Review of the use of radiomics to assess the risk of recurrence in early-stage non-small cell lung cancer
Introduction
Radiomics applies advanced image analysis to extract detailed information from high-data content medical imaging scans [e.g., computed tomography (CT), magnetic resonance imaging (MRI), and positron emission tomography (PET). In oncology, radiomic features can be developed as imaging biomarkers to potentially capture a variety of tumor and disease-related radiologic-based characteristics. These signatures can then be used for theranostic, biologic, or pathologic purposes. Most radiomic-based investigations require the use of specialized software to mine high-content information followed by relatively intense mathematical modeling using distinct algorithms and/or statistical and AI/ML (artificial intelligence/machine learning) learning approaches to derive an imaging signature(s). This has resulted in the development of several open source and “black-box” methods for both research and commercial purposes. As expected, there is a lack of uniform methodology and independent validation. Despite these shortcomings radiomics continues to hold promise by offering either unique and/or supplemental information regarding the diseased state beyond the traditional evaluation of cancer by tumor stage, histology, and other clinical features.
Non-small cell lung cancer (NSCLC) is one such tumor type in which radiomic signatures have shown the potential to predict clinical outcomes and prognosis (Table 1). While the conventional methods of tumor staging, histology, and mutational characterization remain the mainstay in clinical decision making, newer methods (e.g., assessment of circulating tumor DNA) are beginning to influence treatment recommendations. In addition, the endorsement of low-dose CT for the early detection of lung cancer in high-risk individuals and the commercial availability of Laboratory Developed Tests has the potential to identify NSCLC patients at an early stage of diagnosis. Such efforts have begun to provide an opportunity to offer surgery at centers that promote their usage. What remains unknown is which of these patients would benefit from surgical resection alone vs. neoadjuvant therapy or require adjuvant therapy. Since all patients with NSCLC will undergo at least a pre-surgical standard-of-care CT scan (and/or PET at certain centers), the radiomic analysis of this patient population makes it feasible to discover, validate, and deploy specific radiomic signatures that may risk-stratify patient(s) for clinical decision-making purposes. Although NSCLC radiomics studies have found interesting radiomic signatures, most studies use either anecdotal and/or small retrospective data sets that may not be generalizable to the broader patient population. Therefore, is not surprising that a universal radiomic signature for NSCLC prognosis has yet to be found nor has there been a prospective, randomized control trial(s) to formally test any of the defined radiomic signatures.
Table 1
Risk stratification scenarios for radiomics in NSCLC |
• Distinguishing benign from malignant disease |
• Determining biologic drivers of tumor histology (e.g., tumor mutation status, tumor mutational burden, etc.) |
• Assessing the risk of nodal and metastatic involvement in patients without advanced disease on imaging |
• Predicting treatment response to a variety of regimens (i.e., chemoradiation, targeted therapy, immune therapy, and other rational combinations |
• Evaluating prognosis (e.g., recurrence-free survival, progression-free survival, overall survival) |
NSCLC, non-small cell lung cancer.
In this article, an outline of the essential elements that are required for radiomic signature(s) development is presented. This is followed by a literature review focusing on radiomics to potentially determine disease recurrence in the early-stage NSCLC setting. The emphasis on this particular population is especially relevant because of the growing use of screening methods for early detection in high-risk individuals. However, the early detection of NSCLC has begun to raise several questions regarding the risk/benefit of additional treatments (either neoadjuvant or adjuvant therapy) versus active surveillance in which radiomics may have a role to play. Finally, the future of radiomics in NSCLC will be discussed in the context of its use for patient care. This article is presented in accordance with the Narrative Review reporting checklist (available at https://tlcr.amegroups.com/article/view/10.21037/tlcr-23-5/rc).
Methods
A search of the PubMed database using the following terms was conducted by one of the authors (AL). The key inclusion and exclusion terms used is included in Table S1. Each resulting paper was then reviewed for appropriateness based upon study population, patient number (n>50), use of well described radiomic methodologies, suitable model building features, and well-defined testing/training and validation when feasible. Final inclusion of an article for review was determined in consensus by the three authors. A total of 41 articles were identified (Table S2), and 13 studies were considered suitable for inclusion in this review based upon the process (Table 2).
Table 2
Study | Population | Data sets | Survival endpoint | Modality | Segmentation method | Methodology for radiomic feature extraction | Radiomic models | Features associated with endpoints | High level radiomic features | Reference |
---|---|---|---|---|---|---|---|---|---|---|
1 | N=217; AC =41% | S:I | RFS | CECT | Semiautomated for tumor and automated for peritumoral region | PyRadiomics | Nearest neighborhood analysis, SVM, RF | 13 intratumoral features including two 1st order, eight 2nd order and three shape features. Nine peritumoral features including 5 1st order and 4 2nd order features. For tumors <5 cm peritumoral and combined intra/peritumoral features outperformed the intratumoral radiomic features. For tumors >5 cm intratumoral radiomic features outperformed peritumoral and combined radiomic features | Shape, 1st, 2nd order | (1) |
Stage I–IIIA (6th and 7th) | ||||||||||
Age =73 years | ||||||||||
Males =98% | ||||||||||
2 | N=461 (268 primary; 193 validation); AC =100% | S:I | RFS; OS | Non-CECT | Semi-automated; tumor and peritumoral region | PyRadiomics | GLM, Naïve Bayes, RF, and SVM | 5 radiomic features predicted MP vs. So patterns and outcomes including Shape_Surface volume ratio, 1st order_Root mean squared GLRLM_Run entropy, GLCM_Joint average and GLSZM_Zone entropy | Shape, 1st and 2nd order | (2) |
Stage = I–IIA (8th) | ||||||||||
Age =61 years (P); 60 (V) | ||||||||||
Males =44% (P); 48% (V) | ||||||||||
3 | N=378; AC =94% | S:I | RFS | CECT | Manual: tumor | PyRadiomics | LASSO with l tuning; Cox proportional hazard model, KM analysis for RFS | 8 radiomic features predicting outcomes including entropy, sphericity, maximum 2D diameter row, gray level non-uniformity normalized, long run low gray level emphasis, low gray level run emphasis, small area emphasis and small dependence emphasis | 1st and 2nd order | (3) |
Stage = IA and 1B (8th) | ||||||||||
Age ≤65 years (71%) | ||||||||||
Males =68% | ||||||||||
4 | N=592; AC =83% | S:I | RFS | CECT | Manual: tumor, and automated for peritumoral region | PyRadiomics | LASSO; X-tile | Multiple features (18 Total) including eight intratumoral 3D ROI features, five intratumoral 2D ROI features, five peritumoral features: Shape, first order, GLCM and GLSZM being top 5 features | Shape, 1st and 2nd order | (4) |
Stage = IA (7th) of So | ||||||||||
Age =61 years (O) | ||||||||||
Males =55% (O) | ||||||||||
5 | N=200 (100 Tr, 50 internal V, 50 external V); AC =100% | S:I | PFS | CECT | Semiautomated: tumor | Radiomics (v1.2.3 Siemens Healthineers) based upon PyRadiomics | Rad-score using RF; LASSO; KM curve with log-rank test and univariate Cox proportional-hazards model for outcomes; Harrell’s concordance index (C-index) for predicting outcomes; DCA for determining clinical benefit of the Rad-score | 20 Radiomic features contributed to Rad-Score; Top 5 performing features include Exponential_gldm_DependenceEntropy, Log_Sigma_0.5 mm 3D_GLCM_IDM, Log_1st Order_mean, Wavelet LLL_GLSZM Large area High gray level emphasis, Wavelet LLH GLCM_Inverse Variance | 1st and 2nd order, Wavelet | (5) |
Stage = I–IV (8th) | ||||||||||
Age =62, 61, 60 years, respectively | ||||||||||
Males =52%, 54%, 58%, respectively | ||||||||||
6 | N=1,058 (754 Tr; 304 V); AC =100% | S:I | DFS, OS | CECT | Semiautomated: tumor | PyRadiomics | Rad-score: LASSO; KM curve with log-rank test and univariate Cox proportional-hazards model for outcome | Two radiomic features were common predictors of DFS and OS: shape_SurfaceVolumeRatio, first order_90 Percentile | Shape and 1st order | (6) |
Stage = I–IIIA (7th) | ||||||||||
Age =63 years | ||||||||||
Males =49%, 51%, respectively | ||||||||||
7 | N=422; AC =12% | M: Lung datasets from Maastro (The Netherlands) | OS | CECT | Manual tumor | Matlab, NOS | Harrell’s concordance index (C-index) for performance and stability; multivariate Cox proportional hazards regression model for prediction of survival | Four radiomic features for prognosis includes statistics energy, shape compactness shape compactness, grey level nonuniformity, wavelet grey level nonuniformity HLH | Shape, 1sr and 2nd order, wavelet | (7) |
Stage = I–IIIb (edition NOS) | ||||||||||
Age =67 years | ||||||||||
Males = NOS | ||||||||||
8 | N=368 surgical cohort; AC =62% (Tr), 49% (Tu), 100% (Te) | M: 7 independent datasets from 5 institutions including 3 surgical data sets from Moffitt, MUMC, M-Spore | OS | CECT | Manual tumor and peritumoral | PyRadiomics | 3D convolutional CNN derived from radiation subjects but then applied to surgical patients | deep learning networks in predicting 2-year overall survival of NSCLC patients from CT data; specific radiomic features not stated. Importance of peritumoral region noted. | Specific classes of radiomic features not stated | (8) |
Stage = I–III (edition NOS) | ||||||||||
Age =69 years | ||||||||||
Males = not specified | ||||||||||
9 | N=460 of which 350 from CCF and UPenn, 106 from TCGA-LUAD and TCGA LUSC, 211 from TCIA; AC =79% for CCF and UPenn cohort | M: CCF, UPenn, TCGA (LUAD and LUSC) and TCIA | TTP, RFS | Non-CECT | Manual: tumor and automated for peritumoral region | Not stated | RRS developed from 12 Machine Learning twelve machine-learning classifiers from nine classifier families; PI-AUC scores for feature stability; LDA, LASSO univariant/multivariant Cox proportionality for feature reduction and K-M survival analysis for outcomes | Multiple features (46) including Laws and Laws-Laplacian, Gabor features of both intratumoral and peritumoral regions | Shape, 1st and 2nd order | (9) |
Stage I–IIA (8th) | ||||||||||
Age =67 years for CCF and UPenn Cohort | ||||||||||
Males =49% for CCF and U PENN cohort | ||||||||||
10 | N=93; AC=62% | S:I | RFS, OS | 18F-FDG-PET | Automated: tumor | Chang-Gung Image Texture Analysis toolbox | Feature reduction performed using Information gain ratio, Gini index, and chi-square; Radiomic model building testing for best performance with RF, neural network, naive Bayes, LR, and SVM | Contrast and busyness texture features from neighborhood grey-level difference matrix are best predictors of RFS; with RF modeling providing the best performance | 2nd order | (10) |
Stage I–IIIa (edition NOS) | ||||||||||
Age =63 years | ||||||||||
Males =59% | ||||||||||
11 | N=291 (Tr 145, V 146); AC =78% (Tr), 71% (V) | S: I (dataset available on TCIA) | DFS for Stage I–IIIA and PFS for Stage IIIB and IV | 18F-FDG-PET | Semiautomated: tumor; automated penumbra | Matlab-Quantitative Image Feature Engine | LASSO l tuning for risk of recurrence; univariant/multivariant Cox proportionality with; K-M survival analysis | Stage and MTV-Penumbra (Gray-level Cooccurrence Matrix Maximum Probability most associated with DFS) | 2nd order | (11) |
Stage I–IIIA (7th; 97%) | ||||||||||
Age =69 years (Tr), 71 years (V) | ||||||||||
Males =75% (Tr), 60% (V) | ||||||||||
12 | N=227; AC =79% (Tr); 58% (V) | M: TCIA, I | DFS | 18F-FDG | Manual: (I) MTV, (II) penumbra (1 cm), (III) MTV-penumbra combined | Matlab-Quantitative Image Feature Engine | LASSO; univariant/multivariant Cox proportionality; K-M survival analysis | Several features including, clinical stage, BM-Penumbra GLCM sum mean skewness energy features, BM-GLCM cluster tendency skewness | 1st and 2nd order | (12) |
Stage I–III (7th; 97%) | ||||||||||
Age =69 years (Tr); 72 (V) | ||||||||||
Males =74% (Tr); 59 (V) | ||||||||||
13 | N=211; AC =82% | S:I (dataset from Stanford and Palo Alto VA available from TCIA) | OS | 18F-FDG PET/CT | Semiautomated with application of 3 different image fusion techniques: tumor | Standardized Environment for Radiomics Analysis Package | 7 ML feature selection techniques including Cox Proportional Hazard, CoxBoost, GLMNET, RF, GLMBoost, GBM, Survival Tree 4-index and 4 Radiomic feature selection including C-Index and three wrapper feature selection methods using random forest variable selection, with tree minimum depth methodology steps. In addition, Combat for feature harmonization to remove batch effects from multiple types of PET/CT acquisitions | Multiple features selected for PET and CT with those selected by GLMNET having the highest average results | 2nd order | (13) |
Stage I–IV (pathological stage with <1% Stage IV) | ||||||||||
Age =68 years | ||||||||||
Males =63% |
AC, adenocarcinoma; Age, mean age; S, single institution; I, internal healthcare center and/or regional centers; RFS, recurrence-free survival; CECT, contrast enhanced CT; SVM, support vector model; RF, Random Forest; 7th, AJCC Cancer Staging Manual 7th edition; 8th, AJCC Cancer Staging Manual 8th edit; OS, overall survival; GLM, General Linear Model; MP, micropapillary; P, primary; V, validation; GLRLM, gray level run length matrix; GLCM, gray-level co-occurrence matrix; GLSZM, gray-level size zone matrix; LDA, Linear discriminant analysis; TCGA-LUAD, The Cancer Genome Atlas-lung cancer adenocarcinoma; TCGA-LUSC, The Cancer Genome Atlas-lung cancer squamous cell carcinoma; RRS, Radiomic Risk Score; TTP, time to progression; PI-AUC, preparation induced instability score-area under the curve; LASSO, least absolute shrinkage and selection operator; ROI, region of interest; IDM, inverse difference moment; LLL, low-pass filter setting-LowLowLow; LLH, low-pass filter setting-LowLowHigh; HLH, high-pass filter setting-HighLowHigh; MUMC, Maastricht University Medical Center; FDG, fluorodeoxyglucose; PFS, progression-free survival; MTV, metabolic tumor volume; So, solid; O, overall; DCA, decision curve analysis; DFS, disease-free survival; NOS, not otherwise specified; Tu, tuning; Te, testing; Tr, training; CNN, convolutional neural networks; CCF, Cleveland Clinic Foundation; M, multi-institutional/multiple; LR, linear regression; BM, bone marrow; TCIA, the cancer imaging archive; CoxBoost, Cox model fitted by likelihood-based boosting; GLMNET, LASSO and Elastic-Net regularized generalized linear model; GLMBoost, gradient boosting with component-wise linear model; GBM, generalized boosted regression model; PET/CT, positron emission tomography/computed tomography.
Background
In the United States, the 5-year relative survival rate for people diagnosed with NSCLC between 2011 and 2017 was approximately 26% (ranging from 64% in early-stage disease to just 8% for patients with distant metastatic tumors [see SEER (Cancer.org)]. Despite recent advances in the treatment of NSCLC with targeted therapies, radiotherapy and immunotherapies, NSCLC remains one of the most challenging cancers to treat. At present, the TNM staging of NSCLC, based in part upon clinical imaging, has been widely used to predict prognosis and guide therapy. The high incidence and mortality rates of NSCLC demonstrates the need for better diagnostic and treatment strategies, as well as ways to monitor response to therapy. However, even patients with the same TNM stage may have different prognoses due to tumor heterogeneity. Molecular characterization of NSCLC may also factor into determinations of patient prognosis and outcomes in the near future, though the majority of focus is currently centered on advanced stage disease (14). In early-stage NSCLC, a major concern is that the risk of recurrence remains high (approximately 18–20% within 2–3 years from start of initial therapy) (15). Identification of these patients with increased risk of recurrence following definitive surgical resection is therefore an unmet need. Radiomics may be uniquely equipped to handle this problem. The section below provides a summary of radiomic methodology and analysis. This is followed by a review of the current radiomic signatures that may define recurrence in early-stage NSCLC disease.
Review of radiomic methodology and analysis
There are 5 essential steps when providing a radiomic analysis of early-stage NSCLC disease for determining outcomes as outlined in Figure 1 and described below.
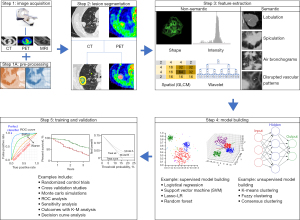
Step 1: image acquisition and calibration
Radiomics is dependent upon acceptable diagnostic quality scans which can be defined as those that maintain image consistency while minimizing the impact of scanner performance differences, slice thickness variability, reconstruction algorithms, and other related issues that lead to “image noise”. The use of IV contrast has a critical impact on a radiomic signature but such signatures can also be obtained from high-quality non-contrast studies. Several studies have shown that acquisition parameters are a major contributor to the stability and reproducibility of a radiomic signature. The impact of these parameters has been tested using both phantom and clinically acquired scans. For example, using a PET phantom, Galavis et al. has demonstrated that up to three-quarters of the radiomic signal in PET radiomics are very unstable (16). Similarly, using a public database, Zhao et al. looked at the impact of slice thickness and reconstruction algorithms in 31 patients with NSCLC who had same-day repeat CT scans. Their results showed that only 19% of 81 radiomic features were repeatable using different acquisition settings and the majority of the radiomic features (up to 75%) were influenced by respiratory artifacts alone (17). Therefore, respiratory gating in lung lesions is critical for obtaining a reliable radiomic signature. In another example, using CT scans to distinguish malignant from benign pulmonary nodules, He et al. retrospectively evaluated solitary pulmonary nodules (SPN) from 240 subjects and found that differences in three acquisition factors (IV contrast, slice thickness, and reconstruction kernels) alone greatly influenced the radiomics signature in SPN. Interestingly, non-contrast, thin-slice CT reconstructed with a standard (soft tissue) convolution kernel-based CT was the most predictive of malignancy (2).
Since it is impractical to control for all of the technical acquisition factors, especially when using real-world data, there is a growing need to apply calibration and correction factors that can correct for acquisition differences between patients. This step of “homogenization of the images” requires the use of computer algorithms to calibrate and normalize the imaging signal within each scan so that patients from different institutions can be pooled together. Such calibration of scans requires a pre-processing step that occurs after image acquisition. Some examples of scan calibration include pixel re-sizing, grey-level normalization, signal re-sampling processes, filtering to provide histogram equalization, de-blurring, and re-sampling. Thus, the pre-processing of images following acquisition and reconstruction can be one of the most important steps to apply to all scans (whether CT, MRI and/or PET) to control for inherent inhomogeneities across multi-institutional or inter-departmental differences in scanners and acquisition protocol, especially when using real-world data to derive stable radiomic imaging signatures. Although a consensus on a universal acquisition and calibration standard across institutions has yet to be reached, various organizations have proposed such using a set standard (18-20).
Step 2: segmentation
The identification and isolation of the tissue/tumor of interest for radiomic analysis requires lesion segmentation. Segmentation of lesions and/or diseased tissue can be obtained by one of three approaches: manual segmentation, semi-automated segmentation, and fully-automated segmentation.
Manually segmentation is a time-consuming process that requires expert reader placement of a 2D region of interest (ROI) or 3D volume of interest (VOI) around the tissues or tumors of interest. These ROIs/VOIs serve to define the Cartesian coordinates (in the x, y, z planes) of tissue/tumor located in anatomic space. The resulting ROIs/VOIs are then used as a template or “mask” for extracting signal within those defined regions to derive a radiomic signature. The use of several general purpose open source tools (e.g., ITK-SNAP: http://itksnap.org) to provide easy-to-use lesion segmentation are readily available for deriving accurate ROIs/VOIs from different data sets and image modalities (21). In addition to providing manual ROI/VOIs for determining derived mathematical values (i.e., non-semantic features), the reader may also provide qualitative (visual) descriptions of the tumor and surrounding tissues (i.e., sematic features). For example, when determining whether a lesion is malignant or benign, the mean and range of pixel density measurement of a tumor on CT (i.e., the non-semantic features) along with a semantic description of the tumor margins by the reader (e.g., either the presence of spiculation or smooth margin) may be very important. In addition to whole tumor analysis, there are circumstances whereby understanding regional radiomic differences within and/or surrounding a lesion may be important (e.g., peritumoral vs. intratumoral vs. distant structures); manual segmentation can be quite flexible in these circumstances. A common drawback for using manually drawn ROIs/VOIs is the imprecision of reader placement of such boundaries around the lesion(s)/tissue(s). Manual segmentation can be quite variable and dependent upon the expertise of the reader. Therefore, when manual segmentation processes are utilized in a radiomic study, a measure of inter- and intra-reader variability of the specific radiomic features may be necessary to determine which particular feature(s) show the greatest degree of variability due to human decision making. Nevertheless, manual segmentation is the most commonly performed process for lesion segmentation and is a necessary method used for studies in which automated methods of lesion segmentation are being developed and tested against the truth-standards of expert readers.
Semi-automated methods provide the placement of a ROI/VOI around lesions/tissues using computer algorithms that have predefined rules for determining the boundaries of a lesion. Semi-automated methods often require manual adjustments to reduce false edges and over segmentation once an ROI/VOI is defined. Boundary detection algorithms have been developed based upon thresholding techniques that define a range of pixel that should be included (or excluded) in the ROI/VOI along with edge detection rules for selecting pixels that should belong to a lesion vs. adjacent tissue signals. Semi-automated methods can also use a regional seed growing processes that starts with a reader defined pixel value on single slice which then grows until all user-defined ranges of pixel values are selected. The use of advanced methods such as fuzzy c-means, fuzzy hidden Markov chains, fuzzy locally adaptive Bayesian (FLAB) segmentation algorithms and watershed segmentation programs are becoming more popular because they can better define the edges along the interface between normal tissue and diseased sites. Semi-automated methods have the advantage of speed and repeatability compared to manual segmentation. For example, Balagurunathan et. al. demonstrated high reproducibility using seed-based image analysis program with segmentation performed by a single reader (22). Others have shown better reproducibility with semi-automated approaches compared to manual segmentation when multiple readers are involved (23,24).
Fully automated methods using either convolutional neural networks (CNN) or deep neural networks (DNN) have been receiving great interest in lesion segmentation and other outcome predictions. In this method, the scans are “fed” into a CNN or DNN algorithm (either supervised or unsupervised) in order train the system to recognize the lesion(s) boundaries. This machine learning approach relies on a stacked neural network structure that undergo image transformation, pooling, activation, full connection, and batch normalization to derive a best-fit solution. The advantages of automated methods include speed reliability, reproducibility, and the capability to learn non-linear complex relationships beyond what is achievable by an expert reader. One of the main drawbacks of CNN and DNN approaches however, is the large number of examples required to train the data. As a proof of using automated segmentation, Lustberg et al. developed a CNN approach to automatically segment organs at risk in lung cancer radiotherapy which outperformed manual segmentation (25).
Step 3: feature extraction
Radiomic features can be described as either qualitative (semantic) or quantitative (non-semantic). Most radiomic studies focus upon the later since it can be standardized. Although semantic features are difficult to convert into mathematical equations, nonetheless, they can provide valuable information. For example, Liu et al. defined a number of semantic features that predicted epidermal growth factor receptor (EGFR) status in lung cancer patients (26).
In general, radiomic features can be grouped into four main categories: (I) lesion shape, (II) first order features, (III) second order features, and (IV) higher order features (e.g., wavelet). The shape of a lesion can provide insight into the nature of the tumor, its outcome and predictable behavior when subjected to treatment. Shape can be defined according to how similar a lesion is to a sphere (e.g., sphericity). Other properties of lesion shape (e.g., spiculation, lobulation, smoothness) can be very important for determining malignancy.
First order features refer to measures of signal intensity. First order features are usually represented by a histogram frequency curve (HFC) in which the signal intensity of a lesion (x-axis) is plotted against the frequency of its occurrence (y-axis). Several features contained within the HFC can be derived, including mean pixel signal (density, signal intensity, or radioactivity for CT, MRI, and PET, respectively), standard deviation, skewness, kurtosis, and entropy or uniformity (measure of heterogeneity). First order features are very sensitive to contrast enhancement effects and slice thickness. The generation of these parameters usually requires some type of voxel re-spacing into an isotropic space (pixel dimension is identical in the x, y, z direction) to make each pixel invariant to rotational effects. Additional adjustments may require the removal of unwanted pixel values (e.g., from air), filtering (e.g., gaussian, Laplacian filters) and discretization of the pixel values (i.e., re-binning the data from a wide range of pixel values into discrete values) to generate a meaningful HFC. First order features generally do not contain information regarding the spatial distribution of the pixel intensities. Using first-order features from CT scans, we previously showed that lower kurtosis and positive skewness were significantly associated with K-ras mutations in NSCLC and prognostic for overall survival (OS) and disease-free survival (DFS) (27).
Second Order features highlight the spatial distribution of pixel signals. These features describe the texture of a lesion (or tissue) and try to capture the complexity of the local signal arrangements. The second order features include grey-level co-occurrence matrices (GLCM), neighborhood gray-tone difference and neighboring grey-level dependence matrices, grey-level run lengths and grey-level size zone-based matrices, just to name a few. In essence, second order features describe the non-semantic arrangement or organization of pixels of similar (or dissimilar) values at pre-defined distances and direction from one-another to depict such textural patterns in terms of their homogeneity (or heterogeneity), contrast, variance, coarseness, complexity, sameness, run-lengths, connectedness, etc.
Higher order features include radiomic properties using wavelet band pass filtering and deep learning methods. Wavelet based methods work by deconvoluting an image to extract first and second order features at different frequency bands of different weights. Such methods have been used successfully in determining the risk for developing lung metastasis in patients with soft tissue sarcomas (28). Deep learning methods, on the other hand, use multi-layer feed-forward neural networks. Various feature variables are used to learn (train) highly discriminative images features for a particular desired outcome. This approach has the advantage of handling large data sets in an efficient manner for machine learning processes to produce stable and sustainable radiomic signatures.
Step 4: model building
Modeling building tasks may apply statistical and/or ML approaches to extracted features using supervised, semi-supervised or unsupervised learning strategies. In supervised learning, the inputs are well labeled. This allows classification of data from previous experience. Supervised learning classifiers include generalized linear models, random forests, support vector machines, least absolute shrinkage and selection operator (LASSO)-logistical regression and neural networks that can parse the information efficiently into designated outcome variables. As an example, Parmar et al. applied 14 different feature selections and 12 different classification methods on retrospective CT images from 484 NSCLC subjects to determine the variability and stability of both classifiers and feature selections on radiomic signatures. Amongst the different combination tested, they found that the dominate source of variation was dependent upon the applied classification models while feature selection had less impact. In their study random forests methods resulted in low total variance of a signature (29). Thus, the selection of the optimal model is critical for generating stable clinically relevant radiomic signatures.
Unsupervised learning allows for more complex processing but can be more unpredictable. In unsupervised learning, the classifier(s) work on its own to discover unknown patterns and provide features in real time with much less manual intervention. Unsupervised learning works by grouping data into clusters-distant measurements between samples. Clustering algorithms, such as k-means clustering, fuzzy clustering, and consensus clustering are used to arrange the extracted features into clusters of sameness or dissimilarity. This approach was more effectively than clinical-based metrics in determining the treatment response to radiation therapy (30).
One of the major limitations in utilizing each of these approaches includes a high degree of dimensionality generated by radiomics since the extracted features can number into the hundreds and even thousands. As a result, many of these features can be interrelated, measuring the same information. Therefore, a crucial component of model building involves a feature reduction step to avoid overfitting. The type of feature reduction techniques includes univariate filter-based (e.g., Fisher score and Wilcoxon tests), multivariate (e.g., minimum redundancy) and principle component analysis. Thus, a multitude of features can be reduced to a handful of quintessential elements. In early work, Segal et al. demonstrated that 28 traits can describe up to 80% of the gene expression patterns in hepatocellular carcinoma (31).
Finally, semi-supervised learning combines both supervised and unsupervised methods. In this approach, large data sets can be trained using an unsupervised learning to minimize the number of features needed to express an outcome. This is followed by a testing a supervised model to determine the association of features to clinical labels. In this way, high throughput, high dimensionality data can be reduced to only a few features for training, testing, and validation of viable radiomic signatures.
Step 5: application and validation
Any application of a radiomic signature(s) for clinical care purposes must be validated. Not only are there several radiomic software programs in use, but it is also difficult to compare radiomic outputs from each of these systems against one another since the mathematical algorithms and outputs behind each system may be quite different. A key component of usefulness is scientific validation. Almost all radiomic studies must begin with a training step that usually consists of a deriving an imaging signature(s) from retrospect collections of images using either a single institution, multicenter study, or public data bases (e.g., The Cancer Imaging Atlas). During training, not all available images should be used since a portion of the available scans will need to be set aside for validation. A validation step is required to understand a signature’s diagnostic performance. In most instances, about two-thirds of the available data sets are randomly selected to undergo training and testing, while one-third are held out for validation when an external data set is not available. The highest level of validation traditionally employs a prospective, randomized control trial design with predefined endpoints. To our knowledge, no such trial has been performed for radiomic signature validation.
One of the key consideration to incorporate a radiomic signature in a clinical trial or for clinical decision-making purposes is to understand how the different aspects of features selection methods, classification models, and the number of top-rated features will impact the results. One critical component for developing a radiomic signature is to determine which radiomic feature(s) are reflecting measurements related to technical factors (e.g., patient preparation and image acquisition/reconstruction) rather than physiology, biology, and pathology. Recently, Singh et al. (32) applied techniques typically used to remove effects of machinery and protocol on gene expression date, so-called ComBat (Combining Batches) harmonization methods to address this issue. Using two publically available image data sets from The Cancer Imaging Archive (Breast I-SPY1 and NSCLC IO), they found that heterogeneity-mitigation using ComBat techniques that addressed voxel resampling, voxel spacing, high kernel resolution, pixel spacing, and slice thickness provided more stable radiomic features compared to raw data without harmonization-mitigation.
Furthermore, Parmar et al. (29) examined the performance and stability of 14 radiomic feature selection methods and 12 classification schemes and the number of features (ranging between 5–50) in predicting the two-year survival of NSCLC in subjects undergoing radiation therapy from two independent institutional cohorts. Not surprisingly, there was wide variability in the predictive performance based upon the selection of different methods used (up to 34% variability). The implication of their conclusions was that the selection of features and classifiers will have a relatively large impact on predicting clinical outcomes and should be carefully determined beforehand if used in a clinical trial and/or clinical setting.
Use of radiomics analysis to define disease recurrence in early-stage NSCLC undergoing surgery
CT based radiomic studies
Table 2 provides a summary of relevant published retrospective studies reporting radiomic signatures using survival outcomes in NSCLC patients undergoing surgical resection. Although most of the studies listed have shown encouraging results, any comparison and aggregation of the studies together can be complicated by variabilities in radiomic signature stability and reproducibility due to inconsistences in patient population (e.g., admixture of early and advanced stage disease and lung cancer histologies, differences in gender, age, smoking history, country of origin, study period, technology advances, etc.), definition and timescales to define disease recurrence, presence or absence of additional radiologic and/or pathologic features known to lead to poor outcomes (e.g., vascular and/or pleural invasion), and tumor mutations.
As expected, radiomics of the intratumoral region itself in early-stage disease (Stage I−IIIA) is very important for outcomes. For example, malignant lesions can be described as being either solid or sub-solid (i.e., semi-solid and/or ground glass opacities) on CT. The latter has been associated with better outcomes. Importantly, the solid vs. subsolid nature of the tumor may be related to tumor histology, which can play an important role in recurrence, and thus drive a radiomic signature. For example, the presence of micropapillary/solid (MP/S) growth patterns by histology (33) may be a poor prognostic indicator. Using radiomics, He et al. evaluated 268 patients for a radiomic signature that would distinguish MP/S histology from other types. Several radiomic features (Table 2) were noted to be able to discriminate MP/S histology vs. other subtypes {area under the curve (AUC) 0.73 [95% confidence interval (CI): 0.62–0.84]} (2). Therefore, ensuring that the different histologic subtypes in a patient population have been accounted for may be an important factor when considering the strengths and limitations of a radiomic signature.
Although most authors have identified tumor shape, and 1st and 2nd order elements as being important determinants of outcomes (Table 2), one surprising finding in recent studies, which was ignored in earlier reports, demonstrates the importance of including the peritumoral space (i.e., tissue surrounding the primary tumor) in radiomic analysis (34). Yet, given the potential importance of this region on tumor spread and recurrence, a precise definition of the peritumoral space has not been optimized, though interrogation of the peritumoral space beyond 1.5 cm may be of limited value (35,36). Furthermore, the dependency of the radiomic signature on the peritumoral region(s) may be related to tumor size. For example, Lee et al. (1) showed that the intra/peritumoral regions of lesions <5 cm are the lesions are needed for a robust radiomic signature while tumors >5 cm only needed the intratumoral components.
In order to risk-stratify patients with early-stage disease, Wang et al. was able to successfully assign stage IA patients into low-risk (LR) and high-risk (HR) groups (mean follow up for recurrence was approximately 69 months). In this study of 378 patients who underwent definitive surgical resection, 8 top performing radiomic signatures (shape, intensity, and texture) were identified on contrast-enhanced CT and used to create a rad-score (r-score) (3). When applied to solid tumors, Kaplan-Meyer (K-M) analysis demonstrated excellent separation in survival between HR vs. LR patients (r-score cutoff of 0.579 for HR vs. LR; P<0.001), while patients with sub-solid tumor did not show as strong a separation using the r-score (P=0.083). This lower r-score performance for sub-solid lesions may have been related to the already excellent prognosis in this patient group. In addition, the radiomic signature for patients with sub-solid lesions was an independent predictor of survival and performed better than clinical-pathological information [Concordance (C)-index: 0.829, 95% CI: 0.787–0.870 vs. 0.706, 95% CI: 0.648–0.764, P<0.001]. Recently, these authors further expanded on their analysis to include both intra-tumoral and peri-tumoral radiomic analysis in stage IA patients with solid lesions only (n=592) (4). Using both internal and external (public) validation sets, a total of 18 (13 intra-tumoral and 5 peri-tumoral) radiomic features were able to distinguish HR from LR patients with similar accuracy as previously reported, though the top performing features were not exactly the same as in their prior study.
Liu et al. developed a ML-based nomogram to predict progression-free survival (PFS) in NSCLC by combining both radiomic and clinical pathologic data from 200 subjects with resectable lung cancer. The lesions were further stratified as solid or sub-solid. An r-score was created using the top 20 performing radiomic features showing the greatest importance (1 wavelet, 2 texture, and 1 intensity features). The ML-based nomogram showed best performance when combined with clinical-pathologic data (C-Index: 0.845 combined, 0.781 radiomic, 0.755 clinical). Included in their clinical-pathologic data was the incorporation of EGFR mutation status which had less impact than expected (5). Unlike Wang et al., Liu et al. did show that the r-score also had good performance for sub-solid lesions as well. These opposing conclusions may be related to more latter stage disease patients in Liu et al.’s study.
Choe et al. performed a retrospective analysis of preoperative CTs in 1,058 patients who underwent definitive surgical resection and demonstrated that radiomics was able to independently predict DFS [hazard ratio (HR), 1.920; P<0.001] (6). They did not address peri-tumoral analysis or sub-solid lesions in their analysis. Two radiomic features of tumor heterogeneity were found to be top-ranking features.
In a separate analysis, Aerts et al. (7), evaluated 5 independent datasets comprised of NSCLC and primary head and neck malignancies. The analysis of one of these NSCLC datasets (i.e., MAASTRO clinic, The Netherlands; n=422 subjects) was used to determine prognosis. Based upon feature stability rank and performance, the top four radiomic features were most associated with outcomes.
In one of the largest radiomic studies to date, Hosny et al. (8) used a deep learning (CNN) approach on 7 independent contrast-enhanced CT data sets of 1,194 patients from 5 institutions to evaluate the 2-year OS rate in patients with NSCLC. Using an external validation set, these authors identified prognostic signatures for patients treated with radiotherapy. Then, in a unique manner, they used a transfer learning approach to apply the radiomic signature to 391 patients treated by surgical resection alone and reported good performance for risk stratification (AUC =0.71; 95% CI: 0.60–0.82; P<0.001). Furthermore, they reported that the peritumoral region, in particular high-density signal on CT from the parenchyma and pleura, was important in predicting outcomes using their CNN method. Although the authors did not report on lesions based upon solid vs. sub-solid features, this study provides promise in finding common radiomic signatures that may be generalizable across multiple populations and clinical scenarios.
Recently, Khorrami et al. (9) sought to determine the feasibility of obtaining a generalizable radiomic classifiers for disease recurrence in early-stage disease (Stage I−IIA, R0 resection) independent on many of the technical factors that can give rise to instability and variability in radiomic signatures (i.e., batch effect due to differences in scanner model, acquisition protocols, and reconstruction settings). Selecting radiomic features that are both stable and discriminatory, they applied a preparation-induced instability score (PI) to eliminate those features that might be due to technical differences between scanners and imaging acquisition differences. By evaluating 650 patients across 4 different settings (2 from large academic centers and 2 from public databases) they developed a radiomic risk score of the most stable-discriminating features that were least affected by technical differences between patients. They showed very good performance in predicting outcomes compared to discriminatory features alone (HR =1.66, C-index of 0.72 vs. HR =1.04, C-index of 0.62). Similar to other publications, they noted that the peritumoral region was an important region for establishing a radiomic signature for recurrence. Interestingly, they observed that peritumoral features were also relatively more stable than intra-tumoral features, possible due to the fact that the peritumoral areas demonstrate less variation in CT signal from different acquisition settings than the intra-tumoral regions themselves.
18F-flurodeoxyglucose (FDG) PET based radiomic studies
18F-FDG PET is considered part of the conventional staging work up of patients with NSCLC due to the high sensitivity of metabolic imaging to detect occult tumors. 18F-FDG PET can also be useful in NSCLC to detect early recurrence and distinguish benign from malignant disease in equivocal cases on CT. As expected, most radiomic studies focus imaging signatures identified on CT rather than 18F-FDG PET. This is due to the less frequent global availability of PET and the inherent difference in poorer spatial resolution on 18F-FDG PET. However, one advantage of PET radiomics over CT is that there more consistency in the PET signal produced from different scanners and from different reconstruction algorithms (37,38). A meta-analysis of several 18F-FDG PET studies have shown that the metabolic activity of the primary tumor [either through standardized uptake value (SUV) or metabolic tumor volume (MTV) measurements] can be prognostic of outcomes (39).
Naturally, there is interest in using PET radiomics to predict outcomes in NSCLC patients. However, few studies have focused on 18F-FDG PET radiomics in patients undergoing definitive surgical resection. As an example, Ahn et al. evaluated 18F-FDG PETs in 93 patients with early-stage disease (Stage I–III) and found that the radiomic features of contrast and busyness texture features from neighborhood grey-level matrix differences were the matrix the best predictors of disease recurrence (10). However, the usefulness of their radiomic signature was diminished because it was not a homogenous population as over 50% of their patients received adjuvant chemotherapy and an external validation dataset was not available.
In a more comprehensive study, Mattonen et al. (11) investigated the potential of 18F-FDG PET radiomics to predict recurrence. They assessed radiomic feature of the tumor and penumbra (peritumoral region) of the 18F-FDG PET scans from 291 patients obtained at multiple institutions (both public and local imaging sites) for training and validation. They found that their top performing features for predicting recurrence was tumor stage and combined metabolic tumor volume-radiomics of the penumbra (2nd order features). Interestingly, size, shape, and SUV measurements were not top performing features. This suggested that the texture-based PET radiomics may play an important role for prognosis beyond MTV tumor uptake. In a separate evaluation of 227 patients (12) the authors included radiomics of the bone marrow signal on 18F-FDG PET in addition to the tumor and penumbra. The consideration of including radiomics of the bone marrow on 18F-FDG PET was based upon the hypothesis that the bone marrow may serve as a marker of altered serum cytokine, C-reactive protein, and hematologic parameters that could affect outcomes. The addition of bone marrow radiomics was noted to be stable and improved the ability to stratify patients into HR vs. LR recurrence groups. A top radiomic model containing tumor stage, bone marrow radiomic features and tumor with penumbra showed good concordance (0.72: 95% CI: 0.64–0.80; P<0.001) and good discrimination of HR vs. LR patients (P<0.001) in an internal (temporal) validation set.
Finally, an across-the-board comparison of the performance of various algorithms applied to multimodality radiomics models (e.g., PET-CT fused information) are still lacking. Amini et al. (13) addressed this shortcoming by performed a comprehensive evaluation of combining both the anatomic signal from CT and with metabolic signals from 18F-FDG PET. They demonstrated which method(s) would is most suitable for image fusion, feature selection and ML algorithms in a multi-modality approach based upon a time to event prediction model. Applying applied different multi-level image fusion methods along with evaluating 7 ML and 4 feature selection techniques for each fusion technique, they found considerable variability based upon the techniques used but concluded that were some combinations that work best (Table 2). Certainly, as PET/CT technology evolves and as more sophisticated ML algorithms become available, the optimal combinations may change. More importantly, however, this study provided an excellent roadmap on how to tackle the challenges of multi-modality imaging in the future.
Conclusions
Future of radiomics in early-stage NSCLC and conclusions
Here we have highlighted some of the methodologies and promise for using radiomic signatures to determine a patient’s risk for recurrence following definitive surgical resection. However, several substantial challenges remain before radiomics can be clinically useful. Indeed, looking over the entire publication history of radiomics, Park et al. (40) recently reported on the overall scientific quality of the published studies and found them wanting. Their conclusions were based on low radiology quality scores and TRIPOD (Transparent Reporting of a multivariable prediction model for Individual Prognosis or Diagnosis) reporting due to lack of feature reproducibility and clinical utility amongst other factors.
Despite these drawbacks, several noteworthy observations stand out. Since the inception of radiomics, the number of accessible publicly-available databases are growing. The democratization and public access to both a growing number of databases and open-source software platforms for radiomics analysis (e.g., PyRadiomcs: www.python.org and brain-CaPTk: www.med.upenn.edu/sbia/captk.html) is advancing this field. More efforts will need to be placed on making additional database available for discovery, testing, training, and validation, particularly independent or external validation.
Next, radiomics has shown the feasibility in extracting high-content information and correlating it with clinical, pathologic, biologic, prognostic, and predictive information and has helped to advance both imaging sciences and AI. The next generation of radiomics will need to focus its efforts on standardization, stability, and generalizability of radiomic features for it to be useful for clinical decision making. Although the exact biological drivers behind the creation of a radiomic signature is largely unknown, it seems that most radiomic signatures provide a measure of tumor heterogeneity and the importance of the peritumoral region cannot be understated (41). With further efforts, radiomics may provide clinically meaningful information, leading to new areas of discovery and potential curative approaches.
Acknowledgments
Funding: None.
Footnote
Reporting Checklist: The authors have completed the Narrative Review reporting checklist. Available at https://tlcr.amegroups.com/article/view/10.21037/tlcr-23-5/rc
Peer Review File: Available at https://tlcr.amegroups.com/article/view/10.21037/tlcr-23-5/prf
Conflicts of Interest: All authors have completed the ICMJE uniform disclosure form (available at https://tlcr.amegroups.com/article/view/10.21037/tlcr-23-5/coif). GJW serves as an unpaid editorial board member of Translational Lung Cancer Research from November 2022 to October 2024. RK is Adjunct Faculty at Translational Genomics. He is the founder of Imaging Endpoints Core Lab. He is a shareholder in Globavir, Renibus, Verve and Telehealth. Issued patents include PCT/US2017/047026, US10854338B2, US10332634B2. GJW is a former employee of SOTIO Biotech Inc., a former employee of Unum Therapeutics; reports personal fees from Imaging Endpoints II, MiRanostics Consulting, Gossamer Bio, Paradigm, International Genomics Consortium, Angiex, IBEX Medical Analytics, GLG Council, Guidepoint Global, Genomic Health, Oncacare, Rafael Pharmaceuticals, Roche, and SPARC-all outside this submitted work; has ownership interest in Unum Therapeutics (now Cogent Biosciences), MiRanostics Consulting, Exact Sciences, Moderna, Agenus, Aurinia Pharmaceuticals, and Circulogene-outside the submitted work; and has issued patents: PCT/US2008/072787, PCT/US2010/043777, PCT/US2011/020612, and PCT/US2011/037616-all outside the submitted work. The other authors have no conflicts of interest to declare.
Ethical Statement: The authors are accountable for all aspects of the work (including full data access, integrity of the data and the accuracy of the data analysis) in ensuring that questions related to the accuracy or integrity of any part of the work are appropriately investigated and resolved.
Open Access Statement: This is an Open Access article distributed in accordance with the Creative Commons Attribution-NonCommercial-NoDerivs 4.0 International License (CC BY-NC-ND 4.0), which permits the non-commercial replication and distribution of the article with the strict proviso that no changes or edits are made and the original work is properly cited (including links to both the formal publication through the relevant DOI and the license). See: https://creativecommons.org/licenses/by-nc-nd/4.0/.
References
- Lee S, Jung J, Hong H, et al. Prediction of Two-Year Recurrence-Free Survival in Operable NSCLC Patients Using Radiomic Features from Intra- and Size-Variant Peri-Tumoral Regions on Chest CT Images. Diagnostics (Basel) 2022;12:1313. [Crossref] [PubMed]
- He B, Song Y, Wang L, et al. A machine learning-based prediction of the micropapillary/solid growth pattern in invasive lung adenocarcinoma with radiomics. Transl Lung Cancer Res 2021;10:955-64. [Crossref] [PubMed]
- Wang T, Deng J, She Y, et al. Radiomics Signature Predicts the Recurrence-Free Survival in Stage I Non-Small Cell Lung Cancer. Ann Thorac Surg 2020;109:1741-9. [Crossref] [PubMed]
- Wang T, She Y, Yang Y, et al. Radiomics for Survival Risk Stratification of Clinical and Pathologic Stage IA Pure-Solid Non-Small Cell Lung Cancer. Radiology 2022;302:425-34. [Crossref] [PubMed]
- Liu G, Xu Z, Zhang Y, et al. Machine-Learning-Derived Nomogram Based on 3D Radiomic Features and Clinical Factors Predicts Progression-Free Survival in Lung Adenocarcinoma. Front Oncol 2021;11:692329. [Crossref] [PubMed]
- Choe J, Lee SM, Do KH, et al. Outcome prediction in resectable lung adenocarcinoma patients: value of CT radiomics. Eur Radiol 2020;30:4952-63. [Crossref] [PubMed]
- Aerts HJ, Velazquez ER, Leijenaar RT, et al. Decoding tumour phenotype by noninvasive imaging using a quantitative radiomics approach. Nat Commun 2014;5:4006. [Crossref] [PubMed]
- Hosny A, Parmar C, Coroller TP, et al. Deep learning for lung cancer prognostication: A retrospective multi-cohort radiomics study. PLoS Med 2018;15:e1002711. [Crossref] [PubMed]
- Khorrami M, Bera K, Leo P, et al. Stable and discriminating radiomic predictor of recurrence in early stage non-small cell lung cancer: Multi-site study. Lung Cancer 2020;142:90-7. [Crossref] [PubMed]
- Ahn HK, Lee H, Kim SG, et al. Pre-treatment (18)F-FDG PET-based radiomics predict survival in resected non-small cell lung cancer. Clin Radiol 2019;74:467-73. [Crossref] [PubMed]
- Mattonen SA, Davidzon GA, Bakr S, et al. [18F] FDG Positron Emission Tomography (PET) Tumor and Penumbra Imaging Features Predict Recurrence in Non-Small Cell Lung Cancer. Tomography 2019;5:145-53. [Crossref] [PubMed]
- Mattonen SA, Davidzon GA, Benson J, et al. Bone Marrow and Tumor Radiomics at (18)F-FDG PET/CT: Impact on Outcome Prediction in Non-Small Cell Lung Cancer. Radiology 2019;293:451-9. [Crossref] [PubMed]
- Amini M, Hajianfar G, Hadadi Avval A, et al. Overall Survival Prognostic Modelling of Non-small Cell Lung Cancer Patients Using Positron Emission Tomography/Computed Tomography Harmonised Radiomics Features: The Quest for the Optimal Machine Learning Algorithm. Clin Oncol (R Coll Radiol) 2022;34:114-27. [Crossref] [PubMed]
- Tan AC, Tan DSW. Targeted Therapies for Lung Cancer Patients With Oncogenic Driver Molecular Alterations. J Clin Oncol 2022;40:611-25. [Crossref] [PubMed]
- >Lung Cancer Survival Rates. American Cancer Society. Updated March 1, 2023. Accessed March 12, 2023. Available online: https://www.cancer.org/cancer/lung-cancer/detection-diagnosis-staging/survival-rates.html
- Galavis PE, Hollensen C, Jallow N, et al. Variability of textural features in FDG PET images due to different acquisition modes and reconstruction parameters. Acta Oncol 2010;49:1012-6. [Crossref] [PubMed]
- Zhao B. Understanding Sources of Variation to Improve the Reproducibility of Radiomics. Front Oncol 2021;11:633176. [Crossref] [PubMed]
- Boellaard R, Delgado-Bolton R, Oyen WJ, et al. FDG PET/CT: EANM procedure guidelines for tumour imaging: version 2.0. Eur J Nucl Med Mol Imaging 2015;42:328-54. [Crossref] [PubMed]
- Sullivan DC, Obuchowski NA, Kessler LG, et al. Metrology Standards for Quantitative Imaging Biomarkers. Radiology 2015;277:813-25. [Crossref] [PubMed]
- Buckler AJ, Bresolin L, Dunnick NR, et al. A collaborative enterprise for multi-stakeholder participation in the advancement of quantitative imaging. Radiology 2011;258:906-14. [Crossref] [PubMed]
- Yushkevich PA, Gao Yang, Gerig G. ITK-SNAP: An interactive tool for semi-automatic segmentation of multi-modality biomedical images. Annu Int Conf IEEE Eng Med Biol Soc 2016;2016:3342-5. [Crossref] [PubMed]
- Balagurunathan Y, Gu Y, Wang H, et al. Reproducibility and Prognosis of Quantitative Features Extracted from CT Images. Transl Oncol 2014;7:72-87. [Crossref] [PubMed]
- Velazquez ER, Parmar C, Jermoumi M, et al. Volumetric CT-based segmentation of NSCLC using 3D-Slicer. Sci Rep 2013;3:3529. [Crossref] [PubMed]
- Parmar C, Rios Velazquez E, Leijenaar R, et al. Robust Radiomics feature quantification using semiautomatic volumetric segmentation. PLoS One 2014;9:e102107. [Crossref] [PubMed]
- Lustberg T, van Soest J, Gooding M, et al. Clinical evaluation of atlas and deep learning based automatic contouring for lung cancer. Radiother Oncol 2018;126:312-7. [Crossref] [PubMed]
- Liu Y, Kim J, Qu F, et al. CT Features Associated with Epidermal Growth Factor Receptor Mutation Status in Patients with Lung Adenocarcinoma. Radiology 2016;280:271-80. [Crossref] [PubMed]
- Weiss GJ, Ganeshan B, Miles KA, et al. Noninvasive image texture analysis differentiates K-ras mutation from pan-wildtype NSCLC and is prognostic. PLoS One 2014;9:e100244. [Crossref] [PubMed]
- Vallières M, Freeman CR, Skamene SR, et al. A radiomics model from joint FDG-PET and MRI texture features for the prediction of lung metastases in soft-tissue sarcomas of the extremities. Phys Med Biol 2015;60:5471-96. [Crossref] [PubMed]
- Parmar C, Grossmann P, Bussink J, et al. Machine Learning methods for Quantitative Radiomic Biomarkers. Sci Rep 2015;5:13087. [Crossref] [PubMed]
- Li H, Galperin-Aizenberg M, Pryma D, et al. Unsupervised machine learning of radiomic features for predicting treatment response and overall survival of early stage non-small cell lung cancer patients treated with stereotactic body radiation therapy. Radiother Oncol 2018;129:218-26. [Crossref] [PubMed]
- Segal E, Sirlin CB, Ooi C, et al. Decoding global gene expression programs in liver cancer by noninvasive imaging. Nat Biotechnol 2007;25:675-80. [Crossref] [PubMed]
- Singh A, Horng H, Chitalia R, et al. Resampling and harmonization for mitigation of heterogeneity in image parameters of baseline scans. Sci Rep 2022;12:21505. [Crossref] [PubMed]
- Cha MJ, Lee HY, Lee KS, et al. Micropapillary and solid subtypes of invasive lung adenocarcinoma: clinical predictors of histopathology and outcome. J Thorac Cardiovasc Surg 2014;147:921-928.e2. [Crossref] [PubMed]
- Zhuo Y, Feng M, Yang S, et al. Radiomics nomograms of tumors and peritumoral regions for the preoperative prediction of spread through air spaces in lung adenocarcinoma. Transl Oncol 2020;13:100820. [Crossref] [PubMed]
- Mohiuddin K, Haneuse S, Sofer T, et al. Relationship between margin distance and local recurrence among patients undergoing wedge resection for small (≤2 cm) non-small cell lung cancer. J Thorac Cardiovasc Surg 2014;147:1169-75; discussion 1175-7. [Crossref] [PubMed]
- Wolf AS, Swanson SJ, Yip R, et al. The Impact of Margins on Outcomes After Wedge Resection for Stage I Non-Small Cell Lung Cancer. Ann Thorac Surg 2017;104:1171-8. [Crossref] [PubMed]
- van Velden FH, Kramer GM, Frings V, et al. Repeatability of Radiomic Features in Non-Small-Cell Lung Cancer [(18)F]FDG-PET/CT Studies: Impact of Reconstruction and Delineation. Mol Imaging Biol 2016;18:788-95. [Crossref] [PubMed]
- Tsujikawa T, Tsuyoshi H, Kanno M, et al. Selected PET radiomic features remain the same. Oncotarget 2018;9:20734-46. [Crossref] [PubMed]
- Liu J, Dong M, Sun X, et al. Prognostic Value of 18F-FDG PET/CT in Surgical Non-Small Cell Lung Cancer: A Meta-Analysis. PLoS One 2016;11:e0146195. [Crossref] [PubMed]
- Park JE, Kim D, Kim HS, et al. Quality of science and reporting of radiomics in oncologic studies: room for improvement according to radiomics quality score and TRIPOD statement. Eur Radiol 2020;30:523-36. [Crossref] [PubMed]
- Wang X, Zhao X, Li Q, et al. Can peritumoral radiomics increase the efficiency of the prediction for lymph node metastasis in clinical stage T1 lung adenocarcinoma on CT? Eur Radiol 2019;29:6049-58. [Crossref] [PubMed]