Genomic alterations dissection revealed MUC4 mutation as a potential driver in lung adenocarcinoma local recurrence
Highlight box
Key findings
• We dissected the mutational landscape of recurrent lung adenocarcinoma. Some genes were more mutated in recurrent tumors, such as the MUC17, KRAS and ZNF families. ErbB signaling pathway, MAPK pathway and cell cycle pathway were highly activated in recurrent tumors and potential mechanisms driving lung adenocarcinoma recurrence.
What is known and what is new?
• Tumor recurrence was an important cause of death of lung adenocarcinoma patients. But the underlying reasons causing tumor recurrence were complicated and not well known.
• We described mutational landscape of recurrent lung adenocarcinoma and identified significantly mutated genes in in recurrent tumors, such as the MUC17, KRAS and ZNF families. Specific pathways such as ErbB signaling pathway, MAPK pathway and cell cycle pathway were highly activated in recurrent tumors. Potential oncogenes such as MUC4 was also identified in this study.
What is the implication, and what should change now?
• Mutational landscape changed during lung adenocarcinoma recurrence and new driver mutations may occur in this procedure. Routine monitoring of mutations during patients’ treatment may be necessary to improve lung adenocarcinoma prognosis. Potential driver genes such as MUC4 was important to lung adenocarcinoma and need further investigation.
Introduction
Lung cancer remains the leading cause of cancer death worldwide (1), and lung adenocarcinoma (LUAD) is the most common histology type (2). Genomic alterations such as driver mutations play an important role in LUAD tumorigenesis, and targeted therapy is widely used and benefits late stage LUAD patients with positive mutations (3-5). However, whether adjuvant targeted therapy could improve patients’ prognosis is still controversial. Targeted therapy such as Osimertinib could prolong the disease-free survival (DFS) of resected LUAD patients, however the over-all survival was not improved (6). About 30–55% of LUAD patients still developed recurrence after complete resection, which remained the main cause of death in LUAD patients (7-9). Therefore, understanding the genomic alterations during tumor recurrence and identifying patients at high-risk of recurrence after resection are vital to improve the prognosis of LUAD patients.
LUAD is a high heterogeneous disease involving not only neoplastic genomic alterations but also interferential individual genomic differences during its recurrence. Therefore, genomic alterations are needed for both primary and recurrent tumors. Conventionally, most patients with recurrence do not receive surgery, especially for patients with distant recurrence, as that would limit the acquiring of the pairwise recurrent tumors. However, for local recurrence patients with isolated and resectable tumors, surgery is still considerable as it would lead to more favorable results than chemotherapy alone (10). The resected tumor samples enable researchers to investigate the underlying genomic alterations driving LUAD recurrence.
In this study, 84 paired primary and recurrent Formalin-Fixed and Parrffin-Embedded (FFPE) tumor samples were collected from 41 LUAD patients, including two patients received a third pulmonary resection surgery due to a second time recurrence. High depth of whole exon sequencing (WES) was performed to identify the genomic alterations and downstream pathways between primary and recurrence LUAD samples. By a randomization test method, several critical mutations such as MUC4, KRAS, MUC17 and ZNF families, driving LUAD recurrence, were further identified. This article is presented in accordance with the MDAR reporting checklist (available at https://tlcr.amegroups.com/article/view/10.21037/tlcr-22-793/rc).
Methods
Patients
This study retrospectively enrolled 41 patients diagnosed with recurrent LUAD from January 2008 to December 2018 in our institution (Fudan University Shanghai Cancer Center). Patients were included in this study according to the following criteria: (I) patients underwent complete resection (R0) for histologically proven primary LUAD; (II) patients underwent second resection for a histologically proven tumor recurrence; (III) primary tumors were all treatment-naïve before the first resection; (IV) each patient had matched primary tumors, recurrent tumors and adjacent normal lung tissue. The discrimination of second primary LUAD and intrapulmonary metastasis/recurrence was based on the American Joint Committee on Cancer eighth edition cancer staging manual (11). Patients diagnosed with second primary lung cancer were excluded.
The paired primary and recurrent tumor samples and adjacent normal lung tissue samples were collected. All samples were available as Formalin-Fixed and Parrffin-Embedded (FFPE) sample blocks, then 5–10 µm unstained sections were made and Hematoxylin-Eosin (HE) staining were performed as per the standard procedure. The pathological feature of each slide was diagnosed and whether the tumor was recurrence was confirmed by experienced pathologists in our institution. There were two patients received three times of pulmonary resections with one primary and two recurrent tumors. Therefore, 41 primary tumor samples, 43 recurrent tumor samples and 41 adjacent normal lung samples were included in the study.
The study was conducted in accordance with the Declaration of Helsinki (as revised in 2013). This study was approved by the Committee for Ethical Review of Research of Fudan University Shanghai Cancer Center Institutional Review Board (No. 090977-1). Informed consents for donating their samples to the tissue bank of Fudan University Shanghai Cancer Center were obtained from patients themselves or their relatives.
DNA extraction and whole exon sequencing
DNA was extracted from unstained FFPE sections using the QIAamp DNA FFPE Tissue Kit (QIAGEN, Cat. No. 56404) according to the instructions of the manufacturer. DNA quantitation was assessed with Qubit 3.0 fluorometer and Qubit dsDNA HS Assay Kit (Thermo Fisher Scientific, Cat. No. Q33216, Cat. No. Q32854). Genomic DNA was fragmented by Bioruptor UCD-200 (Diagenode) into 350 bp fragments, and then processed as end-repaired, A-tailing and ligation with universal pair-end adaptors. Then PCR amplification was performed and fragments containing exome-related regions were captured. PCR amplification was performed again to construct the libraries. Library DNA concentration was detected with Qubit 3.0, and library fragment size distribution was detected with Agilent 2100 bioanalyzer. Finally, high throughput sequencing was carried out on Illumina NovaSeq-6000 sequencer with target sequencing depth 200× for tumor tissue and 100× for normal lung tissue.
WES data processing
Quality control including adapter trimming and low-quality reads filtering was performed on raw sequencing data to generate clean data using fastq (12). After quality control, sequence reads were aligned to the reference human genome (Version human_glk_v37) using BWA (13). SAMtools was used to convert the format of the alignment results (14). Then Picard (http://broadinstitute.github.io/picard/) was used to remove PCR duplications. Base quality adjustment and germline variant calling were performed by GATK4 (15) (Genome Analysis Toolkit) BaseRecalibrator module and Haplotypecaller module (the thresholds for getting passed variants were QD <2.0).
Somatic single-nucleotide variants (SNVs) and small insertions and deletions (indels) were detected using MuTect2 (16). All detected variants were annotated using ANNOVAR (17) based on several databases, including the 1,000 Genomes Project, EXAC, ESP6500, gnomAD, SIFT, clinvar, PolyPhen, MutationTaster, COSMIC (18). Somatic copy number variations (CNV) were identified by Control-FREEC with default settings (19). Genes with total copy number greater than gene-level median ploidy were considered as gains, less than ploidy were considered as loss, total copy number of 0 was considered as homozygous deletion. Somatic structural variations (SVs) were identified using Lumpy (20). There were five main types of SV, including deletion (DEL), duplication (DUP), inversion (INV), intra-chromosomal translocation (ITX) and inter-chromosomal translocation (CTX).
Mutational signature analysis
Synonymous and non-synonymous somatic SNVs were analyzed to identify point mutation types (including six types: T > A, T > C, T > G, C > A, C > G, C > T) in each tumor sample using R package maftools (21). The analysis of point mutation types (including six types: T > A, T > C, T > G, C > A, C > G, C > T) in each tumor sample was estimated using R package maftools. R package Palimpsest was used to estimate the mutational signature contribution of each tumor sample based on the non-negative matrix factorization (NMF) approach (22). Signatures that contributed less than 6% of a sample were removed and mutations were reassigned to the signatures that remained. Obtained signatures were compared with COSMIC signature.
Analysis of significantly mutated genes (SMGs)
Significantly mutated genes were identified using MutsigCV across the whole cohort, primary group and recurrence group (23). Genes with P<0.01 were considered as significantly mutated genes. Genes with P<0.01 and mutated in at least 5% all patients were visualized in oncoprint.
Identification of significantly amplified/deleted regions
Somatic copy number variations were analysis using GISTIC2.0 to further identify the significantly amplified and deleted regions across the samples (24). A confidence interval of 99% was set to determine the significance. The GISTIC2.0 output files were processed by R package maftools, and cytobands with the top 5 lowest q values were visualized. Copy number loss (copy number 1), homozygous deletion (copy number 0), and amplification (copy number >4) were considered in the analysis. Significantly mutated copy number regions were assessed using GISTIC2. Genes in a focal region with P value <0.01 were considered as significant genes.
The Cancer Genome Atlas (TCGA)-LUAD dataset
Somatic mutation and copy number variation and clinical information of TCGA-LUAD cohort were accessed with R packages GDCquery and TCGAmutations. The effects of mutation status on RFS (recurrence-free survival) were analyzed. The RNA-seq data was analyzed with DESeq2 (25).
Identification of primary and recurrence-specific driver genes by randomization test
To identify specific driver genes in primary and recurrent tumors, MutsigCV was performed on 41 primary LUAD samples and 43 recurrent samples. Genes with P<0.01 in one group but P>0.1 in other group were selected as candidate genes. Then, these 84 LUAD samples were randomly split into two groups (41 samples in primary group and 43 samples in recurrence group). Then, MutSigCV was performed on the two groups and significance P values of the candidate genes were transformed to (−log10). This randomization procedure was repeated 100 times and the transformed P values generally followed a normal distribution for each candidate gene. For each candidate gene, the calculation was processed to determine the probability that the significance observed in the primary or metastasis group was whether stronger than expected by chance. Significant genes from the randomization test (two-tail P<0.05) were regarded as the true primary or recurrence- differentiated specific genes.
Pathway analysis
Base on SIFT, PolyPhen and MutationTaster scores, OncodriverFM was used to identify significantly mutated Kyoto Encyclopedia of Genes and Genomes (KEGG) pathways (q<0.05) in primary and recurrence group (26). The R package Graphite was used to map and convert pathway topologies into KEGG pathway-derived gene networks. Only pathways with at least two protein-coding genes mutated in one group were included into analysis. Hierarchical HotNet algorithm was applied to KEGG-derived gene-gene networks to identify highly mutated subnetworks (27). Hierarchical HotNet analysis was conducted using all the somatically mutated genes in the recurrent group. Visualization and annotation of the subnetwork was performed using Cytoscape (version 3.5.1).
Clonal evolution analysis
Pyclone-vi was applied to estimate clone population structure of each tumor (28). To ensure the accuracy, clusters with fewer than 5 mutations or cellular prevalence below 2% were excluded. Besides, two clusters were emerged if their cellular prevalence difference less than 2%. For each tumor sample, the cluster with the highest cellular prevalence was identified as the clonal cluster and clusters with lower cellular frequencies were treated as sub-clones. Citup (version 0.1.0) tool was used to infer the phylogenetic tree from pyclone-vi results. The phylogenetic trees of each patient were visualized by timescape R package.
Tumor purity and intra-tumor heterogeneity (ITH) analysis
ABSOULTE algorithm was used to estimated tumor purity and ploidy based on somatic copy number variation and mutation allele fraction information. Mutant-allele tumor heterogeneity (MATH) score is a simple and quantitative indicator to evaluate ITH. R package maftools was applied to calculate MATH score for each tumor sample in this cohort and TCGA-LUAD cohort.
Statistical analysis
Continuous variables were presented as mean ± standard deviation (SD) or median (interquartile range), and categorical variables were presented as frequency and percentage. Student’s t-test or Mann-Whitney U test was used to compare continuous variables. χ2-test or Fisher’s exact test was used to compare categorical variables. If not noted otherwise, all tests were two-sided and P values <0.05 was considered statistically significant. All statistical analyses were performed by R statistical environment (version 4.0.3).
Results
Sample information and patients’ clinical features
In this study, 84 samples from 41 patients with LUAD who underwent the primary and recurrent tumors resection in our institution were included (Table 1 and Figure 1). Of these 41 patients, 37 patients were diagnosed as intrapulmonary metastasis, 2 patients were chest wall metastasis, 1 patient was lymph node metastasis, and 1 patient was pleural metastasis. Two patients underwent surgery operations for twice recurrence were both diagnosed as intrapulmonary metastases.
Table 1
Characteristics | Recurrent LUAD patients (n=41) |
---|---|
Age at first operation (years) | 59.0±7.42 |
Age at second operation (years) | 62.1±7.73 |
Gender | |
Female | 16 (39.0) |
Male | 25 (61.0) |
Smoking status | |
Former/current smoker | 23 (56.1) |
Never smoker | 18 (43.9) |
Interval time (years) | 2.8 (1.6–4.3) |
Adjuvant therapy before surgery for recurrent LUAD | |
Chemotherapy | 15 (36.6) |
Chemotherapy + radiotherapy | 2 (4.9) |
Chemotherapy + EGFR-TKI | 1 (2.4) |
Palliative therapy after surgery for recurrent LUAD | |
Chemotherapy | 4 (9.8) |
Chemotherapy + radiotherapy | 4 (9.8) |
Chemotherapy + EGFR-TKI | 4 (9.8) |
EGFR-TKI | 3 (7.3) |
Location | |
Lung parenchyma | 37 (90.2) |
Chest | 2 (4.9) |
Pleural | 1 (2.4) |
Lymph nodes | 1 (2.4) |
Data are presented as mean ± SD, median (interquartile range) or n (%). SD, standard deviation; LUAD, lung adenocarcinoma; EGFR-TKI, epidermal growth factor receptor tyrosine kinase inhibitor.
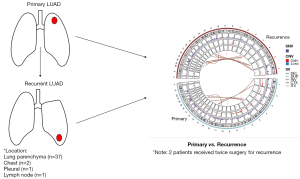
The patients’ average age was 59.0 years old at the primary tumor resection and 62.1 years old at the recurrent tumor resection. A proportion of 61.0% of patients were male and 56.1% of patients had history of smoking. All patients were treatment naïve before primary tumor resection and 18 patients received adjuvant therapy before surgery for recurrent tumor. Among 18 treated patients, 15 patients received chemotherapy alone, 2 patient received chemotherapy and radiotherapy, and 1 patient received chemotherapy and epidermal growth factor receptor tyrosine kinase inhibitor (EGFR-TKI) therapy. After surgery for recurrent tumor, 15 patients received palliative therapy, including 4 patients received chemotherapy only, 4 patients received chemoradiotherapy and 3 patients received EGFR-TKI therapy only. The median interval time between primary tumor resection and recurrent tumor resection was 2.8 years (range, 1.6–4.3 years) (Table 1 and Figure 1).
Genomic alteration landscape revealed specific copy number variations in recurrence LUAD
Genomic DNA was extracted and made into libraries for WES. The sequencing data was analyzed as described in Methods section, and then the somatic mutations, structure variations and copy number variations were identified in both primary and recurrent tumors (Figure S1). In primary tumors, there were 62,920 SNVs, 11,839 CNVs (36,906 CNV genes) and 28,768 SVs. In the meantime, 49,655 SNVs, 9,120 CNVs (31,756 CNV genes) and 25,337 SVs were detected in recurrent tumors. The genomic landscape of SNVs, CNVs and SVs at the chromosome level between primary and recurrent tumors with Circos plot (Figure 1).
CNV events including amplification, deletion and loss of heterozygosity (LOH) events were assessed (Figure 2A). Many CNV events were highly corelated with genomic SVs (Figure 2B). Compared to primary group, less CNV events but more proportion of deleted genes were observed in recurrence group (P<0.01, Figure 2C). In both groups, deletion and duplication were the main types of SVs (Figure 2D). Significantly amplified and deleted regions were subsequently identified using GISTIC2.0 algorithm. Amplifications at 1q21.2, 5p15.33, 6p22.2, 15q11.2 and deletions at 9q34.3 were observed in primary group (Figure 2E). Amplifications at 1q21.2, 5p15.33, 8q24.21 and deletions at 9p21.3 were observed in recurrence group (Figure 2F). Amplification of 8q24.21 and deletion of 9p21.3 were recurrence specific CNV regions, indicating that genes in these two regions maybe related to LUAD recurrence. One of the most studied oncogenes, MYC is located at 8q24.21 region surrounding by numerous non-coding RNAs highly associated with increased cancer risk. Part of genes located in 9p21.3, such as CDKN2A, CDKN2B and MTAP, were tumor suppressors that could regulate cell cycle and prevent tumor developing.
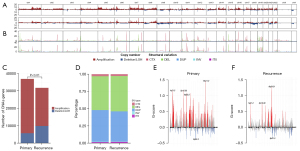
Mutation spectrum and mutation signature were similar between primary and recurrence LUAD
Mutation spectrum depicted the type and number of the point mutation in each primary and recurrent tumor sample (Figure 3A). Point mutation types were consistent between matched primary and recurrent tumors (Table S1). C>T transversion and C>A transition were the most common point mutation types in both primary and recurrence group, which was consistent with previous study (29). Besides, there was a negative correlation between C>T transversion and C>A transition (30).
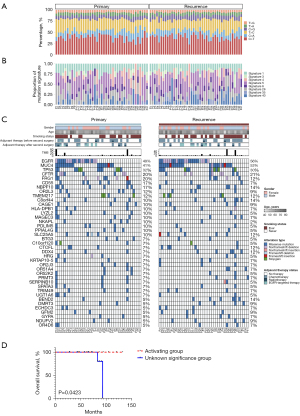
Mutation signature analysis was performed on somatic mutation data of 84 primary and recurrence samples. In total of 8 signatures were identified across all samples, including signature1, 3, 4, 5, 6, 26, 35 and 40 (Figure 3B). In the samples of the current study, mutation signature composition was similar between primary and recurrent tumors. Signature 5 was the dominant signature in both primary (n=35) and recurrence group (n=40).
Landscape of significantly mutated genes
In this study, MutSigCV was used to identify significantly mutated genes (SMGs) across all tumor samples (cutoff P<0.01). Then, 39 genes mutated in at least 5% of all tumor samples were selected for visualization. Besides the significantly mutated genes, patients’ clinical features, including age, gender, smoking status, therapy condition and tumor mutation burden (TMB) were shown by groups (Figure 3C). The top ten genes with highest frequency were as follows: EGFR, MUC4, TP53, CFTR, FRG1, CD55, NBPF10, OR2L3, TEME217, C8orf44. Of these genes, EGFR, TP53 and KRAS are well-known LUAD driver genes (31). Consistent with previous studies, the most mutated gene was EGFR in this study, with mutation frequency of 49% in primary group and 56% in recurrence group. Thirty-six EGFR mutations (including non-coding mutations) were detected in 23 primary tumors, including 22 activating mutations (exon 19 deletions, L858R, E709A and L861Q) and 14 unknown significance mutations (D994D, L62R, L833F, N158N, Q787Q and noncoding mutations). However, no resistance mutation (suck like T790M) was detected in primary tumors. Patients with only activating mutations were defined as activating group (15 patients) and the other patients as unknown significance group (8 patients). The activating group has better over-all survival as the only 2 deaths were observed in unknown significance group (Figure 3D).
TP53 mutated less in this study than previous studies, however, MUC4 had extremely higher mutation frequency. MUC4 was mutated in 41% of primary tumors and 53% of recurrent tumors, much higher than previous studies (12/230 TCGA samples, 5.2%) (30). As a membrane-bound mucin, MUC4 was reported to promote carcinogenetic progression via activating ERBB2 pathway (32). MUC4 mutation-positive LUAD was associated with worse prognosis, as well as MUC4 high expression LUAD (33,34). Most SMGs were reported to be associated with cancer prognosis, however, the role of OR2L3 and C8orf44 in tumors has not been studied yet.
TMB was defined as the total number of somatic nonsynonymous mutations per megabases in tumor and usually used as a biomarker predicting effect of treatment (35). Compared with TCGA-LUAD cohort, the tumors in this study exhibited relatively low TMB level with a median TMB of 2.96 SNVs/Mb (Figure S2). The primary and recurrent tumors showed similar TMB level. The median TMB for primary and recurrence group were 3 SNVs/Mb and 2.94 SNVs/Mb, respectively (P=0.124, Table S2).
Genomic alterations influenced different pathways and gene networks in LUAD recurrence
Although the top mutated genes were similar between primary and recurrent tumors, the influenced pathways and gene networks were different. Mutational pathway analysis was performed and revealed several KEGG pathways of primary and recurrence group (Figure 4A). The p53 signaling pathway was the only pathway enriched in both groups. In primary tumors, mutated genes were more involved in pathways regulating RNA degradation and transcription. More diverse pathways were enriched in recurrent tumors than in primary tumors, of which, most pathways were critical and highly connected with tumor malignancy and prognosis, such as MAPK, ErbB, Wnt and cell cycle pathways.
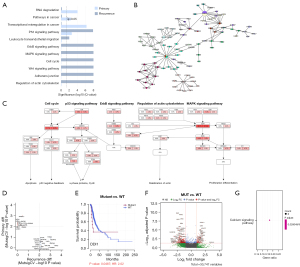
To further explore the functional interaction between coding-gene mutation of recurrence adenocarcinoma, gene-gene network analysis utilizing Hierarchical-Hotnet algorithm was performed to identify significantly mutated functional gene-gene subnetwork. Eleven KEGG pathways and 66 interactive genes were displayed (Figure 4B).
Five common pathways including MAPK, p53, ErbB signaling pathway, cell cycle, regulation of actin cytoskeleton were also identified in gene network analysis, indicating they may be the pivotal pathways contributing to LUAD recurrence (Figure 4C).
Identification of recurrence specifically significantly mutated genes
In this study, a randomization test strategy named MutSigCV (see Methods) was used to identify specific SMGs in primary and recurrent tumors. Twelve genes for primary group and 20 genes for recurrence group were identified (Figure 4D). For each gene, the relation between mutation status and patient recurrence-free survival (RFS) was analyzed based on TCGA-LUAD cohort. DDI1 mutation in the recurrence group, ARHGEF15 and OR52K2 mutation in the primary group were associated with worse RFS (Figure 4E; Figure S3A,S3B). DDI1 mutation was also correlated with chemotherapy resistance of esophageal squamous cancer (34). Besides, some primary group-specific genes, including CSTL1, HEY2, PBOV1, LEMD1, WNT1, were reported to be associated with tumor growth and progression in other tumors (34,36-41). Consistent with previous studies, the primary-specific SMGs were merely mutated in recurrent tumors, but the recurrence-specific SMGs were also frequently mutated in primary tumors (42). This finding indicates that some recurrence associated genomic alterations occurred in early stage of tumorigenesis.
In this study, MUC4 was frequently mutated in both primary and recurrent tumors, much more frequent than TCGA-LUAD cohort. MUC4 was a high-molecular-weight glycoprotein served as a barrier for some cell-cell and cell-extracellular matrix interactions and as a potential reservoir for certain growth factors. By comparing RNA-seq data between MUC4-mutated samples and MUC4 wild-type samples in TCGA-LUAD cohort, calcium signaling pathway was enriched with significantly upregulated expression of EGF and SLC8A2 (Figure 4F,4G). Calcium signaling pathway was highly connected with several pathways regulating cell proliferation and apoptosis, such as CAMK, PKC and ERK.
Clone analysis revealed similar tumor inter-heterogeneity between primary and recurrent LUAD
Based on sample somatic mutations (SNVs and indels) and CNVs, pyclone-vi was used to perform clonal analysis in primary and recurrent tumors. Clonality analysis showed high degree of tumor inter-heterogeneity (ITH) across all samples, varying from 1 to 8 clones (median: 4). Most patients have experienced significant process of clone substitution (Figure S4A). When compared with primary tumors, more clones were identified in recurrent tumors (median: 4), but the difference was not significant (P=0.101, Figure 5A). In this study, treatment status before recurrence didn’t influence LUAD clone numbers. The clone number between primary and recurrent tumors were not significantly different, neither in treated group (n=18) nor in untreated group (n=23) (Figure 5B). MATH score is a quantified indicator for ITH and high MATH score relates to worse prognosis. Primary and recurrence group had similar MATH score (Figure 5C), which indicated similar ITH level in both groups. Compared with TCGA-LUAD cohort, tumors in this study had a higher MATH score (Figure 5D, P=0.0221), which may result from the increased malignancy of recurrent LUAD in this study.
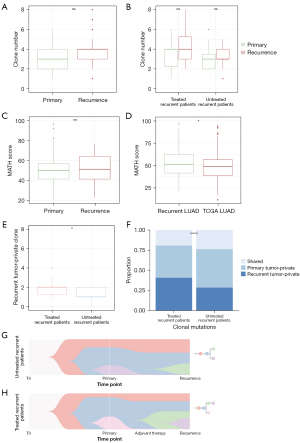
To explore the mutation evolution of LUAD progression, clone analysis was performed on SMGs in primary and recurrence groups. Although the SMGs were similar, the distribution of clone and subclone mutation for each SMG was different between two groups. The clone mutation proportion is 36.4% for primary group and 23.8% for subclone mutation (Figure S4B, P=0.0036). The percentage of subclone mutations significantly increased from primary tumors to recurrent tumors, consistent with previous reports.
Eighteen patients received adjuvant therapy before surgery for recurrent tumors. Adjuvant therapy increased the number of recurrent tumor-specific clone when compared with untreated group (Figure 5E, P=0.0371). Besides, the recurrent tumor-specific mutations also increased in adjuvant therapy group (Figure 5F, P<0.001). Although the clonal evolution patterns were different, new clones and subclone expansion were more likely to generate in adjuvant therapy group (Figure 5G,5H).
Discussion
Post-operative recurrence is the major death-relative cause of LUAD patients, and exploration of the underlying molecular mechanism would enhance clinicians’ ability to identify patients with high risk of recurrence and potential therapy biomarkers (43,44). In this study, more genomic alterations were detected in primary tumors, but more deletions were found in recurrent tumors. Chromosome 9p21.3 deletion occurred in a variety of tumors and was only detected in recurrent LUAD tumors (45). The correlated CNV loss of known TSGs (CDKN2A, CDKN2B and MTAP) in this region highly contributed to LUAD recurrence.
EGFR was the gene with the highest mutation rate in both primary and recurrent tumors. And a mutation shift between L858R and L861Q was observed in 2 patients (patient-23 and patient-34), which indicating a re-detection of genomic mutations was necessary during the treatment LUAD patients. Interestingly, MUC4 was the second most mutated gene in this study, with much higher mutation rate than TCGA-cohort. MUC4 mutations were reported to be associated with LUAD worse prognosis, mostly through interacting with ERBB2 and influencing the downstream pathways (31). In TCGA-LUAD samples, significant up-regulation of EGF and calcium signaling pathway activation were observed in MUC4 mutated tumors. MUC4 may be playing major roles in LUAD recurrence and is a potential therapeutic target which need further investigation.
Compared with primary tumors, recurrence-specific SMGs mainly affected MAPK pathway and ErbB signaling pathway. These pathways are highly correlated with EGFR mutation and EGF, and highly depend on Calcium-ion to activate key component proteins. Previous studies have discovered the hyper-activation of MAPK was associated with LUAD migration and invasion. These results again suggested MUC4 may involve in LUAD recurrence by regulating MAPK pathway, but more experiments are needed to verify this hypothesis.
Several limitations in this study need further exploration or could affect the accuracy of the results. Firstly, this retrospective study only enrolled patients with recurrence may cause selection bias. Secondly, DNA was extracted from FFPE samples which had lower coverage depth and more false mutations than frozen or fresh samples when used for WES. The sample selection bias and DNA quality may cause extra bias in mutation calling. Thirdly, only WES data was analyzed. Paired transcriptome or epigenetic data could help make deeper understanding of the biological changes of LUAD recurrence. Finally, potential targets were found in the current study but further verification with biology experiments is required.
Conclusions
WES was performed on paired primary and recurrent LUAD tumors to characterize the genomic alteration features. Interesting novel biomarkers such as MUC4 may play key roles in LUAD recurrence and maybe potential therapeutic targets. This study investigated the molecular mechanism of tumor recurrence and provided some new insights for further genomic research.
Acknowledgments
We acknowledged Yang Zhang for his support in the writing. We acknowledged Qiang Zheng and Xuxia Shen for their support in the material collection and pathological diagnosis. We also acknowledged all members of Pro. Meng’s lab for their general support in the data processing and analysis.
Funding: This work was supported by Chinese Minister of Science and Technology (Nos. 2017YFA0505501, 2018YFA0107602, and 2018YFA0800203), National Natural Science Foundation of China (No. 82172744), National Basic Research Program of China (No. 2020YFA0803300), Science and Technology Commission of Shanghai Municipality (No. 21Y11913700) and Beijing Xisike Clinical Oncology Research Foundation (No. Y-2019AZQN-0511).
Footnote
Reporting Checklist: The authors have completed the MDAR reporting checklist. Available at https://tlcr.amegroups.com/article/view/10.21037/tlcr-22-793/rc
Data Sharing Statement: Available at https://tlcr.amegroups.com/article/view/10.21037/tlcr-22-793/dss
Conflicts of Interest: All authors have completed the ICMJE uniform disclosure form (available at https://tlcr.amegroups.com/article/view/10.21037/tlcr-22-793/coif). The authors have no conflicts of interest to declare.
Ethical Statement: The authors are accountable for all aspects of the work in ensuring that questions related to the accuracy or integrity of any part of the work are appropriately investigated and resolved. The study was conducted in accordance with the Declaration of Helsinki (as revised in 2013). This study was approved by the Committee for Ethical Review of Research of Fudan University Shanghai Cancer Center Institutional Review Board (No. 090977-1). Informed consents for donating their samples to the tissue bank of Fudan University Shanghai Cancer Center were obtained from patients themselves or their relatives.
Open Access Statement: This is an Open Access article distributed in accordance with the Creative Commons Attribution-NonCommercial-NoDerivs 4.0 International License (CC BY-NC-ND 4.0), which permits the non-commercial replication and distribution of the article with the strict proviso that no changes or edits are made and the original work is properly cited (including links to both the formal publication through the relevant DOI and the license). See: https://creativecommons.org/licenses/by-nc-nd/4.0/.
References
- Siegel RL, Miller KD, Fuchs HE, et al. Cancer statistics, 2022. CA Cancer J Clin 2022;72:7-33. [Crossref] [PubMed]
- Thai AA, Solomon BJ, Sequist LV, et al. Lung cancer. Lancet 2021;398:535-54. [Crossref] [PubMed]
- Devarakonda S, Morgensztern D, Govindan R. Genomic alterations in lung adenocarcinoma. Lancet Oncol 2015;16:e342-51. [Crossref] [PubMed]
- Campbell JD, Alexandrov A, Kim J, et al. Distinct patterns of somatic genome alterations in lung adenocarcinomas and squamous cell carcinomas. Nat Genet 2016;48:607-16. [Crossref] [PubMed]
- Relli V, Trerotola M, Guerra E, et al. Abandoning the Notion of Non-Small Cell Lung Cancer. Trends Mol Med 2019;25:585-94. [Crossref] [PubMed]
- Wu YL, Tsuboi M, He J, et al. Osimertinib in Resected EGFR-Mutated Non-Small-Cell Lung Cancer. N Engl J Med 2020;383:1711-23. [Crossref] [PubMed]
- Uramoto H, Tanaka F. Recurrence after surgery in patients with NSCLC. Transl Lung Cancer Res 2014;3:242-9. [PubMed]
- Saynak M, Veeramachaneni NK, Hubbs JL, et al. Local failure after complete resection of N0-1 non-small cell lung cancer. Lung Cancer 2011;71:156-65. [Crossref] [PubMed]
- Hung JJ, Yeh YC, Jeng WJ, et al. Predictive value of the international association for the study of lung cancer/American Thoracic Society/European Respiratory Society classification of lung adenocarcinoma in tumor recurrence and patient survival. J Clin Oncol 2014;32:2357-64. [Crossref] [PubMed]
- Kasprzyk M, Sławiński G, Musik M, et al. Completion pneumonectomy and chemoradiotherapy as treatment options in local recurrence of non-small-cell lung cancer. Kardiochir Torakochirurgia Pol 2015;12:18-25. [Crossref] [PubMed]
- Rami-Porta R, Asamura H, Travis WD, et al. Lung cancer - major changes in the American Joint Committee on Cancer eighth edition cancer staging manual. CA Cancer J Clin 2017;67:138-55.
- Chen S, Zhou Y, Chen Y, et al. fastp: an ultra-fast all-in-one FASTQ preprocessor. Bioinformatics 2018;34:i884-90. [Crossref] [PubMed]
- Li H, Durbin R. Fast and accurate short read alignment with Burrows-Wheeler transform. Bioinformatics 2009;25:1754-60. [Crossref] [PubMed]
- Li H, Handsaker B, Wysoker A, et al. The Sequence Alignment/Map format and SAMtools. Bioinformatics 2009;25:2078-9. [Crossref] [PubMed]
- McKenna A, Hanna M, Banks E, et al. The Genome Analysis Toolkit: a MapReduce framework for analyzing next-generation DNA sequencing data. Genome Res 2010;20:1297-303. [Crossref] [PubMed]
- Cibulskis K, Lawrence MS, Carter SL, et al. Sensitive detection of somatic point mutations in impure and heterogeneous cancer samples. Nat Biotechnol 2013;31:213-9. [Crossref] [PubMed]
- Wang K, Li M, Hakonarson H. ANNOVAR: functional annotation of genetic variants from high-throughput sequencing data. Nucleic Acids Res 2010;38:e164. [Crossref] [PubMed]
- Forbes SA, Bindal N, Bamford S, et al. COSMIC: mining complete cancer genomes in the Catalogue of Somatic Mutations in Cancer. Nucleic Acids Res 2011;39:D945-50. [Crossref] [PubMed]
- Boeva V, Popova T, Bleakley K, et al. Control-FREEC: a tool for assessing copy number and allelic content using next-generation sequencing data. Bioinformatics 2012;28:423-5. [Crossref] [PubMed]
- Layer RM, Chiang C, Quinlan AR, et al. LUMPY: a probabilistic framework for structural variant discovery. Genome Biol 2014;15:R84. [Crossref] [PubMed]
- Mayakonda A, Lin DC, Assenov Y, et al. Maftools: efficient and comprehensive analysis of somatic variants in cancer. Genome Res 2018;28:1747-56. [Crossref] [PubMed]
- Shinde J, Bayard Q, Imbeaud S, et al. Palimpsest: an R package for studying mutational and structural variant signatures along clonal evolution in cancer. Bioinformatics 2018;34:3380-1. [Crossref] [PubMed]
- Lawrence MS, Stojanov P, Polak P, et al. Mutational heterogeneity in cancer and the search for new cancer-associated genes. Nature 2013;499:214-8. [Crossref] [PubMed]
- Mermel CH, Schumacher SE, Hill B, et al. GISTIC2.0 facilitates sensitive and confident localization of the targets of focal somatic copy-number alteration in human cancers. Genome Biol 2011;12:R41. [Crossref] [PubMed]
- Love MI, Huber W, Anders S. Moderated estimation of fold change and dispersion for RNA-seq data with DESeq2. Genome Biol 2014;15:550. [Crossref] [PubMed]
- Gonzalez-Perez A, Lopez-Bigas N. Functional impact bias reveals cancer drivers. Nucleic Acids Res 2012;40:e169. [Crossref] [PubMed]
- Reyna MA, Leiserson MDM, Raphael BJ. Hierarchical HotNet: identifying hierarchies of altered subnetworks. Bioinformatics 2018;34:i972-80. [Crossref] [PubMed]
- Gillis S, Roth A. PyClone-VI: scalable inference of clonal population structures using whole genome data. BMC Bioinformatics 2020;21:571. [Crossref] [PubMed]
- Imielinski M, Berger AH, Hammerman PS, et al. Mapping the hallmarks of lung adenocarcinoma with massively parallel sequencing. Cell 2012;150:1107-20. [Crossref] [PubMed]
- Comprehensive molecular profiling of lung adenocarcinoma. Nature 2014;511:543-50. [Crossref] [PubMed]
- Carraway KL, Perez A, Idris N, et al. Muc4/sialomucin complex, the intramembrane ErbB2 ligand, in cancer and epithelia: to protect and to survive. Prog Nucleic Acid Res Mol Biol 2002;71:149-85. [Crossref] [PubMed]
- Chaturvedi P, Singh AP, Chakraborty S, et al. MUC4 mucin interacts with and stabilizes the HER2 oncoprotein in human pancreatic cancer cells. Cancer Res 2008;68:2065-70. [Crossref] [PubMed]
- Rokutan-Kurata M, Yoshizawa A, Sumiyoshi S, et al. Lung Adenocarcinoma With MUC4 Expression Is Associated With Smoking Status, HER2 Protein Expression, and Poor Prognosis: Clinicopathologic Analysis of 338 Cases. Clin Lung Cancer 2017;18:e273-81. [Crossref] [PubMed]
- Jonckheere N, Van Seuningen I. Integrative analysis of the cancer genome atlas and cancer cell lines encyclopedia large-scale genomic databases: MUC4/MUC16/MUC20 signature is associated with poor survival in human carcinomas. J Transl Med 2018;16:259. [Crossref] [PubMed]
- Fancello L, Gandini S, Pelicci PG, et al. Tumor mutational burden quantification from targeted gene panels: major advancements and challenges. J Immunother Cancer 2019;7:183. [Crossref] [PubMed]
- Suo D, Wang L, Zeng T, et al. NRIP3 upregulation confers resistance to chemoradiotherapy in ESCC via RTF2 removal by accelerating ubiquitination and degradation of RTF2. Oncogenesis 2020;9:75. [Crossref] [PubMed]
- Miao TW, Du LY, Xiao W, et al. Identification of Survival-Associated Gene Signature in Lung Cancer Coexisting With COPD. Front Oncol 2021;11:600243. [Crossref] [PubMed]
- Hammouz RY, Kostanek JK, Dudzisz A, et al. Differential expression of lung adenocarcinoma transcriptome with signature of tobacco exposure. J Appl Genet 2020;61:421-37. [Crossref] [PubMed]
- Sasahira T, Kurihara M, Nakashima C, et al. LEM domain containing 1 promotes oral squamous cell carcinoma invasion and endothelial transmigration. Br J Cancer 2016;115:52-8. [Crossref] [PubMed]
- Pan T, Wu R, Liu B, et al. PBOV1 promotes prostate cancer proliferation by promoting G1/S transition. Onco Targets Ther 2016;9:787-95. [Crossref] [PubMed]
- Guo Y, Wu Z, Shen S, et al. Nanomedicines reveal how PBOV1 promotes hepatocellular carcinoma for effective gene therapy. Nat Commun 2018;9:3430. [Crossref] [PubMed]
- Hu Z, Li Z, Ma Z, et al. Multi-cancer analysis of clonality and the timing of systemic spread in paired primary tumors and metastases. Nat Genet 2020;52:701-8. [Crossref] [PubMed]
- Goldstraw P, Chansky K, Crowley J, et al. The IASLC Lung Cancer Staging Project: Proposals for Revision of the TNM Stage Groupings in the Forthcoming (Eighth) Edition of the TNM Classification for Lung Cancer. J Thorac Oncol 2016;11:39-51. [Crossref] [PubMed]
- Travis WD, Brambilla E, Noguchi M, et al. International association for the study of lung cancer/american thoracic society/european respiratory society international multidisciplinary classification of lung adenocarcinoma. J Thorac Oncol 2011;6:244-85. [Crossref] [PubMed]
- Solimini NL, Xu Q, Mermel CH, et al. Recurrent hemizygous deletions in cancers may optimize proliferative potential. Science 2012;337:104-9. [Crossref] [PubMed]