Radiomic signature accurately predicts the risk of metastatic dissemination in late-stage non-small cell lung cancer
Highlight box
Key findings
• PET/CT imaging is a routine procedure for radiotherapy planning in NSCLC. NSCLC is a highly metastatic cancer. Prediction of risk of metastasis and a time when metastatic appear is of high interest. Radiomics could be applied for predicting the risk of metastasis.
What is known and what is new?
• Radiomics was applied in the prediction of NSCLC survival before.
• Here, we applied a cohort of heterogenous NSCLC patients for metastasis risk prediction. We extracted a radiomics signature for risk prediction with high prediction performance (concordance index =0.84)
What is the implication, and what should change now?
• We can predict the risk of metastasis based on routinely collected PET/CT images from a primary tumor.
Introduction
Lung cancer is one of the most frequently diagnosed cancer types worldwide, constituting over 11% of all cancer cases. With 2.2 million new diagnoses in 2020 alone, it was surpassed in incidence only by breast cancer, making the lung the most prevalent cancer site in men (with over 1.43 million diagnoses) and the third most prevalent in women after breast and colorectal cancers, which had 0.77 million diagnoses (1,2). While tobacco smoking is recognized as the primary cause of lung cancer, it can also be attributed to environmental factors such as air pollution, occupational exposure, and genetic predisposition (3-5). It is usually diagnosed at an advanced stage due to non-specific early-stage symptoms, which is reflected in the very high mortality rate. Indeed, the five-year survival rate for lung cancer does not exceed 20% (6-8), thus, it is the leading cause of cancer-related mortality and is responsible for 18% of all deaths from cancer (1).
Diagnosis of lung cancer involves medical imaging, including X-ray and positron emission tomography/computed tomography (PET/CT), which allows for classification according to the tumor node metastasis (TNM) staging system. Detected lesions are sampled by endobronchial ultrasound (EBUS) guided bronchoscopy and undergo histopathological assessment. Management is stage-specific (8), with clinical guidelines divided into early-stage, locally advanced, and metastatic cancer (9,10). In early-stage lung cancer, lobectomy is the preferred treatment option. If the tumor is not initially resectable, neoadjuvant chemotherapy can be implemented to downgrade the tumor, which would eventually allow for surgery. For selected patients with comorbidities, stereotactic ablative radiotherapy (SABR) may also be considered. For locally advanced cancer with lymph node involvement, platinum-based chemotherapy administered concurrently or sequentially with radiotherapy (RT) is the most commonly used curative therapeutic option, and it can be followed by maintenance immunotherapy. For advanced metastatic cancer, immune checkpoint inhibitors, with or without chemotherapy, are a viable therapeutic option. As molecular diagnostics becomes routinely available, targeted therapies aimed at epidermal growth factor receptor (EGFR) (11), fibroblast growth factor receptor (FGFR) (12), anaplastic lymphoma kinase (ALK) (13), or Kirsten rat sarcoma virus (KRAS) (14) are being used to treat mutation carriers.
One of the main reasons for the high mortality seen in lung cancer is its invasiveness, and most patients develop distant metastases. Unfortunately, metastatic tumors are often resistant to treatment, which leads to much shorter survival times for these patients. Although the exact mechanisms of metastasis are still being investigated, it is known that cancer cells can spread by both blood and lymphatic vessels (15,16). Lung cancer metastases are most frequently observed in the brain, bones, liver, lung, and adrenal gland (16). Since the occurrence of distant metastasis is the turning point in the course of the disease, it might be considered an important endpoint in prognostic analysis, along with the standard endpoints. Furthermore, the ability to predict when lung cancer will metastasize could guide clinical decision-making and may be used to indicate the need for therapy intensification in high-risk patients.
The search for accurate prognostic biomarkers in lung cancer is hindered by its high heterogeneity and complexity. Nonetheless, clinical and molecular characteristics have shown some promise in predicting metastasis. Metastasis-associated lung adenocarcinoma transcript 1 (MALAT-1), a long non-coding ribonucleic acid (RNA), was demonstrated to be significantly associated with metastasis in non-small cell lung cancer (NSCLC) (8). Meanwhile, cancer antigen 125 (CA125) and neuron-specific enolase (NSE) were found to be indicative of liver metastasis (17).
The radiomics-based approach has been successfully applied for different endpoints in lung cancer, including overall survival (OS) and progression-free survival (PFS) (18). It has also shown promising results for the prediction of distant metastases. Coroller et al. (19) selected a radiomic signature based on CT images to predict distant metastasis in lung adenocarcinoma. Wu et al. constructed and validated a Cox proportional hazards model using 18F-fluorodeoxyglucose PET (18F-FDG PET) imaging to predict freedom of distant metastasis in early-stage NSCLC patients (20). Fave et al. (21) demonstrated that adding pre-treatment radiomic features extracted from CT images could improve the ability of clinical prognostic models to predict distant metastasis (21). Meanwhile, Dou et al. (22) focused on locally advanced lung adenocarcinoma and investigated radiomic features from the primary tumor and peritumoral region (22).
In this study, 115 NSCLC patients with various histological subtypes were retrospectively analyzed. The prognostic value of standard clinical features, and radiomic features extracted from PET/CT images acquired for RT planning, were evaluated by determining if they could be used to predict time to distant metastasis. To answer this question, machine learning models were constructed for continuous and categorical metastasis-free survival (MFS) prediction. We present this article in accordance with the TRIPOD reporting checklist (available at https://tlcr.amegroups.com/article/view/10.21037/tlcr-23-60/rc).
Methods
Study design
A cohort of NSCLC patients was collated to investigate if PET/CT imaging routinely performed for RT planning could help in planning the future treatment strategy, with a focus on predicting the risk and time of relapse with distant metastases. MFS was defined as the time elapsed between diagnosis and the detection of distant metastasis or the time of death/last follow-up if distant metastases did not emerge. In addition, classification algorithms were used to predict if MFS would be short or long.
As the prediction of metastasis risk was the focus of the study, the primary lung cancer tumor was the region of interest (ROI). Using the available PET/CT scans, radiomic features were extracted from the ROI and assessed.
The specific clinical question considered in this work was whether or not a radiomic signature could be extracted that would help discriminate between a primary tumor that has the potential to metastasize early from one that metastasizes late or not at all.
The pipeline of our method is presented in Figure 1. The method includes processing the clinical and PET/CT data and applying them as predictors (features) in the calibration of survival models (Cox regression, random survival forest) and perform classification.
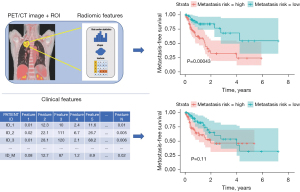
Study population
Data were collected retrospectively at the Maria Sklodowska-Curie National Research Institute of Oncology, Gliwice Branch (NRIO). The cohort consisted of 115 patients with NSCLC who were treated with curative intent at the Institute between 2009 and 2017. All patients in the cohort had been treated with a combination of chemotherapy and RT. Most of the patients received a platinum-based doublet with vinorelbine. Patients received between one and six cycles (median four), followed by RT with a total dose between 60 and 70 Gray (Gy) in two Gy fractions. The study was conducted in accordance with the Declaration of Helsinki (as revised in 2013). The study was approved by the institutional review board of Maria Sklodowska-Curie National Research Institute of Oncology (Gliwice Branch) (No. KB/430-48/23) and individual consent for this retrospective analysis was waived. The clinical data were anonymized before the computational analysis.
All patients underwent PET/CT imaging for RT planning. Only patients with non-detectable distant tumors at the onset of treatment were assessed. However, most patients had locally disseminated tumors to the lymph nodes, as they were diagnosed late due to non-specific symptoms.
In the cohort, 72.2% of patients were males, and 27.8% were female. This is consistent with population data showing that most lung cancer patients are male. The median age of patients in the cohort was 61 years, and over half of the patients had tumors located in the left lung. The most prevalent cancer subtype was squamous cell carcinoma, which constituted two-thirds of all cases, followed by not otherwise specified (24.3%) and adenocarcinoma (7.0%). Detailed characteristics of the cohort are presented in Table 1.
Table 1
Characteristics | Subtypes | Patients (n=115) |
---|---|---|
Sex, n (%) | Male | 83 (72.2) |
Female | 32 (27.8) | |
Age (years) | Median [Q1–Q3] | 61 [57–67] |
Histopathology, n (%) | Squamous | 77 (67.0) |
Adenocarcinoma | 8 (7.0) | |
Not otherwise specified | 28 (24.3) | |
Large cell | 2 (1.7) | |
Location, n (%) | Left | 65 (56.5) |
Right | 50 (43.5) | |
T, n (%) | 1 | 4 (3.5) |
2 | 37 (32.2) | |
3 | 37 (32.2) | |
4 | 37 (32.2) | |
N, n (%) | 0 | 19 (16.5) |
1 | 6 (5.2) | |
2 | 83 (72.2) | |
3 | 7 (6.1) | |
M, n (%) | 0 | 115 (100.0) |
1 | 0 (0) | |
Zubrod score, n (%) | 0 | 34 (29.6) |
1 | 80 (69.6) | |
2 | 1 (0.9) |
Extraction of radiomic features
Feature extraction was performed with PyRadiomics version 3.0.1, a Python package designed to increase the reproducibility of radiomic studies (23). Using the PET dataset, 105 standard features were calculated. Radiomic features belong to one of three classes, including first-order statistics such as energy, entropy, and minimum, as well as shape features such as volume, surface area, and sphericity, and texture features including gray level co-occurrence matrix (GLCM), gray level dependence matrix (GLDM), gray level run length matrix (GLRLM), gray level size zone matrix (GLSZM), and neighboring-gray tone difference matrix (NGTDM).
MFS categorization
For the classification, a threshold of one year was used to create two classes, which included patients with MFS below and over this threshold. Due to the presence of censored observations (in the cohort this primarily signified the patient’s death), such stratification divided patients into a group who suffered either metastasis or death within a year (66 patients), and those who did not (49 patients). To create subgroups that were more related to the research question, the binary MFS was defined as “short” if the patient developed metastasis within a year (25 patients) and “long” if the patient developed metastasis or was censored after longer than a year (49 patients).
Statistical analysis
Statistical analysis was performed using the R environment (version 4.1.3). For survival analysis, survival (version 3.2-13) was used, caret (version 6.0.93) and RandomForest (version 4.7-1.1) were used for classification, and randomForestSRC (version 3.1.1) was used to perform random survival forest. A heatmap of the radiomic features was created with ComplexHeatmap (version 2.10.0).
Filtering of the radiomic features was applied based on the Pearson correlation coefficient to avoid redundancy, with a cutoff threshold equal to 0.9 (see Table S1). Since the PET images were acquired using two scanners, principal component analysis was applied to determine if there was any grouping of samples due to the scanner used (see Figure S1).
The clinical and radiomic features with potential for event-free survival (EFS) and MFS prediction were assessed (see Table S2). In addition, differences in the values of radiomic and clinical features between ‘short’ and ‘long’ MFS patient subgroups were investigated statistically. Fisher’s exact test was performed for categorical variables, while the Mann-Whitney U test was used for continuous variables (see Table S3). A log-rank test was also conducted for both categorical and continuous features (see Table S4). As the log-rank test assesses if there is a significant difference between two or more survival curves, continuous features were binarized with respect to the median value.
Cross-validation
The value of any type of predictive model lies in its applicability to unknown data, and not just its ability to fit the training data. Cross-validation enables evaluation of the model’s ability to generalize by removing part of the data from the cohort and applying them in the estimation of model performance. In addition, data partitioning at the beginning of each iteration prevents information leakage.
For a more consistent comparison between the regression and classification results, modified k-fold data partitioning was applied. Firstly, the data was ordered according to (continuous) MFS values. Then, the observations were assigned consecutive numbers, from one to five, which were used as cross-validation folds. Such partitioning ensures proper stratification of both continuous and binarized MFS.
Classification algorithms
The observed relationships between binary MFS and binary EFS and extracted features (both clinical radiomic) were verified by employing classification models. Firstly, three main feature selection methods were applied, including Student’s t-test, Wilcoxon test, and a mutual information test. To investigate the impact of a varying number of features on classification quality, between 1 and 10 features were tested. Since only the mutual information method handles both categorical and continuous variables, a hybrid selection was used for the other two methods by applying the main method for continuous variables and Fisher’s exact test for categorical variables. The categorical variables that passed the significance threshold equal to 0.1 were added to the model.
The following classification methods were tested: K nearest neighbor (KNN) with different K values (for clarity, only the best one, K=5, is presented), random forest, support vector machines (SVM) with linear and radial kernels, and logistic regression (LogReg). Considering the inconsistent orders of magnitude for radiomic features, a z-score transformation was used to scale the data. In each k-fold iteration, the scaling parameters (mean and standard deviation) were determined from the training set and applied to both the training and test sets. Classification accuracy was then used to assess model performance.
Regression algorithms
For the prediction of continuous MFS, Cox proportional hazards regression (using survival R package) and random survival forest (using randomForestSRC R package) were applied. Variable selection was performed based on univariate analysis, with the Harrell concordance index (C-index) adopted as a ranking metric. The model performance was validated using the k-fold partitioning described above. Again, models containing between 1 and 10 features were tested.
Radiomic-based risk score
Although cross-validation facilitates the estimation of prediction quality, the results and selected features can be different in each iteration due to subsampling. Therefore, all selections were repeated on the entire dataset to obtain conclusive feature rankings. To demonstrate the validity of the obtained signature, the Cox model was chosen, which is the classic approach to survival data analysis with known interpretation. The patients were then divided into high-risk and low-risk groups based on the calculated median risk score, and MFS was compared using Kaplan-Meier curves.
Results
Patient characteristics
The cohort included only NSCLC patients, as it is the most common type of lung cancer. Most patients (67%) had squamous histopathological subtypes, and almost two-thirds had an advanced stage of the primary tumor (T3 or T4). In total, 37 patients eventually developed distant metastases. Figure 2 shows the radiomics features of a whole cohort and the time-to-metastasis as a survival curve. The median time-to-metastasis was 2.77 years, with a secondary tumor observed most frequently in the second lung, brain, bones, and liver.
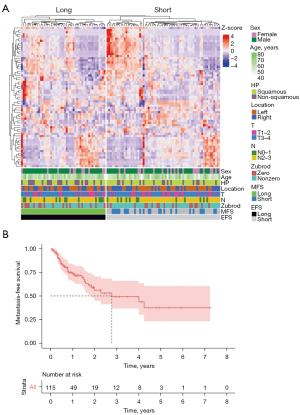
None of the clinical features of the cohort were informative in relation to the time to metastasis onset (Table S2). This means that clinicians are unable to predict if a particular patient will develop metastatic cancer, based only on clinical variables at diagnosis. On the other hand, 34 radiomic features were statistically significant against continuous MFS, 36 features against the binarized MFS, and 18 against EFS.
PET/CT data acquisition and segmentation
The PET/CT images were acquired at the NRIO using Philips GeminiGXL 16 (Philips, Amsterdam, Netherlands) (24 patients) and Siemens Biograph mCT 131 (Siemens AG, Munich, Germany) (88 patients) PET/CT scanners. For each patient, the ROI was contoured by the same experienced nuclear medicine specialist using Medical Image Merge (MIM) 7.0.1 software and the PET Edge™ tool (both MIM Software Inc., OH, USA).
Integration of clinical and radiomic data
Figure 2A shows the normalized z-score values of radiomic features for each patient. The patients were divided into short and long EFS groups. As can be seen from the results, the hierarchical clustering correctly divided patients into these two groups. Furthermore, it was observed that the radiomic feature spectrum varied between patients with short and long EFS. This demonstrates that there is potential for the use of radiomic features in predicting EFS.
We also investigated the correlation between radiomic features. High correlations were observed between the radiomic features, which resulted in only 65 of 105 features passing the initial correlation filtering (Figure S2). The highest redundancy was found for the first-order features (6 out of 18 were kept) and the lowest for the GLSZM features (15 out of 16 were kept) (see Table S1).
Classification of advanced NSCLC
As expected, no clinical features were selected by the models. The feature rankings obtained for EFS (Figure 3) and MFS (Figure 4) prediction differed, which aligns with the different interpretations of these endpoints. While the rankings varied with respect to feature selection and classification methods, there was some consistency among the top features. Indeed, TotalEnergy, ZoneEntropy, and RootMeanSquared favored EFS prediction, while Variance, TotalEnergy, RunLengthNonUniformity (GLRLM), SizeZoneNonUniformityNormalized, and Maximum2DDiameterColumn favored MFS prediction.
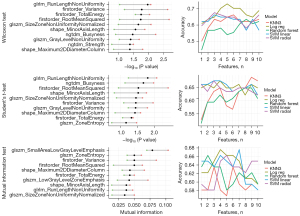
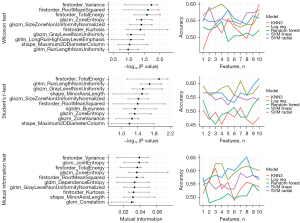
The highest accuracy for EFS prediction (approx. 0.65) was achieved using the SVM classifier with linear kernels for the mutual information selection of eight features. The highest accuracy for MFS prediction (approx. 0.73) was achieved using the LogReg classifier for the five features selected using the Wilcoxon test. Due to the imbalanced classes, with “long” (treated as the negative class) being the predominant group, the models for MFS tended to yield high specificity and relatively low sensitivity. Most models performed better for a small number of features.
Prediction of risk of metastasis
For regression-based models, the tendency was similar, with the highest predictive ability observed for a small number of features. The highest median C-index across folds was reached for two features (GLRLM and NGTDM Business) in Cox regression and one feature (shapeMinorAxisLength) in the random survival forest (Figure 5).
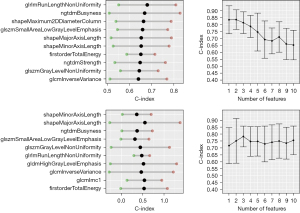
The mean C-index for the best set of features using Cox regression was 0.84, whereas the C-index for the random survival forest was 0.8. The inclusion of more features in the model resulted in a loss of prediction quality due to overfitting. No clinical features were selected for the best models, which is consistent with the preliminary patient cohort analysis.
Feature selection on the entire dataset revealed that the two top features for Cox regression, SmallAreaLowGrayLevelEmphasis (GLSZM) and RunLengthNonUniformity (GLRLM), also held high-ranking positions in the classification approach. Also, univariate analysis shows that those two features could discriminate high risk from low-risk patients (Figures S3,S4). Therefore, the Cox model was constructed using those two features. The high-risk and low-risk groups (Figure 6) had significantly different MFS, with the log-rank test P<0.001.
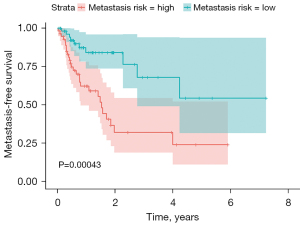
Discussion
Lung cancer is the leading cause of cancer-related death worldwide, claiming over 1.7 million lives yearly. It is characterized by high invasiveness, and the occurrence of distant spread significantly influences survival and treatment options. This necessitates the search for prognostic biomarkers that could help determine the time to metastasis onset. With the rapid development of the radiomics field, researchers have turned to medical imaging, which is routinely performed and non-invasive, as a source of information that could shed some light on the tumor dissemination process and aid clinicians in therapy planning.
A cohort of NSCLC patients with different subtypes and stages of the disease was collated. It was concluded that the standard clinical data available for the patients, except for higher metastatic potential exhibited by the squamous subtype, were largely uninformative regarding metastasis occurrence. To assess the potential of radiomics for MFS prediction, we extracted 105 radiomic features from PET/CT scans, using the primary tumor as the ROI.
Correlations between radiomic and clinical features were mostly low, signifying that both datasets carried independent information. Also, the hierarchical clustering of radiomic features did not correspond to any discernible grouping of clinical features (see Figure 2).
Regression and machine learning methods were then used to select radiomic signatures that could predict the risk of metastasis and achieved a C-index of 0.84 for the Cox proportional hazards model and 0.8 for the random survival forest, and an accuracy of 0.72 for the KNN classifier. These results confirm that medical images contain information that could be successfully applied to MFS prediction.
Several studies have shown the potential of radiomic features in predicting distant metastasis in lung cancer, with most of them focusing on either a particular subtype or stage (24,25). Coroller et al. (19) investigated radiomic features extracted from CT images for predicting distant metastasis in lung adenocarcinoma, which had a C-index of 0.61 on an independent validation set. Fave et al. (21) demonstrated that combining pre-treatment radiomic features with clinical information improved the ability of prognostic models to predict distant metastasis in stage III NSCLC patients, reporting a C-index of 0.63 (19). Wu et al. used features extracted from PET images to predict the freedom of distant metastasis, with a high C-index of 0.71 in independent validation (20). However, this work only focused on early-stage lung cancer. Dou et al. (22) presented an interesting approach, extracting features from both the tumor and tumor rim and achieving a C-index of 0.64 in a cohort of patients with locally advanced lung adenocarcinoma (22). In the current work, significantly better model quality was achieved in a cohort including patients with varying subtypes (squamous cell carcinoma, adenocarcinoma, large cell carcinoma) and stages.
While a regression approach, such as a Cox proportional hazards model, is typically used for survival-type analysis, the risk score it yields does not directly translate to the time of event occurrence. The C-index only compares pairs of observations, resulting in a global assessment of whether a higher risk is related to a shorter time-to-event. Therefore, classification was also performed and achieved an accuracy of 0.72 in cross-validation.
After testing several methods and approaches to variable selection, it was observed that similar predictive ability could be achieved for different feature sets, which indicates that even unrelated radiomic features carry equivalent information. Interestingly, the quality dropped drastically with increased feature numbers in all models. This suggests that features with high predictive potential perform much worse when combined than when used in isolation, and emphasizes the importance of selecting algorithms that are sensitive to feature interactions.
Certain variables retained high positions across different selections. These included GLRLM RunLengthNonUniformity, NGTDM Strength, and NGTDM Business. This demonstrates that these radiomic features are important for predicting if and when metastasis will occur in a lung cancer patient.
This analysis was not without limitations. While the study design ensured all images were contoured by one expert, which prevented bias, this did not allow for an assessment of the reproducibility of radiomic feature extraction. In addition, plans are in place to collect an independent patient cohort to validate the signature. Future work will also investigate tumor growth and dissemination dynamics, to achieve more clinically meaningful predictions.
Conclusions
Based on a cohort comprising 115 NSCLC patients, clinical features routinely collected during diagnostic procedures are not sufficient for the prediction of the risk of metastasis. Medical images (PET/CT scans) were investigated as a potential source of prognostic markers by assessing radiomic features in various classes of predictive models. A model based on two texture features (GLSZM and GLRLM) was constructed, which divided the patient cohort into low-risk and high-risk groups that significantly differed in MFS. The findings of this study have the potential to help clinicians make adjustments to therapy and create a rational basis for the intensification of systemic treatment in high-risk lung cancer patients.
Acknowledgments
Funding: This work was supported by the Polish National Science Centre (No. UMO-2020/37/B/ST6/01959). Calculations were performed on the Ziemowit computer cluster in the Laboratory of Bioinformatics and Computational Biology, created in the EU Innovative Economy Programme POIG.02.01.00-00-166/08 and expanded in the POIG.02.03.01-00-040/13 project. Data analysis was partially carried out using the Biotest Platform developed within project PBS3/B3/32/2015, which was financed by the Polish National Centre of Research and Development (NCBiR). This work was carried out in part by the Silesian University of Technology internal research funding (to K.F., J.S.). The funders have no role in designing the study and writing the manuscript.
Footnote
Reporting Checklist: The authors have completed the TRIPOD reporting checklist. Available at https://tlcr.amegroups.com/article/view/10.21037/tlcr-23-60/rc
Data Sharing Statement: Available at https://tlcr.amegroups.com/article/view/10.21037/tlcr-23-60/dss
Peer Review File: Available at https://tlcr.amegroups.com/article/view/10.21037/tlcr-23-60/prf
Conflicts of Interest: All authors have completed the ICMJE uniform disclosure form (available at https://tlcr.amegroups.com/article/view/10.21037/tlcr-23-60/coif). The authors have no conflicts of interest to declare.
Ethical Statement: The authors are accountable for all aspects of the work and will ensure that questions related to the accuracy or integrity of any part of the work are appropriately investigated and resolved. The study was conducted in accordance with the Declaration of Helsinki (as revised in 2013). The study was approved by the institutional review board of Maria Sklodowska-Curie National Research Institute of Oncology (Gliwice Branch) (No. KB/430-48/23), and individual consent for this retrospective analysis was waived.
Open Access Statement: This is an Open Access article distributed in accordance with the Creative Commons Attribution-NonCommercial-NoDerivs 4.0 International License (CC BY-NC-ND 4.0), which permits the non-commercial replication and distribution of the article with the strict proviso that no changes or edits are made and the original work is properly cited (including links to both the formal publication through the relevant DOI and the license). See: https://creativecommons.org/licenses/by-nc-nd/4.0/.
References
- Malvezzi M, Santucci C, Boffetta P, et al. European cancer mortality predictions for the year 2023 with focus on lung cancer. Ann Oncol 2023;34:410-9. [Crossref] [PubMed]
- Sung H, Ferlay J, Siegel RL, et al. Global Cancer Statistics 2020: GLOBOCAN Estimates of Incidence and Mortality Worldwide for 36 Cancers in 185 Countries. CA Cancer J Clin 2021;71:209-49. [Crossref] [PubMed]
- Alberg AJ, Samet JM. Epidemiology of lung cancer. Chest 2003;123:21S-49S. [Crossref] [PubMed]
- Dela Cruz CS, Tanoue LT, Matthay RA. Lung cancer: epidemiology, etiology, and prevention. Clin Chest Med 2011;32:605-44. [Crossref] [PubMed]
- Barta JA, Powell CA, Wisnivesky JP. Global Epidemiology of Lung Cancer. Ann Glob Health 2019;85:8. [Crossref] [PubMed]
- Lu T, Yang X, Huang Y, et al. Trends in the incidence, treatment, and survival of patients with lung cancer in the last four decades. Cancer Manag Res 2019;11:943-53. [Crossref] [PubMed]
- Torre LA, Siegel RL, Jemal A. Lung Cancer Statistics. Adv Exp Med Biol 2016;893:1-19. [Crossref] [PubMed]
- Molina JR, Yang P, Cassivi SD, et al. Non-small cell lung cancer: epidemiology, risk factors, treatment, and survivorship. Mayo Clin Proc 2008;83:584-94. [Crossref] [PubMed]
- Planchard D, Popat S, Kerr K, et al. Metastatic non-small cell lung cancer: ESMO Clinical Practice Guidelines for diagnosis, treatment and follow-up. Ann Oncol 2018;29:iv192-237. [Crossref] [PubMed]
- Postmus PE, Kerr KM, Oudkerk M, et al. Early and locally advanced non-small-cell lung cancer (NSCLC): ESMO Clinical Practice Guidelines for diagnosis, treatment and follow-up. Ann Oncol 2017;28:iv1-iv21. [Crossref] [PubMed]
- Le T, Gerber DE. Newer-Generation EGFR Inhibitors in Lung Cancer: How Are They Best Used? Cancers (Basel) 2019;11:366. [Crossref] [PubMed]
- Zhou Z, Liu Z, Ou Q, et al. Targeting FGFR in non-small cell lung cancer: implications from the landscape of clinically actionable aberrations of FGFR kinases. Cancer Biol Med 2021; Epub ahead of print. [Crossref] [PubMed]
- Yuan M, Huang LL, Chen JH, et al. The emerging treatment landscape of targeted therapy in non-small-cell lung cancer. Signal Transduct Target Ther 2019;4:61. [Crossref] [PubMed]
- Salgia R, Pharaon R, Mambetsariev I, et al. The improbable targeted therapy: KRAS as an emerging target in non-small cell lung cancer (NSCLC). Cell Rep Med 2021;2:100186. [Crossref] [PubMed]
- Popper HH. Progression and metastasis of lung cancer. Cancer Metastasis Rev 2016;35:75-91. [Crossref] [PubMed]
- Zhu T, Bao X, Chen M, et al. Mechanisms and Future of Non-Small Cell Lung Cancer Metastasis. Front Oncol 2020;10:585284. [Crossref] [PubMed]
- Wang CF, Peng SJ, Liu RQ, et al. The Combination of CA125 and NSE Is Useful for Predicting Liver Metastasis of Lung Cancer. Dis Markers 2020;2020:8850873. [Crossref] [PubMed]
- Zhang Y, Oikonomou A, Wong A, et al. Radiomics-based Prognosis Analysis for Non-Small Cell Lung Cancer. Sci Rep 2017;7:46349. [Crossref] [PubMed]
- Coroller TP, Grossmann P, Hou Y, et al. CT-based radiomic signature predicts distant metastasis in lung adenocarcinoma. Radiother Oncol 2015;114:345-50. [Crossref] [PubMed]
- Wu J, Aguilera T, Shultz D, et al. Early-Stage Non-Small Cell Lung Cancer: Quantitative Imaging Characteristics of (18)F Fluorodeoxyglucose PET/CT Allow Prediction of Distant Metastasis. Radiology 2016;281:270-8. [Crossref] [PubMed]
- Fave X, Zhang L, Yang J, et al. Delta-radiomics features for the prediction of patient outcomes in non-small cell lung cancer. Sci Rep 2017;7:588. [Crossref] [PubMed]
- Dou TH, Coroller TP, van Griethuysen JJM, et al. Peritumoral radiomics features predict distant metastasis in locally advanced NSCLC. PLoS One 2018;13:e0206108. [Crossref] [PubMed]
- van Griethuysen JJM, Fedorov A, Parmar C, et al. Computational Radiomics System to Decode the Radiographic Phenotype. Cancer Res 2017;77:e104-7. [Crossref] [PubMed]
- Liu RS, Ye J, Yu Y, et al. The predictive accuracy of CT radiomics combined with machine learning in predicting the invasiveness of small nodular lung adenocarcinoma. Transl Lung Cancer Res 2023;12:530-46. [Crossref] [PubMed]
- Li J, Zhang B, Ge S, et al. Prognostic value of (18)F-FDG PET/CT radiomic model based on primary tumor in patients with non-small cell lung cancer: A large single-center cohort study. Front Oncol 2022;12:1047905. [Crossref] [PubMed]