APOBEC mutational signature predicts prognosis and immunotherapy response in nonsmoking patients with lung adenocarcinoma
Highlight box
Key findings
• The APOBEC3 mutation is prevalent in nonsmoking lung adenocarcinoma (LUAD) patients. The APOBEC3 score was established to better understand the mutation pattern of LUAD and provide a prognostic biomarker for patients with LUAD.
What is known and what is new?
• The APOBEC3 gene family has been shown to play an important role in molecular tumorigenesis of non-small cell lung cancer.
• We found that the APOBEC3 family plays an important role in the mutation pattern of nonsmoking patients with LUAD.
What is the implication, and what should change now?
• APOBEC3 score provides a novel means of predicting prognosis and improving the immunotherapy response for future applications.
Introduction
According to GLOBOCAN 2021 data, lung cancer remains the most common malignancy and the leading cause of cancer mortality worldwide (1). Non-small cell lung cancer (NSCLC) is the dominant form of lung cancer, with lung adenocarcinoma (LUAD) being the most common histologic type of NSCLC, accounting for approximately 40% of total cases. Substantial promising advances in lung cancer evaluation and treatment have been achieved in recent years, including the increased utilization of low-dose chest computed tomography screen, advances in surgical strategies (2), improvements in chemotherapy (3,4), optimization of stereotactic ablative radiotherapy (5,6), and ever-emerging targeted agents and immunotherapy (7). However, the 5-year overall survival rate for advanced stage NSCLC remains remarkably poor. Unfortunateley, most lung cancers (up to 60%) are diagnosed at such later stages (8,9). Therefore, better understanding of the mechanisms of oncogenesis and greater identification of novel biomarkers for response or resistance to combination therapies are critical to herald the era of personalized medicine and provide greater benefits to more patients.
Acquisition of mutational patterns in NSCLC has been shown to play a role in somatic mutagenesis, leading to the initiation or progression of cancer (10). Somatic mutagenesis results from accumulated interactions with environmental factors and inherent susceptibility (11). Although tobacco smoking has always been considered the leading risk factor for NSCLC, the frequency of diagnosed nonsmoking NSCLC patients has dramatically increased from 8% to one-third in recent years and is even more common in women and those of Eastern Asian background (12-14). Recent studies have suggested that nonsmoking NSCLC should be distinguished from smoking NSCLC (15-17). However, the nonsmoking-related carcinogenesis and etiology remain poorly understood. Smoking carcinogens, especially benzo[a]pyrene cause the misreplication of DNA damage (18). Formation of DNA adducts, and the ultimate production of oncogenic mutations, which could be characterized as smoking-specific mutational signatures by mainly C > A transversion scattered in some hotspot regions (P53 and KRAS) (19,20).
Beyond extrinsic exposure, such as smoking, endogenous normal enzymatic activities, including DNA replication, DNA repair, and the DNA modification system, can also be sources of DNA mutation that play predominant roles in mutagenesis of nonsmoking NSCLC (17,20). Among various mutation-promoting genes, the apolipoprotein B mRNA editing enzyme, catalytic polypeptide-like 3 (APOBEC3) family has been implicated in extensive mutagenesis in multiple cancer types. These APOBEC induced mutations can accumulate constantly and contribute to tumor development, progression, metastasis, and therapeutic resistance (21,22). The APOBEC3 is an evolutionarily conserved cytidine deaminase family that catalyzes the transformation of cytosine into uracil (C > U) in single-stranded DNA and consists of 7 members (APOBEC3A–APOBEC3H) (23). Although each APOBEC3 family member has not been settled independently for cancer mutagenesis, evidence is available to suggest that most members are involved in the global mutation in cancer (24).
In the present study, we sought to establish a comprehensive characterization of the various genomic components that contribute to and result from APOBEC3 deamination in a single experimental system. We found that the APOBEC mutation particularly prevailed in nonsmoking LUAD patients by analyzing the mutation profile data of NSCLC in the TCGA database. Based on APOBE3 family gene expression levels, an assessment model for evaluating the APOBEC mutation status was constructed from nonsmoking patients in TCGA-LUAD. The significant predictive value of this model was further determined in other independent patient cohorts. Furthermore, our model can provide new insights into prognosis prediction and immunotherapy selection for nonsmoking LUAD patients. We present the following article in accordance with the TRIPOD reporting checklist (available at https://tlcr.amegroups.com/article/view/10.21037/tlcr-23-150/rc).
Methods
Data sets
This investigation evaluated LUAD patients with somatic mutation and RNA sequencing data from The Cancer Genome Atlas (TCGA). After removing duplicate samples, samples with less than 30 days of overall survival, and samples with incomplete clinical information, a total of 502 samples were deemed appropriate for inclusion and grouped by smoking history, with those who smoked less than 1 pack per year as the nonsmoking group and those who smoked more than 1 pack per year as the smoking group. Our study was validated using mutation, RNA sequencing, and clinical data from 46 nonsmoking LUAD patients from the luad_cptac_2020 dataset in the cBioportal database. To assess the predictive value of APOBEC3 score in immunotherapy, RNA sequencing and clinical data from 4 nonsmoking LUAD datasets were subsequently screened in the Gene Expression Omnibus: GSE135222 (n=27; treated with anti-PD-1), GSE13212 (n=56; log2 transformed robust multichip average normalized read count), GSE50081 (n=25), and GSE31210 (n=116). The study was conducted in accordance with the Declaration of Helsinki (as revised in 2013).
Data acquisition
Transcriptome and mutation data were obtained from the TCGA dataset for LUAD patients and from GSE135222 for lung cancer patients. TCGA mutation profiles and expression profiles (FPKM type) were obtained from the TCGA GDC platform (https://https://portal.gdc.cancer.gov/) and from the cBioportal database to obtain transcriptomic and mutational data from the luad_cptac_2020 dataset (https://www.cbioportal.org/study/summary?id=luad_cptac_2020). GSE135222, GSE50081, GSE13212, and GSE31210 transcriptome data were downloaded from the GEO database.
Data preprocessing
For transcriptome data, genes that were not expressed at more than 70% were removed, and the ComBet function in the R package “sva” was used to eliminate batch effects. Pooled IDs were mapped to gene symbols using annotations from GRCh38, and gene expression values were normalized using the “limma” (25) package in R. Transcriptomic data from GSE135222, GSE50081, GSE13212, and GSE31210 were converted to FPKM type. All samples in the dataset with an overall survival of more than 30 days were used for subsequent analysis.
Mutational signature analysis
Mutation features were extracted from TCGA-LUAD somatic mutation data using an R/CRAN package “sigminer” (26) (https://cran.r-project.org/web/packages/sigminer/) and the package maftools (27). sigminer first classified the mutation information into 6 substitution subtypes: C > A, C > G, C > T, T > A, T > C, and T > G. In addition, each substitution was examined by incorporating information from the 5' and 3' bases immediately adjacent to each mutant base, generating 96 possible mutation types. The copy number profile was calculated by counting the breakpoint count per 10 Mb per tumor (named BP10MB); breakpoint count per chromosome arm (named BPArm); copy number of fragments (named CN); copy number difference between adjacent fragments (named CNCP); length of oscillating copy number fragment strands (named OsCN); copy number fragment size based on log10 (named SS); the minimum number of chromosomes with 50% copy number variation (named NC50); and the genome-wide distribution of 8 basic copy number features such as chromosome burden (named BoChr) to count the copy number profiles. The above genomic alteration components were defined as SBS tumor-by-component matrices under the action of the hybrid model and were fed into the nonnegative matrix decomposition (NMF) algorithm for feature extraction. After performing 50 NMFs, the number of SBS signatures was determined. Features were extracted for downstream analysis based on co-epitope versus signature number plots. Relative and jedi exposures of each tumor signature were obtained using the sigminer software package. The relative exposure of each feature represents the ratio of its contribution to the tumor.
Construction of the APOBEC3 score
The least absolute shrinkage and selection operator (LASSO) regression model was used to identify the key markers among the APOBEC gene family and the corresponding coefficients for constructing the model in the TCGA cohort. All 11 genes of the APOBEC gene family with gene expression values were submitted to LASSO regression analysis, and LASSO regression was performed using the “glmnet” package (28) of R software. Finally, APOBEC3A and APOBEC3B with nonzero coefficients were identified to use the model construction, and the optimal lambda was 0.0048.
To quantify the APOBEC3 score model, we transformed the individual gene expression values of the mutation markers into an APOBEC3 score and calculated the score for each sample in LUAD using the gene expression values and the coefficients obtained by the LASSO algorithm. The APOBEC3 score was calculated for each sample. According to the median of the APOBEC3 score, LUAD samples were divided into the high- and low-APOBEC3 score subgroup. To assess the prognostic value of the APOBEC3 score, Kaplan-Meier (K-M) analysis was performed. The nomogram and the 1/3-year calibration curve validate the accuracy of the APOBEC3 scoring model.
Differential expressed genes (DEGs) and functional enrichment analysis
The samples were classified into high- or low-APOBEC3 score subgroups. The DEGs were selected between the high- and low- score subgroups, with the thresholds of P value <0.01 and log2|FoldChange| >1. Subsequently, these DEGs were submitted to DAVID (https://david.ncifcrf.gov/) for Kyoto Encyclopedia of Genes and Genomes (KEGG) analysis and Gene Ontology (GO) enrichment analysis to identify possible differences in pathways and functions. Among them, GO enrichment analysis included 3 categories: cellular component (CC), biological process (BP), and molecular function (MF). The results were visualized by using the R package “clusterProfiler”.
Immune-related features
“ssGSEA”, which is an R package that identifies immune cell infiltration in the tumor microenvironment using single-sample gene set enrichment analysis for the luad_cptac_2020 nonsmoking dataset. The CIBERSORT algorithm with 1,000 permutations was employed to evaluate the relative proportions of 22 types of immune cells.
Statistical analysis
R software (version 4.1.0) was used to conduct statistical analysis and generate figures. Kaplan–Meier survival analysis using “survival” and “surminer” R packages. The “forestplot” R package was used to plot forest plots. The plots were generated in this paper by R packages: “ggplot2”, “ggpubr”, “pheatmap”, “enrichplot”, and “corrplot”. The Spearman correlation test was used for APOBEC3 score-related analysis. The significance level was set at 0.05 (P<0.05).
Results
Mutational landscape in NSCLC
To explore the mutational landscape of NSCLC, we analyzed the somatic mutation data of NSCLC patients downloaded from the TCGA dataset. The results showed that missense mutations were the predominant variant classification occurring in patients with NSCLC (Figure 1A,1B). SNPs (single nucleotide polymorphisms) are the main form of variant (Figure 1C). C > A, C > G, and C > T base substitutions were the main common SNP types in patients with NSCLC (Figure 1D). During the development of cancer, characteristic mutation patterns that reveal the underlying mutational process were left behind. To investigate the mutational pattern during the development of NSCLC, we used the nonnegative matrix factorization (NMF) downscaling technique to identify the mutational signature. Based on multiple NMF runs, 4 mutational signatures were extracted from NSCLC somatic mutations, respectively, and cosine similarity analysis was performed between them and COSMIC signatures. Somatic mutation extraction results for NSCLC showed that signature1 was most similar to the COSMIC signature of smoking (cosine similarity =0.977), signature 2 resembled the DNA mismatch repair mutation signature (cosine similarity =0.829), signature 3 was associated with the APOBEC mutation (cosine similarity =0.707), and signature 4 resembled the UV irradiation mutation signature (cosine similarity =0.961; Figure S1A-S1C).
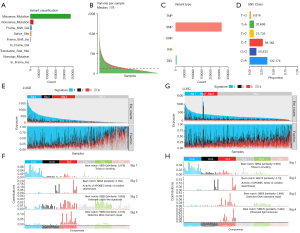
Given that LUAD and lung squamous cell carcinoma (LUSC) have different biological characteristics, clinical phenotypes, and treatments, it is reasonable to expect distinct mutation patterns to exist between LUAD and LUSC. Therefore, we analyzed the mutational characteristics of LUAD and LUSC. Mutational signatures were extracted from the somatic mutations of LUSC and LUAD patients in the TCGA database, respectively. The mutational patterns extracted in LUSC were consistent with the results for NSCLC (Figure 1E,1F; Figure S1D), except for fewer APOBEC mutation signatures. In contrast, LUADs contained more APOBEC3 mutations and no UV irradiation mutation signature (Figure 1G,1H; Figure S1E). These results suggested substantial heterogeneity of mutation patterns among NSCLC patients. At the same time, smoking-induced exogenous mutations and endogenous mutations caused by the APOBEC3 family dominated the mutation pattern. LUAD patients had more APOBEC mutation features compared to LUSC patients, and, as such, we speculate that APOBEC mutations may play a more important role in LUAD progression.
APOBEC mutagenesis is a major mutation pattern in nonsmoking patients with LUAD
Although associated with an increased mutational burden in LUAD, smoking was eliminated from mutagens in nonsmoking LUAD patients. To further investigate the mutagens and their related mutation patterns in nonsmoking LUAD patients, samples in TCGA-LUAD were divided into 2 cohorts: the nonsmoking cohort (n=111) and the smoking cohort (n=491) based on the medical information. After analyzing the overall proportion of SNPs in the nonsmoker and smoker cohorts, we found that C > T base substitution was the most common type in nonsmoking patients, whereas C > A transitions were most common in smoking patients (Figure 2A-2C). To further investigate the mutational patterns in smoking and nonsmoking patients, we identified several signatures in the smoker and nonsmoker cohorts based on NMFanalysis (Figure 2D-2G). The APOBEC mutation signature was enriched in the nonsmoker cohort, whereas tobacco smoking, DNA mismatch repair, and APOBEC mutations were present together in the smoker cohort. We then found that more than 76% of samples in the nonsmoker cohort were enriched for the APOBEC mutation feature. In contrast, in the smoker cohort, the mutation pattern was unevenly distributed across the 3 features (Figure 2H-2I). These findings were consistent with those of the luad_cptac_2020 dataset, in which APOBEC mutation occurred predominantly in the nonsmoking samples (Figure S2A-S2D). These results suggest that different mutation patterns existed between smoking and nonsmoking patients, and the mutation pattern of nonsmoking patients was primarily associated with an APOBEC mutation.
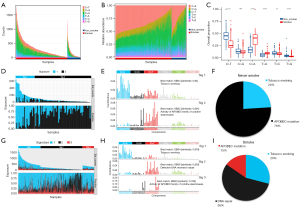
Assessment of mutational characteristics of APOBEC mutations in nonsmoking LUAD
The APOBEC mutation signature caused by the APOBEC3 family is one of the major endogenous mutations in human cancers. Recent study has shown that the APOBEC3 family was a major cause of APOBEC mutation, and a combination of mutations (including TCA to TTA or TGA, TCT to TTT or TGT) in the TCW (W = A or T) mutation count can indicate the status of APOBEC mutations (21). Therefore, we calculated TCW counts for each sample in the TCGA nonsmoking dataset and determined the effect of the APOBEC3 family of genes on TCW mutation counts (Figure 3A-3D; Figure S2E-S2G). The results showed that APOBEC3A, APOBEC3B, APOBEC3D, and APOBC3F were significantly associated with TCW counts. To comprehensively analyze the effect of APOBEC3 family gene expression on APOBEC mutations, we performed LASSO regression analysis using the above genes to establish APOBEC mutation assessment scores. Finally, an assessment score consisting of APOBEC3A and APOBEC3B was constructed based on the LASSO regression analysis (Figure 3E,3F). To verify the accuracy of the APOBEC3 score, we determined the correlation between the assessment score and the TCW counts. APOBEC3 score was more significantly related to the TCW counts (Figure 3G; r=0.45; P<0.0001) than the individual genes of the APOBEC3 family. The results were consistent in the luad_cptac_2020 dataset (Figure 3H; r=0.36; P<0.001). Subsequently, we divided the sample into 2 groups based on the median of APOBEC3 scores (Figure 3I). TCW counts were significantly higher in the high APOBEC3 score group than in the low APOBEC3 score group (Figure 3J). There were more tumor neoantigens in the high APOBEC3 score group (P=0.0019; Figure 3K).
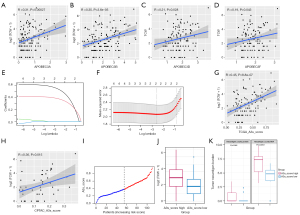
Immune features between the APOBEC3 score high and low patients
To further understand the differences between the low APOBEC3 score and high APOBEC3 score groups, we first identified differentially expressed genes (DEGs) between the 2 subgroups. We detected 1,018 DEGs in the TCGA nonsmoking LUAD and 433 DEGs in luad_cptac_2020 nonsmoking mRNA datasets, and 218 DEGs in the luad_cptac_2020 proteome data (Figure S3A-S3C). We then further analyzed the enrichment pathways for mutation signature by performing GO terminology and KEGG pathway enrichment using these DEGs. The results showed that DEGs upregulated in the APOBEC3 score high group were significantly enriched in BPs, mainly in the regulation of immune cells such as leukocytes, lymphocytes, and T cells, besides in cell cycle regulation, with the regulation of cytotoxicity and natural killer cell also observed in the analysis of the luad_cptac_2020 dataset (Figure 4A; Figure S3D). KEGG pathway enrichment analysis revealed that these DEGs were enriched in the categories of natural killer cell-mediated cytotoxicity as well as IL-17 signaling pathway and cytokine-cytokine interactions (Figure 4B; Figure S3E). Proteome enrichment analysis shows the categories related to the immune regulation process, including migration of immune cells (such as T cells, monocytes, and B cells) and humoral immunity (Figure 4C,4D). To further investigate the immune profiles of high APOBEC3 and low APOBEC3 scoring patients, we deconstructed the tumor samples using the CIBERSORT algorithm to determine differences in the proportions of 22 immune cell types between high and low scoring subtypes (Figure 4E,4F). Similar results were revealed in the luad_cptac_2020 dataset using the ssGSEA algorithm (Figure 4G). The results showed that patients in the high subgroup had more resting CD4 memory T cells and CD8 T cells compared to the low subgroup, but there were also more tumor-associated macrophages in the high subgroup. Immune cells such as dendritic cells, resting mast cells, activated natural killer (NK) cells, and macrophages were present in the low APOBEC3 score group.
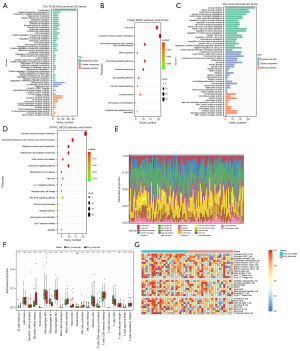
Prognostic value of the mutation signature
With the aim of further elucidating the prognostic value of the APOBEC3 scoring model in nonsmoking LUAD, we used Kaplan–Meier analysis to assess the prognostic effect of the model in several different cohorts. Kaplan–Meier survival curves showed that patients in the high scoring subgroup had a significantly worse prognosis (Figure 5A). To quantify the analysis of the prognosis of LUAD patients, we constructed a nomogram combining the APOBEC3s_score and clinical information from the TGGA-LUAD cohort (Figure 5B). This nomogram showed that APOBEC3 score and clinical staging were important prognostic factors for nonsmoking LUAD patients. The predictive power of the 3-year overall survival (OS) calibration chart was reduced compared to the 1-year OS calibration chart (Figure 5C,5D). In 3 other independent nonsmoking LUAD cohorts, the APOBEC3 score model was validated by K-M survival curves, and all results showed a poorer prognosis for patients in the high APOBEC3 score group (Figure 5E,5F). In addition, given the higher ICB-associated immune cell infiltration in the group with high APOBEC3 scores, we additionally validated this in another cohort receiving anti-PD-1 therapy (GSE135222), where we observed a significantly higher durable clinical benefit (DCB) APOBEC3 score than patients with nondurable benefit (Figure 5G). The progression-free survival in the group with high APOBEC3 scores compared to the group with low APOBEC3 scores time was longer (Figure 5H), and significantly more mutations were observed in patients with durable clinical benefit (DCB) than in patients with nondurable benefit (NDB; Figure 5I). These results suggest our model can be used as an independent prognostic factor.
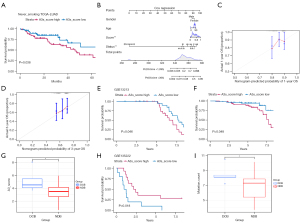
Discussion
NSCLC is a dynamic and complex malignancy, with disease courses spanning steps of initiation, progression, metastasis, and relapse. Somatic mutations acquired stochastically play more important roles than stationary germline and epigenetic variations. Although the somatic mutations distributing in whole genome cancer sequences are extremely large, mutational processes could be mainly caused by both exogenous and endogenous mutagens (29,30). Moreover, the mutations exhibit distinct mutagens-specific mutational signatures, and we may extrapolate the different mutagens through mathematical deconvolution analysis in somatic mutations from individual cancer genomes (26). In this study, we generated the mutational landscape of NSCLC and extracted the mutational signatures, which mainly were attributed to smoking-induced exogenous mutations and APOBEC family-induced endogenous mutations. We emphasized mutation patterns between smoking and nonsmoking patients and indicated that nonsmoking NSCLC patients predominantly tend to have APOBEC mutation signatures.
The APOBEC3 family of enzymes has been considered a major endogenous source of somatic mutations found in more than 75% of cancer types and approximately 50% of all cancer genomes (31-33). There are 7 APOBEC3 families of enzymes in humans, which can all catalyze cytosine deamination to form uracil in the genome and induce somatic mutations, including several types of clustered single-, doublet- and multi-base substitutions, diffuse hypermutation (omikli), and longer strand-coordinated events (kataegis) (34,35). Our findings showed that APOBEC3A, APOBEC3B, APOBEC3D, and APOBEC3F were positively involved in the APOBEC mutation of nonsmoking LUAD. Given that each APOBEC gene is expressed at different levels in nonsmoking NSCLC individuals and the specific mechanism of individual APOBEC3 enzymes is not well established, we performed LASSO regression analysis and generated the APOBEC3 score, which can incorporate the expression level of APOBEC3A and APOBEC3B respectively. Among all APOBEC3 enzymes, APOBEC3A and APOBEC3B were reported to be the predominant mutagens (36), APOBEC3A preferred YTCA sequence, and APOBEC3B favored RTCA sequence (Y and R indicates purine, and pyrimidine, respectively) (35,37). APOBEC3A/APOBEC3B double-knockout and APOBEC3A-APOBEC3B hybrid transcript research implied that APOBEC3A/APOBEC3B crosstalk would contribute to the APOBEC mutation signature in cancer (33). Our analysis demonstrated that the APOBEC score was more significantly correlated with APOBEC mutation signature than each individual APOBEC expression, and the average mutation number of high-APOBEC3 score patients is significantly higher than low-APOBEC3 score patients.
Immune checkpoint blockade (ICB) therapy, including monoclonal antibodies targeting the immune inhibitory proteins, and programmed death-1 (PD-1), has achieved remarkable clinical benefits in LUAD (38). However, the identity of biomarkers that predict the response to ICB remains challenging. Tumor mutational burden (TMB) that may generate mutant tumor peptides (immune-reactive neoantigens) and increase tumor immunogenicity emerged as a leading candidate biomarker (39). Our results showed that the vast majority of somatic mutations or TMB in the nonsmoking samples could be categorized as APOBEC-associated mutations. Translational research in LUAD yields an ever-growing database, and transcriptome data were far more than somatic mutation. By employing APOBEC score analysis in RNA sequencing data or original expression data of the APOBECs family, we could effectively estimate the TMB of nonsmoking LUAD.
Recent study has also demonstrated that overexpression of APOBEC3B can induce heteroclitic neoepitopes, which promotes sensitivity to ICB in a mouse model of melanoma, and APOBEC activity promotes T cell infiltration in HER2-driven mouse mammary tumors (40). In order to investigate the relationship between APOBEC-mediated hypermutation and immunogenicity in nonsmoking LUAD, GO, and KEGG pathway enrichment, analysis showed that high-APOBEC3 score patients appeared to contain more immune response-related genes and altered immune pathways. Furthermore, we investigated the APOBEC3 score effect on diverse immune cell types in nonsmoking LUAD samples, and the high APOBEC3 score group was enriched with CD4 memory T cells and CD8 T cells infiltration. It is well known that Tumor microenvironment (TME) in LUAD plays a prominent role in the multiple interactions between tumor cells and the immune system, and is correlated with response to cancer treatment, especially immunotherapy. TME-based signatures in LUAD will play a reliable role as predictors of immunotherapy. CD4 memory T cells and CD8 T cells infiltration have been identified as a valuable biomarker in discriminating patients with a significantly longer PFS after ICI treatment (41,42). In addition, the APOBEC3 family is engaged directly in the immune response, including the origin and evolution of immunity, diversification of antigen receptor genes, and virus protection. We have shown the relationships between APOBEC3-mediated mutational signature and immune alterations and immunotherapy response of cancer, and that the APOBEC3 score could serve as a promising biomarker for ICB during nonsmoking LUAD therapy.
Conclusions
We analyzed mutation patterns in patients with NSCLC and found that APOBEC3 mutations were prevalent in nonsmoking LUAD patients. Furthermore, we constructed a model for predicting APOBEC3 mutation status in nonsmoking LUAD patients. We validated the predictive effect of this model in other cohorts. Additionally, our model provides new insights to assess patient prognosis and predict the immunotherapy response in nonsmoking patients with LUAD.
Acknowledgments
The authors appreciate the academic support from the AME Lung Cancer Collaborative Group.
Funding: This work was supported by the National Natural Science Foundation of China (No. 81902329), and The National Science Foundation of Heilongjiang Province of China (No. CZKYF2021B004).
Footnote
Reporting Checklist: The authors have completed the TRIPOD reporting checklist. Available at https://tlcr.amegroups.com/article/view/10.21037/tlcr-23-150/rc
Peer Review File: Available at https://tlcr.amegroups.com/article/view/10.21037/tlcr-23-150/prf
Conflicts of Interest: All authors have completed the ICMJE uniform disclosure form (available at https://tlcr.amegroups.com/article/view/10.21037/tlcr-23-150/coif). The authors have no conflicts of interest to declare.
Ethical Statement: The authors are accountable for all aspects of the work in ensuring that questions related to the accuracy or integrity of any part of the work are appropriately investigated and resolved. The study was conducted in accordance with the Declaration of Helsinki (as revised in 2013).
Open Access Statement: This is an Open Access article distributed in accordance with the Creative Commons Attribution-NonCommercial-NoDerivs 4.0 International License (CC BY-NC-ND 4.0), which permits the non-commercial replication and distribution of the article with the strict proviso that no changes or edits are made and the original work is properly cited (including links to both the formal publication through the relevant DOI and the license). See: https://creativecommons.org/licenses/by-nc-nd/4.0/.
References
- Sung H, Ferlay J, Siegel RL, et al. Global Cancer Statistics 2020: GLOBOCAN Estimates of Incidence and Mortality Worldwide for 36 Cancers in 185 Countries. CA Cancer J Clin 2021;71:209-49. [Crossref] [PubMed]
- Lemjabbar-Alaoui H, Hassan OU, Yang YW, et al. Lung cancer: Biology and treatment options. Biochim Biophys Acta 2015;1856:189-210. [PubMed]
- Heinrich S, Lang H. Neoadjuvant Therapy of Pancreatic Cancer: Definitions and Benefits. Int J Mol Sci 2017;18:1622. [Crossref] [PubMed]
- Versteijne E, van Dam JL, Suker M, et al. Neoadjuvant Chemoradiotherapy Versus Upfront Surgery for Resectable and Borderline Resectable Pancreatic Cancer: Long-Term Results of the Dutch Randomized PREOPANC Trial. J Clin Oncol 2022;40:1220-30. [Crossref] [PubMed]
- Shah JL, Loo BW Jr. Stereotactic Ablative Radiotherapy for Early-Stage Lung Cancer. Semin Radiat Oncol 2017;27:218-28. [Crossref] [PubMed]
- Mirimanoff RO. Stereotactic ablative body radiotherapy (SABR): an alternative to surgery in stage I-II non-small-cell cancer of the lung? Chin Clin Oncol 2015;4:42. [PubMed]
- Nagasaka M, Gadgeel SM. Role of chemotherapy and targeted therapy in early-stage non-small cell lung cancer. Expert Rev Anticancer Ther 2018;18:63-70. [Crossref] [PubMed]
- Casal-Mouriño A, Valdés L, Barros-Dios JM, et al. Lung cancer survival among never smokers. Cancer Lett 2019;451:142-9. [Crossref] [PubMed]
- Koike T, Goto T, Kitahara A, et al. Characteristics and timing of recurrence during postoperative surveillance after curative resection for lung adenocarcinoma. Surg Today 2017;47:1469-75. [Crossref] [PubMed]
- Ruwali M, Shukla R. Interactions of Environmental Risk Factors and Genetic Variations: Association with Susceptibility to Cancer. In: Singh A, Srivastava S, Rathore D et al., editors. Environmental Microbiology and Biotechnology: Volume 2: Bioenergy and Environmental Health. Singapore: Springer Singapore; 2021: 211-34.
- Devarakonda S, Li Y, Martins Rodrigues F, et al. Genomic Profiling of Lung Adenocarcinoma in Never-Smokers. J Clin Oncol 2021;39:3747-58. [Crossref] [PubMed]
- Chen YJ, Roumeliotis TI, Chang YH, et al. Proteogenomics of Non-smoking Lung Cancer in East Asia Delineates Molecular Signatures of Pathogenesis and Progression. Cell 2020;182:226-244.e17. [Crossref] [PubMed]
- Kawaguchi T, Matsumura A, Fukai S, et al. Japanese ethnicity compared with Caucasian ethnicity and never-smoking status are independent favorable prognostic factors for overall survival in non-small cell lung cancer: a collaborative epidemiologic study of the National Hospital Organization Study Group for Lung Cancer (NHSGLC) in Japan and a Southern California Regional Cancer Registry databases. J Thorac Oncol 2010;5:1001-10. [Crossref] [PubMed]
- Ben X, Tian D, Liang J, et al. APOBEC3B deletion polymorphism and lung cancer risk in the southern Chinese population. Ann Transl Med 2021;9:656. [Crossref] [PubMed]
- Corrales L, Rosell R, Cardona AF, et al. Lung cancer in never smokers: The role of different risk factors other than tobacco smoking. Crit Rev Oncol Hematol 2020;148:102895. [Crossref] [PubMed]
- Couraud S, Zalcman G, Milleron B, et al. Lung cancer in never smokers – A review. European Journal of Cancer 2012;48:1299-311. [Crossref] [PubMed]
- Gou LY, Niu FY, Wu YL, et al. Differences in driver genes between smoking-related and non-smoking-related lung cancer in the Chinese population. Cancer 2015;121:3069-79. [Crossref] [PubMed]
- Alexandrov LB, Ju YS, Haase K, et al. Mutational signatures associated with tobacco smoking in human cancer. Science 2016;354:618-22. [Crossref] [PubMed]
- VanderLaan PA, Rangachari D, Mockus SM, et al. Mutations in TP53, PIK3CA, PTEN and other genes in EGFR mutated lung cancers: Correlation with clinical outcomes. Lung Cancer 2017;106:17-21. [Crossref] [PubMed]
- Liu X, Lin XJ, Wang CP, et al. Association between smoking and p53 mutation in lung cancer: a meta-analysis. Clin Oncol (R Coll Radiol) 2014;26:18-24. [Crossref] [PubMed]
- Roper N, Gao S, Maity TK, et al. APOBEC Mutagenesis and Copy-Number Alterations Are Drivers of Proteogenomic Tumor Evolution and Heterogeneity in Metastatic Thoracic Tumors. Cell Rep 2019;26:2651-66.e6. [Crossref] [PubMed]
- Jakobsdottir GM, Brewer DS, Cooper C, et al. APOBEC3 mutational signatures are associated with extensive and diverse genomic instability across multiple tumour types. BMC Biol 2022;20:117. [Crossref] [PubMed]
- Salter JD, Bennett RP, Smith HC. The APOBEC Protein Family: United by Structure, Divergent in Function. Trends Biochem Sci 2016;41:578-94. [Crossref] [PubMed]
- Covino DA, Gauzzi MC, Fantuzzi L. Understanding the regulation of APOBEC3 expression: Current evidence and much to learn. J Leukoc Biol 2018;103:433-44. [Crossref] [PubMed]
- Ritchie ME, Phipson B, Wu D, et al. limma powers differential expression analyses for RNA-sequencing and microarray studies. Nucleic Acids Res 2015;43:e47. [Crossref] [PubMed]
- Wang S, Li H, Song M, et al. Copy number signature analysis tool and its application in prostate cancer reveals distinct mutational processes and clinical outcomes. PLoS Genet 2021;17:e1009557. [Crossref] [PubMed]
- Mayakonda A, Lin DC, Assenov Y, et al. Maftools: efficient and comprehensive analysis of somatic variants in cancer. Genome Res 2018;28:1747-56. [Crossref] [PubMed]
- Engebretsen S, Bohlin J. Statistical predictions with glmnet. Clin Epigenetics 2019;11:123. [Crossref] [PubMed]
- Martincorena I, Campbell PJ. Somatic mutation in cancer and normal cells. Science 2015;349:1483-9. [Crossref] [PubMed]
- Morley AA, Turner DR. The contribution of exogenous and endogenous mutagens to in vivo mutations. Mutat Res 1999;428:11-5. [Crossref] [PubMed]
- Guo H, Zhu L, Huang L, et al. APOBEC Alteration Contributes to Tumor Growth and Immune Escape in Pan-Cancer. Cancers (Basel) 2022;14:2827. [Crossref] [PubMed]
- Roberts SA, Lawrence MS, Klimczak LJ, et al. An APOBEC cytidine deaminase mutagenesis pattern is widespread in human cancers. Nat Genet 2013;45:970-6. [Crossref] [PubMed]
- Petljak M, Dananberg A, Chu K, et al. Mechanisms of APOBEC3 mutagenesis in human cancer cells. Nature 2022;607:799-807. [Crossref] [PubMed]
- Bergstrom EN, Luebeck J, Petljak M, et al. Mapping clustered mutations in cancer reveals APOBEC3 mutagenesis of ecDNA. Nature 2022;602:510-7. [Crossref] [PubMed]
- Mas-Ponte D, Supek F. DNA mismatch repair promotes APOBEC3-mediated diffuse hypermutation in human cancers. Nat Genet 2020;52:958-68. [Crossref] [PubMed]
- Wang S, Jia M, He Z, et al. APOBEC3B and APOBEC mutational signature as potential predictive markers for immunotherapy response in non-small cell lung cancer. Oncogene 2018;37:3924-36. [Crossref] [PubMed]
- Jalili P, Bowen D, Langenbucher A, et al. Quantification of ongoing APOBEC3A activity in tumor cells by monitoring RNA editing at hotspots. Nat Commun 2020;11:2971. [Crossref] [PubMed]
- Yu Y, Zeng D, Ou Q, et al. Association of Survival and Immune-Related Biomarkers With Immunotherapy in Patients With Non-Small Cell Lung Cancer: A Meta-analysis and Individual Patient-Level Analysis. JAMA Netw Open 2019;2:e196879. [Crossref] [PubMed]
- Wang P, Chen Y, Wang C. Beyond Tumor Mutation Burden: Tumor Neoantigen Burden as a Biomarker for Immunotherapy and Other Types of Therapy. Front Oncol 2021;11:672677. [Crossref] [PubMed]
- Driscoll CB, Schuelke MR, Kottke T, et al. APOBEC3B-mediated corruption of the tumor cell immunopeptidome induces heteroclitic neoepitopes for cancer immunotherapy. Nat Commun 2020;11:790. [Crossref] [PubMed]
- Fumet JD, Richard C, Ledys F, et al. Prognostic and predictive role of CD8 and PD-L1 determination in lung tumor tissue of patients under anti-PD-1 therapy. Br J Cancer 2018;119:950-60. [Crossref] [PubMed]
- Fumet JD, Richard C, Ledys F, et al. Correction: Prognostic and predictive role of CD8 and PD-L1 determination in lung tumor tissue of patients under anti-PD-1 therapy. Br J Cancer 2019;121:283. [Crossref] [PubMed]
(English Language Editor: C. Mullens)