumor cell patterns with single-cell RNA sequencing integrating primary lung adenocarcinoma and brain metastasis tumor
Highlight box
Key findings
• This study revealed the diversity of LUAD tumor cell transcriptional patterns and identified high-risk subtypes of tumor cells that could potentially impact tumor progression.
What is known and what is new?
• Bulk omics analyses identified the risk subtype of LUAD, and genetic markers have shown potential predictive value for patients’ prognosis.
• Single-cell sequencing was used to further explore the co-occurrence of cellular subtypes in LUAD primary tumors and brain metastases, and their expressing profile showed potential predictive value for patients’ prognosis.
What is the implication, and what should change now?
• Tumor subtypes, determined by bulk sequencing, have cells with different expression patterns, showing nonnegligible impact on tumor progression. However, intratumor heterogeneity remains a major therapeutic challenge. Tumor cell expression profile based on the microenvironment could help predict therapeutic response and display possible target cell populations to develop future therapeutic approaches.
Introduction
Lung cancer, particularly the subtype lung adenocarcinoma, is a major cause of cancer-related deaths globally, largely due to brain metastasis. Around 20–40% of non-small cell lung cancer patients develop brain metastasis. Treatment options for brain metastasis include surgery, chemotherapy, radiotherapy, and immunotherapy, but their effectiveness is uncertain. Although research has attempted to identify genetic alterations in tumor progression, the underlying molecular mechanisms and cellular heterogeneity are not well understood.
Hayes et al. reported reproducible LUAD tumor subtypes, including bronchioid, squamoid, and magnoid types. Bronchioid tumors were correlated with improved survival in early-stage disease, whereas squamoid tumors were associated with better survival in advanced disease (stages III and IV) relative to the bronchioid and magnoid types (1). As the most malignant tumor type, the majority of magnoid tumors exhibit the highest glycolytic and the lowest lipid metabolic levels as well as the highest potency of metastasis (2). As a malignant subtype, further dissecting at the cell level could reveal more info about its malignance.
Previous studies have identified a high-risk subtype of lung adenocarcinoma using genetic markers (1,3-6), while a study combining ribonucleic acid (RNA) and protein profiling identified pathways linked to brain metastasis in these patients (7). Abnormal expression of immune genes has been found to contribute to cancer progression (8-10), with immune factors linked to both brain metastasis and lung adenocarcinoma (11). Previous study mainly based on bulk sample, making role of tumor cells bewildered.
Tumors are comprising of complex multi-type of cells (12-15). Modulation of tumor cell, immune microenvironment, extracellular matrix and angiogenesis critically shapes tumor aggressiveness, progression and drug resistance (10,16,17). The emergence of acquired drug resistance limits the duration of tumor response and defines the main obstacle to a more important effect on survival in genotype-matched cure (18-21). Through these researches, we assume that under environmental and therapeutic pressure, the residual tumor cells can switch into different tumor cells, fleeing their initial reliance on the driver mechanism (12). Thus, there is a critical need for a bold approach to recognizing early molecular drivers of metastasis or progression in originator tumor cells to develop standards to predict or fight their emergence preemptively.
It is now possible to quantify the expression level of each gene in individual cells using single cell RNA sequence (scRNA-seq). In contrast to bulk RNA-seq, innovative sequencing technologies allow high-throughput and high-resolution transcriptomic profiling of single cells facilitating tumor cell dissection (12).
Metastasis-initiating cells (MICs) can seed metastatic colonies in the distal region, hijacking some of the stem cell pathways to increase the cellular endurance to selective pressure induced by the intracranial microenvironment (22,23). MICs are hard to identify, track and capture, which leads to an exceedingly difficult challenge in characterizing the mechanism of brain metastasis. However, single-region sequencing may be sufficient to identify the most known cancer gene mutations in LUAD (15). Thus, scRNA-seq integrating primary and BM tumors permit us to dissect and characterize samples at the cellular level, allowing us to identify distinctive tumor expression patterns potentially responsible for tumor progression.
In this study, we analyzed tumor epithelial cells and associated nonmalignant cells by scRNA-seq, extending the knowledge of previous LUAD gene expression subtypes to the single-cell level. We discovered that the heterogeneity within different LUAD subtypes varies. Bulking RNA-seq indicated dominate cellular subtypes in LUAD tumor, but other subtypes were still present to varying degrees across samples. Using copy number variant (CNV) score, we identified malignant cells following specific expression patterns that were significantly different from those of nonmalignant epithelial cells. Translation of our findings to The Cancer Genome Atlas (TCGA)-LUAD cohort evaluated through gene expression and gene signature revealed potential prognostic value. We present the following article in accordance with the MDAR reporting checklist (available at https://tlcr.amegroups.com/article/view/10.21037/tlcr-23-107/rc).
Methods
Patients and data sets
The present study obtained the samples of a total of 8 treatment-naïve patients with LUAD BM who underwent surgery at Tiantan Hospital (Beijing, China). According to the post-operative histological evaluation, all the 8 tissue slides were confirmed to be LUAD BM by two pathologists with blind individual assays. The study was conducted in accordance with the Declaration of Helsinki (as revised in 2013). The study was approved by Ethics Committee of Beijing Tiantan Hospital, Capital Medical University, Beijing, China (No. KY 2021-052-01). All patients provided written informed consent before inclusion.
We collected 8 freshly resected LUAD BM specimens from 8 treatment-naïve patients and used 10 primary tissue samples and their adjacent normal lung tissues from the Gene Expression Omnibus (GEO) databases GSE131907. as controls (10). To ensure cell viability, tumor specimens were cut along the inside of tumor edge immediately after tumor exposure. While obtain specimens, contamination of specimens due to bleeding was avoided. All samples were evaluated by 2 pathologists to determine pathologic diagnosis and tumor histological subtypes.
Tissue dissociation and single-cell isolation
Tissue specimens of ~0.1–0.5 cm3 were stored on ice in Tissue Storage Solution (Miltenyi Biotec, Bergisch Gladbach, Germany) for transport. After tissue mincing, tissues were disassociated using Acutase (STEMCELL Technologies, Vancouver, BC, Canada) for 15–30 min at room temperature. Cell suspensions were filtered using a 75-µm filter, pelleted by centrifugation at 300 ×g in bovine serum albumin (BSA)-coated low-binding tubes, treated with 1 mL of ammonium-chloride-potassium (ACK) erythrocyte lysis buffer for 1 min, washed with Dulbecco’s Modified Eagle Medium (DMEM), pelleted, and resuspended in phosphate-buffered saline (PBS).
scRNA sequencing
Single-cell library production was conducted with 5,000 single cells applying the Chromium Single Cell 3' Reagent Kit v.3 and the Chromium Controller (10× Genomics, Pleasanton, CA, USA) according to the manufacturer’s protocol. Libraries were sequenced on a HiSeq 4000 Sequencer (Illumina, San Diego, CA, USA) at an average of 54,000 reads per cell.
scRNA-seq data analysis
Unique molecular identifiers (UMIs) were generated using Cellranger 4.0.2 (10× Genomics) with reference to transcriptome GRCh38. Following analyses were conducted using R package “Seurat v3” (24). Single-cell gene expression data of all patients were merged, and 10 samples of primary LUAD and their matched adjacent normal tissues from GSE131907 were merged into this cohort as a reference. The transcriptomes were screened for cells with 500–10,000 genes detected, 1,000–100,000 UMIs counted, and a fraction of mitochondrial reads <30%. After screening out cells of low quality, UMI counts were adjusted using “sctransform” function of “Seurat” with 3,000 variably expressed features for variance-stability (25); meanwhile, the number of UMIs and the percentage of mitochondrial reads were regressed out. The top 20 principal components were used to construct a shared nearest-neighbor (SNN) graph and uniform manifold approximation and projection (UMAP) embedding. Scoring expression level of canonical cell type markers across clusters identified the primary cell types. Principal component analysis (PCA), SNN graph construction, and UMAP embedding were reconducted on the primary cell type subsets. Epithelial and immune polluted clusters were confirmed by evaluating EPCAM or PTPRC and removed before further analyses. Epithelial cell clusters presented in tumor tissue samples were annotated as tumor cells.
Differential and enrichment analysis
Applying the FindAllMarkers function of “Seurat”, we identified marker genes for each individual by comparing the cell cluster versus the other respective ones with the following parameters: fraction of expressing cells within the cluster ≥0.25, the difference between the fraction of expressing cells within and beyond the cluster ≥0.25, and log fold change between cells within and beyond the cluster ≥0.25. The pseudo-bulk for each patient was converted with the aggregated cells within a biological replicate before statistical analysis was performed (26). Tumor pseudo-bulk and single-cell subtypes were defined by comparing the gene list across samples using centroid and gene set enrichment analysis (1). We conducted enrichment analyses, using Gene Ontology (GO) and Kyoto Encyclopedia of Genes and Genomes (KEGG) tools on the differentially expressed genes with the R package “clusterProfiler” (27).
Malignant cell annotation
Cells previously annotated as the epithelial subset were reclustered using the methods described above. Malignant tumor cells were identified using InferCNV (https://github.com/broadinstitute/inferCNV) with default parameters. InferCNV can be used to discover cells with numerous CNVs. It was processed by classifying the expressed feature according to the chromosomal position and applying a shifting average, a moving window of 100 genes within an individual chromosome, to the relative expression level. All tumor epithelial cells were used as input, and epithelial cells from normal lung tissue were used as a control reference. We classified each cell according to the extent of CNV signal and cluster epithelial cells from the tumors into benign, intermedium, and malignant cells (28).
Analysis of TCGA-LUAD cohort
We downloaded, and log2-transformed Fragment per kilobase exon (FPKM) normalized gene expression data of 533 LUAD patients in the TCGA cohort using the R package “TCGAbiolinks” (29). Survival info of 524 case in TCGA LUAD cohort were accessible. We use centroids and gene set enrichment analysis to evaluate the gene list and define the sample subtypes (1).
Statistical analysis
We performed all statistical analysis using the R programming language (version 4.2.2; https://www.r-project.org/). T-test and Mann-Whitney test (2-sided, unequal variances) were used to evaluate the significance between sample groups. Kaplan-Meier survival curve was applied to determine the clinical outcome across groups, and log-rank tests were performed to assess the statistical significance. All statistical tests were 2-sided. We constructed univariate and multivariate Cox proportional hazard models to evaluate the prognostic value of the risk pattern centroid determined according to its signature. Receiver operating characteristic (ROC) curves and the area under the curve (AUC) at the 1-, 3-, and 5-year follow-ups were computed to examine the predictability using the “timeROC” package. P values <0.05 were considered significant.
Results
Characterization of tumor cell heterogeneity
We cataloged 258,566 cells from BM tumor, lung tumor, and lung normal tissue into 3 distinct cell lineages annotated using canonical marker gene expression (Figure 1A-1C), thus identifying epithelial (SCGB3A2, KRT19, KRT18, EPCAM), stromal (ACTA2, VWF, DCN, KIT, IGFBP7), and immune cells (LYZ, NKG7, CD68, PTPRC) as the common cell types (Figure 1D-1G).

We extracted epithelial cells from all annotated cells and then calculated the pseudo-bulk expression of every detected gene respectively by using the AverageExpression function from “Seurat” after scaling the data. The pseudo-bulk and single cells were all assigned to different LUAD subtypes by comparing the gene list defined before across samples using centroid and gene enrichment analysis (1). Among the 8 samples from BM, 5 pseudo-bulk samples were assigned to the magnoid subtype and 3 pseudo-bulk samples were assigned to the squamoid subtype. Samples from the primary LUAD were all assigned to bronchioid or squamoid subtype (Figure 2A). As single cells were also classified into different LUAD subtypes (Figure 2B, Figure S1A), we explored the composition of different LUAD subtypes. Pseudo-bulk samples assigned to the bronchioid or squamoid subtype had a large proportion of identical subtype cells (Figure 2C,2D). However, pseudo-bulk assigned to the magnoid subtype also had the largest proportion of magnoid-like subtype cells, but the magnoid subtype also had an abundance of the other 2 subtypes (Figure 2C,2D). Thus, we inferred that the magnoid subtype of LUAD had the stronger heterogenicity compared to the other LUAD subtypes. The cell subtype distribution of brain metastatic tumor cells was similar to that of the magnoid subtype, whereas the cell subtype distribution of the primary LUAD was similar to that of the bronchioid subtype (Figure 2E).

After cells were grouped according to their origin, samples originating from BM had a large proportion of bronchioid-like and squamoid-like cells. However, primary LUAD had the largest proportion of bronchioid-like cells. In order to verify this conclusion, we introduced all samples from GSE131907 and found that samples acquired from the metastasis site commonly had a larger proportion of magnoid-like or squamoid-like cells and a lower proportion of bronchioid-like cells (Figure S1B).
According to a previous study (1), the magnoid and squamoid subtype are more closely correlated to each other than either is to the bronchioid subtype and are more strongly associated with an overall higher tumor grade. In this study, we found cells with features of the magnoid and squamoid subtypes were more concentrated in samples from the metastasis site (Figure 2E), which is consistent with previous results (1).
We also explored the prognosis of these 3 subtypes in TCGA-LUAD samples. Samples delineated as the squamoid and magnoid subtype had a significantly worse prognosis than did those delineated as the bronchioid subtype, and the prognosis was similar for those patients characterized by the squamoid or magnoid subtypes (Figure 2F).
The origin of malignant tumor cells
Cells with high a CNV level are considered to be malignant cells (30). In the present study, we separated the definitive malignant tumor cells from a nonmalignant population by using genetic aberrations through inferring CNVs from the gene expression data (31,32). We included the epithelial cells from normal lung tissues as a normal reference to evaluate the CNVs of the 3 tumor cell subtypes. Chromosomal deletion (blue) and amplification (red) were mapped to each chromosomal position (columns) (Figure 3A).
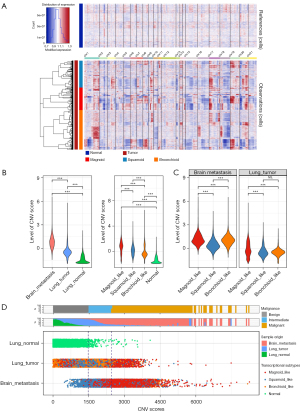
We inferred that CNV patterns were associated with stronger perturbations in bronchioid, squamoid, and magnoid cells than in normal epithelial cells. Specifically, the tumor cells showed an incremental increase of chromosomal gain/loss occurrences from the normal to metastatic types (Figure 3B), while the epithelial cells from normal tissues had little or no CNVs (Figure 3A,3B). The tumor cells from BM displayed a significantly higher number of CNVs than did those of the primary tumor. Magnoid-like cells had the highest CNV level compared to the other subtypes, both in BM and primary lung tumor (Figure 3B,3C).
We separated tumor cells according to CNV levels and calculated the meannor and deviationnor of CNV scores of cells from normal tissue. Cells from tumor with CNV score less than sum1-fold (sum1-fold = meannor + deviationnor) were placed into a benign group, tumor cells with a CNV score higher than sum1-fold and less than sum2-fold (sum2-fold = meannor + 2 × deviationnor) were placed into intermedium group, and tumor cells with CNV scores higher than sum3-fold (sum3-fold = meannor + 3 × deviationnor) were placed into a malignant group. Both in primary lung LUAD and BM, magnoid-like cells were mainly distributed in the malignant group (Figure 3D). Therefore, the results demonstrated strong genetic evidence supporting cells of the magnoid type as being critically involved in tumor malignant behavior.
Transcriptional trajectory of benign and malignant cells
Using the definitive malignant, intermedium, and benign cells, we built a transcriptional trajectory (33), to find essential gene expression programs ruling the tumor progression. Indeed, transcriptional states in the trajectory revealed differentiated courses, as well as malignancy shifts between benign and malignant tumors (Figure 4A). Malignant tumor cells were mainly found in separate trajectory branches, indicating their distinctly differentiated status (Figure 4A,4B). A portion of the tumor cells from primary and metastatic tumors were located between benign and malignant cells, representing an intermediate differentiation stage. Finally, malignant cells formed 2 distinct branches, presenting 2 distinct transcriptive stages. To clarify the transcriptional signatures defining cellular states in the trajectory, we identified differentially expressed genes that exhibited dynamic expression over pseudo-time (q-value <0.05) and classified them into 2 clusters (clusters 1 and 2), indicating 2 endpoint stages of tumor progression.
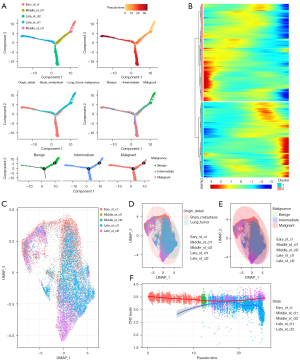
To gain more information about the 2 terminal stages, we applied GO and KEGG analysis to explore the underlying function of cells located in the terminal branches and in each stage. The top enriched signatures in cells of the late-stage branches included cell cycle and cell division (Figure S2A), which showed that the cells increased in proliferative ability with an increase from a benign to malignant state.
The pseudo-temporal expression dynamics of the specific stages of representative genes also showed a progression of early-stage cells to intermediate- and late-stage branches (Figure S2B).
The most enriched signatures in the early stage included MAPK signaling pathway, P53 signal pathway, and TNF signal pathway; The most enriched signatures in the late stages included cell cycle and P53 signal pathway. Cell adhesion molecules were enriched in the intermediate stage. Antigen processing and presentation, which is required for the immunosurveillance of cancer cells (34-37) and the modulation of antigen expression and human leukocyte antigen type I (HLA-I) surface levels, were enriched in all 3 stages (Figure S2C). Alterations in the antigen processing and presentation could be protective for tumor cells via immune evasion (34). The gene signature of the late stages was highly enriched in antigen processing and presentation (Figure S2C), which indicated that cells from the late stages may adopt antigen processing and presentation modulation to escape immune recognition, and this may also be a characteristic of malignant metastatic cells. In subsequent study, tumor cells were separated into early-stage, middle stage clusters (middle_st_cl1 and middle_st_cl2), and late-stage (late_st_cl1 and late_st_cl2) clusters according to the transcriptional trajectory states.
The characteristics and gene markers of malignant cells
We selected the top 2,000 variable features of stage-annotated cells and ran unsupervised UMAP to determine the distribution of these cells (Figure 4C). We found that late_st_cl2 could be separated into 2 distinct clusters. One of which was within the 95% CI range of primary lung tumor cells and intermediate-stage cells. The others, beyond the scope of nonmalignant cells, potentially showed significantly different transcriptional profiles (Figure 4D,4E). To clarify the malignancy, CNV scores were scrutinized: cells from BM had higher CNV levels than did the cells from primary lung tumor, whose CNV levels increased in the early stages and decreased in the late stages (Figure 4F). Additionally, in the late-stage cells, late_st_cl2 cells had high CNV scores, while the scores of late_st_cl1 cells were significantly decreased. Therefore, late_st_cl2 cells may contain extremely malignant cell types which promote tumor progression.
We used the findcluster function in the “Seurat” package with 0.5 resolution to group cells into 9 clusters. Although cluster 4, cluster 5, and cluster 6 cells had a large proportion of late_st_cl2 cells, cluster 4 and cluster 6 also had late_st_cl2 cells with a distinct transcription profile of malignant characteristics (Figure 5A). Despite cluster 5 cells containing the largest number of late_st_cl2 cells (Figure 5B,5C), many of these cells still had a transcriptome profile similar to that of cells in the primary tumor but originated from BM (Figure 5D). Cells from the primary tumor of cluster 5 only had a small proportion of cluster 5, most of which were mainly from BM, indicating a restricted transcriptional state could emerge in in brain tumor microenvironment.

We found the differentially expressed genes of each cluster (Figure 5E). Cluster 4 had increased expression of PCLAF, which was significantly associated with poor prognosis in TCGA-LUAD cohort (Figure 5F). Kim et al. reported that PCLAF could drive cell quiescence exit to promote lung tumorigenesis by remodeling the DREAM complex (38). TYMS and ATAD2 were highly expressed in cluster 4 and were associated with worse prognosis in TCGA-LUAD cohort (Figure 5F and Figure S3A), suggesting cluster 4 cells play a vital role in tumorigenesis, proliferation, and migration (39-41).
Cluster 6 had a high expression of CENPF and thus could also potentially be a risk cluster promoting tumor cell malignancy (Figure 5G) (42,43). TOPA2, UBE2S, CCNB1, and HMGB2 were all found to be differentially highly expressed in cluster 6, providing further evidence for its role in the deterioration of patient prognosis (Figure 5G) (44-47). All the biomarkers in cluster 6 were validated in TCGA cohort and were confirmed to have a negative impact on patients’ survival (Figure S3B). In addition, the markers in cluster 4 and cluster 6 had a significantly higher expression in metastasis samples in TCGA cohort (Figure S3C,S3D).
Therefore, cluster 4 and cluster 6 cells could potentially indicate an emerging tumor cell type both in the primary tumor and BM tumor that can lead to tumor proliferation, expansion, invasion, and metastasis. Their biomarkers can potentially be targeted to suppress tumor growth or transformation to malignancy.
Evaluation of patient survival based on risky cluster features
We identified the clusters of risky of tumor cells. Both clusters 4 and 6 expressed malignant biomarkers, and in order to clarify their impact on clinical outcome, we integrated the top 10, 30, and 50 differentially expressed genes to separate bulk samples from TCGA cohort into a feature-expressed group and negative group. Regardless of the number of top differentially expressed genes (DEGs) we used, samples with characteristics of cluster 4 and cluster 6 had a significantly worse clinical prognosis (Figure 6A,6B). In addition, for prognostic prediction, the AUC reached the maximum in the first year, indicating the prognostic model constructed from these 2 cells signature had the most accurate prediction ability in the first year (Figure 6C,6D).
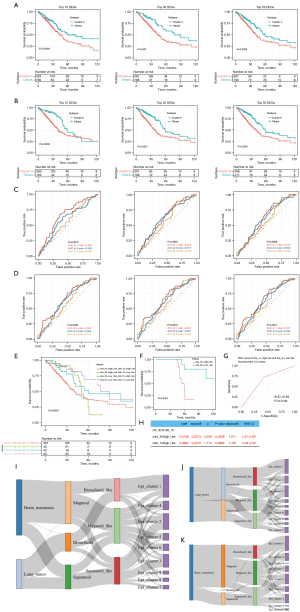
As the 2 cell types had obvious differences in marker genes and expression profiles and because there might also have been a coexpression of the 2 characteristics in the same sample, we divided all samples into 4 groups based on whether the 2 expression patterns were followed (cls4_High_risk cls6_High_risk, cls4_High_risk cls6_Low_risk, cls4_Low_risk cls6_High_risk and cls4_Low_risk cls6_High_risk) to gain further insight into the prognostic differences between the 2 types. We additionally compared the models built by the top 10, top 30, and top 50 differentially expressed genes, respectively.
Among the 3 models incorporating both the cls4 and cls6 signatures (cls4_10 and cls6_10, cls4_30 and cls6_30, cls4_50 and cls6_50), the high expression of either cls4 or cls6 features was associated with worse prognosis of patients as compared to the dual low-risk patients (Figure S4A-S4C). Patients with low risk for both cls4 and cls6 had the best prognosis. Patients with high expression of both cls4 and cls6 features had a significantly worse prognosis regardless of the number of signature genes (Figure S4A-S4C), and the cls6 signature (cls6_10, cls6_30, cls6_50) was an independent risk factor of LUAD (Figure S4D). In general, the cls6 signature genes had prognostic value in predicting patients’ death within 1 years (Figure 6D).
Interestingly, we observed that the cls6 signature was an independent risk factor in patients with LUAD in all models (Figure S4D), whereas the cls4 signature was merely found to be independently associated with prognosis in models that included 10 differentially expressed genes. Moreover, in the cls4_High_risk group, many deaths occurred between 30 and 60 months. Considering that cls4 and cls6 cells belong to the same large group of cells, they have the most similar expression profiles to each other compared to other clusters. Incorporating more cls6 features may underestimate the danger of cls4 cells. Therefore, we included more cls4 signatures (the top 30 signature genes included) and further compressed the cls6 signature genes (top 10 signature genes included) to build the model. Patients with cls4_30High_risk or cls6_10Low_risk had the worst prognosis within 3 years (Figure 6E and Figure S4E). After excluding patients with cls6_10High_risk and grouping patients according to cls4_30 risk, the prognosis of patients in the cls4_30High_risk group was significantly worse (log-rank P=0.0057; Figure 6F) than cls4_30Low_risk group, and the model had prognostic value in patients with a survival period of more than 3.5 years (AUC =0.64; P=0.015; Figure 6G). Both cls4_30High_risk and cls6_10High_risk were independent risk factors for patients with LUAD in multivariate Cox regression (Figure 6H). In general, although cluster 4 and cluster 6 tumor cells had a similar expression profile, they play different roles in tumor progression, with patients with a high expression of cluster 6 features having a poorer prognosis in early stages and patients with a high expression of cluster 4 features having a poorer prognosis in the late stage.
By comparing the sample subtype results from the bulk and single-cell sequencing, we determined that the cells defined by bulk tumor sequencing are basically the same as those defined by single-cell sequencing, suggesting that the bulk subtypes are dominated by cells of the same subtype (Figure 1C,1D). However, other subtypes of cells were also fairly common (Figure 1E and Figure S1B). We determined the risk of cls4 and cls6, and We clarified which transcriptional subtype these high-risk cell clusters originated from. Both cls4 and cls6 cells are mainly derived from cells of the magnoid-like subtype, and although they are mainly derived from the magnoid subtype, bronchioid-like and squamoid-like cells are also involved in the magnoid-like subtype but in lower proportions (Figure 6I). This phenomenon was also present in the primary lung tumor and BM (Figure 6J,6K). We thus surmised that compared with the squamoid and bronchioid subtype, the magnoid subtype is more likely to develop into cluster 4 and cluster 6, which may significantly worsen the patient’s outcome.
Discussion
In this research, we evaluated single-cell data from BM, primary tumor, and normal lung tissue. With high-resolution information provided by the single-cell approach, we assigned the samples and cells to bronchioid, squamoid, and magnoid subtypes by comparing the gene list from previous studies across cohorts utilizing gene centroids and gene set enrichment analysis. In a previous study, patients with the bronchioid subtype usually had a better prognosis, and all had a good prognosis in the early stages of the disease. In contrast, patients with either magnoid or squamoid subtypes had a shorter survival, and in addition, the squamoid subtype was associated with a better prognosis in the advanced stages of the disease (1). In this study, we found cells of the same subtype predominately concentrated in the tumor; however, other subtypes of cells were also present. Interestingly, the magnoid subtype tumors, although dominated by cells of the magnoid-like cell subtype, also had a large proportion of other cell types and was the least pure of the 3 subtypes. The squamoid subtype had the second highest percentage of magnoid subtype cells after the cells of its major subtype. We therefore inferred that there still exists significant tumor cell heterogeneity in the subtypes defined according to bulk tumor.
We observed a large presence of magnoid subtype cells in both BM and other metastases. Meanwhile, in the primary tumors, we only found predominantly bronchioid subtype cells with a small number of other subtypes, and the magnoid subtype appeared to be inextricably linked to the malignant behavior of LUAD tumors, which is consistent with the findings of a previous study (1). Cells with high CNV level are considered to be malignant cells (30). We calculated the CNV of all cells by InferCNV but did not recognize a subtype-specific CNV pattern. However, cells from BMs had higher CNV levels compared to those from the primary tumor, and cells with high CNV levels were mainly derived from magnoid subtype cells. Regardless of whether the magnoid subtype cells originated from the primary or metastatic foci, the magnoid subtype had significantly higher CNV levels, and thus we further clarified the risk of the magnoid subtype.
Based on CNV levels, all tumor cells were classified into benign, intermediate, and malignant tumor cells. In unsupervised cell pseudo-time trajectory analysis, all tumor cells were divided into 3 branches. The transcriptional states in the trajectory showed progression-associated shifts in cancer cells. During the progression of cells from benign to malignant, we find that malignant tumor cells showed a prominent pattern of division, proliferation, and drug resistance. By ordering cells along pseudo-time, cell transcriptional states were assigned into 3 major stages (early, intermediate, and late stages). We found that the processing and presentation of antigens was persistent in all 3 stages. The immunosurveillance of cancer requires the presentation of peptide antigens on major histocompatibility complex class I molecules (37). Thus, we could deduce that after tumor formation, presentation of peptide antigens needs to be maintained the tumor’s presence. Apoptosis signals were only identified at the early and intermediate stages but missing in the late stages. We also found that 1 branch of late stage maintained a high CNV level. This implies that a highly malignant, rapidly proliferating, immune-escape capable, and apoptosis-resistant cell subtype may be embedded in the late-stage cells.
Wang et al. (23) mentioned S100A9 could potentially be a marker gene for brain metastasis-associated epithelial cells, which may be the origin of brain metastasis. However, S100A9 is commonly regarded as a marker of inflammatory cells such as neutrophils or monocytes. In this research, we confirmed S100A9 was significantly overexpressed in cluster 3 tumor epithelial cells (Figure 5E). Cluster 3, mainly composed of cells from the late stage and with a similar expression profile of benign and intermedium cells, was abundant in both brain metastasis and primary LUAD (Figure 4E,5D), indicating its potential role in early phase of brain metastasis process. We further summarized the marker genes (overexpress S100A9, S100A8, FTH1, SCGB3A2, LCN2, RPS20, RPL31 and SAA2) of this expression pattern (Figure 5E). If cells that follow cluster 3 expression pattern can be sorted, and further explore the effect of its overexpressed genes on these cells and the effect of various drugs on this subset of cells, these results will be of great value for the early identification and treatment of BM patients in the future.
We also identified 2 high-risk subtypes from late-stage cells, the expression profiles of which had a significant association with the prognosis of patients from TCGA cohort. The biomarkers of these 2 clusters were also significantly overexpressed in metastasis samples. Specifically, cluster 4 cells overexpressing PCLAF, TYMS, and ATAD2 indicated a pattern driving tumorigenesis, proliferation, and migration. Cluster 6 cells overexpressing CENPF, UBE2C, PTTG1, TOP2A, UBE2S, CCNB1, HMGB2, TPX2, and HMMR indicated a tumor pattern leading to early-stage (less than 1 year) deterioration of patients’ prognosis.
The expression profile of cluster 6 was an independent risk factor for patients’ overall death; however, only when the top 10 differential genes were selected, could we observe an independent prognostic value of the cluster 4 profile. Since cluster 4 and cluster 6 had a similar expression profile, we considered the risk of cluster 4 profile to potentially be underestimated while the cluster 6 profile might have included too many genes that were expressed in both clusters. Thus, we selected the top 10 DEGs from cluster 4 and the top 30 from cluster 6 to group patients. Finally, we found that patients only expressing the cluster 4 profile achieved better prognosis within 3.5 years as compared to patients only expressing the cluster 6 profile. Beyond 3.5 years, patients’ expressing the cluster 4 profile had worse prognosis than did the patients assigned to cluster 4 low risk group. Cluster 4 and cluster 6 had the most similar expression pattern than did any of the other 2 subtypes; however, whether these 2 cell types undergo type conversion as the tumor develops needs to be further addressed. They may originate from the same tumor lineage and play an essential role in the development and metastasis of tumors.
In the study, we identified cells’ malignancy and found benign cells underwent pattern switching from benign into two different cell statuses mainly composed of malignant and intermedium cells. This plasticity might uncover the mechanisms driving LUAD brain metastasis or tumor progression. In the early stage, KEGG pathway enrichment revealed pathways that may promote tumor cell metastasis (Figure S2C). We validate our findings from scRNA-seq by assigning TCGA LUAD patients to different risk groups according to a set of 10, 30, and 50 genes recapitulating expression patterns of predictive tumor cell subtypes derived from clusters with distinctive expression features from non-malignant cells. Our findings indicate a prognostic relevance of tumor cell patterns which could benefit malignant cell recognition and therapeutic decision-making. In addition, our result extending our knowledge of previous identified LUAD subtype, Cells following the malignant pattern were mainly derived from the magnoid subtype (Figure 6I), but the magnoid-like cells could originate from the other subtypes. All LUAD subtypes have the capacity to assume dangerous expression patterns.
Cancer cells and cells in the tumor microenvironment collectively determine disease progression and response or avoidance to treatment (10). scRNA-seq including glioma and LUAD brain metastasis mainly focus on the non-tumor cells reported LUAD BM reprogramed cells into immune suppressed state, and immunotherapy could potentially an effective treatment (48). Study on tumor cell at cell level may provide target of tumor cells, combined with immunotherapy which may improve treatment efficacy. Previous study dissecting tumor cells from patient-derived xenografts (PDX) reported key genes responsible for poor outcome (49). Due to the heterogeneity of xenografts, there is large heterogeneity among PDX cells, resulting in unstable quality of single-cell data. This study mainly focused on the tumor malignant cells from patient and its expression pattern, which may provide reliable potential target for tumor cells.
We have to admit that this research still has some shortcomings. First, all expression features of risky tumor clusters need further screening and validation in multi-omics data. Unequivocal discrimination of the key feature of risky expression pattern is challenging. If a key feature highly related to brain metastasis can be identified and cells following the risk pattern can be sorted out, the effect of drug on the subcluster of tumor cells can be further characterized, the result can be a huge impact on the early diagnosis and treatment of brain metastasis. Second, the samples included in this study were all treatment-naïve brain metastasis, which may not accurately represent the real-world situation. To validate the risky expression signature and enhance its credibility, we included LUAD patients from TCGA in our study.
Conclusions
Our results illustrate a complex tumor cell composition picture, but our scRNA-seq approach also refined a gene expression pattern that may provide insight into the relevant cell groups, and by bypassing other indistinct features, might aid in advancing the study of tumor cells.
Acknowledgments
Funding: This work was financially supported by the National Natural Science Foundation of China (grant No. 82003075, to Xiudong Guan), the Beijing Hospitals Authority Youth Program (grant No. QML20210502, to Xiudong Guan) and the the National Natural Science Foundation of China (grant No. 81802483, to Chuanbao Zhang).
Footnote
Reporting Checklist: The authors have completed the MDAR reporting checklist. Available at https://tlcr.amegroups.com/article/view/10.21037/tlcr-23-107/rc
Data Sharing Statement: Available at https://tlcr.amegroups.com/article/view/10.21037/tlcr-23-107/dss
Peer Review File: Available at https://tlcr.amegroups.com/article/view/10.21037/tlcr-23-107/prf
Conflicts of Interest: All authors have completed the ICMJE uniform disclosure form (available at https://tlcr.amegroups.com/article/view/10.21037/tlcr-23-107/coif). XG reports that this work was financially supported by the National Natural Science Foundation of China (grant No. 82003075) and the Beijing Hospitals Authority Youth Program (grant No. QML20210502). CZ reports that this work was financially supported by the National Natural Science Founddation of China (grant No. 81802483). The other authors have no conflicts of interest to declare.
Ethical Statement: The authors are accountable for all aspects of the work in ensuring that questions related to the accuracy or integrity of any part of the work are appropriately investigated and resolved. The study was conducted in accordance with the Declaration of Helsinki (as revised in 2013). The study was approved by Ethics Committee of Beijing Tiantan Hospital, Capital Medical University, Beijing, China (No. KY 2021-052-01). All patients provided written informed consent before inclusion.
Open Access Statement: This is an Open Access article distributed in accordance with the Creative Commons Attribution-NonCommercial-NoDerivs 4.0 International License (CC BY-NC-ND 4.0), which permits the non-commercial replication and distribution of the article with the strict proviso that no changes or edits are made and the original work is properly cited (including links to both the formal publication through the relevant DOI and the license). See: https://creativecommons.org/licenses/by-nc-nd/4.0/.
References
- Hayes DN, Monti S, Parmigiani G, et al. Gene expression profiling reveals reproducible human lung adenocarcinoma subtypes in multiple independent patient cohorts. J Clin Oncol 2006;24:5079-90. [Crossref] [PubMed]
- Li X, Tang L, Deng J, et al. Identifying metabolic reprogramming phenotypes with glycolysis-lipid metabolism discoordination and intercellular communication for lung adenocarcinoma metastasis. Commun Biol 2022;5:198. [Crossref] [PubMed]
- Peinado-Serrano J, Quintanal-Villalonga Á, Muñoz-Galvan S, et al. A Six-Gene Prognostic and Predictive Radiotherapy-Based Signature for Early and Locally Advanced Stages in Non-Small-Cell Lung Cancer. Cancers (Basel) 2022;14:2054. [Crossref] [PubMed]
- Cheung WK, Zhao M, Liu Z, et al. Control of alveolar differentiation by the lineage transcription factors GATA6 and HOPX inhibits lung adenocarcinoma metastasis. Cancer Cell 2013;23:725-38. [Crossref] [PubMed]
- Tang H, Wang S, Xiao G, et al. Comprehensive evaluation of published gene expression prognostic signatures for biomarker-based lung cancer clinical studies. Ann Oncol 2017;28:733-40. [Crossref] [PubMed]
- Bueno R, Richards WG, Harpole DH, et al. Multi-Institutional Prospective Validation of Prognostic mRNA Signatures in Early Stage Squamous Lung Cancer (Alliance). J Thorac Oncol 2020;15:1748-57. [Crossref] [PubMed]
- Zhang L, Liang J, Han Z, et al. Micro-ribonucleic acids (miRNAs) and a proteomic profile in lung adenocarcinoma cases with brain metastasis. Ann Transl Med 2022;10:1389. [Crossref] [PubMed]
- Blumenfeld-Kan S, Staun-Ram E, Miller A. Fingolimod reduces CXCR4-mediated B cell migration and induces regulatory B cells-mediated anti-inflammatory immune repertoire. Mult Scler Relat Disord 2019;34:29-37. [Crossref] [PubMed]
- Wang Q, Ding H, He Y, et al. NLRC5 mediates cell proliferation, migration, and invasion by regulating the Wnt/β-catenin signalling pathway in clear cell renal cell carcinoma. Cancer Lett 2019;444:9-19. [Crossref] [PubMed]
- Kim N, Kim HK, Lee K, et al. Single-cell RNA sequencing demonstrates the molecular and cellular reprogramming of metastatic lung adenocarcinoma. Nat Commun 2020;11:2285. [Crossref] [PubMed]
- Chen C, Guo Q, Tang Y, et al. Screening and evaluation of the role of immune genes of brain metastasis in lung adenocarcinoma progression based on the TCGA and GEO databases. J Thorac Dis 2021;13:5016-34. [Crossref] [PubMed]
- Lim ZF, Ma PC. Emerging insights of tumor heterogeneity and drug resistance mechanisms in lung cancer targeted therapy. J Hematol Oncol 2019;12:134. [Crossref] [PubMed]
- Wu F, Fan J, He Y, et al. Single-cell profiling of tumor heterogeneity and the microenvironment in advanced non-small cell lung cancer. Nat Commun 2021;12:2540. [Crossref] [PubMed]
- Bischoff P, Trinks A, Obermayer B, et al. Single-cell RNA sequencing reveals distinct tumor microenvironmental patterns in lung adenocarcinoma. Oncogene 2021;40:6748-58. [Crossref] [PubMed]
- Zhang J, Fujimoto J, Zhang J, et al. Intratumor heterogeneity in localized lung adenocarcinomas delineated by multiregion sequencing. Science 2014;346:256-9. [Crossref] [PubMed]
- Altorki NK, Markowitz GJ, Gao D, et al. The lung microenvironment: an important regulator of tumour growth and metastasis. Nat Rev Cancer 2019;19:9-31. [Crossref] [PubMed]
- Li Q, Jiang Y, Song N, et al. An Immune-Related Genetic Feature Depicted the Heterogeneous Nature of Lung Adenocarcinoma and Squamous Cell Carcinoma and Their Distinctive Predicted Drug Responses. Oxid Med Cell Longev 2022;2022:8447083. [Crossref] [PubMed]
- Shien K, Papadimitrakopoulou VA, Ruder D, et al. JAK1/STAT3 Activation through a Proinflammatory Cytokine Pathway Leads to Resistance to Molecularly Targeted Therapy in Non-Small Cell Lung Cancer. Mol Cancer Ther 2017;16:2234-45. [Crossref] [PubMed]
- Yu Z, Boggon TJ, Kobayashi S, et al. Resistance to an irreversible epidermal growth factor receptor (EGFR) inhibitor in EGFR-mutant lung cancer reveals novel treatment strategies. Cancer Res 2007;67:10417-27. [Crossref] [PubMed]
- Yu HA, Arcila ME, Rekhtman N, et al. Analysis of tumor specimens at the time of acquired resistance to EGFR-TKI therapy in 155 patients with EGFR-mutant lung cancers. Clin Cancer Res 2013;19:2240-7. [Crossref] [PubMed]
- Zhang Z, Lee JC, Lin L, et al. Activation of the AXL kinase causes resistance to EGFR-targeted therapy in lung cancer. Nat Genet 2012;44:852-60. [Crossref] [PubMed]
- Celià-Terrassa T, Kang Y. Distinctive properties of metastasis-initiating cells. Genes Dev 2016;30:892-908. [Crossref] [PubMed]
- Wang Z, Wang Y, Chang M, et al. Single-cell transcriptomic analyses provide insights into the cellular origins and drivers of brain metastasis from lung adenocarcinoma. Neuro Oncol 2023;noad017. [Crossref] [PubMed]
- Stuart T, Butler A, Hoffman P, et al. Comprehensive Integration of Single-Cell Data. Cell 2019;177:1888-1902.e21. [Crossref] [PubMed]
- Hafemeister C, Satija R. Normalization and variance stabilization of single-cell RNA-seq data using regularized negative binomial regression. Genome Biol 2019;20:296. [Crossref] [PubMed]
- Squair JW, Gautier M, Kathe C, et al. Confronting false discoveries in single-cell differential expression. Nat Commun 2021;12:5692. [Crossref] [PubMed]
- Yu G, Wang LG, Han Y, et al. clusterProfiler: an R package for comparing biological themes among gene clusters. OMICS 2012;16:284-7. [Crossref] [PubMed]
- Maynard A, McCoach CE, Rotow JK, et al. Therapy-Induced Evolution of Human Lung Cancer Revealed by Single-Cell RNA Sequencing. Cell 2020;182:1232-1251.e22. [Crossref] [PubMed]
- Colaprico A, Silva TC, Olsen C, et al. TCGAbiolinks: an R/Bioconductor package for integrative analysis of TCGA data. Nucleic Acids Res 2016;44:e71. [Crossref] [PubMed]
- Xu Q, Chen S, Hu Y, et al. Single-cell RNA transcriptome reveals the intra-tumoral heterogeneity and regulators underlying tumor progression in metastatic pancreatic ductal adenocarcinoma. Cell Death Discov 2021;7:331. [Crossref] [PubMed]
- Puram SV, Tirosh I, Parikh AS, et al. Single-Cell Transcriptomic Analysis of Primary and Metastatic Tumor Ecosystems in Head and Neck Cancer. Cell 2017;171:1611-1624.e24. [Crossref] [PubMed]
- Patel AP, Tirosh I, Trombetta JJ, et al. Single-cell RNA-seq highlights intratumoral heterogeneity in primary glioblastoma. Science 2014;344:1396-401. [Crossref] [PubMed]
- Qiu X, Mao Q, Tang Y, et al. Reversed graph embedding resolves complex single-cell trajectories. Nat Methods 2017;14:979-82. [Crossref] [PubMed]
- Jhunjhunwala S, Hammer C, Delamarre L. Antigen presentation in cancer: insights into tumour immunogenicity and immune evasion. Nat Rev Cancer 2021;21:298-312. [Crossref] [PubMed]
- Donia M, Harbst K, van Buuren M, et al. Acquired Immune Resistance Follows Complete Tumor Regression without Loss of Target Antigens or IFNγ Signaling. Cancer Res 2017;77:4562-6. [Crossref] [PubMed]
- Schumacher TN, Schreiber RD. Neoantigens in cancer immunotherapy. Science 2015;348:69-74. [Crossref] [PubMed]
- Jaeger AM, Stopfer LE, Ahn R, et al. Deciphering the immunopeptidome in vivo reveals new tumour antigens. Nature 2022;607:149-55. [Crossref] [PubMed]
- Kim MJ, Cervantes C, Jung YS, et al. PAF remodels the DREAM complex to bypass cell quiescence and promote lung tumorigenesis. Mol Cell 2021;81:1698-1714.e6. [Crossref] [PubMed]
- Wan S, Liu Z, Chen Y, et al. MicroRNA-140-3p represses the proliferation, migration, invasion and angiogenesis of lung adenocarcinoma cells via targeting TYMS (thymidylate synthetase). Bioengineered 2021;12:11959-77. [Crossref] [PubMed]
- Sun T, Du B, Diao Y, et al. ATAD2 expression increases [18F]Fluorodeoxyglucose uptake value in lung adenocarcinoma via AKT-GLUT1/HK2 pathway. BMB Rep 2019;52:457-62. [Crossref] [PubMed]
- Fu F, Zhang Y, Gao Z, et al. Development and validation of a five-gene model to predict postoperative brain metastasis in operable lung adenocarcinoma. Int J Cancer 2020;147:584-92. [Crossref] [PubMed]
- Chen Y, Zhang Y. CircDLG1 promotes malignant development of non-small cell lung cancer through regulation of the miR-630/CENPF axis. Strahlenther Onkol 2023;199:169-81. [Crossref] [PubMed]
- Li MX, Zhang MY, Dong HH, et al. Overexpression of CENPF is associated with progression and poor prognosis of lung adenocarcinoma. Int J Med Sci 2021;18:494-504. [Crossref] [PubMed]
- Ma W, Wang B, Zhang Y, et al. Prognostic significance of TOP2A in non-small cell lung cancer revealed by bioinformatic analysis. Cancer Cell Int 2019;19:239. [Crossref] [PubMed]
- Ho JY, Lu HY, Cheng HH, et al. UBE2S activates NF-κB signaling by binding with IκBα and promotes metastasis of lung adenocarcinoma cells. Cell Oncol (Dordr) 2021;44:1325-38. [Crossref] [PubMed]
- Liu J, Wen Y, Liu Z, et al. VPS33B modulates c-Myc/p53/miR-192-3p to target CCNB1 suppressing the growth of non-small cell lung cancer. Mol Ther Nucleic Acids 2020;23:324-35. [Crossref] [PubMed]
- Zhang LX, Gao J, Long X, et al. The circular RNA circHMGB2 drives immunosuppression and anti-PD-1 resistance in lung adenocarcinomas and squamous cell carcinomas via the miR-181a-5p/CARM1 axis. Mol Cancer 2022;21:110. [Crossref] [PubMed]
- Sun HF, Li LD, Lao IW, et al. Single-cell RNA sequencing reveals cellular and molecular reprograming landscape of gliomas and lung cancer brain metastases. Clin Transl Med 2022;12:e1101. [Crossref] [PubMed]
- Zhang Z, Cui F, Zhou M, et al. Single-cell RNA Sequencing Analysis Identifies Key Genes in Brain Metastasis from Lung Adenocarcinoma. Curr Gene Ther 2021;21:338-48. [Crossref] [PubMed]
(English Language Editor: J. Gray)