A CT-based radiomics integrated model for discriminating pulmonary cryptococcosis granuloma from lung adenocarcinoma—a diagnostic test
Highlight box
Key findings
• An integrated radiomics model could distinguish pulmonary cryptococcosis from adenocarcinoma.
What is known and what is new?
• Radiomics features has the potential to differentiate between benign and malignant pulmonary nodules.
• Radiomics combined with demoradiological features could improve the diagnostic performance in the differentiation of disease mimickers, with a multi-institution design.
What is the implication, and what should change now?
• This integrated model has the potential to help make better clinical decision, and need to be further validated and widely applied.
Introduction
Pulmonary cryptococcosis is a major opportunistic invasive mycosis caused predominantly by Cryptococcus neoformans or Cryptococcus gattii (1). While immunocompromised individuals are susceptible to pulmonary cryptococcal infections, approximately 30% of the population with normal immunity may not exhibit any significant symptoms or laboratory findings. Therefore, chest computed tomography (CT) serves as a critical tool in the diagnosis and evaluation of pulmonary cryptococcosis (2-5).
Evaluating patients with CT Chest for cryptococcosis can be challenging since these patients are commonly misdiagnosed with lung cancer due to similar radiological manifestations, such as pulmonary nodules, cavities, and enlarged lymph nodes (2-5). In 57–82% of pulmonary cryptococcosis cases, the disease manifests as a nodule or mass with necrosis and cavities, lobulation, and air bronchogram signs on CT (3,6), mimicking peripheral lung cancer. Unexperienced radiologists are more likely to misdiagnose. As a result, some patients undergo unnecessary surgeries with the risk of disseminated cryptococcal infection. A study of 18 fluorine-fluorodeoxyglucose (18F-FDG) positron emission tomography (PET)-CT revealed that approximately 88% of lung lesions in pulmonary cryptococcosis demonstrate high FDG uptake, mimicking a possible malignant condition (7), further limiting the use of PET in the diagnosis. Moreover, the treatment for patients with cryptococcal pneumonia requires antifungal therapy while prompt resection in patients with early-stage carcinoma usually improves prognosis. Therefore, it can be very helpful to differentiate the two diseases accurately with an effective non-invasive method.
Recently, radiomics has been gaining traction as it provides a quantitative method to identify the intrinsic heterogeneity of a lesion and ensures precise classification (8-10). Several previous studies have revealed radiomics analysis with the potential to differentiate between benign and malignant pulmonary nodules on noncontrast CT (11-16), including infectious diseases and carcinoma. For example, Beig et al. proposed a model combining radiomics features of intranodular and perinodular regions on lung CT images, and the accuracy of distinguishing adenocarcinomas from granulomas improved to 0.80 compared with the accuracy of 0.6 from two radiologists (17). However, only a few studies have focused on radiomics or computerized feature-based classification of fungal infections and carcinomas (16,18-20). Moreover, small sample sizes and single-center designs have rendered the results unconvincing. In this study, a multi-institution design with larger sample sizes were used to investigate the ability of a CT-based radiomics classifier to differentiate pulmonary cryptococcosis nodules and lung adenocarcinomas. We present this article in accordance with the TRIPOD reporting checklist (available at https://tlcr.amegroups.com/article/view/10.21037/tlcr-23-389/rc).
Methods
Study population and image acquisition
The study was conducted in accordance with the Declaration of Helsinki (as revised in 2013). The study was approved by institutional ethics committees of Shanghai Chest Hospital [No. KS(Y)1990] and the other clinical center was informed and agreed with this study. Individual consent for this retrospective analysis was waived. Noncontrast lung CT images were collected from two clinical centers (Huashan Hospital, Center I; Shanghai Chest Hospital, Center II) between 2015 and 2019, the imaging parameters are listed in Table S1.
Non-immunocompromised patients with pulmonary cryptococcosis confirmed by histopathologic examination via surgical resection or biopsy were screened using the electronic medical record, and cases with lung adenocarcinoma were randomly selected and matched with the control group at a ratio of 1:3. The inclusion criteria were as follows: available baseline preoperative noncontrast CT scans demonstrating solid pulmonary nodules; pulmonary nodules <4 cm in diameter; and a reconstructed slice thickness of CT scans <1.5 mm. The exclusion criteria were as follows: pathologically confirmed cryptococcus combined with other pathogen infections; pulmonary nodules manifested as ground-glass opacities (GGOs); and poor image quality or artifacts.
Data for 215 patients were collected from the two centers. The data for 108 patients from Center I constituted the training set for the model (31 pulmonary cryptococcoses and 77 lung cancer). Data for 107 patients from Center II were used as an independent test set to assess model performance (53 pulmonary cryptococcoses and 54 lung adenocarcinomas) (Figure 1).
CT image annotation and feature extraction
Three types of volumes of interest (VOIs) were generated, as indicated in Figure 2. Lesions were manually delineated by a radiologist (Hu B, with 7 years of experience) using three-dimensional (3D) slicer software (version 4.8.1), and each lesion volume (LV) of interest was obtained. Then, the extended lesion volume (ELV) of interest was generated using the dilation operation to extend the boundary of the LV by 5 mm. Regions of tissues outside the lung were excluded from the ELV by manual correction. In addition, the perilesion volume (PLV) of interest was obtained by subtracting the LV from the ELV.
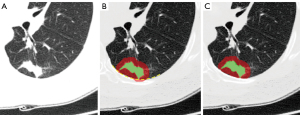
Radiomics features were extracted from the LV, ELV, and PLV. For each type of VOI, 851 radiomics features were extracted and classified as follows (21): (I) 14 shape features describe the geometric information of the VOI; (II) 18 statistical features represent the characteristics of the intensity histogram within the VOI; (III) 75 texture features quantify the spatial heterogeneity of the intensity within the VOI; and (IV) 744 wavelet features derived from the wavelet filtered images highlight the local details or approximate shape of the images. The radiomics features were extracted using the open-source tool Pyradiomics (version 2.1.2), and the details can be found at the public website (https://pyradiomics.readthedocs.io/en/2.1.2/features.html).
Radiomics model building
Radiomics models were built for LV, ELV, and PLV (denoted as MRads_LV, MRads_ELV, and MRads_PLV, respectively); the procedure included two steps: feature selection and model training.
For feature selection, Spearman’s correlation coefficient (SCC) of each radiomics feature pair was calculated. The radiomics feature pair with SCC >0.9 was considered to be highly correlated (22). In the feature pair, the feature with a high mean SCC was considered redundant and therefore was eliminated. To decrease the risk of overfitting, the minimum redundancy maximum relevance (mRMR) feature ranking algorithm was used to score each feature according to its importance in discriminating the two diseases (23). Only the top-ranking features were selected for model building, and the number of features was limited to 1/10th of the number of cases (24).
Selected radiomics features were input in the least absolute shrinkage and selection operator (LASSO) for radiomics model building (25). L1 regularization in logistic regression facilitated LASSO-mediated compression of the selected features, thereby improving the performance of the radiomics model. The hyperparameter λ controlled the degree of feature compression. For each type of VOI, a radiomics model was trained by LASSO, and the hyperparameter λ was determined using fivefold cross-validation in the training set. The radiomics model was built as the linear weighted sum of the radiomics features.
Demoradiological and integrated models
Demographic features, including age and sex, were collected. Radiological features, including the location, number and shape of lesions and whether the lesions had the spicular sign, lobular sign, air bronchus sign, pseudocavitation, vascular convergence sign, halo sign, calcification, satellite lesions, or pleural change were documented by two qualified radiologists (Li Y and Zhu L, each with 20 years of experience) blinded to the diagnosis. Univariate logistic regression was used to assess the disease-discriminative ability of each demographic and radiological feature. The significant features identified by univariate logistic regression were further input into multivariate logistic regression to identify independent discriminative features and build a demoradiological model, Mdemoradiological.
The integrated model Mintegrated was built by combining the predictions of the radiomics model and the independent discriminative demoradiological features using multivariate logistic regression.
Model testing and statistical analysis
Performance of the models was assessed in the independent test set. Moreover, two radiologists (Tang Y and Li Y, with 10 and 20 years of experience, respectively) blinded to the histological results were invited to evaluate the pulmonary nodules on a scale of 1–4, indicating benign to malignant. The test performances of the models and radiologists were quantified by the area under the receiver operating characteristic (ROC) curve (AUC), accuracy (ACC), sensitivity (SEN) and specificity (SPE). Differences between the ROC curves were assessed using the DeLong test (26). The Mann-Whitney U test was used for numerical characteristics in statistical tests of demographics, and the Fisher’s exact test was applied for categorical characteristics. P<0.05 was considered statistically significant. The feature selection, model building, model performance test, and statistical analysis were conducted using R (v. 3.6.2, https://www.r-project.org/).
Results
Demographic information of subjects
The patients’ profiles are listed in Table 1. The mean age of the patients with pulmonary cryptococcosis was significantly younger than that of the patients with lung adenocarcinoma in both the training and test sets. The sex distribution did not show a significant difference between the two types of diseases in both sets.
Table 1
Variables | Training set | Test set | |||||
---|---|---|---|---|---|---|---|
Pulmonary cryptococcosis (n=31) | Lung cancer (n=77) | P value | Pulmonary cryptococcosis (n=53) | Lung cancer (n=54) |
P value | ||
Age (years), mean ± SD | 46.42±12.52 | 59.74±9.15 | <0.001 | 53.60±10.58 | 60.87±7.94 | <0.001 | |
Sex | 0.490 | 0.485 | |||||
Male | 18 | 39 | 20 | 24 | |||
Female | 13 | 38 | 33 | 30 |
SD, standard deviation.
Diagnostic performance of the radiomics model
A total of 851 radiomics features were extracted from each kind of VOI. Among these, 4, 8, and 11 radiomics features were selected and trained for the LV-, ELV-, and PLV-based radiomics models, respectively. The formula of the radiomics models was as follows:
The AUCs of the LV-based radiomics model (MRads_LV) and the PLV-based radiomics model (MRads_PLV) were 0.558 and 0.470, respectively. Compared to the former, the ELV-based radiomics model (MRads_ELV) achieved a higher AUC of 0.757.
Diagnostic performance of the demoradiological model
The details of the radiological features that appeared in each case were documented and summarized for both the training and test sets (Table S2). Univariate logistic regression demonstrated that the significant demoradiological features predicting disease classification were age, number of lesions, spicular sign, lobular sign, and satellite lesions. Results of multivariate logistic regression identified that age, number, and lobular sign were independent discriminative features. The diagnostic performance of the demoradiological model is shown in Table 2. The AUC of Mdemoradiological was 0.770, and the formula of Mdemoradiological was:
Table 2
Feature | Univariate | Multivariate | |||
---|---|---|---|---|---|
OR (95% CI) | P value | OR (95% CI) | P value | ||
Age | 1.14 (1.07–1.21) | <0.001 | 1.15 (1.07–1.23) | <0.001 | |
Sex | 0.74 (0.32–1.72) | 0.486 | – | – | |
Upper lobe | – | – | – | – | |
Middle lobe | 0.92 (0.17–5.05) | 0.920 | – | – | |
Lower lobe | 0.41 (0.17–0.99) | 0.050 | – | – | |
Number of lesions | 0.09 (0.03–0.3) | <0.001 | 0.07 (0.01–0.38) | 0.002 | |
Shape (irregular, regular) | 1.15 (0.5–2.68) | 0.739 | – | – | |
Spicular sign | 3.09 (1.26–7.58) | 0.014 | 1.37 (0.39–4.82) | 0.628 | |
Lobular sign | 3.63 (1.48–8.92) | 0.005 | 7.98 (1.99–32.01) | 0.003 | |
Air bronchus sign | 1.67 (0.6–4.62) | 0.326 | – | – | |
Pseudocavitation | 1.36 (0.45–4.11) | 0.582 | – | – | |
Calcification | – | 0.992 | – | – | |
Halo sign | 0.53 (0.19–1.46) | 0.220 | – | – | |
Satellite lesions | 0.19 (0.05–0.7) | 0.013 | 0.21 (0.04–1.16) | 0.073 | |
Vascular convergence sign | 4.11 (0.89–18.99) | 0.070 | – | – | |
Pleural change | – | – | – | – | |
Indentation | 1.65 (0.64–4.22) | 0.296 | – | – | |
Attachment | 1.32 (0.37–4.73) | 0.670 | – | – |
The OR of location was calculated by considering the upper lobe as a reference. The OR of calcification could not be calculated, as only one patient in each dataset (with lung adenocarcinoma) had calcification. OR, odds ratio; CI, confidence interval.
Diagnostic performance of the integrated model
Combining the predictions of the radiomics model and the independent discriminative demoradiological features, an integrated model (Mintegrated) was constructed. It achieved an AUC of 0.801, which was the highest AUC among all models. The formula of Mintegratedwas:
PredictionRads_ELV is the prediction of MRads_ELV.
Comparison of diagnostic ability between classifier models and radiologists
In terms of the diagnostic performance of radiologists, AUCs were 0.784 and 0.689 for the senior and junior radiologists, respectively. The AUC of MRads_ELV was compared to the AUC of Mdemo-radiological (P=0.579), radiologist 1 (P=0.165), and radiologist 2 (P=0.654). The AUC of Mintegrated was significantly higher than that of radiologist 1 (P=0.024) and Mdemo-radiological (P=0.015) but had not significantly different from that of radiologist 2 (P=0.388).
The AUC, accuracy, sensitivity, and specificity of each model and two radiologists are listed in Table 3. ROC curves are illustrated in Figure 3.
Table 3
Model/radiologist | AUC | ACC | SEN | SPE |
---|---|---|---|---|
MRads_LV | 0.558 | 0.383 | 0.296 | 0.472 |
MRads_ELV | 0.757 | 0.757 | 0.704 | 0.811 |
MRads_PLV | 0.470 | 0.523 | 0.167 | 0.887 |
Mdemoradiological | 0.770 | 0.729 | 0.815 | 0.642 |
Mintegrated | 0.801 | 0.757 | 0.759 | 0.755 |
Radiologist 1 | 0.689 | 0.654 | 0.889 | 0.415 |
Radiologist 2 | 0.784 | 0.720 | 0.852 | 0.585 |
AUC, area under the receiver operating characteristic curve; ACC, accuracy; SEN, sensitivity; SPE, specificity; LV, lesion volume; ELV, extended lesion volume; PLV, perilesion volume.
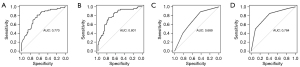
Among 107 cases in the external test set, 20 were correctly classified by the integrated model but misdiagnosed by at least one radiologist. Sixteen cases were classified incorrectly by the model but correctly diagnosed by at least one radiologist. Six cases of pulmonary cryptococcosis showed irregular shape, spiculation sign, air bronchi sign, and vascular convergence sign but were neither accurately predicted by the classifier model nor the radiologists. The representative easy-mimicking pulmonary nodules are shown in Figure 4.
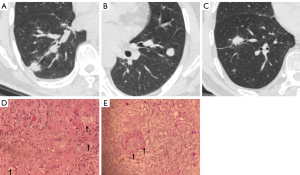
Discussion
In clinical practice, distinguishing the solid pulmonary nodules of cryptococcosis from carcinomas on CT is challenging. In this radiomics study with 215 cases, we constructed an integrated model combining radiomics, demographic, and radiological features, which achieved an AUC of 80.1% in the independent test set. The performance of the integrated model surpassed the level of the senior radiologist or the model based on traditional radiological features.
Lung squamous cell carcinoma typically presents as a central lung cancer and is often accompanied by necrosis or cavitation. These imaging features are different from those of pulmonary cryptococcosis. According to the location and characteristics of the disease, a large overlap between pulmonary adenocarcinoma and cryptococcosis manifested as solid nodules can be observed. Therefore, we enrolled only adenocarcinoma cases in the present study. For radiologists, recognizing radiological features is critical in differentiating between pulmonary cryptococcosis and lung cancer. Liu et al. demonstrated that clinically relevant radiological features, including attachment to the vessel, nodule location, border definition, and concavity, could effectively determine the lung cancer risk of solitary pulmonary nodules (27). Several studies have also revealed typical signs of pulmonary cryptococcosis, which can be noted as pulmonary nodules with segmental or lobar consolidation or a reticulonodular pattern of opacities (4,6,28,29). However, there is some overlap between the two diseases. Compared to lung cancer, nodules with cryptococcosis are more common in the outer field of the lung, with lower tension, rare deep lobulation, and more air bronchogram signs (5). In the current study, radiological features, such as lobular signs, satellite lesions, and vascular convergence signs, were shown to be significantly different between the two groups after logistic regression, consistent with previous studies (28,30-32). Moreover, when the age factor was considered, the AUC improved to 0.770, which was even better than the performance of a junior radiologist. These results suggest the importance of precise identification of radiological features in diagnosis which may be challenging for radiologists with limited experience.
Therefore, with a growing need for practical and automated means, radiomics has become a solution. Lesion segmentation is a principal procedure in construction of a radiomics classifier model. In this study, we defined three types of VOIs (LV, ELV, and PLV) following the method of Beig et al. (17); the ELV-based radiomics model showed better performance than the others, indicating that the perinodular region could possess valuable information for improving the efficiency of intranodular radiomics analysis. Among radiomics studies related to chest CT, the majority have focused solely on lung nodule shape and texture analysis (11,33,34). Nonetheless, information in the nodular-surrounding tissues, including characteristics of tumor-infiltrating lymphocytes and tumor-associated stromal macrophages not reflected in the lesion, is yet to be determined (11). Thus, assessment of the entire tumor together with the surrounding tissue might provide complementary information, which is crucial for accurate diagnosis.
In addition to VOI selection, coalescing of information is a strategy for improving classifier performance. In this study, discrimination based on integrated models combined with demographic, radiological, and radiomics features was superior to any other models, indicating that human-machine integration is important for decision-making. Additionally, it is challenging to identify subtle differences in image features by visualization, even for senior radiologists, and radiomics signatures based on quantitative parameters can be helpful. Moreover, radiological features such as lesion number and location provide valuable information at a “global” level; some unique manifestations such as “satellite lesions” are also key clues in the diagnosis. On the other hand, it is challenging for machines alone to conduct precise identification between two diseases, rendering the experience of expert radiologists critical with respect to traditional qualitative features and in interpreting low-quality imaging data (35).
The present study has several limitations. First, we only considered age and sex as demographic features. If other risk factors, such as smoking history and epidemiological history, are considered, the diagnostic performance of the model could be improved. Second, the case cohort is not generalizable, although a large number of cases were collected in two clinical centers, the sample size of pulmonary cryptococcus infection was small due to its low incidence rate, and a further external validation is needed. Third, although the integrated model provided a combination of experts’ experiences and imaging features, it is still a semiautomatic method. In terms of feasibility and practicality, whole-lung automated recognition methods might dominate in the future.
Conclusions
In conclusion, radiomics features of pulmonary nodules can distinguish cryptococcosis granulomas from solid adenocarcinomas on noncontrast CT and improve classification accuracy. Additionally, incorporating perinodular texture features with intranodular texture improves the diagnostic performance of our novel classifier. Importantly, machine complementation of humans could be an effective strategy in the differentiation of disease mimickers.
Acknowledgments
Funding: This work was supported by grants from
Footnote
Reporting Checklist: The authors have completed the TRIPOD reporting checklist. Available at https://tlcr.amegroups.com/article/view/10.21037/tlcr-23-389/rc
Data Sharing Statement: Available at https://tlcr.amegroups.com/article/view/10.21037/tlcr-23-389/dss
Peer Review File: Available at https://tlcr.amegroups.com/article/view/10.21037/tlcr-23-389/prf
Conflicts of Interest: All authors have completed the ICMJE uniform disclosure form (available at https://tlcr.amegroups.com/article/view/10.21037/tlcr-23-389/coif). AW has done some consulting work for Noah Medical, Ambu, and Medtronic, and receives payment or honoraria for speaker bureaus for Biodesix, and also done some medicolegal work in the area of lung nodules. The other authors have no conflicts of interest to declare.
Ethical Statement: The authors are accountable for all aspects of the work in ensuring that questions related to the accuracy or integrity of any part of the work are appropriately investigated and resolved. The study was conducted in accordance with the Declaration of Helsinki (as revised in 2013). The study was approved by institutional ethics committees of Shanghai Chest Hospital [No. KS(Y)1990] and the other clinical center was informed and agreed with this study. Individual consent for this retrospective analysis was waived.
Open Access Statement: This is an Open Access article distributed in accordance with the Creative Commons Attribution-NonCommercial-NoDerivs 4.0 International License (CC BY-NC-ND 4.0), which permits the non-commercial replication and distribution of the article with the strict proviso that no changes or edits are made and the original work is properly cited (including links to both the formal publication through the relevant DOI and the license). See: https://creativecommons.org/licenses/by-nc-nd/4.0/.
References
- Setianingrum F, Rautemaa-Richardson R, Denning DW. Pulmonary cryptococcosis: A review of pathobiology and clinical aspects. Med Mycol 2019;57:133-50. [Crossref] [PubMed]
- Deng H, Zhang J, Li J, et al. Clinical features and radiological characteristics of pulmonary cryptococcosis. J Int Med Res 2018;46:2687-95. [Crossref] [PubMed]
- Qu Y, Liu G, Ghimire P, et al. Primary pulmonary cryptococcosis: evaluation of CT characteristics in 26 immunocompetent Chinese patients. Acta Radiol 2012;53:668-74. [Crossref] [PubMed]
- Fox DL, Müller NL. Pulmonary cryptococcosis in immunocompetent patients: CT findings in 12 patients. AJR Am J Roentgenol 2005;185:622-6. [Crossref] [PubMed]
- Zhang Y, Li N, Zhang Y, et al. Clinical analysis of 76 patients pathologically diagnosed with pulmonary cryptococcosis. Eur Respir J 2012;40:1191-200. [Crossref] [PubMed]
- Xie LX, Chen YS, Liu SY, et al. Pulmonary cryptococcosis: comparison of CT findings in immunocompetent and immunocompromised patients. Acta Radiol 2015;56:447-53. [Crossref] [PubMed]
- Wang SY, Chen G, Luo DL, et al. (18)F-FDG PET/CT and contrast-enhanced CT findings of pulmonary cryptococcosis. Eur J Radiol 2017;89:140-8. [Crossref] [PubMed]
- Lee SH, Lee SM, Goo JM, et al. Usefulness of texture analysis in differentiating transient from persistent part-solid nodules (PSNs): a retrospective study. PLoS One 2014;9:e85167. [Crossref] [PubMed]
- Chae HD, Park CM, Park SJ, et al. Computerized texture analysis of persistent part-solid ground-glass nodules: differentiation of preinvasive lesions from invasive pulmonary adenocarcinomas. Radiology 2014;273:285-93. [Crossref] [PubMed]
- Fan L, Fang M, Li Z, et al. Radiomics signature: a biomarker for the preoperative discrimination of lung invasive adenocarcinoma manifesting as a ground-glass nodule. Eur Radiol 2019;29:889-97. [Crossref] [PubMed]
- Coroller TP, Grossmann P, Hou Y, et al. CT-based radiomic signature predicts distant metastasis in lung adenocarcinoma. Radiother Oncol 2015;114:345-50. [Crossref] [PubMed]
- Feng B, Chen X, Chen Y, et al. Radiomics nomogram for preoperative differentiation of lung tuberculoma from adenocarcinoma in solitary pulmonary solid nodule. Eur J Radiol 2020;128:109022. [Crossref] [PubMed]
- Wu L, Gao C, Xiang P, et al. CT-Imaging Based Analysis of Invasive Lung Adenocarcinoma Presenting as Ground Glass Nodules Using Peri- and Intra-nodular Radiomic Features. Front Oncol 2020;10:838. [Crossref] [PubMed]
- Uthoff J, Nagpal P, Sanchez R, et al. Differentiation of non-small cell lung cancer and histoplasmosis pulmonary nodules: insights from radiomics model performance compared with clinician observers. Transl Lung Cancer Res 2019;8:979-88. [Crossref] [PubMed]
- Khorrami M, Bera K, Thawani R, et al. Distinguishing granulomas from adenocarcinomas by integrating stable and discriminating radiomic features on non-contrast computed tomography scans. Eur J Cancer 2021;148:146-58. [Crossref] [PubMed]
- Chen X, Feng B, Chen Y, et al. A CT-based radiomics nomogram for prediction of lung adenocarcinomas and granulomatous lesions in patient with solitary sub-centimeter solid nodules. Cancer Imaging 2020;20:45. [Crossref] [PubMed]
- Beig N, Khorrami M, Alilou M, et al. Perinodular and Intranodular Radiomic Features on Lung CT Images Distinguish Adenocarcinomas from Granulomas. Radiology 2019;290:783-92. [Crossref] [PubMed]
- Alilou M, Prasanna P, Bera K, et al. A Novel Nodule Edge Sharpness Radiomic Biomarker Improves Performance of Lung-RADS for Distinguishing Adenocarcinomas from Granulomas on Non-Contrast CT Scans. Cancers (Basel) 2021;13:2781. [Crossref] [PubMed]
- Zhang Y, Chu Z, Yu J, et al. Computed tomography-based radiomics for identifying pulmonary cryptococcosis mimicking lung cancer. Med Phys 2022;49:5943-52. [Crossref] [PubMed]
- Zhang ZX, Mu XY, Yu J, et al. Establishment and evaluation of a CT-based radiomic model for AIDS-associated pulmonary cryptococcosis. BMC Med Imaging 2022;22:185. [Crossref] [PubMed]
- van Griethuysen JJM, Fedorov A, Parmar C, et al. Computational Radiomics System to Decode the Radiographic Phenotype. Cancer Res 2017;77:e104-7. [Crossref] [PubMed]
- Xia W, Hu B, Li H, et al. Multiparametric-MRI-Based Radiomics Model for Differentiating Primary Central Nervous System Lymphoma From Glioblastoma: Development and Cross-Vendor Validation. J Magn Reson Imaging 2021;53:242-50. [Crossref] [PubMed]
- Peng H, Long F, Ding C. Feature selection based on mutual information: criteria of max-dependency, max-relevance, and min-redundancy. IEEE Trans Pattern Anal Mach Intell 2005;27:1226-38. [Crossref] [PubMed]
- Nie K, Shi L, Chen Q, et al. Rectal Cancer: Assessment of Neoadjuvant Chemoradiation Outcome based on Radiomics of Multiparametric MRI. Clin Cancer Res 2016;22:5256-64. [Crossref] [PubMed]
- Friedman J, Hastie T, Tibshirani R. Regularization Paths for Generalized Linear Models via Coordinate Descent. J Stat Softw 2010;33:1-22.
- DeLong ER, DeLong DM, Clarke-Pearson DL. Comparing the areas under two or more correlated receiver operating characteristic curves: a nonparametric approach. Biometrics 1988;44:837-45.
- Liu Y, Wang H, Li Q, et al. Radiologic Features of Small Pulmonary Nodules and Lung Cancer Risk in the National Lung Screening Trial: A Nested Case-Control Study. Radiology 2018;286:298-306. [Crossref] [PubMed]
- Soubani AO. The evaluation and management of the solitary pulmonary nodule. Postgrad Med J 2008;84:459-66. [Crossref] [PubMed]
- MacMahon H, Naidich DP, Goo JM, et al. Guidelines for Management of Incidental Pulmonary Nodules Detected on CT Images: From the Fleischner Society 2017. Radiology 2017;284:228-43. [Crossref] [PubMed]
- Wu W, Pierce LA, Zhang Y, et al. Comparison of prediction models with radiological semantic features and radiomics in lung cancer diagnosis of the pulmonary nodules: a case-control study. Eur Radiol 2019;29:6100-8. [Crossref] [PubMed]
- Edey AJ, Hansell DM. Incidentally detected small pulmonary nodules on CT. Clin Radiol 2009;64:872-84. [Crossref] [PubMed]
- Gould MK, Ananth L, Barnett PG, et al. A clinical model to estimate the pretest probability of lung cancer in patients with solitary pulmonary nodules. Chest 2007;131:383-8. [Crossref] [PubMed]
- Huang Y, Liu Z, He L, et al. Radiomics Signature: A Potential Biomarker for the Prediction of Disease-Free Survival in Early-Stage (I or II) Non-Small Cell Lung Cancer. Radiology 2016;281:947-57. [Crossref] [PubMed]
- He L, Huang Y, Ma Z, et al. Effects of contrast-enhancement, reconstruction slice thickness and convolution kernel on the diagnostic performance of radiomics signature in solitary pulmonary nodule. Sci Rep 2016;6:34921. [Crossref] [PubMed]
- Lee G, Lee HY, Park H, et al. Radiomics and its emerging role in lung cancer research, imaging biomarkers and clinical management: State of the art. Eur J Radiol 2017;86:297-307. [Crossref] [PubMed]