Development and validation of a deep transfer learning-based multivariable survival model to predict overall survival in lung cancer
Highlight box
Key findings
• Integration of deep learning and transfer learning improves the performance of a survival model for lung cancer.
What is known and what is new?
• Many deep learning-based survival models are emerging in different disease interests. However, models integrating deep learning and transfer learning are rare.
• Our innovative multivariable survival model for lung cancer integrates deep learning and transfer learning. It has superior performance compared with the traditional Cox model. The model also has a robust performance in the setting of missing data and outputs the AI certainty of the prediction, which are two unique features.
What is the implication, and what should change now?
• The survival model can be used for patient self-evaluation and baseline risk stratification in clinical trials. The pre-trained model also allows researchers to fine-tune the model with a sample from the targeted population and explore other prognostic factors by integrating their own database.
Introduction
Lung cancer is a concerning global health burden with a 5-year survival rate of 18.6%, much lower than for other leading cancers, such as colorectal (64.5%), breast (89.6%), and prostate (98.2%), according to Surveillance, Epidemiology, and End Results (SEER) Cancer Statistics (1). Given the relatively poor outcome of lung cancer, it is imperative for a precise survival prediction system to risk-stratify patients in clinical practice and research.
The tumor-node-metastasis (TNM) staging is widely used to evaluate prognosis and guide the treatment strategy. However, it classifies patients based on the anatomic extent of the tumor broadly without weighting other prognostic factors (2). Other risk factors have been proved as independent and related to prognosis, including sex, age, and marital status at diagnosis (3-5). Therefore, heterogeneity in clinical outcomes exists even between patients with comparable stages of the disease. Moreover, the standard Cox proportional hazards (CPH) model, which is one of the most popular models used in survival analysis, is a linear regression model that oversimplifies survival analysis for real-world applications because it assumes linearity between variables, which is not valid.
Artificial intelligence (AI) is becoming widely applied in the medical field, driven by a staggering increase in computational power and data volumes. Deep learning is a subset of machine learning that has greatly interested medical practitioners and researchers (6-10). Transfer learning is another subset of machine learning, in which a model developed for one domain can be used for a different but related domain, independent of data size and distribution. In comparison, traditional machine learning is characterized by training data that have the same input feature space and data distribution characteristics (11).
Many deep learning-based models are emerging to predict outcomes in different disease areas (8,9). For example, Bergquist et al. utilized computerized methods such as random forests, lasso regression, and neural networks to predict lung cancer stages with 93% accuracy by analyzing clinical data from the SEER cancer registry (12). Similarly, Corey et al. developed Pythia, a software package based on machine learning models that incorporates various factors including age, sex, clinical baseline, race/ethnicity, and comorbidity history to predict the risk of postoperative complications or deaths (13). However, models integrating deep learning and transfer learning are rare. Deep learning models may have limitations in real-world populations due to differences in variables and characteristics between the training and real-world populations. However, transfer learning, a subset of machine learning, can overcome this limitation by allowing a model developed for one domain to be used for a related domain. In this study, we aimed to integrate deep learning and transfer learning through fine-tuning to develop a new model that can be tailored to different populations worldwide. We present the following article in accordance with the TRIPOD reporting checklist (available at https://tlcr.amegroups.com/article/view/10.21037/tlcr-23-84/rc).
Methods
We present an elaborate deep transfer learning-based survival model for lung cancer using a training cohort of 601,480 patients from the SEER database (Surveillance, Epidemiology, and End Results database 2006–15) and a testing cohort of 4,512 patients from the GYFY database (the First Affiliated Hospital of Guangzhou Medical University database 2006–18). The outcome was based on overall survival, the period (in months) between diagnosis and death or loss of follow-up from any cause. The performance of our model was compared with that of a traditional Cox model by C-indexes. We developed the primary model based on the DeepSurv model (14) with a training dataset from the SEER database. The primary model was internally validated with a dataset from SEER, and finally externally validated by transfer learning with the GYFY database. We also explore the model’s performance in the setting of missing data and output the AI certainty of the prediction. More information regarding data collection, data preparation, data coding, deep learning algorithm, model evaluation and statistical analysis can be found in the Appendix 1.
In this study, only de-identified data were used, so ethical review and informed consent were waived by the institutional review board of The First Affiliated Hospital of Guangzhou Medical University. The study was conducted in accordance with the Declaration of Helsinki (as revised in 2013).
Statistical analysis
The outcome was measured based on overall survival, the period (in months) between diagnosis and death or loss of follow-up from any cause, as reported in the SEER and GYFY databases. Features were expressed as counts and percentages for categorical variables and as the mean [standard deviation (SD)] or median (range) for continuous variables. Qualitative and quantitative differences between subgroups were analyzed using the chi-squared test or Fisher’s exact test for categorical parameters and Student’s t-test or the Mann-Whitney U test for continuous parameters, as appropriate. Univariable and multivariable Cox proportional hazards regression models were used to estimate the effects of various variables on the hazard of lung cancer occurrence and develop the lung cancer prediction model. The cumulative incidence of death was estimated by the Kaplan-Meier (K-M) method and compared using the log-rank test. The concordance index (C-index) was used to assess the discriminatory powers of the models, and the survival calibration curve was calculated to evaluate the calibration of the probability of survival as predicted by the model versus the observed probability. Statistical analysis was performed with R (Version 4.0.0). P<0.05 was statistically significant, and all tests were two-sided.
Results
Demographics and risk factor analysis
The whole modeling flow of the deep transfer learning-based survival model and its applications are shown in Figure 1. A total of 601,480 patients with lung cancer in the SEER database and 4,521 patients in the GYFY database were included; 52.7% and 56.2% were men in SEER and GYFY, respectively. Additionally, SEER had 82.7% white population, 10.9% black population, and 6.3% others, and GYFY only contained an Asian population. The median ages of diagnosis were 70 and 59 years in these two databases (see Table 1 for details). Univariable and multivariable Cox regression analyses showed that all 18 variables, except race, were significantly associated with outcome in the SEER dataset. In the GYFY dataset, the factors that significantly related to the outcome were sex, age, grade, Collaborative staging (CS) extension (2004–15), CS lymph nodes (2004–15), and CS site-specific factor 1 (2004–) (see Tables S1,S2 in Supplementary for details).
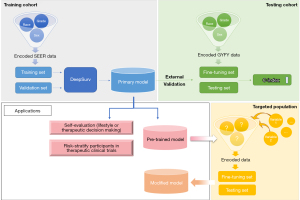
Table 1
Variable | Training cohort (N=601,480) | Testing cohort (N=4,521) |
---|---|---|
Age (years), mean (SD) | 69.63 (11.26) | 58.77 (10.65) |
CS-tumor size (mm), median (IQR) | 49 (26, 994) | 25.00 (15.00, 40.00) |
CS extension (2004–15), median (IQR) | 420 (100, 720) | 400.00 (100.00, 410.00) |
CS lymph nodes (2004–15), median (IQR) | 200 (0, 200) | 0.00 (0.00, 200.00) |
CS Mets at dx (2004–), median (IQR) | 15 (0, 40) | 20.00 (0.00, 25.00) |
Regional nodes exam (1988–), median (IQR) | 0 (0, 4) | 15.00 (7.00, 25.00) |
Regional nodes positive (1988–), median (IQR) | 98 (95, 98) | 0.00 (0.00, 5.00) |
Sex, n (%) | ||
Male | 316,856 (52.7) | 2,539 (56.2) |
Female | 284,624 (47.3) | 1,982 (43.8) |
Race, n (%) | ||
White | 497,675 (82.7) | 0 (0.0) |
Black | 65,743 (10.9) | 0 (0.0) |
Other | 37,541 (6.2) | 4,521 (100.0) |
Unknown | 521 (0.1) | 0 (0.0) |
Marital status at diagnosis, n (%) | ||
Married (including common law) | 299,132 (49.7) | 4,354 (96.3) |
Divorced | 72,991 (12.1) | 4 (0.1) |
Separated | 6,413 (1.1) | 0 (0.0) |
Single (never married) | 76,133 (12.7) | 58 (1.3) |
Unknown | 26,112 (4.3) | 105 (2.3) |
Widowed | 119,984 (19.9) | 0 (0.0) |
Unmarried or domestic partner | 715 (0.1) | 0 (0.0) |
Chemotherapy recode, n (%) | ||
No/unknown | 356,650 (59.3) | 3,142 (69.5) |
Yes | 244,830 (40.7) | 1,379 (30.5) |
Grade, n (%) | ||
Well differentiated; Grade I | 29,752 (4.9) | 503 (11.1) |
Moderately differentiated; Grade II | 88,404 (14.7) | 1,538 (34.0) |
Poorly differentiated; Grade III | 137,294 (22.8) | 1,103 (24.4) |
Undifferentiated; anaplastic; Grade IV | 22,721 (3.8) | 7 (0.2) |
Unknown | 323,309 (53.8) | 1,370 (30.3) |
Laterality, n (%) | ||
Not a paired site | 0 (0.0) | 24 (0.5) |
Bilateral, single primary | 7654 (1.3) | 9 (0.2) |
Left—origin of primary | 232,999 (38.8) | 1,792 (39.6) |
Right—origin of primary | 327,185 (54.4) | 2,670 (59.1) |
Only one side or side unspecified | 2,970 (0.5) | 13 (0.3) |
Paired site, but no information concerning laterality | 30,193 (5.0) | 13 (0.3) |
Radiation sequence with surgery, n (%) | ||
Intraoperative rad with other rad before/after surgery | 35 (0.0) | 12 (0.3) |
Intraoperative rad | 144 (0.0) | 13 (0.3) |
No rad and/or cancer-directed surgery | 551,636 (91.7) | 4,372 (96.7) |
Rad after surgery | 43,367 (7.2) | 92 (2.0) |
Rad before and after surgery | 703 (0.1) | 8 (0.2) |
Rad prior to surgery | 4,708 (0.8) | 17 (0.4) |
Sequence unknown, but both given | 7,77 (0.1) | 7 (0.2) |
Surgery both before and after rad | 110 (0.0) | 0 (0.0) |
Radiation recode, n (%) | ||
Beam radiation | 210,309 (35.2) | 87 (1.9) |
Combination of beam with implants or isotopes | 488 (0.1) | 1 (0.0) |
None/unknown | 372,124 (62.2) | 4,396 (97.2) |
Radiation, NOS method or source not specified | 0 (0.0) | 26 (0.6) |
Radioactive implants | 764 (0.1) | 0 (0.0) |
Radioisotopes | 117 (0.0) | 0 (0.0) |
Recommended, unknown if administered | 4,632 (0.8) | 9 (0.2) |
Refused | 9,492 (1.6) | 2 (0.0) |
Lung-surgery to primary site (1988–2015), n (%) | ||
Complete/total/standard pneumonectomy; pneumonectomy, NOS | 2,021 (0.3) | 43 (1.0) |
Extended pneumonectomy | 244 (0.0) | 3 (0.1) |
Lobectomy/bilobectomy | 91,031 (15.1) | 3,461 (76.6) |
Local surgical excision or destruction of lesion | 1,802 (0.3) | 0 (0.0) |
No surgery of primary site | 464,612 (77.2) | 76 (1.7) |
Partial/wedge/segmental Resec., lingulectomy, partial lobectomy, sleeve Resec. | 30,099 (5.0) | 896 (19.8) |
Radical pneumonectomy (complete pneumonectomy plus dissection of mediastinal ln) | 3,894 (0.6) | 32 (0.7) |
Surgery, NOS | 1,303 (0.2) | 0 (0.0) |
Unknown | 6,474 (1.1) | 10 (0.2) |
CS site-specific factor 1 at presentation (2004–), n (%) | ||
No separate tumor nodules noted | 210,446 (35.0) | 2,724 (60.3) |
Separate tumor nodules in ipsilateral lung, same lobe | 21,252 (3.5) | 404 (8.9) |
Separate tumor nodules in ipsilateral lung, different lobe | 19,135 (3.2) | 610 (13.5) |
Separate tumor nodules, ipsilateral lung, same and different lobe | 12,180 (2.0) | 311 (6.9) |
Separate tumor nodules, ipsilateral lung, unknown if same or different lobe | 13,476 (2.2) | 104 (2.3) |
Not applicable: information not collected for this case | 252,900 (42.0) | 0 (0.0) |
Unknown if separate tumor nodules; separate tumor nodules cannot be assessed; not documented in patient record | 72,091 (12.0) | 368 (8.1) |
Histologic Type ICD-O-3, n (%) | ||
Adenocarcinoma | 231,441 (38.5) | 3,530 (78.1) |
Adenosquamous | 6,637 (1.1) | 63 (1.4) |
Large cell carcinoma | 8,777 (1.5) | 25 (0.6) |
Neuroendocrine cancer | 19,881 (3.3) | 54 (1.2) |
Non-small cell lung cancer | 66,504 (11.1) | 10 (0.2) |
Sarcomatoid carcinoma | 3,785 (0.6) | 47 (1.0) |
Signet ring cell carcinoma | 762 (0.1) | 0 (0.0) |
Small cell carcinoma | 74,067 (12.3) | 64 (1.4) |
Squamous cell carcinoma | 119,682 (19.9) | 620 (13.7) |
Undifferentiated carcinoma | 470 (0.1) | 1 (0.0) |
Other | 69,474 (11.6) | 107 (2.4) |
Sequence number (the sequence of all reportable neoplasms over the lifetime of the patient), n (%) | ||
1 primary only | 428,044 (71.2) | 4,347 (96.2) |
1st of ≥2 primaries | 27,153 (4.5) | 35 (0.8) |
2nd of ≥2 primaries | 116,426 (19.4) | 137 (3.0) |
3rd of ≥3 primaries | 24,170 (4.0) | 2 (0.0) |
4th of ≥4 primaries | 4,587 (0.8) | 0 (0.0) |
5th of ≥5 primaries | 845 (0.1) | 0 (0.0) |
6th of ≥6 primaries | 165 (0.0) | 0 (0.0) |
7th of ≥7 primaries | 52 (0.0) | 0 (0.0) |
8th of ≥8 primaries | 13 (0.0) | 0 (0.0) |
9th of ≥9 primaries | 5 (0.0) | 0 (0.0) |
10th of ≥10 primaries | 5 (0.0) | 0 (0.0) |
15th of ≥15 primaries | 1 (0.0) | 0 (0.0) |
20th of ≥20 primaries | 1 (0.0) | 0 (0.0) |
Unknown seq. number | 13 (0.0) | 0 (0.0) |
CS Site-Specific Factor 1 coding system, please refer to https://staging.seer.cancer.gov/cs/input/02.05.50/lung/ssf1/?version=/tnm/home/1.7/. SD, standard deviation; CS, collaborative staging; IQR, interquartile range; Mets, metastases; dx, diagnosis; NOS, not otherwise specified; Resec., resection; ICD-O-3, ICD-O-3 histology coding system, please refer to https://seer.cancer.gov/icd-o-3/; seq., sequence.
Comparison of DeepSurv and Cox models
The concordance indexes (C-indexes) in the training dataset (SEER full sample) with the DeepSurv and Cox models were 0.792 (0.791–0.792) and 0.714 (0.713–0.715), respectively. The respective values were 0.727 (0.704–0.750) and 0.692 (0.666–0.718) after applying the trained model in the test dataset (GYFY). To better show the difference in the predictive performance of the DeepSurv and Cox models, a variety of comparisons were conducted. The results showed that the all aspects of the predictive performance of the DeepSurv model were better than the Cox model (Table 2).
Table 2
Test scenario* | C-index (95% CI) | |
---|---|---|
AI model | Cox model | |
seer_full_train_out | 0.7917 (0.7909–0.7924) | 0.7141 (0.7132–0.7149) |
seer_full_valid_out | 0.7900 (0.7885–0.7916) | 0.7130 (0.7112–0.7148) |
seer_full_test_out | 0.7267 (0.7039–0.7495) | 0.6920 (0.6659–0.7180) |
seer_match11_train_out | 0.8554 (0.8455–0.8652) | 0.7988 (0.7877–0.8099) |
seer_match11_valid_out | 0.8594 (0.8407–0.8781) | 0.8165 (0.7951–0.8380) |
seer_match11_test_out | 0.6801 (0.6541–0.7061) | 0.6712 (0.6442–0.6982) |
seer_match12_train_out | 0.8678 (0.8611–0.8744) | 0.8054 (0.7975–0.8133) |
seer_match12_valid_out | 0.8417 (0.8261–0.8572) | 0.7973 (0.7810–0.8137) |
seer_match12_test_out | 0.6870 (0.6608–0.7133) | 0.6722 (0.6450–0.6994) |
seer_match13_train_out | 0.8576 (0.8518–0.8634) | 0.8014 (0.7947–0.8081) |
seer_match13_valid_out | 0.8593 (0.8479–0.8707) | 0.8075 (0.7940–0.8211) |
seer_match13_test_out | 0.6770 (0.6510–0.7030) | 0.6662 (0.6382–0.6941) |
seer_match14_train_out | 0.8624 (0.8574–0.8675) | 0.8031 (0.7972–0.8090) |
seer_match14_valid_out | 0.8526 (0.8419–0.8632) | 0.8046 (0.7929–0.8163) |
seer_match14_test_out | 0.6827 (0.6572–0.7083) | 0.6703 (0.6425–0.6981) |
gyfy_match11_train_out | 0.7507 (0.7241–0.7772) | 0.7049 (0.6767–0.7332) |
gyfy_match11_valid_out | 0.7754 (0.7260–0.8248) | 0.7537 (0.7021–0.8054) |
gyfy_match11_test_out | 0.8018 (0.7920–0.8117) | 0.7491 (0.7375–0.7606) |
gyfy_match12_train_out | 0.7594 (0.7315–0.7872 ) | 0.7111 (0.6810–0.7413) |
gyfy_match12_valid_out | 0.7347 (0.6847–0.7847) | 0.7038 (0.6551–0.7524) |
gyfy_match12_test_out | 0.7986 (0.7915–0.8057) | 0.7663 (0.7584–0.7743) |
gyfy_match13_train_out | 0.7553 (0.7279–0.7826) | 0.7094 (0.6793–0.7395) |
gyfy_match13_valid_out | 0.7688 (0.7220–0.8155) | 0.6908 (0.6352–0.7463) |
gyfy_match13_test_out | 0.7960 (0.7900–0.8020) | 0.7653 (0.7588–0.7719) |
gyfy_match14_train_out | 0.7469 (0.7195–0.7742) | 0.7024 (0.6718–0.7330) |
gyfy_match14_valid_out | 0.7483 (0.6899–0.8067) | 0.7219 (0.6699–0.7739) |
gyfy_match14_test_out | 0.7973 (0.7921–0.8025) | 0.7729 (0.7672–0.7786) |
gyfy_full_train_out | 0.7804 (0.7555–0.8053) | 0.7209 (0.6933–0.7485) |
gyfy_full_valid_out | 0.7489 (0.6961–0.8016) | 0.6784 (0.6144–0.7425) |
gyfy_full_test_out | 0.6605 (0.6596–0.6614) | 0.6514 (0.6505–0.6523) |
*, test scenario format: a_b_c_out. “a” is the database used for initial training (SEER or GYFY); “b” is the sample size used (full: full samples, match11: 1:1 matched samples, match12: 1:2 matched samples, match13: 1:3 matched samples, match14: 1:4 matched samples); “c” is the modeling steps in this scenario (train: the initial training step; valid: internal validation using the same database as in the training step; test: external validation using the other database). AI, artificial intelligence; CI, confidence interval; SEER, Surveillance, Epidemiology, and End Results database; GYFY, The First Affiliated Hospital of Guangzhou Medical University database.
For example, we treated the GYFY dataset as a training set and the SEER dataset as a test set. To control the difference in data distribution, SEER and GYFY were matched 1:1, 1:2, 1:3, and 1:4 with the propensity score matching method. Next, model verification and remodel verification were performed. The scenario where we used GYFY as the training set and SEER as the test set with the 1:1 matched data, showed the best performance. In this scenario, the C-indexes in the DeepSurv and Cox model trained with GYFY training dataset were 0.751 (0.724–0.777) and 0.705 (0.677–0.733), respectively. After testing with the SEER dataset; the C-indexes in the DeepSurv and Cox model were 0.802 (0.792–0.812) and 0.749 (0.738–0.761) (Table 2).
Credibility assessment
The DeepSurv model not only provided the predicted relative risk but also output the AI certainty of the relative risk of the patient. The higher the certainty, the more confident the model was in the accuracy of the results. As shown in Figure 2, the certainty of the DeepSurv model output was from 0.98 to 1, and the probability of death of the patient suddenly increased after certainty reached a certain point (0.996). It showed that the certainty was proportional to the risk of death, which meant the model might have higher predictive accuracy for patients with a high risk of death.
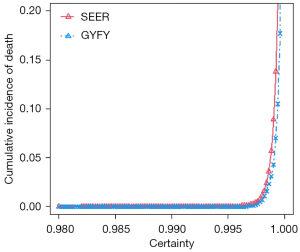
Performance of transfer learning through fine-tuning
We studied the application of transfer learning in survival prediction. The model was firstly pre-trained on source domain data (SEER) until convergence and then the initialized model was further trained on a subset from the target domain data (GYFY). Table 3 shows the transfer learning result: prediction results with/without pre-training, prediction results with/without fine-tuning (on 20% and 30% of target domain data) on target domain data were compared. The results indicated that experiments with pre-training and fine-tuning operations outperformed the other two settings (no pre-training or fine-tuning). With more fine-tuning of the dataset, the test set (80% and 70% of target domain data) could achieve a higher C-index (20% vs. 30% fine-tuning data).
Table 3
Training set | Test set | Pre-trained model | Fine-tuning (20%) | Test (80%), [C-index (95% CI)] | Fine-tuning (30%) | Test (70%), [C-index (95% CI)] |
---|---|---|---|---|---|---|
SEER | GYFY | √ | √ | 0.7300 (0.7039–0.7561) | √ | 0.7387 (0.7119–0.7654) |
√ | × | 0.7191 (0.6923–0.7460) | × | 0.7327 (0.7055–0.7599) | ||
× | √ | 0.7078 (0.6791–0.7365) | √ | 0.7257 (0.6963–0.7552) |
SEER, Surveillance, Epidemiology, and End Results database; GYFY, The First Affiliated Hospital of Guangzhou Medical University database; CI, confidence interval.
AI for missing data
The DeepSurv model was more accurate than the traditional Cox model in predicting with missing data. After random data loss of 5%, 10%, 15%, and 20%, and median fill-in missing values, the Cox prediction performance dropped rapidly, and the predictive performance of the deep learning models was more robust than the Cox model (Table 4).
Table 4
Test scene | C-index (95% CI) | |
---|---|---|
AI model | Cox model | |
seer_train, gyfy out | 0.7267 (0.7039–0.7495) | 0.6920 (0.6659–0.7180) |
seer_train, gyfy 5% missing | 0.7192 (0.6954–0.7430) | 0.6673 (0.6372–0.6975) |
seer_train, gyfy 10% missing | 0.7098 (0.6860–0.7336) | 0.6572 (0.6273–0.6872) |
seer_train, gyfy 15% missing | 0.6956 (0.6714–0.7198) | 0.6219 (0.5896–0.6542) |
seer_train, gyfy 20% missing | 0.6735 (0.6480–0.6990) | 0.6161 (0.5848–0.6474) |
seer_train, gyfy out_median_missing | 0.7178 (0.6945–0.7411) | 0.7032 (0.6780–0.7285) |
gyfy_train, seer out_median_missing | 0.6661 (0.6652–0.6669) | 0.5991 (0.5981–0.6000) |
AI, artificial intelligence; CI, confidence interval; SEER, Surveillance, Epidemiology, and End Results database; GYFY, The First Affiliated Hospital of Guangzhou Medical University database.
Discussion
Compared with the standard Cox model, the model using the DeepSurv algorithm yielded better performance in all scenarios with higher C-indexes (Table 4). DeepSurv is a non-linear deep learning-based model for survival analysis that is more appropriate for revealing real-world situations (14). Moreover, pre-training and fine-tuning improved the C-index of the model, which implied that our deep transfer learning-based model has a furtherly enhanced performance compared to deep learning-based models and the conventional Cox model.
There are two unique features of the model that highlight its superiority to other survival models. One is that it evaluates the accuracy of its prediction simultaneously by outputting the AI certainty using dropout neural networks (NNs) (15). The higher the AI certainty, the more confident the model is in the accuracy of the results. Additionally, as showed in Figure 2, the certainty was proportional to the risk of death, which meant the model might have a higher predictive accuracy for patients with a high risk of death. The second advantage is that the model showed superior performance with both complete and missing data. After random data loss of 5%, 10%, 15%, and 20%, and median fill-in missing values, the predictive performance of the deep model was more stable and accurate, whereas the performance of the standard Cox model rapidly declined in the same scenarios (Table 4). These two features make the survival model superior for practical applications because it is very common for real-world data to be incomplete. Overall, our model proved that the integration of deep learning and transfer learning achieved better performance in survival analysis than the standard Cox model or a model using deep learning only.
The pre-trained model can also be used to create an evaluation tool and a model frame. The evaluation tool can be used for patient self-evaluation to guide lifestyle planning and therapeutic decision-making. Additionally, the evaluation tool can help risk-stratify participants in lung cancer therapeutic clinical trials, whereas the model frame is best for further fine-tuning according to the target population and exploration of other prognostic factors, which will benefit researchers worldwide (Figure 1).
In clinical practice, the evaluation tool enables patients to self-evaluate survival possibilities in a convenient, precise, and individualized way. Given the relatively miserable outcome of lung cancer, decision making is a grinding process for both physicians and patients (1,16). However, patients’ autonomy and participation during the decision-making process are necessary and contribute to improving patient safety and experience in modern patient-centered healthcare, compared with being passive spectators in traditional paternalistic healthcare (17-19). It is noteworthy that health literacy is central to enhancing patients’ participation in their care because low health literacy and lack of knowledge of the subject decrease confidence and willingness to engage in decision-making (18,19). This evaluation tool can assist patients to weigh the pros and cons of a decision and improve their participation in their healthcare. Additionally, it may ease communication difficulties between physicians and patients. With a more precise survival probability provided, physicians can formulate more individualized and stratified treatment strategy and lifestyle planning for patients.
The evaluation tool can also play a vital role in lung cancer clinical trials. Although randomized controlled trials are considered the most reliable scientific evidence to guide clinical practice, the overall results are not always generalizable to individual patients (20,21). Substantial variation in the individual baseline risk within a trial is common because patients have multiple known or unknown characteristics that can affect the outcome, resulting in heterogeneity of the treatment effect between subgroups of patients in trials (21,22). In other words, the lack of a consistent analytic approach to baseline risk evaluation limits the application of summary results of clinical trials to individual patients. Risk stratification of participants was well powered to minimize bias and explore the most beneficial subgroup for the treatment (23). A multivariate model combining risk factors into a score that describes a single dimension of the risk is the key to baseline risk stratification, rather than one-variable-at-a-time subgroup analysis (21,22,24). Therefore, the evaluation tool developed from the pre-trained model has great potential as a standardized tool for baseline risk stratification in lung cancer trials.
Issues to be addressed in traditional model-building include small sample size, unqualified external validation, and waning performance of the model over time due to changes in diagnosis and treatment (25-27). A rational way to solve these issues is to integrate individual participant data from multiple studies and include new prognostic factors to update the model (28,29). However, collaboration between research groups can be problematic in the sharing of data with all details. Our pre-trained model can be exploited to integrate new data and explore new prognostic factors by other researchers as per request.
In terms of sample size, we used the SEER database, the biggest lung cancer database that includes 601,480 patients, as the training cohort to explore the coefficients of prognostic factors. After pre-training, our model could provide fixed coefficients of the 18 variables that were closest to the real coefficients among the global population, which enabled more accurate predictions compared with current models. Furthermore, researchers can integrate their own data and fine-tune the online pre-trained model to a more applicable one using a dataset from their target population. With the fixed coefficients, overfitting can be minimized, even using a relatively small sample size to study other prognostic factors (30). Therefore, we envision that our deep transfer learning-based model for survival prediction in lung cancer will have great utility in research and clinical practice.
However, there are limitations when it comes to a machine learning-based prognostic model. Firstly, machine learning algorithms may be subject to biases, which include those related to missing data and patients not identified by algorithms, sample size and underestimation, and misclassification and measurement error (31). Moreover, the model requires frequent maintenance even though it is superior to the traditional models. The coefficients of the prognostic factors in the pre-trained model can differ over decades due to the evolution of practice since the current model based on SEER data from between 2006 and 2015. Therefore retraining of the pretrained model with new data from the SEER database will be required. New prognostic factors related to targeted therapy or immunotherapy may emerge, correlated with changes in medical practice in the real world, which also requires modification of the pre-trained model with new prognostic factors. The last challenge for future application is the need for a prospective study of the model in the real world rather than only a retrospective assessment based on historical data (32).
Conclusions
Therefore, we present this new approach of machine learning by combining deep learning and transfer learning. The survival model for lung cancer outperformed the traditional Cox model, was robust with missing data and provided the AI certainty of prediction. It can be used for patient self-evaluation and risk stratification in clinical trials. Researchers can fine-tune the pre-trained model and integrate their own database to explore other prognostic factors for lung cancer in the future.
Acknowledgments
We sincerely thank Chaonan Zhu, Lili Wang, Cong Fang, Wei Shen and Qiang Lin from Yitu Tech for their support in AI modeling.
Funding: None.
Footnote
Reporting Checklist: The authors have completed the TRIPOD reporting checklist. Available at https://tlcr.amegroups.com/article/view/10.21037/tlcr-23-84/rc
Data Sharing Statement: Available at https://tlcr.amegroups.com/article/view/10.21037/tlcr-23-84/dss
Peer Review File: Available at https://tlcr.amegroups.com/article/view/10.21037/tlcr-23-84/prf
Conflicts of Interest: All authors have completed the ICMJE uniform disclosure form (available at https://tlcr.amegroups.com/article/view/10.21037/tlcr-23-84/coif). WL serves as an unpaid Associate Editor-in-Chief of the Translational Lung Cancer Research from May 2021 to April 2023. The other authors have no conflicts of interest to declare.
Ethical Statement: The authors are accountable for all aspects of the work in ensuring that questions related to the accuracy or integrity of any part of the work are appropriately investigated and resolved. In this study, only de-identified data were used, so ethical review and informed consent were waived by the institutional review board of The First Affiliated Hospital of Guangzhou Medical University. The study was conducted in accordance with the Declaration of Helsinki (as revised in 2013).
Open Access Statement: This is an Open Access article distributed in accordance with the Creative Commons Attribution-NonCommercial-NoDerivs 4.0 International License (CC BY-NC-ND 4.0), which permits the non-commercial replication and distribution of the article with the strict proviso that no changes or edits are made and the original work is properly cited (including links to both the formal publication through the relevant DOI and the license). See: https://creativecommons.org/licenses/by-nc-nd/4.0/.
References
- Noone AM, Howlader N, Krapcho M, et al. SEER Cancer Statistics Review, 1975–2015, National Cancer Institute. Bethesda, MD, Available online: https://seer.cancer.gov/csr/1975_2015/, based on November 2017 SEER data submission, posted to the SEER web site, April 2018.
- Goldstraw P, Chansky K, Crowley J, et al. The IASLC Lung Cancer Staging Project: Proposals for Revision of the TNM Stage Groupings in the Forthcoming (Eighth) Edition of the TNM Classification for Lung Cancer. J Thorac Oncol 2016;11:39-51. [Crossref] [PubMed]
- Hsu LH, Chu NM, Liu CC, et al. Sex-associated differences in non-small cell lung cancer in the new era: is gender an independent prognostic factor? Lung Cancer 2009;66:262-7. [Crossref] [PubMed]
- Asmis TR, Ding K, Seymour L, et al. Age and comorbidity as independent prognostic factors in the treatment of non small-cell lung cancer: a review of National Cancer Institute of Canada Clinical Trials Group trials. J Clin Oncol 2008;26:54-9. [Crossref] [PubMed]
- Ou SH, Ziogas A, Zell JA. Prognostic factors for survival in extensive stage small cell lung cancer (ED-SCLC): the importance of smoking history, socioeconomic and marital statuses, and ethnicity. J Thorac Oncol 2009;4:37-43. [Crossref] [PubMed]
- Sidey-Gibbons JAM, Sidey-Gibbons CJ. Machine learning in medicine: a practical introduction. BMC Med Res Methodol 2019;19:64. [Crossref] [PubMed]
- Esteva A, Robicquet A, Ramsundar B, et al. A guide to deep learning in healthcare. Nat Med 2019;25:24-9. [Crossref] [PubMed]
- Lee S, Lee HW, Kim HJ, et al. Deep Learning-Based Prediction Model Using Radiography in Nontuberculous Mycobacterial Pulmonary Disease. Chest 2022;162:995-1005. [Crossref] [PubMed]
- Yin M, Lin J, Liu L, et al. Development of a Deep Learning Model for Malignant Small Bowel Tumors Survival: A SEER-Based Study. Diagnostics (Basel) 2022;12:1247. [Crossref] [PubMed]
- Lee JH, Hwang EJ, Kim H, et al. A narrative review of deep learning applications in lung cancer research: from screening to prognostication. Transl Lung Cancer Res 2022;11:1217-29. [Crossref] [PubMed]
- Weiss K, Khoshgoftaar TM, Wang DD. A survey of transfer learning. Journal of Big Data 2016;3:9. [Crossref]
- Bergquist SL, Brooks GA, Keating NL, et al. Classifying Lung Cancer Severity with Ensemble Machine Learning in Health Care Claims Data. Proc Mach Learn Res 2017;68:25-38. [PubMed]
- Corey KM, Kashyap S, Lorenzi E, et al. Development and validation of machine learning models to identify high-risk surgical patients using automatically curated electronic health record data (Pythia): A retrospective, single-site study. PLoS Med 2018;15:e1002701. [Crossref] [PubMed]
- Katzman JL, Shaham U, Cloninger A, et al. DeepSurv: personalized treatment recommender system using a Cox proportional hazards deep neural network. BMC Med Res Methodol 2018;18:24. [Crossref] [PubMed]
- Gal Y, Ghahramani Z. Dropout as a bayesian approximation: Representing model uncertainty in deep learning. International Conference on Machine Learning, 2016:1050-9.
- Mitchell KR, Brassil KJ, Rodriguez SA, et al. Operationalizing patient-centered cancer care: A systematic review and synthesis of the qualitative literature on cancer patients' needs, values, and preferences. Psychooncology 2020;29:1723-33. [Crossref] [PubMed]
- Emanuel EJ, Emanuel LL. Four models of the physician-patient relationship. JAMA 1992;267:2221-6. [Crossref] [PubMed]
- Longtin Y, Sax H, Leape LL, et al. Patient participation: current knowledge and applicability to patient safety. Mayo Clin Proc 2010;85:53-62. [Crossref] [PubMed]
- Coulter A, Ellins J. Effectiveness of strategies for informing, educating, and involving patients. BMJ 2007;335:24-7. [Crossref] [PubMed]
- Rothwell PM. Can overall results of clinical trials be applied to all patients? Lancet 1995;345:1616-9. [Crossref] [PubMed]
- Kent DM, Hayward RA. Limitations of applying summary results of clinical trials to individual patients: the need for risk stratification. JAMA 2007;298:1209-12. [Crossref] [PubMed]
- Rothwell PM. Treating individuals 2. Subgroup analysis in randomised controlled trials: importance, indications, and interpretation. Lancet 2005;365:176-86. [Crossref] [PubMed]
- Hingorani AD, Windt DA, Riley RD, et al. Prognosis research strategy (PROGRESS) 4: stratified medicine research. BMJ 2013;346:e5793. [Crossref] [PubMed]
- Hayward RA, Kent DM, Vijan S, et al. Multivariable risk prediction can greatly enhance the statistical power of clinical trial subgroup analysis. BMC Med Res Methodol 2006;6:18. [Crossref] [PubMed]
- Mallett S, Royston P, Waters R, et al. Reporting performance of prognostic models in cancer: a review. BMC Med 2010;8:21. [Crossref] [PubMed]
- Vergouwe Y, Steyerberg EW, Eijkemans MJ, et al. Substantial effective sample sizes were required for external validation studies of predictive logistic regression models. J Clin Epidemiol 2005;58:475-83. [Crossref] [PubMed]
- Moons KG, Altman DG, Vergouwe Y, et al. Prognosis and prognostic research: application and impact of prognostic models in clinical practice. BMJ 2009;338:b606. [Crossref] [PubMed]
- Riley RD, Lambert PC, Abo-Zaid G. Meta-analysis of individual participant data: rationale, conduct, and reporting. BMJ 2010;340:c221. [Crossref] [PubMed]
- Steyerberg EW, Moons KG, van der Windt DA, et al. Prognosis Research Strategy (PROGRESS) 3: prognostic model research. PLoS Med 2013;10:e1001381. [Crossref] [PubMed]
- Babyak MA. What you see may not be what you get: a brief, nontechnical introduction to overfitting in regression-type models. Psychosom Med 2004;66:411-21. [PubMed]
- Gianfrancesco MA, Tamang S, Yazdany J, et al. Potential Biases in Machine Learning Algorithms Using Electronic Health Record Data. JAMA Intern Med 2018;178:1544-7. [Crossref] [PubMed]
- Rajkomar A, Dean J, Kohane I. Machine Learning in Medicine. N Engl J Med 2019;380:1347-58. [Crossref] [PubMed]
(English Language Editor: K. Brown)