Establishing a predictive model for tumor mutation burden status based on CT radiomics and clinical features of non-small cell lung cancer patients
Highlight box
Key findings
• The efficacy of the prediction model based on radiomics features was significantly better than that of the clinical model (AUC: 0.822 vs. 0.683). The nomogram, established by combining smoking history, pathologic type, and rad-score, showed the best diagnostic efficacy (AUC =0.844) and performed well in distinguishing the status of TMB-high and TMB-low.
What is known and what is new?
• TMB is a biomarker for ICIs efficacy. Unfortunately, invasive procedures during specimen acquisition and the high cost of whole exome sequencing hinder the dynamic monitoring of TMB status.
• The radiomics model based on CT is a non-invasive, affordable, rapid and can eliminate the impact of tumor heterogeneity detection method, so it is more practical in clinical practice.
What is the implication, and what should change now?
• Radiomics is a new method to detect TMB status. The nomogram could provide additional information on the treatment opportunity and plan of immunotherapy.
Introduction
In recent years, immune checkpoint inhibitors (ICIs) represented by monoclonal antibodies of programmed cell death protein-1 (PD-1), programmed cell death protein ligand-1 (PD-L1), and cytotoxic T lymphocyte antigen (CTLA-4) have been put into clinical application, which have completely changed the treatment landscape of lung cancer (1-4). The significant survival benefit of ICIs makes them the first-line treatment option for advanced or locally advanced non-small cell lung cancer (NSCLC) and extensive small cell lung cancer (5-7).
However, only some NSCLC patients can benefit from ICI treatment (8-12), so it is crucial to screen out target patients for immunotherapy. Tumor mutation burden (TMB) refers to the total number of non-synonymous mutations per megabase (Mb) in the gene exon coding region of the evaluated tumor cell (13-16). Clinical studies have shown that patients with high TMB (TMB-H) can achieve better survival benefits regardless of whether PD-1/PD-L1 inhibitors are applied in first-line or after-line therapy (7,17,18), thus TMB is considered a crucial predictive biomarker of efficacy in ICIs therapy (17,19-21). TMB plays a significant role in ICI treatment of NSCLC, and accumulated evidence supports its clinical predictive value for ICI efficacy and patients’ prognosis, including the objective response rate (ORR), disease control rate (DCR), progression-free survival (PFS), and overall survival (OS) (10,15,16,22,23). Expert Consensus on Lung Cancer Immunotherapy recommends that TMB detection should be conducted for advanced NSCLC patients without prior immunotherapy history before receiving ICI monotherapy (17,24). The Food and Drug Authority (FDA) has also approved the use of pembrolizumab in solid tumors of TMB-H (20,25).
The TMB status of tumors is heterogeneous; for example, chemotherapy may lead to the conversion of low TMB (TMB-L) to TMB-H in some lung cancer patients (8,10,26). Therefore, accurate and rapid detection of TMB status is beneficial for doctors to determine the right patient and appropriate time to use immunotherapy in NSCLC (15). Next generation sequencing (NGS), a high-throughput gene sequencing method that can simultaneously and rapidly detect multiple tumor mutations (11,18), is the current gold standard for TMB detection. Unfortunately, invasive procedures during specimen acquisition and the high cost of whole exome sequencing (WES) hinder the widespread use of TMB detection and the dynamic monitoring of tumor TMB status (27,28). In clinical practice, a simple and noninvasive detection method with short detection cycle, favorable price, and low requirements for detection specimens is urgently needed, so that TMB can be widely used as a biomarker in ICI therapy, creating conditions for further development of TMB in clinical and scientific research.
Radiomics is a concept that was proposed by Philippe Lambin in 2012, which refers to the process of automatic and high-throughput extraction of characteristic information in medical images and transformation of it into deep quantitative data (29,30). The workflow includes image acquisition, reconstruction, and standardization, region of interest (ROI) segmentation, extraction of radiomic features, establishment and verification of radiomic models, and so on (29,31). ROI segmentation is a key step in radiomics analysis. Due to the high contrast resolution between lung tumors and lung parenchyma (32,33), tumors can be easily distinguished from adjacent lung tissue and isolated as ROI, thus radiomics is considered a suitable tool in the field of lung cancer research (30,34). Recently, radiomics has shown promising advantages in the diagnosis, differentiation, determination of invasiveness, evaluation of efficacy, and even prediction of gene mutation status of lung cancer (30,34-37). Some studies have attempted to predict pathological subtypes of lung adenocarcinoma (LUAD) with different gene mutation states based on radiomics, and the resulting area under the curve (AUC) of the models ranged from 0.62 to 0.89 (30). Researcher found that EGFR mutation state could be distinguished by a radiomics model with an AUC of 0.75 (38). These studies demonstrate the potential of radiomic signatures to identify microscopic genetic and molecular differences (30,34,38,39).
To date, no studies have explored the intrinsic correlation between computed tomography (CT) radiomic features and TMB status in NSCLC patients, and the diagnostic value of radiomic features in the peritumor area has not been fully developed. Therefore, this study combined the clinical characteristics of NSCLC patients and the radiomic features related to the tumor primary lesions to construct a nomogram to distinguish the TMB-H and TMB-L status of the tumor. The purpose of this study was to explore a non-invasive TMB detection method with short detection cycle, favorable price, understandable results, and less interference from tumor heterogeneity, in order to be widely promoted, so as to benefit more patients. We present the following article in accordance with the TRIPOD reporting checklist (available at https://tlcr.amegroups.com/article/view/10.21037/tlcr-23-171/rc).
Methods
Study cohort
NSCLC patients who had been pathologically diagnosed in the First Medical Center of the Chinese People’s Liberation Army General Hospital between 30 November 2016 and 1 January 2021 were retrospectively included, and their clinical data and CT imaging data were collected. Patients were grouped according to the NGS detection results of tissue TMB, and the TMB ≥10/Mb was set as the TMB-H group; TMB <10/Mb was set as the TMB-L group. All patients were randomly divided into a training set (n=132) and a validation set (n=57) in a ratio of 7:3.
The inclusion criteria were as follows: (I) the histopathological diagnosis of primary lung lesions were NSCLC; (II) NGS detection of tumor tissue TMB was performed, and confirmed TMB detection results were obtained; (III) the completion time of thin-slice chest CT should be within 30 days before tissue sampling, and the thickness of CT slice should not exceed 1.5 mm; (IV) complete clinical data and CT image data. The exclusion criteria were as follows: (I) pathological diagnosis was thoracic malignant tumors or benign lesions other than NSCLC; (II) NGS detection specimens were not primary lung lesions; (III) the completion time of CT exceeded 30 days before histopathological examination or the thickness of CT layer exceeded 1.5 mm; (IV) incomplete clinical, pathological, or NGS detection data.
Through consulting medical records, the following 14 clinical features of patients were collected: sex, age, smoking history (light smokers refer to smoking index <500, heavy smokers refer to smoking index ≥500), allergy history, history of surgical anesthesia, family lung cancer history, family malignant tumor history, pathological type (adenocarcinoma, squamous cell carcinoma), pathological stage, differentiation degree (low, medium, high), lymphatic metastasis, multiple primary lung cancer, lesion location/location of lung lobe (upper right, middle right, lower right, upper left, lower left), and multifocal lesion. The study was conducted in accordance with the Declaration of Helsinki (as revised in 2013). This study was approved by the Ethics Committee of the First Medical Center of Chinese People’s Liberation Army General Hospital (No. S2020-173-01), and the requirement for informed consent of the patients was waived.
TMB detection
The NGS method was used to detect the TMB status, which was realized by the illumina sequencing platform provided by Nanjing Shihe Gene Biotechnology Co. Ltd. (Nanjing, China). The test specimens were tumor tissues from surgical resection or primary puncture biopsy of patients, paraffin-embedded tumor tissue samples were used, and whole blood samples were used as normal control [2–5 mL ethylenediaminetetraacetic acid (EDTA) anticoagulant peripheral blood, stored and transported at room temperature with special sampling vessel for free DNA]. The test specimens were examined by third-party detection institution unrelated to researchers. The specific detection methods and parameters were as follows: the panel probe capture method was used to detect the site combination of TMB. All non-synonymous mutations and synonymous mutations were included in the combination, and the high-frequency mutation sites associated with tumorigenesis and development in the Chinese population were excluded. The coding base covered by targeted sequencing panel was 1.44 Mb, and the minimum effective sequencing depth was ≥1,000×.
CT scanning protocols
CT images were performed by either of the following CT scanners: Brilliance iCT (Philips Medical Systems, Amsterdam, Netherlands; collimation 0.625 mm × 128; layer thickness 1 mm; layer interval 1 mm; reconstruction kernel iDose3), Somatom Definition (Siemens Medical Systems, Erlangen, Germany; collimation 0.75 mm × 128; layer thickness 1.25 mm; layer interval 1.25 mm; reconstruction kernel B70f).
Image segmentation, radiomic feature extraction, and inter-observer consistency assessment
Both image segmentation and radiomic feature extraction processes were implemented by 3D Slicer software (version 4.10.2; https://download.slicer.org/). The ROI in this study was set for the area inside the lung tumor (i.e., the intra-tumoral area) and 5 mm adjacent to the tumor (i.e., the peritumoral area, which was confined to the lung tissue). As shown in Figure 1, CT images of the patient (DICOM format) were first introduced, and then a physician outlined layer by layer along the edge of the tumor lesion to complete the segmentation of the intra-tumoral area. The segmentation file was saved as Mask1 (green area in Figure 1A), and a 3D model of the lesion was constructed (Figure 1C). Next, the “Hollow” tool and the “inside surface” option were used to obtain the 5 mm peri-tumor area around the tumor, and the segmentation file was saved as Mask2 (green area in Figure 1B). In addition, the voxel points in the intra-tumoral region (Mask1) and peritumoral region (Mask2) were resampled into a 1 mm × 1 mm × 1 mm matrix to eliminate the interference of patient size, reconstruction methods, CT thickness, and other factors. Finally, the “SlicerRadiomics” extension was used to calculate and extract 1,223 radiomics features, including the following five categories: (I) shape features; (II) first-order features; (III) texture features; (IV) wavelet features; (V) Laplacian of Gaussian (LoG) features. Besides, Z-score method was used to standardize the radiomic features.
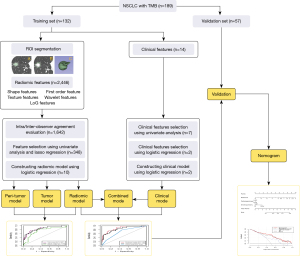
Inter-observer agreement, the influence of different physicians on the robustness of radiomic features, was assessed by calculating interclass correlation coefficients (ICC). ROI segmentation was performed on 30 randomly selected lung CT images by two senior physicians (10 and 20 years of professional experience, respectively) who did not have access to patient information and research content, and intra-tumoral and peritumoral radiomic features were obtained. ICCs for each radiomic feature and mean ICC for all radiomic features were calculated. Features with ICC ≥0.75 were considered to have good inter-observer consistency, whereas features with ICC <0.75 were filtered due to lack of consistency.
Clinical feature screening and model construction
Univariate analysis was used to select clinical features related to TMB status, and multivariate analysis was used to further select clinical features that were independent predictors of TMB. The clinical model was constructed by using the selected clinical features based on logistic regression method.
Radiomic feature screening, model construction, and model evaluation
All patients were randomly divided into a training set and a validation set (7:3), and the proportion of patients with TMB-H and TMB-L remained at the same level in both sets. In the training set, the univariate analysis and the least absolute shrinkage and selection operator (LASSO) were used to screen out the significant radiomics features, and the LASSO parameter (λ) was determined by a 10-fold cross-validation method. Then, the selected radiomics features were used to construct radiomics models in the training set and validation set based on logistic regression method, and the AUC of receiver operating characteristic (ROC) curve was used to evaluate the diagnostic performance of the models. We established 3 radiomic models based on the radiomic features of intra-tumoral region, peritumoral region, and overall region, and their diagnostic performance was compared.
Construction and evaluation of nomogram
Radiomics score (rad-score) was calculated according to the radiomics model. Selected clinical features and rad-score were taken as independent risk factors of TMB status, and the nomogram was constructed with those factors based on multivariate logistic regression model. The diagnostic validity of the nomogram was evaluated by drawing a calibration curve. In addition, decision curve analysis (DCA) was used to evaluate the clinical efficacy of nomogram by calculating the net benefit under different threshold probabilities.
Statistical analysis
The software SPSS 26.0 (IBM Corp., Armonk, NY, USA) was used for statistical analysis of clinical features. Mann-Whitney U test was used for univariate analysis of continuous variables and ordered categorical variables, and chi-square test or Fisher’s exact test was used for univariate analysis of disordered categorical variables. Multivariate analysis was performed by binary logistic regression. Variables with a P<0.05 were considered statistically significant and P<0.05 is two-sided. R software (version 4.0.3; The R Foundation for Statistical Computing, Vienna, Austria) was used for random grouping, screening of radiomic features, construction, and evaluation of models and nomogram. R packages including glmnet, pROC, e1071, hmisc, lattice, survival, formula, ggplot2, rms, and rmda were used in the above process. Radiomics features with ICC ≥0.75 were considered robust. Delong test was used to compare the AUC of the two ROC curves.
Results
Baseline characteristics of patients
A total of 189 patients were included in this study, and the overall clinical features of the patients are shown in Table 1. Patients were grouped according to the NGS detection results of TMB, including 46 cases in the TMB-H group and 143 cases in the TMB-L group.
Table 1
Clinical features | Total (N=189) | Training set (n=132) | Validation set (n=57) | ||||||||
---|---|---|---|---|---|---|---|---|---|---|---|
TMB-L group | TMB-H group | P value | TMB-L group | TMB-H group | P value | TMB-L group | TMB-H group | P value | |||
Gender | 0.025 | 0.159 | 0.053 | ||||||||
Male | 54 (37.8) | 26 (56.5) | 39 (39.0) | 17 (53.1) | 15 (34.9) | 9 (64.3) | |||||
Female | 89 (62.2) | 20 (43.5) | 61 (61.0) | 15 (46.9) | 28 (65.1) | 5 (35.7) | |||||
Age (years), mean ± SD | 54.8±10.5 | 60.9±9.7 | 0.001 | 54.4±10.9 | 61.5±10.0 | 0.002 | 55.6±9.5 | 59.6±9.1 | 0.220 | ||
Smoking history* | 0.001 | 0.012 | 0.030 | ||||||||
No | 110 (76.9) | 25 (54.3) | 76 (76.0) | 18 (56.3) | 34 (79.1) | 7 (50.0) | |||||
Light | 18 (12.6) | 5 (10.9) | 14 (14.0) | 3 (9.4) | 4 (9.3) | 2 (14.3) | |||||
Heavy | 15 (10.5) | 16 (34.8) | 10 (10.0) | 11 (34.4) | 5 (11.6) | 5 (35.7) | |||||
Allergic history | 1.000 | 0.555 | 0.057 | ||||||||
Yes | 12 (8.4) | 4 (8.7) | 12 (12.0) | 2 (6.2) | 0 (0.0) | 2 (14.3) | |||||
No | 131 (91.6) | 42 (91.3) | 88 (88.0) | 30 (93.8) | 43 (100.0) | 12 (85.7) | |||||
History of surgical anesthesia | 0.998 | 0.758 | 0.965 | ||||||||
Yes | 28 (19.6) | 9 (19.6) | 18 (18.0) | 5 (15.6) | 10 (23.3) | 4 (28.6 | |||||
No | 115 (80.4) | 37 (80.4) | 82 (82.0) | 27 (84.4) | 33 (76.7) | 10 (71.4) | |||||
Family lung cancer history | 0.166 | 0.580 | 0.448 | ||||||||
Yes | 19 (13.3) | 10 (21.7) | 10 (10.0) | 5 (15.6) | 9 (20.9) | 5 (35.7) | |||||
No | 124 (86.7) | 36 (78.3) | 90 (90.0) | 27 (84.4) | 34 (79.1) | 9 (64.3) | |||||
Family malignant tumor history | 0.256 | 0.718 | 0.231 | ||||||||
Yes | 46 (32.2) | 19 (41.3) | 34 (34.0) | 12 (37.5) | 12 (27.9) | 7 (50.0) | |||||
No | 97 (67.8) | 27 (58.7) | 66 (66.0) | 20 (62.5) | 31 (72.1) | 7 (50.0) | |||||
Pathological type | <0.001 | 0.018 | <0.001 | ||||||||
Lung adenocarcinoma | 139 (97.2) | 34 (73.9) | 96 (96.0) | 26 (81.3) | 43 (100.0) | 8 (57.1) | |||||
Squamous carcinoma | 4 (2.8) | 12 (26.1) | 4 (4.0) | 6 (18.7) | 0 (0.0) | 6 (42.9) | |||||
Pathological stage | 0.014 | 0.076 | 0.087 | ||||||||
I | 89 (62.2) | 21 (45.7) | 64 (64.0) | 16 (50.0) | 25 (58.1) | 5 (35.7) | |||||
II | 22 (15.4) | 4 (8.7) | 17 (17.0) | 4 (12.5) | 5 (11.6) | 0 (0.0) | |||||
III | 18 (12.6) | 12 (26.1) | 12 (12.0) | 7 (21.9) | 6 (14.0) | 5 (35.7) | |||||
IV | 14 (9.8) | 9 (19.6) | 7 (7.0) | 5 (15.6) | 7 (16.3) | 4 (28.6) | |||||
Differentiated degree* | 0.026 | 0.206 | 0.033 | ||||||||
Low | 33 (23.1) | 20 (43.5) | 24 (24.0) | 14 (43.8) | 9 (20.9) | 6 (42.9) | |||||
Medium | 59 (41.3) | 14 (30.4) | 44 (44.0) | 8 (25.0) | 15 (34.9) | 6 (42.9) | |||||
High | 51 (35.6) | 12 (26.1) | 32 (32.0) | 10 (31.2) | 19 (44.2) | 2 (14.3) | |||||
Lymphatic metastasis | 0.001 | 0.032 | 0.021 | ||||||||
Yes | 30 (21.0) | 21 (45.7) | 19 (19.0) | 12 (37.5) | 11 (25.6) | 9 (64.3) | |||||
No | 113 (79.0) | 25 (54.3) | 81 (81.0) | 20 (62.5) | 32 (74.4) | 5 (35.7) | |||||
Multiple primary lung cancer | 0.368 | 0.261 | 1.000 | ||||||||
Yes | 13 (9.1) | 7 (15.2) | 7 (7.0) | 5 (15.6) | 6 (14.0) | 2 (14.3) | |||||
No | 130 (90.9) | 39 (84.8) | 93 (93.0) | 27 (84.4) | 37 (86.0) | 12 (85.7) | |||||
Lesion location | 0.886 | 0.847 | 0.895 | ||||||||
RU | 50 (35.0) | 16 (34.8) | 38 (38.0) | 11 (34.4) | 12 (27.9) | 5 (35.7) | |||||
RM | 9 (6.3) | 2 (4.3) | 5 (5.0) | 2 (6.3) | 4 (9.3) | 0 (0.0) | |||||
RL | 28 (19.6) | 12 (26.1) | 22 (22.0) | 10 (31.3) | 6 (14.0) | 2 (14.3) | |||||
LU | 33 (23.1) | 10 (21.7) | 20 (20.0) | 5 (15.6) | 13 (30.2) | 5 (35.7) | |||||
LL | 23 (16.1) | 6 (13.0) | 15 (15.0) | 4 (12.5) | 8 (18.6) | 2 (14.3) | |||||
Multifocal lesion | 0.425 | 0.496 | 0.015 | ||||||||
Yes | 56 (39.2) | 15 (32.6) | 34 (34.0) | 13 (40.6) | 22 (51.2) | 2 (14.3) | |||||
No | 87 (60.8) | 31 (67.4) | 66 (66.0) | 19 (59.4) | 21 (48.8) | 12 (85.7) |
The data are expressed as n (%). Smoking history*: no, no smoking; light, smoking index <500; heavy, smoking index ≥500. Differentiated degree*: Low, poorly differentiated adenocarcinoma or squamous cell carcinoma; Medium, moderately differentiated adenocarcinoma or squamous cell carcinoma; High, high differentiation adenocarcinoma or squamous cell carcinoma. TMB-L, low tumor mutation burden; TMB-H, high tumor mutation burden; RU, upper right lung, RM, middle right lung; RL, lower right lung; LU, upper left lung; LL, lower left lung.
Clinical features, predictive model and evaluation of model
In the whole data set, univariate analysis showed that there were statistically significant differences (P<0.05) between the TMB-H group and the TMB-L group in gender, age, smoking history, pathological type, pathological stage, differentiation degree, and lymphatic metastasis. Compared with the TMB-L group, the average age of patients in the TMB-H group was older, the proportion of males and heavy smokers was higher, the tumor types were mostly adenocarcinoma, and the tumors with advanced stage, low differentiation, and lymph node metastasis accounted for a higher proportion. Other clinical features, including allergic history, history of surgical anesthesia, family lung cancer history, family malignant tumor history, multiple primary lung cancer, lesion location, and multifocal lesions, were not significantly different between the two groups. The above 7 clinical features with P<0.05 were included in the multivariate analysis, and the results showed that smoking history and pathological type were independent risk factors for TMB status, with odds ratio (OR) values and 95% confidence intervals (CIs) of 1.884 (1.215, 2.921) and 10.231 (2.993, 34.972), respectively (Table S1). Using the best threshold points (0.338), in the training set, the calculated accuracy of the model was 0.788, sensitivity is 0.469, specificity is 0.89; in the validation set, the accuracy is 0.852, sensitivity is 0.643, specificity is 0.884. The two clinical features of smoking history and pathological type were used to establish a logistic regression model, and the AUC in the training set and the validation set was 0.683 and 0.803, respectively.
Robustness of radiomic features
A total of 2,446 radiomic features were extracted from 30 randomly selected CT images in both intra-tumoral and peritumoral regions. The ICC of these features was calculated for observer consistency analysis to evaluate the robustness of the features. The results showed that the ICC of 1,642 radiomic features was higher than 0.75, including 888 intra-tumoral features and 754 peritumoral features. These features had good robustness and could be used in the next screening process. The ICC of the remaining 804 features was lower than 0.75, which was not robust and was deleted.
Radiomics models and evaluation
The 189 patients were randomly assigned to a training set (n=132) or a validation set (n=57). In the training set, 1,642 radiomic features were included in univariate analysis by observer consistency analysis, and 348 features were significantly different between the TMB-H and TMB-L groups (P<0.05), including 187 intra-tumoral features and 161 peritumoral features. LASSO was applied to further feature screening: in the screening of intra-tumoral features, 10-fold cross-validation showed that the model was optimal when λ=0.046 and log λ=−3.086 (the first dotted line on the left of Figure 2A). At this time, 9 radiomic features were selected. In the screening of peritumoral features, the model was optimal when λ=0.069 and log λ=−2.670 (the first dotted line on the left of Figure 2B), and 1 radiomic feature was included. A total of 10 radiomics features significantly correlated with TMB-H were screened (Table 2).
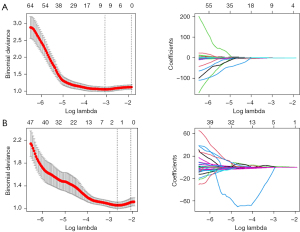
Table 2
Number | Radiomic feature |
---|---|
X1 | Inner-log-sigma-0-5-mm-3D glcm MCC |
X2 | Inner-log-sigma-0-5-mm-3D first order Mean |
X3 | Inner-log-sigma-1-0-mm-3D first order 90Percentile90 |
X4 | Inner-log-sigma-1-0-mm-3D first order Minimum |
X5 | Inner-log-sigma-2-0-mm-3D first order Energy |
X6 | Inner-log-sigma-2-0-mm-3D first order Total Energy |
X7 | Inner-wavelet-LLL gldm Dependence Variance |
X8 | Inner-wavelet-LLL glcm Joint Entropy |
X9 | Inner-Original first order Root Mean Squared |
X10 | Outer-sigma-2-0-mm-3D gldm Small Dependence Emphasis |
LASSO, least absolute shrinkage and selection operator; MCC, maximum correlation coefficient.
In order to explore the diagnostic value of intra-tumoral and peritumoral features respectively, an intra-tumoral model, a peritumoral model, and a combined radiomics model were established based on the above screened features (Figure 3). In the validation set, the AUC of the intra-tumoral model and the peritumoral model was 0.816 and 0.728, the accuracy was 0.773 and 0.632, sensitivity is 0.786 and 0.857, specificity is 0.767 and 0.558, respectively, and the AUC of the combined radiomics model was 0.819, the accuracy was 0.754, sensitivity is 0.786, specificity is 0.744. These results indicated that peritumoral features could also be a predictor of TMB status of NSCLC, but the prediction ability of the intra-tumoral model was better than that of peritumoral model, and the combined radiomics model had the best efficacy. Therefore, the combined radiomics model was used to construct the nomogram.
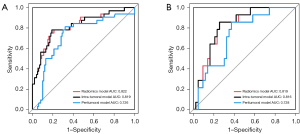
Establishment of combined model and comparison of different models
The rad-score was calculated according to the radiomics model, and the average rad-score of TMB-H group was significantly higher than that of the TMB-L group both in the training set (−0.166±1.547 vs. −1.937±1.088, P<0.001) and the validation set (−0.514±1.035 vs. −1.938±1.223, P<0.001). Smoking history, pathological type, and rad-score were used to establish a combined clinical-radiomics model. Using the best threshold points (0.243), in the training set, the calculated accuracy of the model was 0.754, sensitivity is 0.643, specificity is 0.791; in the validation set, the accuracy is 0.811, sensitivity is 0.812, specificity is 0.82. The AUC of this model in the training set was 0.844 (Figure 4A) and that in the validation set was 0.841 (Figure 4B), both of which were significantly higher than that of the radiomics model (training set AUC =0.822, validation set AUC =0.819) and clinical model (training set AUC =0.683, validation set AUC =0.803) (P<0.001). Also, the radiomics model had a higher AUC than the clinical model.
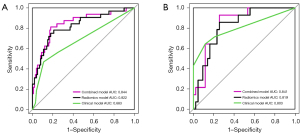
The establishment and evaluation of nomogram
Parameters in the combined clinical-radiomics model were used to establish a visual nomogram model, which could specifically determine the probability of NSCLC being TMB-H based on the smoking history of the patient, the pathological type of the tumor, and the rad-score of CT images (Figure 5). The specific calculation process of probability was as follows: according to the patient’s smoking history grade, the pathological type of NSCLC, and the specific value of the rad-score, the score of each parameter was obtained by upward comparison in the points column. The scores for each parameter were then added together to obtain a total point and a downward comparison to obtain the final probability of whether the lung cancer was a TMB-H state.
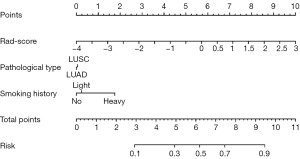
Figure 6 displays the calibration curve of nomogram. The prediction curve and the diagonal are consistent, indicating that the prediction probability of nomogram was close to the actual probability and had an excellent fitting.
In this study, DCA was used to evaluate the clinical application value of the nomogram, radiomics model, and clinical model established above. Figure 7 shows that the application of nomogram and radiomics model to predict TMB-H status had more net benefit than the clinical model over the 20–90% threshold probability range, and the nomogram had more benefit than the radiomics model for most threshold probabilities.
Discussion
Although ICIs have transformed treatment regimens for a variety of cancers (4,18,19,40), including NSCLC, not all patients respond to immunotherapy in the same way (10,26,41-45). Therefore, the identification of biomarkers that predict ICI efficacy will benefit to improve the prognosis of NSCLC patients (16,40).
In clinical practice, PD-L1 expression is one of the biomarkers for selecting the target patients for ICI treatment (21,22,42). However, different studies have shown that PD-L1 expression does not always have a good predictive effect due to its own limitations and differences in immunohistochemical analysis methods (13,15,38,46). For example, in a study cohort without driver gene mutations, the response to ICIs was also considerable in patients with PD-L1 <1% (4,47). Therefore, better identification of biomarkers of sensitivity and resistance to immunotherapy is fundamental to diagnosis and treatment for NSCLC patients. With the development of sequencing technology, it has been found that non-synonymous mutations can generate neoantigens that trigger cytotoxic responses to tumors (17,18,48), which have proven to be more closely related to the clinical advantages of ICI therapy (17,20). The TMB is the total number of non-synonymous mutations per DNA Mb (4,17,47). Many previous studies have shown that TMB was a predictive biomarker of ICI response (9,40). Pembrolizumab has been approved by the FDA for the treatment of solid tumors with TMB-H (≥10 mutation/Mb), and it is expected that more patients will receive ICIs after TMB screening.
Currently, NGS is the mainstream method to detect TMB (18,28), which can be used for quantitative analysis of TMB across multiple tumors, so as to select the beneficiaries of ICI treatment (49,50). However, NGS detection still has several limitations (28,46,51). First, TMB analysis is technically difficult, requiring a professional team to operate, and complex data results need to be interpreted by bioinformatics experts. Second, NGS testing is lengthy and expensive, so it has not been included in China’s social medical insurance. Third, NGS detection requires invasive biopsy or surgical excision to obtain specimens, and the paraffin-embedded tissue samples used for testing have DNA/RNA degradation problems. In addition, the heterogeneity of the tumor will also lead to the difference in the distribution of TMB (41). Therefore, small biopsy specimens from local lesions are often insufficient to evaluate the overall TMB of the tumor, resulting in the bias of the detection results. The above biological, methodological, and economic problems reduce the sensitivity of current TMB detection methods to ICI efficacy prediction and the acceptance of patients (18,28). A new TMB detection method that can overcome the above shortcomings is needed clinically.
Radiomics is a research method combining imaging, artificial intelligence, and big data, which has a wide range of applications (29,52). At present, quantitative analysis based on CT images has been involved in the diagnosis, efficacy evaluation, and prognosis of lung diseases (26,39). Different from traditional imaging, radiomics can extract thousands of features and contains a huge amount of information, which can reflect the lesion image information at multiple levels and from multiple angles. Also, radiomics can extract a large number of subtle features in medical images that cannot be detected by the naked eye, so as to find the changes in the lesion area more keenly (32,35). Based on the above 2 points, this study aimed to apply radiomics to TMB detection, in order to present a complete panorama of the tumor TMB status, so that the detection results will no longer be affected by the surgical resection site or puncture biopsy site, and avoid the bias of the detection results caused by tumor heterogeneity. Furthermore, as a non-invasive detection method, the radiomics model can quickly determine the TMB status of lung cancer patients only with CT images, which can completely overcome the disadvantages of invasive biopsy, specimen DNA/RNA degradation, and long detection cycle of NGS (41,50). Moreover, the model established by radiomics parameters is not affected by doctors’ own technical level and subjective factors, so it can draw more objective and accurate diagnostic conclusions. In view of the simple detection process and intuitive detection results, patients have a high acceptance of radiomics.
This study was designed to analyze the correlation between radiomics features and the TMB of NSCLC, and to evaluate the clinical value of radiomics models for the determination of TMB-H status. A total of 189 NSCLC patients were included, and 14 clinical features and 2,446 intra-tumoral/peritumoral radiomic features were extracted. Through the process of feature screening and model construction, a nomogram based on radiomics features and clinical features was established. It was found that the AUC of the tumor radiomics model and the nomogram was close to each other, which indicated that both had good diagnostic ability of TMB-H and clinical application value.
A few clinical features have been found to be associated with the TMB status of lung cancer (41,51). Sharpnack et al. (41) observed a weak positive correlation between TMB and smoking history in the LUAD group (P=0.20), yet no correlation in lung squamous cell carcinoma (LUSC) group (P=0.026), suggesting that never smoking was a predictor of TMB-L status. Zhang et al. (53) revealed that a TMB-H state was more common in LUSC (P<0.017) and tumors with TP53 mutations (P<0.0001). Another study involving 499 formalin-fixed paraffin-embedded lung cancer excised specimens showed a significant association between TMB status and clinicopathologic features, including pathological subtype (P<0.001), histological subtype (P<0.001), parabronchial lymph node (P<0.001), lymph node metastasis (P=0.009), and so on. In the TMB-H group, there were more males, LUSC, and invasive patients (72.4%), whereas in the TMB-L group, most patients had negative parabronchial lymph node (94.5%) and no lymph node metastasis (88%) (48).
Our study included several clinical and pathological features of NSCLC patients, and finally found that patients’ smoking history and tumor pathological type could be used as predictors of TMB status, and heavy smokers and LUSC accounted for a higher proportion in the TMB-H group. The AUC of the prediction model established by the above two clinical features in the training set (AUC =0.683) and the validation set (AUC =0.803) was lower than that in the radiomics model, and the difference in predictive efficacy between the training set and the validation set was significant, suggesting that the clinical model was unstable. Therefore, it is risky for clinical features to independently predict the TMB status, which are not enough to be biomarkers to predict the ICI efficacy. Next, we explored the ability of radiomics to distinguish between TMB-H or TMB-L status of NSCLC and attempted to construct a diagnostic model with better discriminative performance to predict the TMB status.
A total of 10 robust radiomics features were screened to construct intra-tumoral and peritumoral combined radiomics model, which showed promising predictive efficacy (AUC of 0.844 and 0.841 in the training and validation set, respectively (46). Guan et al.’s (54) study showed that the radiomics model also had a certain predictive value for TMB status in small cell lung cancer. In contrast, this study included image transformation features including wavelet features and LoG features. Gauss-Laplacian operator is a kind of edge enhancement filter. Firstly, image smoothing operation is carried out by Gaussian operator, and then Laplacian operator is carried out to enhance the edge effect in the image, thus amplifying the change of local gray value. In this study, the original image was used to generate four different transformation images by LoG operators of 4 different apertures (0.5 mm, 1 mm, 1.5 mm, 2 mm), and then all the above texture features were further extracted from the transformation image, that is, LoG transformation features were formed. Among the radiomic features that we finally screened into the model, only 1 original feature, 2 texture features, and the remaining 7 were all LoG transformation features, which showed the importance of image transformation features in TMB prediction. The transformation of the image through the filter can enhance some features of the original image and expose some previously hidden information.
The radiomics model is quite effective in predicting the TMB status, but it only contains the image information of the patient. The combination of clinical, pathological, and image information can more comprehensively summarize the characteristics of the tumor, so as to more accurately evaluate the TMB status. The prediction efficiency of the clinical model was better than that of the radiomics model (accuracy: 0.825 vs. 0.754, specificity: 0.884 vs. 0.744). Therefore, smoking history, pathologic type, and rad-score were selected as independent predictors of TMB status to construct the nomogram. The comparison of the predictive efficacy of different models is shown in Figure 7, the nomogram had the highest clinical application value, and the radiomics model also had good predictive potential, both of which were significantly superior to the clinical model. The quantitative probability of NSCLC in the state of TMB-H could be accurately calculated according to the nomogram, which could be used as an important reference for the assessment of the TMB status and help clinicians predict the efficacy of immunotherapy in NSCLC.
Peritumoral tissues surrounding lung cancer constitute the tumor microenvironment, which is closely related to the occurrence and development of lung cancer. Researchers found that the number of tumor-associated inflammatory cells (TAICs) in the peritumoral area of NSCLC was higher than that in the intra-tumoral area (35). Individual differences in the tumor microenvironment will lead to subtle changes in the image, whereas the imaging signs in the peritumoral region are likely to contain a large amount of tumor-related information, which has potential predictive value (55,56). Therefore, a radiomics model of the peritumoral region can be established to evaluate the differences in the tumor microenvironment (29,39). Braman et al. (57) identified the molecular subtypes of human epidermal growth factor receptor 2 (HER2)+ breast cancer by combining intra-tumoral and peritumoral features on imaging. Pérez-Morales et al. (58) used intra-tumoral and peritumoral radiomics features of low-dose CT images to predict survival. Currently, no studies have investigated the value of peritumoral regional features in distinguishing the TMB status in lung cancer. Our study evaluated the predictive performance of peritumoral radiomics features extracted from a 5-mm annular area around NSCLC lesions. The results showed that the radiomics model of the peritumoral region could distinguish the TMB status to a certain extent, and the AUC of the validation set was 0.728, which had auxiliary diagnostic value. This suggests that radiomic features were of great significance in the 5 mm peritumoral environment around the tumor and should be taken seriously in future radiomics studies.
In summary, compared with clinical features, radiomics has high-dimensional data, which can reflect tumor TMB status more accurately and comprehensively. It is a potentially valuable non-invasive, simple, and economical method for evaluating TMB status. In addition, TMB is highly dynamic with tumor development or treatment outcome, and the non-invasive detection method of radiomics can also make real-time dynamic monitoring of TMB possible.
The study had several limitations. First, the cohort in this study included both surgically resected patients and lung aspiration biopsy patients. The TMB detection results of the latter were derived from small puncture specimens, which may not have been accurate enough due to tumor heterogeneity, thus interfering with the predictive efficacy of the model. Second, this was a single-center retrospective study without external validation, so the diagnostic efficacy of the models needed to be further verified. Finally, in the process of ROI segmentation, there was the influence of physicians’ subjective judgment, and the subsequent research should be further improved.
Conclusions
The radiomics model performed better than the clinical model in evaluating the TMB status of NSCLC patients. The radiomic features of the intra-tumoral area had better diagnostic efficacy, and the radiomics features of the peritumoral area also played an important role in the evaluation of the TMB status. The nomogram combined with clinical and radiomics features had the best predictive ability and clinical value, which could provide more information for clinicians in the TMB status assessment, ICI efficacy prediction, and immunotherapy program formulation. The nomogram is a non-invasive, affordable, rapid, simple, and can eliminate the impact of tumor heterogeneity detection method, more in line with the clinical needs of tumor patients, so it is more practical in clinical practice. In the future, from the perspective of radiomics, a multivariate efficacy prediction model can be established by using TMB and other factors related to ICI efficacy to help clinicians make more reasonable combination therapy decisions, so as to bring higher survival rate for lung cancer patients and avoid additional toxicity.
Acknowledgments
Funding: This study was supported by the Big Data Project of Chinese People’s Liberation Army General Hospital (No. 2019MBD-052) and Beijing Capital Development Special Project for Health Research (No. 2020-1-5011).
Footnote
Reporting Checklist: The authors have completed the TRIPOD reporting checklist. Available at https://tlcr.amegroups.com/article/view/10.21037/tlcr-23-171/rc
Data Sharing Statement: Available at https://tlcr.amegroups.com/article/view/10.21037/tlcr-23-171/dss
Peer Review File: Available at https://tlcr.amegroups.com/article/view/10.21037/tlcr-23-171/prf
Conflicts of Interest: All authors have completed the ICMJE uniform disclosure form (available at https://tlcr.amegroups.com/article/view/10.21037/tlcr-23-171/coif). The authors have no conflicts of interest to declare.
Ethical Statement: The authors are accountable for all aspects of the work in ensuring that questions related to the accuracy or integrity of any part of the work are appropriately investigated and resolved. The study was conducted in accordance with the Declaration of Helsinki (as revised in 2013). This study was approved by the Ethics Committee of the First Medical Center of Chinese People’s Liberation Army General Hospital (No. S2020-173-01), and the requirement for informed consent of the patients was waived.
Open Access Statement: This is an Open Access article distributed in accordance with the Creative Commons Attribution-NonCommercial-NoDerivs 4.0 International License (CC BY-NC-ND 4.0), which permits the non-commercial replication and distribution of the article with the strict proviso that no changes or edits are made and the original work is properly cited (including links to both the formal publication through the relevant DOI and the license). See: https://creativecommons.org/licenses/by-nc-nd/4.0/.
References
- Skoulidis F, Goldberg ME, Greenawalt DM, et al. STK11/LKB1 Mutations and PD-1 Inhibitor Resistance in KRAS-Mutant Lung Adenocarcinoma. Cancer Discov 2018;8:822-35. [Crossref] [PubMed]
- Rittmeyer A, Barlesi F, Waterkamp D, et al. Atezolizumab versus docetaxel in patients with previously treated non-small-cell lung cancer (OAK): a phase 3, open-label, multicentre randomised controlled trial. Lancet 2017;389:255-65. [Crossref] [PubMed]
- Topalian SL, Hodi FS, Brahmer JR, et al. Five-Year Survival and Correlates Among Patients With Advanced Melanoma, Renal Cell Carcinoma, or Non-Small Cell Lung Cancer Treated With Nivolumab. JAMA Oncol 2019;5:1411-20. [Crossref] [PubMed]
- Provencio M, Ortega AL, Coves-Sarto J, et al. Atezolizumab Plus Bevacizumab as First-line Treatment for Patients With Metastatic Nonsquamous Non-Small Cell Lung Cancer With High Tumor Mutation Burden: A Nonrandomized Controlled Trial. JAMA Oncol 2023;9:344-53. [PubMed]
- Antonia SJ, Borghaei H, Ramalingam SS, et al. Four-year survival with nivolumab in patients with previously treated advanced non-small-cell lung cancer: a pooled analysis. Lancet Oncol 2019;20:1395-408. [Crossref] [PubMed]
- Garon EB, Hellmann MD, Rizvi NA, et al. Five-Year Overall Survival for Patients With Advanced Non‒Small-Cell Lung Cancer Treated With Pembrolizumab: Results From the Phase I KEYNOTE-001 Study. J Clin Oncol 2019;37:2518-27. [Crossref] [PubMed]
- Carbone DP, Reck M, Paz-Ares L, et al. First-Line Nivolumab in Stage IV or Recurrent Non-Small-Cell Lung Cancer. N Engl J Med 2017;376:2415-26. [Crossref] [PubMed]
- Blank CU, Haanen JB, Ribas A, et al. Cancer Immunology. The "cancer immunogram". Science 2016;352:658-60. [Crossref] [PubMed]
- Dudnik E, Peled N, Nechushtan H, et al. BRAF Mutant Lung Cancer: Programmed Death Ligand 1 Expression, Tumor Mutational Burden, Microsatellite Instability Status, and Response to Immune Check-Point Inhibitors. J Thorac Oncol 2018;13:1128-37. [Crossref] [PubMed]
- Gao J, Shi LZ, Zhao H, et al. Loss of IFN-γ Pathway Genes in Tumor Cells as a Mechanism of Resistance to Anti-CTLA-4 Therapy. Cell 2016;167:397-404.e9. [Crossref] [PubMed]
- Chae YK, Viveiros P, Lopes G, et al. Clinical and Immunological Implications of Frameshift Mutations in Lung Cancer. J Thorac Oncol 2019;14:1807-17. [Crossref] [PubMed]
- Bubendorf L, Zoche M, Dafni U, et al. Prognostic impact of tumour mutational burden in resected stage I and II lung adenocarcinomas from a European Thoracic Oncology Platform Lungscape cohort. Lung Cancer 2022;174:27-35. [Crossref] [PubMed]
- Yang Y, Shen S, Sun Y, et al. The relationship between different subtypes of KRAS and PD-L1 & tumor mutation burden (TMB) based on next-generation sequencing (NGS) detection in Chinese lung cancer patients. Transl Lung Cancer Res 2022;11:213-23. [Crossref] [PubMed]
- Sharabi A, Kim SS, Kato S, et al. Exceptional Response to Nivolumab and Stereotactic Body Radiation Therapy (SBRT) in Neuroendocrine Cervical Carcinoma with High Tumor Mutational Burden: Management Considerations from the Center For Personalized Cancer Therapy at UC San Diego Moores Cancer Center. Oncologist 2017;22:631-7. [Crossref] [PubMed]
- Büttner R, Longshore JW, López-Ríos F, et al. Implementing TMB measurement in clinical practice: considerations on assay requirements. ESMO Open 2019;4:e000442. [Crossref] [PubMed]
- Samstein RM, Lee CH, Shoushtari AN, et al. Tumor mutational load predicts survival after immunotherapy across multiple cancer types. Nat Genet 2019;51:202-6. [Crossref] [PubMed]
- Zheng M. Tumor mutation burden for predicting immune checkpoint blockade response: the more, the better. J Immunother Cancer 2022;10:e003087. [Crossref] [PubMed]
- Fumet JD, Truntzer C, Yarchoan M, et al. Tumour mutational burden as a biomarker for immunotherapy: Current data and emerging concepts. Eur J Cancer 2020;131:40-50. [Crossref] [PubMed]
- Freedman AN, Klabunde CN, Wiant K, et al. Use of Next-Generation Sequencing Tests to Guide Cancer Treatment: Results From a Nationally Representative Survey of Oncologists in the United States. JCO Precis Oncol 2018;2:PO.18.00169.
- Karamitopoulou E, Andreou A, Wenning AS, et al. High tumor mutational burden (TMB) identifies a microsatellite stable pancreatic cancer subset with prolonged survival and strong anti-tumor immunity. Eur J Cancer 2022;169:64-73. [Crossref] [PubMed]
- Galvano A, Gristina V, Malapelle U, et al. The prognostic impact of tumor mutational burden (TMB) in the first-line management of advanced non-oncogene addicted non-small-cell lung cancer (NSCLC): a systematic review and meta-analysis of randomized controlled trials. ESMO Open 2021;6:100124. [Crossref] [PubMed]
- D'Arcangelo M, D'Incecco A, Ligorio C, et al. Programmed death ligand 1 expression in early stage, resectable non-small cell lung cancer. Oncotarget 2019;10:561-72. [Crossref] [PubMed]
- Hellmann MD, Callahan MK, Awad MM, et al. Tumor Mutational Burden and Efficacy of Nivolumab Monotherapy and in Combination with Ipilimumab in Small-Cell Lung Cancer. Cancer Cell 2019;35:329. [Crossref] [PubMed]
- Wu YL, Wang CL, Liao ML, et al. A consensus on immunotherapy from the 2017 Chinese Lung Cancer Summit expert panel. Transl Lung Cancer Res 2018;7:428-36. [Crossref] [PubMed]
- Sun C, Mezzadra R, Schumacher TN. Regulation and Function of the PD-L1 Checkpoint. Immunity 2018;48:434-52. [Crossref] [PubMed]
- Xu F, Guan Y, Zhang P, et al. Tumor mutational burden presents limiting effects on predicting the efficacy of immune checkpoint inhibitors and prognostic assessment in adrenocortical carcinoma. BMC Endocr Disord 2022;22:130. [Crossref] [PubMed]
- Bravaccini S, Bronte G, Ulivi P. TMB in NSCLC: A Broken Dream? Int J Mol Sci 2021;22:6536. [Crossref] [PubMed]
- Sung MT, Wang YH, Li CF. Open the Technical Black Box of Tumor Mutational Burden (TMB): Factors Affecting Harmonization and Standardization of Panel-Based TMB. Int J Mol Sci 2022;23:5097. [Crossref] [PubMed]
- Guo Y, Song Q, Jiang M, et al. Histological Subtypes Classification of Lung Cancers on CT Images Using 3D Deep Learning and Radiomics. Acad Radiol 2021;28:e258-66. [Crossref] [PubMed]
- Jia TY, Xiong JF, Li XY, et al. Identifying EGFR mutations in lung adenocarcinoma by noninvasive imaging using radiomics features and random forest modeling. Eur Radiol 2019;29:4742-50. [Crossref] [PubMed]
- Yan M, Wang W. Development of a Radiomics Prediction Model for Histological Type Diagnosis in Solitary Pulmonary Nodules: The Combination of CT and FDG PET. Front Oncol 2020;10:555514. [Crossref] [PubMed]
- Ninatti G, Kirienko M, Neri E, et al. Imaging-Based Prediction of Molecular Therapy Targets in NSCLC by Radiogenomics and AI Approaches: A Systematic Review. Diagnostics (Basel) 2020;10:359. [Crossref] [PubMed]
- Bak SH, Lee HY, Kim JH, et al. Quantitative CT Scanning Analysis of Pure Ground-Glass Opacity Nodules Predicts Further CT Scanning Change. Chest 2016;149:180-91. [Crossref] [PubMed]
- Hong D, Xu K, Zhang L, et al. Radiomics Signature as a Predictive Factor for EGFR Mutations in Advanced Lung Adenocarcinoma. Front Oncol 2020;10:28. [Crossref] [PubMed]
- Weng Q, Zhou L, Wang H, et al. A radiomics model for determining the invasiveness of solitary pulmonary nodules that manifest as part-solid nodules. Clin Radiol 2019;74:933-43. [Crossref] [PubMed]
- Wang S, Shi J, Ye Z, et al. Predicting EGFR mutation status in lung adenocarcinoma on computed tomography image using deep learning. Eur Respir J 2019;53:1800986. [Crossref] [PubMed]
- Luo T, Xu K, Zhang Z, et al. Radiomic features from computed tomography to differentiate invasive pulmonary adenocarcinomas from non-invasive pulmonary adenocarcinomas appearing as part-solid ground-glass nodules. Chin J Cancer Res 2019;31:329-38. [Crossref] [PubMed]
- Prestipino A, Zeiser R. Clinical implications of tumor-intrinsic mechanisms regulating PD-L1. Sci Transl Med 2019;11:eaav4810. [Crossref] [PubMed]
- Tu L, Choi HHF, Clark H, et al. Machine Learning-Generated Radiomic and Clinical Data for Lung Cancer Survival Prediction. Medical Physics 2022;49:E689.
- Yarchoan M, Albacker LA, Hopkins AC, et al. PD-L1 expression and tumor mutational burden are independent biomarkers in most cancers. JCI Insight 2019;4:e126908. [Crossref] [PubMed]
- Sharpnack MF, Cho JH, Johnson TS, et al. Clinical and Molecular Correlates of Tumor Mutation Burden in Non-Small Cell Lung Cancer. Lung Cancer 2020;146:36-41. [Crossref] [PubMed]
- Chowell D, Morris LGT, Grigg CM, et al. Patient HLA class I genotype influences cancer response to checkpoint blockade immunotherapy. Science 2018;359:582-7. [Crossref] [PubMed]
- Routy B, Le Chatelier E, Derosa L, et al. Gut microbiome influences efficacy of PD-1-based immunotherapy against epithelial tumors. Science 2018;359:91-7. [Crossref] [PubMed]
- Shen L, Zhang J, Lee H, et al. RNA Transcription and Splicing Errors as a Source of Cancer Frameshift Neoantigens for Vaccines. Sci Rep 2019;9:14184. [Crossref] [PubMed]
- Derosa L, Routy B, Fidelle M, et al. Gut Bacteria Composition Drives Primary Resistance to Cancer Immunotherapy in Renal Cell Carcinoma Patients. Eur Urol 2020;78:195-206. [Crossref] [PubMed]
- Bregni G, Sticca T, Camera S, et al. Feasibility and clinical impact of routine molecular testing of gastrointestinal cancers at a tertiary centre with a multi-gene, tumor-agnostic, next generation sequencing panel. Acta Oncol 2020;59:1438-46. [Crossref] [PubMed]
- Slansky JE, Spellman PT. Alternative Splicing in Tumors - A Path to Immunogenicity? N Engl J Med 2019;380:877-80. [Crossref] [PubMed]
- Qiu P, Poehlein CH, Marton MJ, et al. Measuring Tumor Mutational Burden (TMB) in Plasma from mCRPC Patients Using Two Commercial NGS Assays. Sci Rep 2019;9:114. [Crossref] [PubMed]
- Gandara DR, Paul SM, Kowanetz M, et al. Blood-based tumor mutational burden as a predictor of clinical benefit in non-small-cell lung cancer patients treated with atezolizumab. Nat Med 2018;24:1441-8. [Crossref] [PubMed]
- Qiu Y, Liu L, Yang H, et al. Integrating Histologic and Genomic Characteristics to Predict Tumor Mutation Burden of Early-Stage Non-Small-Cell Lung Cancer. Front Oncol 2021;10:608989. [Crossref] [PubMed]
- Mosele F, Remon J, Mateo J, et al. Recommendations for the use of next-generation sequencing (NGS) for patients with metastatic cancers: a report from the ESMO Precision Medicine Working Group. Ann Oncol 2020;31:1491-505. [Crossref] [PubMed]
- Feng B, Chen X, Chen Y, et al. Radiomics nomogram for preoperative differentiation of lung tuberculoma from adenocarcinoma in solitary pulmonary solid nodule. Eur J Radiol 2020;128:109022. [Crossref] [PubMed]
- Zhang N, Wu J, Yu J, et al. Integrating Imaging, Histologic, and Genetic Features to Predict Tumor Mutation Burden of Non-Small-Cell Lung Cancer. Clin Lung Cancer 2020;21:e151-e163. [Crossref] [PubMed]
- Guan C, Li M, Jiang L, et al. Predicting tumor mutational burden in squamous cell lung carcinoma by constructing a computed tomography radiomics model. Journal of China Medical University 2022;51:481-6.
- Wilson R, Devaraj A. Radiomics of pulmonary nodules and lung cancer. Transl Lung Cancer Res 2017;6:86-91. [Crossref] [PubMed]
- Fan L, Fang M, Li Z, et al. Radiomics signature: a biomarker for the preoperative discrimination of lung invasive adenocarcinoma manifesting as a ground-glass nodule. Eur Radiol 2019;29:889-97. [Crossref] [PubMed]
- Braman N, Kunte S, Bera K, et al. Change in intra-lesion heterogeneity on CT predicts long-term survival following treatment with CDK4/6 inhibitors in hormone receptor-positive metastatic breast cancer (MBC). Cancer Res 2021;81:PS5-42. [Crossref]
- Pérez-Morales J, Lu H, Mu W, et al. Volume doubling time and radiomic features predict tumor behavior of screen-detected lung cancers. Cancer Biomark 2022;33:489-501. [Crossref] [PubMed]
(English Language Editor: J. Jones)