Construction and validation of a novel ferroptosis-related prognostic signature for lung adenocarcinoma
Highlight box
Key findings
• A ferroptosis-related prognostic signature for LUAD was constructed.
What is known and what is new?
• Ferroptosis generates a complex role in the pathogenesis of cancer and anticancer treatment.
• A novel ferroptosis-related prognostic signature showed potential clinical function in LUAD.
What is the implication, and what should change now?
• Further research on ferroptosis as a functional and therapeutic target in LUAD is warranted.
Introduction
Lung cancer, the major cause of cancer-associated death worldwide (1), is classified into two broad histological subtypes, including small-cell lung cancer (SCLC) and non-small cell lung cancer (NSCLC). NSCLC accounts for 85% of all lung cancer cases (2). Lung adenocarcinoma (LUAD) is the predominant form of NSCLC (1). Despite improvements in lung cancer survival rates due to gene-targeted therapies and immunotherapies (3), intrinsic and acquired resistance are inevitable, driving the clinical need to identify new prognostic and therapeutic targets for LUAD treatment.
Ferroptosis refers to one type of programmed cell death based on Fenton reaction-induced lipid peroxidation (4). Reactive oxygen species (ROS) (oxygen in partial reduction forms) production contributes to oxidized cellular membrane lipids, especially polyunsaturated fatty acid (PUFA)-containing phospholipids (PUFA-PLs), causing lipid peroxidation and ferroptosis (4,5). Recently, the relevance of ferroptosis in multiple pathological processes, including cardiomyopathy (6), hemochromatosis (7), pulmonary fibrosis (8), nervous system diseases (9,10), and sepsis (11), has been reported. In addition, according to increasing evidence, ferroptosis plays a critical role in malignant tumor pathogenesis (12,13), with great prognostic and therapeutic interest. Furthermore, ferroptosis shows a close relationship to immunosuppression in the tumor microenvironment and drug resistance cancer (14,15). However, the prognostic significance of ferroptosis in LUAD is largely unknown.
In this study, we aimed to investigate the role of ferroptosis-related genes (FRGs) as a prognostic marker for LUAD to construct a new prognostic signature in accordance with FRGs through the use of a large-scale public database. Gene Ontology (GO) and Kyoto Encyclopedia of Genes and Genomes (KEGG) analyses were conducted with the aim of investigating the potential mechanism underlying the prognostic relevance of ferroptosis. We propose potential immune subtypes and drug sensitivity based on the FRGs cluster analysis that may lead to improved treatment for LUAD.
Notably, ferroptosis is cellular membranes lipid hydroperoxide accumulation-related cell death. The sensitivity to ferroptosis is tightly linked to PUFA metabolism (8,14). During the FRGs, the long-chain fatty acyl CoA synthetase (ACSL3) and peroxiredoxin-6 (PRDX6) participated in cell membrane phospholipid metabolism (16,17). Thus, manipulating ACSL3 or PRDX6 may limit tumor development and could be harnessed for cancer therapy through ferroptosis. To further verification of its role in the ferroptosis of lung cancer, we demonstrated the higher expression of ACSL3 and PRDX6 in paired LUAD tumors and non-cancer samples. The sensitivity to ferroptosis inducers and lipid peroxidation was also tested under the siRNA silencing ACSL3 or PRDX6. We present this article in accordance with the TRIPOD reporting checklist (available at https://tlcr.amegroups.com/article/view/10.21037/tlcr-23-351/rc).
Methods
Study design and data accession
The Cancer Genome Atlas (TCGA) database (https://tcga-data.nci.nih.gov/tcga/) provided RNA-seq and clinical information of LUAD cases. By removing patients whose survival times were <30 days and duplicate samples, we selected a total of 493 pathologically confirmed LUAD patients. Subsequently, TCGA-LUAD patients were randomized as an experimental group and a validation group at a 1:1 ratio. FRGs were obtained at the FerrDb website (http://www.zhounan.org/ferrdb/) (18), and 382 FRGs were chosen after excluding the noncoding genes.
Prognostic signature establishment and verification
Univariate Cox regression was conducted to detect the FRGs correlated with survival outcomes in the experimental cohort with P<0.05. Least Absolute Shrinkage and Selection Operator (LASSO) regression was conducted using the R package “glmnet” based on univariate Cox regression results, we chose an optimal λ value (λ=7) to minimize overfitting. Multivariate Cox regression analysis was conducted based on LASSO regression and survival outcomes. Then seven FRGs significantly related to prognosis were detected to establish a risk signature in accordance with the RNA expression and coefficient. A Cox model was adopted for building the prognosis signature, and risk scores of cases were computed with “survival” R package, where risk score = coefficient gene-1 × gene-1 level + coefficient gene-2 × gene-2 level +.... + coefficient gene-n × gene-n level. Patients were then divided into low- and high-risk groups according to their mean risk score.
Univariate Cox regression, multivariate regression, the receiver operating characteristic (ROC), and Kaplan-Meier (KM) curves were used for evaluating prognostic capacity in the experimental group, the validation group, and the entire cohort of the signature with the “survival”, “survivalROC” and “survminer” R packages, respectively.
Construction of a predictive nomogram
To determine whether the clinical feature (age, gender, risk score, and stage) or the prognostic signature independently predicted LUAD prognosis, univariate and multivariate Cox analyses were employed. Consistent with the findings of the independent prognostic factor analysis, R packages “regplot” and “rms” were used for plotting calibration curves together with nomograms, and prognostic factors were provided with specific scores. To generate the overall risk score, we supplemented the score for every prognostic factor, and the overall risk score was adopted for the prediction of LUAD survival at 1, 2, and 3 years. Additionally, to demonstrate the accuracy of the nomogram, the calibration curve was employed.
Functional enrichment analysis
The R packages “scatterplot3d” and “limma” were adopted to perform principal component analysis (PCA) on risk-related genes, all genes, and FRGs based on the LUAD dataset, and results were visualized by creating three-dimensional (3D) scatter plots. GO and KEGG analyses were made in R package “clusterProfiler”, for detecting key genes and pathways engaged in LUAD based on differentially expressed genes (DEGs) in both risk groups, with cutoff values of false discovery rate (FDR) <0.05 and log2-fold change (log2FC) >1.
Investigation of immune cell infiltration, immunotherapy, and drug screening
Transcriptomic data of LUAD patients were downloaded from the TCGA database.
The reference gene expression signatures were uploaded to the CIBERSORT (https://cibersortx.stanford.edu) (19) and the immune infiltration status was investigated. LM22 gene signature, which may distinguish 22 human hematopoietic cell phenotypes, was used to calculate the ratio of 22 immune cells of both risk groups of LUAD. The RNA expression level of crucial immunomodulators of both groups was also analyzed by nonparametric tests.
This study investigated the response to small molecule inhibitors in LUAD cases of both risk groups. For obtaining new target candidates for LUAD patients, we calculated the half maximal inhibitory concentration (IC50) through the R “pRRophetic” package with the aim of evaluating the response to small molecule inhibitors.
Cell culture
A549 and H1299 cells, acquired in the cell bank of the Chinese Academy of Science (Shanghai, China), have been cultured in a 37 °C incubator with 5% concentration of CO2. Cells were kept in Dulbecco’s modified Eagle’s medium (DMEM, Gibco) added with 10% fetal bovine serum (Biological Industries, Israel), and 1% penicillin-streptomycin (Biological Industries, Israel).
Small interfering RNA (siRNA) transfection
Following the manufacturer’s protocol, siRNA targeting ACSL3 and PRDX6 were synthesized by GenePharma and transfected with lipofectamine 3000 (L3000015, Invitrogen).
Cell viability assay
After inoculating cells (5,000 per well) into the 96-well plates, the ferroptosis inducer RSL3 (2.5 µM for H1299, 8 µM for A549, T3646, Top science) or co-treatment with the ferroptosis inhibitor ferrostatin-1 (Fer-1, 2 µM, T6500, Top science) was added 6 hours, then cell viability was measured using a Cell Counting Kit-8 (CCK-8) (C0005, Top Science). Plates were incubated to 60-min CCK8, followed by reading at 450 nm. All experiments were repeated at least three times.
Lipid peroxidation assay
Compounds [RSL3 or co-treatment with the ferroptosis inhibitor liproxstatin-1 (Lip-1, 2 µM, S7699, Selleck)] (8) were added for cell treatment. After collection, cells were rinsed with PBS and resuspended within PBS including BODIPY-C11 581/591 (5 µM, D3861, Thermo Fisher Scientific). Lipid peroxidation was evaluated by adopting the flow cytometer Becton Dickinson FACS Calibur machine with a 488 nm laser By using FlowJo, the data was analyzed.
Western blot (WB)
The preparation of whole cell/tissue lysates was performed by RIPA buffer with a cocktail (1:100). Protein separation was completed with SDS/PAGE gel, followed by transfer to NC membranes and block with 5% non-fat dry milk for 1 hour, which were subject to incubation through the night with the corresponding primary antibodies at 4 °C. The primary antibodies included ACSL3 (#20710-1-AP from Proteintech, 1:1,000), PRDX6 (#13585-1-AP from Proteintech, 1:1,000), HSP90 (TA-12 from ZSGB-BIO, 1:2,000), β actin (TA-09 from ZSGB-BIO, 1:2,000), and GAPDH (R24404 from Zenbio, 1:2,000). Subsequently, secondary antibodies conjugated with HRP (Jackson ImmunoResearch Laboratories, 1:5,000) and enhanced chemiluminescence solution (Tanon) were applied to detect protein expression signals.
ACSL3 and PRDX6 protein levels were measured by WB analysis. The expression patterns of ACSL3 and PRDX6 were validated in paired cancer and matched normal samples in LUAD cases. The samples had to meet the following inclusion criteria: (I) age >18; (II) pathologically confirmed with LUAD; (III) the patients included had no other serious systemic comorbidities. From April 2021 to April 2022, 15 LUAD and matched healthy lung tissues were acquired at Zhengzhou University People’s Hospital. The information of the LUAD patients was listed in the Table S1.
Immunohistochemistry (IHC) staining
After fixation within 4% paraformaldehyde, tumor tissue was paraffin-embedded sectioned, and later stained with the corresponding antibody (ACSL3, #20710-1-AP from Proteintech, 1:200). Three samples of LUAD tumor tissues were selected from Zhengzhou University People’s Hospital and the inclusion criteria was as listed before (Table S1).
Statistical analysis
Continuous data were compared with t-tests, and proportion was compared with Chi-squared tests. The Wilcox test was used for paired-data analysis, and the log-rank test was employed to test the significance of KM curves. Statistical analyses were completed using R version 4.2.0 and using this or GraphPad Prism version 9 graphs were drawn.
Ethics approval and consent to participate
The study was conducted in accordance with the Declaration of Helsinki (as revised in 2013). The study was approved by the Ethics Committee of Zhengzhou University People’s Hospital (No. 2021-27) and informed consent was taken from all the patients.
Results
A ferroptosis-related prognostic signature in LUAD
Figure 1 shows the flow chart of the study, and Table 1 lists the clinical characteristics of LUAD patients in the experimental and validation cohorts. Totally, 382 FRGs were chosen. In the experimental cohort, univariate Cox analyses were adopted for identifying FRGs correlated with the outcome of LUAD patients and 20 prognostic genes were revealed. LASSO was carried out following univariate Cox analysis, and 14 genes were identified by an optimal value of λ (Figure 2A,2B). Seven genes were selected for modeling by multivariate Cox regression analysis, including PRDX6, ACSL3, aryl hydrocarbon receptor nuclear translocator-like protein 1 (ARNTL), carbonic anhydrase 9 (CA9), DNA damage induced transcript 4 (DDIT4), RELA proto-oncogene (RELA), and 12R-lipoxygenase (ALOX12B). Calculation of the risk score was indicated below: risk score = (0.354 × level of PRDX6) + (0.237 × level of ACSL3) + (−0.547 × level of ARNTL) + (0.080 × level of CA9) + (0.179 × level of DDIT4) + (0.699 × level of RELA) + (0.281 × level of ALOX12B). The forest map and HR values of the seven genes are shown in Figure 2C. The level of PRDX6, ACSL3, CA9, DDIT4, RELA, and ALOX12B was associated with a higher risk of death (HR >1), while ARNTL was associated with a lower risk of death (HR <1). Moreover, KM analysis revealed a lower expression of ARNTL or a higher expression of PRDX6, ACSL3, CA9, RELA, ALOX12B, and DDIT4 was related to the worse survival outcome (Figure S1A-S1G).
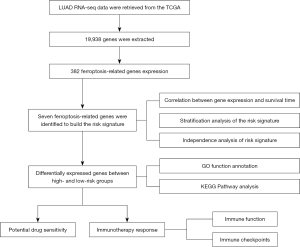
Table 1
Characteristics | Total, n (%) | Group, n (%) | P value | |
---|---|---|---|---|
Validation cohort | Experimental cohort | |||
Age (years) | 0.68 | |||
≤65 | 232 (47.06) | 113 (45.93) | 119 (48.18) | |
>65 | 251 (50.91) | 128 (52.03) | 123 (49.8) | |
Unknown | 10 (2.03) | 5 (2.03) | 5 (2.02) | |
Gender | 0.75 | |||
Female | 264 (53.55) | 134 (54.47) | 130 (52.63) | |
Male | 229 (46.45) | 112 (45.53) | 117 (47.37) | |
Clinical stage | 0.65 | |||
I | 265 (53.75) | 127 (51.63) | 138 (55.87) | |
II | 116 (23.53) | 60 (24.39) | 56 (22.67) | |
III | 79 (16.02) | 44 (17.89) | 35 (14.17) | |
IV | 25 (5.07) | 12 (4.88) | 13 (5.26) | |
Unknown | 8 (1.62) | 3 (1.22) | 5 (2.02) | |
TNM stage | ||||
T | 0.17 | |||
T1 | 164 (33.27) | 82 (33.33) | 82 (33.2) | |
T2 | 265 (53.75) | 125 (50.81) | 140 (56.68) | |
T3 | 43 (8.72) | 24 (9.76) | 19 (7.69) | |
T4 | 18 (3.65) | 13 (5.28) | 5 (2.02) | |
Unknown | 3 (0.61) | 2 (0.81) | 1 (0.40) | |
N | 0.92 | |||
N0 | 319 (64.71) | 156 (63.41) | 163 (65.99) | |
N1 | 93 (18.86) | 48 (19.51) | 45 (18.22) | |
N2 | 68 (13.79) | 36 (14.63) | 32 (12.96) | |
N3 | 2 (0.41) | 1 (0.41) | 1 (0.40) | |
Unknown | 11 (2.23) | 5 (2.03) | 6 (2.43) | |
M | 1.00 | |||
M0 | 327 (66.33) | 161 (65.45) | 166 (67.21) | |
M1 | 24 (4.87) | 12 (4.88) | 12 (4.86) | |
Unknown | 142 (28.80) | 73 (29.67) | 69 (27.94) |
LUAD, lung adenocarcinoma; T, tumor; N, node; M, metastasis.
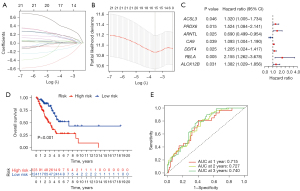
Based on the signature formula, we calculated risk scores for each sample in experimental and validation cohorts. Using the median risk score, samples were stratified into the high- or low-risk group. In the experimental cohort, overall survival (OS) was notably lower in the high-risk group than low-risk group, and the median OS was 1.652 vs. 2.167 years (P<0.001) (Figure 2D). The ROC curve for the prediction of 1-, 2-, and 3-year OS in the experimental cohort had the area under the curve (AUC) of 0.715, 0.727, and 0.740, respectively (Figure 2E).
Validation of the signature
Then the ferroptosis-related prognostic signature of LUAD was further tested for stability and reproducibility, and the levels of the seven genes in the validation cohort and the entire cohort were obtained. As confirmed in the experimental cohort, the KM curve demonstrated that the median OS of LUAD patients in the high-risk group was notably worse when compared with the low-risk group in the validation cohort (1.784 vs. 1.847 years, P=0.015) and the entire cohort (1.671 vs. 3.186 years, P<0.001) (Figure S2A,S2B). The AUC value of the ROC curve for the prediction of 1-, 2-, and 3-year OS in LUAD were 0.705, 0.703, and 0.648 in the validation cohort and 0.709, 0.712, and 0.694 in the entire cohort, respectively (Figure S2C,S2D). Furthermore, the KM curve demonstrated that the OS of LUAD patients in the high-risk group was significantly shorter than the low-risk group in different TNM stages among patients with stage I–II (P<0.001) or stage III–IV (P=0.038) disease (Figure S2E,S2F).
Development of a nomogram to predict OS
With the purpose of facilitating the clinical application of our signature, we developed a nomogram for OS prediction in LUAD patients. Univariate Cox regression showed that stage and risk score were prognostic indicators in LUAD (Figure 3A), while after adjustment for other prognostic factors (age, gender, and stage), the risk score was a significant independent prognostic factor (Figure 3B). A nomogram was then constructed using a quantitative method with the risk score and traditional clinical variables (Figure 3C). The calibration plot for 1-, 2-, and 3-year survival probability suggested optimal consistency between the prediction and observation lines, indicating the nomogram high accuracy (Figure 3D). Moreover, the AUC value of the multivariate ROC curve of the risk score and clinical variables indicated that our predictive signature (AUC =0.740) showed superiority to that of clinical predictors, including age (AUC =0.550), sex (AUC =0.526), and stage (AUC =0.683) (Figure 3E).
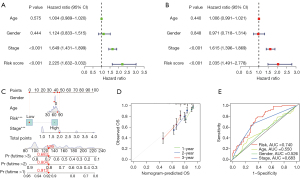
Enrichment analysis
To study the different biological functions and pathways in different risk score groups, GO and KEGG enrichment analyses were performed. PCA was adopted for verifying whether the prognostic signature was efficient in distinguishing patients between high- and low-risk groups, and whether the involved patients in these groups were accurately differentiated by the prognostic signature (Figure 4A-4C).
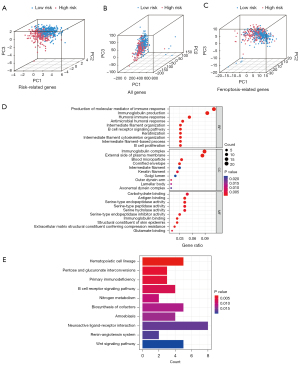
Based on the DEGs in patients with high- and low-risk scores, GO and KEGG enrichment analyses were conducted. Among the categories of GO pathways (BP: biological process; CC: cellular component; and MF: molecular function), DEGs were mostly enriched in BP (especially “production of molecular mediator of immune response”, “immunoglobulin production” and “humoral immune response”), CC (especially “immunoglobulin complex”) and MF (especially “carbohydrate binding”) categories (Figure 4D). In addition, KEGG enrichment analysis revealed 10 pathways notably related to DEGs, containing Wnt signaling pathway and hematopoietic cell lineage (Figure 4E).
Prediction of immune response and drug sensitivity
The infiltration status of 22 immune cells was assessed with CIBERSORT. Plasma cells, M0 macrophages, resting dendritic cells (DCs), and activated DCs displayed obvious differences between high- and low-risk groups (Figure 5A). The levels of 49 common immune checkpoint molecules were explored in the high- and low-risk groups (Figure 5B). PDCD1 (encoding PD-1), CD27, CTLA-4, LAIR1, and the other 25 checkpoints were differentially expressed between the two groups. Infiltration of CD8+ T cells, which could be activated by immune checkpoint inhibitors (anti-PD-1 or anti-CTLA4) and enhanced tumor cell death (20), exhibited no difference in high- or low-risk groups. Macrophage M0 cells were higher in the high-risk group. However, the low-risk group was accompanied by higher plasma cell infiltration and higher expressions of PDCD1 and CTLA4 (Figure 5A,5B).
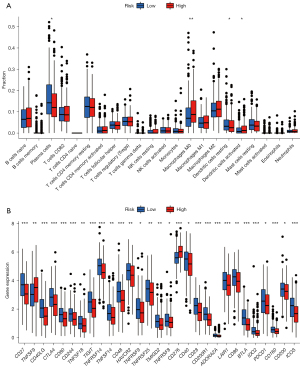
Additionally, the connection between the risk signature and drug sensitivity was explored with “pRRophetic” to identify potentially sensitive drug-targeted LUAD. In our study, talazoparib (PARP inhibitor) (Figure S3A), cisplatin (DNA damage-inducing) (Figure S3B) showed a lower IC50 value (P<0.0001) in the high-risk group, while navitoclax (the BCL-2 gene family inhibitor) (Figure S3C) showed a higher IC50 value (P<0.0001). Indicated talazoparib, cisplatin, and navitoclax may be potential agents suitable for certain subgroups of LUAD patients.
Expression of ACSL3 and PRDX6 in tumor tissues
We investigated the expression of ACSL3 and PRDX6 in LUAD tissues. ACSL3 and PRDX6 protein expressions were upregulated in LUADs compared to paired normal controls with WB and quantification analysis (Figure 6A,6B). Unlike the high expression of PRDX6 in each LUAD case, the expression level of ACSL3 showed an upward or downward trend in individual WB for each sample pair. Then the IHC experiment was used to detect the protein expression of ACSL3 in LUAD tumor tissues and confirmed its differential expression in different cases XVI, XVII, and XVIII (Figure 6C).
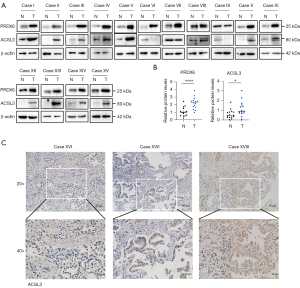
Silencing PRDX6 or ACSL3 induced ferroptosis in lung cancer cells
The high expression of ACSL3 and PRDX6 associated with worse outcomes of LUAD (Figure S1A,S1B) may partially depend on its ferroptosis-related features. To identify whether they participated in ferroptosis resistance in lung cancer cells, PRDX6 or ACSL3 were silenced with siRNA in H1299 and A549 cell lines (Figure 7A-7D). ACSL3- or PRDX6-silenced cells had increased sensitivity to RSL3-induced cell death than control cells and were completely rescued by the ferroptosis-specific inhibitor Fer-1 (Figure 7E-7H) (21,22). Additionally, BODIPY-C11 581/591 staining revealed that upon RSL3 treatment, the level of endogenous lipid peroxidation in H1299 cells was elevated (compared to that in DMSO-treated cells), and the elevation was significantly stronger in cells with ACSL3 or PRDX6 knockdown than in control cells. Furthermore, this effect could be reversed by lip-1(Figure 7I-7L). These observations suggested that ACSL3 or PRDX6 mediate ferroptosis resistance in lung cancer cells.
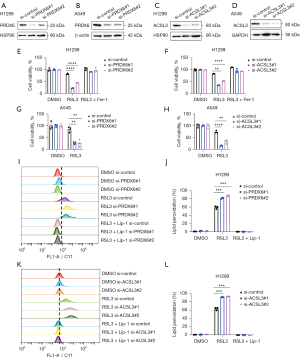
Discussion
Ferroptosis refers to an iron-dependent phospholipid peroxidation-induced type of cell death (23). Iron overload or excessive PUFA-PLs may induce ferroptosis in cells (24). With metabolic reprogramming to support rapid proliferation in tumor cells, enrichment of PUFA-PLs, an overload of iron, and imbalanced ferroptosis defense systems will provide an opportunity to target ferroptosis strategies (25). It has been documented that ferroptosis makes an impact on chemotherapy, radiotherapy, targeted therapy, and immunotherapy-mediated effects in LUAD (8). Intriguingly, ferroptosis is also correlated with cancer therapy resistance (26,27), and inducing it is a novel approach to reverse drug resistance (28). Thus, ferroptosis plays a complex role in the pathogenesis of cancer and in anticancer treatment, and further investigation is needed.
In the present study, we investigate a prognostic signature, including seven FRGs, which reveals that the high-risk group have a poor prognosis. Meanwhile, the immune response and drug sensitivity are also predicted by the prognostic signature. Upon interaction with the tumor microenvironment, macrophages are susceptible to transformation into tumor-associated macrophages (TAMs) (29). TAMs accelerate immunosuppressive environment development (30) and are associated with each stage of cancer progression and distant metastasis (31). The abundance of TAMs is considered to correlate with poor prognoses (31), which is consistent with the ratio of macrophages being higher in the high-risk individuals in our data. Meanwhile, the low-risk group is accompanied by higher plasma cell infiltration, which may be associated with a better response to immune checkpoint inhibitors (ICIs) and better outcomes (32). Additionally, a higher expression of PDCD1 and CTLA4 in the low-risk group indicated that the response to anti-PD-1 and anti-CTLA4 immunotherapy might be better in the low-risk group. Drug sensitivity is predicted by R “pRRophetic” package. Interestingly, talazoparib and cisplatin which are regarded as ferroptosis inducers (8,33) has lower IC50 values in the high-risk group, while navitoclax which inhibits BCL-2 gene family and induces apoptosis have higher IC50 values in the high-risk group. This is to say LUAD patients in the high-risk group would respond more effectively to ferroptosis inducers due to their higher expression of ferroptosis resistance genes, while those in the low-risk group would respond more effectively to apoptosis inducers. However, in vitro and in vivo experiments are needed to confirm that the different risk scores influence the response to these drugs.
Among the seven FRGs in the signature, ARNTL was previously considered a ferroptosis driver, and PRDX6, CA9, DDIT4, RELA, and ACSL3 were considered ferroptosis inhibitors. ALOX12B was one of the arachidonate lipoxygenase (ALOX) genes in humans, and silencing ALOX genes resulted in resistance to IKE (a ferroptosis inducer) treatment-induced glutathione (GSH) depletion and ferroptosis but did not alter sensitivity to RSL3 (34). In our study, ALOX12B is related to a poor prognosis in LUAD, which may be related to ALOX12B being essential for the proliferation of epidermoid carcinoma cells, including lung cancer cells (35). The biological function of ALOX12B in regulating lung cancer development deserves further studies.
GPX4 was considered as a primary enzyme in anti-ferroptosis progression. RSL3, a GPX4 inhibitor, led to increased levels of uncontrolled lipid peroxidation and ferroptosis. However, opposing PUFA activation and incorporation into membrane PLs may prevent PUFAs from oxidized and ferroptosis following GPX4 inhibition (8). PRDX6 and ACSL3 were reported to participate in cell membrane phospholipid metabolism and ferroptosis. As the result of our study, lung cancer cells would be more sensitive to ferroptosis inducer RSL3 and improved lipid peroxidation under the knockdown of ACSL3 or PRDX6, which would be rescued by ferroptosis-specific inhibitors. PRDX6 belongs to peroxiredoxins, an important family of non-selenium peroxidases (36), and utilizes GSH as the physiological reductant to perform peroxidase activity (37). Moreover, PRDX6 has Ca2+-independent phospholipase A2 activity to hydrolyze peroxidized PUFAs and lysophospholipids (LPCs) (16) to defend against ferroptosis. PRDX6 has been identified as a regulator of ferroptosis, and silencing it enhances erastin-induced ferroptosis (38). Our results show that the expression of PRDX6 is higher at the protein level in tumor tissues of LUAD, and its higher expression is correlated with poor prognosis, which is consistent with its ferroptosis defense biological function in lung cancer cell lines. ACSL3 belongs to the ACSLs family. Among ACSLs, ACSL4 was the first to be identified to promote lipid peroxidation and ferroptosis (39). In contrast, ACSL3 mediates ferroptosis resistance by replacing PUFAs in PLs with monounsaturated fatty acids (MUFAs) (17). Therefore, LUAD selectively highly expressed ACSL3 to defend against ferroptosis, while ACSL3 had different expression levels in different LUAD patients. Post-transcriptional regulation or sampling error may explain the different protein expression levels of ACSL3 in individual tumor tissues.
Some research supports the function of the signature in ferroptosis regulation. The selective autophagic degradation of ARNTL (a circadian clock regulator), called clockophagy, stimulates ferroptosis in tumor cells (40). The level of ARNTL stabilizes the expression of HIF1A, which promotes lipid storage to reduce PUFA-PLs to protect against ROS toxicity to inhibit ferroptosis (41,42). Under hypoxic conditions in tumor cells, the expression of CA9 is upregulated, followed by an increase in catalytic Fe(II), and CA9 inhibition is accompanied by reduced protein levels of iron transporters [transferrin receptor 1 (TFRC) and ferroportin-1 (FPN-1)] and elevated the expression of iron storage proteins [ferritin light chain (FTL) and ferritin heavy chain (FTH)] (43). Downregulation of DDIT4 elevates the level of lipid peroxidation and iron concentration in A549 cell line (44). RELA, also known as p65, a subunit of nuclear factor κB, regulates the transcription of SLC7A11 to defend against ferroptosis (45).
Several limitations of our research should be mentioned. First, although we performed validation, real-world data are required to confirm the prognostic capacity of our signature. A second limitation is the bioinformatic nature of this research, and in-depth mechanistic research and prospective clinical studies are required to demonstrate the specific regulatory mechanism and the clinical application value of our prognostic signature.
Conclusions
In this study, we focused on constructing and validating a ferroptosis-related 7-gene signature in LUAD, and our results suggested that it was independently correlated with OS in both experimental and validation cohorts. Additionally, the potential roles of ACSL3 and PRDX6 in ferroptosis were investigated. Further research on ferroptosis as a functional and therapeutic target in LUAD is warranted.
Acknowledgments
The authors appreciate the great support from Dr. Akikazu Kawase (Hamamatsu University School of Medicine, Japan), Dr. Armida D’Incecco (“G. Mazzini” Hospital of Teramo, Italy), Dr. Apar Kishor Ganti (University of Nebraska Medical Center, USA) and Dr. Ahmed Hasnain Jalal (Utah Valley University, USA) in improving the quality of this paper.
Funding: None.
Footnote
Reporting Checklist: The authors have completed the TRIPOD reporting checklist. Available at https://tlcr.amegroups.com/article/view/10.21037/tlcr-23-351/rc
Data Sharing Statement: Available at https://tlcr.amegroups.com/article/view/10.21037/tlcr-23-351/dss
Peer Review File: Available at https://tlcr.amegroups.com/article/view/10.21037/tlcr-23-351/prf
Conflicts of Interest: All authors have completed the ICMJE uniform disclosure form (available at https://tlcr.amegroups.com/article/view/10.21037/tlcr-23-351/coif). The authors have no conflicts of interest to declare.
Ethical Statement: The authors are accountable for all aspects of the work in ensuring that questions related to the accuracy or integrity of any part of the work are appropriately investigated and resolved. The study was conducted in accordance with the Declaration of Helsinki (as revised in 2013). The study was approved by the Ethics Committee of Zhengzhou University People’s Hospital (No. 2021-27) and informed consent was taken from all the patients.
Open Access Statement: This is an Open Access article distributed in accordance with the Creative Commons Attribution-NonCommercial-NoDerivs 4.0 International License (CC BY-NC-ND 4.0), which permits the non-commercial replication and distribution of the article with the strict proviso that no changes or edits are made and the original work is properly cited (including links to both the formal publication through the relevant DOI and the license). See: https://creativecommons.org/licenses/by-nc-nd/4.0/.
References
- Thai AA, Solomon BJ, Sequist LV, et al. Lung cancer. Lancet 2021;398:535-54. [Crossref] [PubMed]
- Oser MG, Niederst MJ, Sequist LV, et al. Transformation from non-small-cell lung cancer to small-cell lung cancer: molecular drivers and cells of origin. Lancet Oncol 2015;16:e165-e172. [Crossref] [PubMed]
- Howlader N, Forjaz G, Mooradian MJ, et al. The Effect of Advances in Lung-Cancer Treatment on Population Mortality. N Engl J Med 2020;383:640-9. [Crossref] [PubMed]
- Chen X, Li J, Kang R, et al. Ferroptosis: machinery and regulation. Autophagy 2021;17:2054-81. [Crossref] [PubMed]
- Stockwell BR. Ferroptosis turns 10: Emerging mechanisms, physiological functions, and therapeutic applications. Cell 2022;185:2401-21. [Crossref] [PubMed]
- Fang X, Wang H, Han D, et al. Ferroptosis as a target for protection against cardiomyopathy. Proc Natl Acad Sci U S A 2019;116:2672-80. [Crossref] [PubMed]
- Wang H, An P, Xie E, et al. Characterization of ferroptosis in murine models of hemochromatosis. Hepatology 2017;66:449-65. [Crossref] [PubMed]
- Yang L, Cao LM, Zhang XJ, et al. Targeting ferroptosis as a vulnerability in pulmonary diseases. Cell Death Dis 2022;13:649. [Crossref] [PubMed]
- Do Van B, Gouel F, Jonneaux A, et al. Ferroptosis, a newly characterized form of cell death in Parkinson’s disease that is regulated by PKC. Neurobiol Dis 2016;94:169-78. [Crossref] [PubMed]
- Zhang YH, Wang DW, Xu SF, et al. α-Lipoic acid improves abnormal behavior by mitigation of oxidative stress, inflammation, ferroptosis, and tauopathy in P301S Tau transgenic mice. Redox Biol 2018;14:535-48. [Crossref] [PubMed]
- Wei S, Bi J, Yang L, et al. Serum irisin levels are decreased in patients with sepsis, and exogenous irisin suppresses ferroptosis in the liver of septic mice. Clin Transl Med 2020;10:e173. [Crossref] [PubMed]
- Jiang L, Kon N, Li T, et al. Ferroptosis as a p53-mediated activity during tumour suppression. Nature 2015;520:57-62. [Crossref] [PubMed]
- Dixon SJ, Lemberg KM, Lamprecht MR, et al. Ferroptosis: an iron-dependent form of nonapoptotic cell death. Cell 2012;149:1060-72. [Crossref] [PubMed]
- Viswanathan VS, Ryan MJ, Dhruv HD, et al. Dependency of a therapy-resistant state of cancer cells on a lipid peroxidase pathway. Nature 2017;547:453-7. [Crossref] [PubMed]
- Kim R, Hashimoto A, Markosyan N, et al. Ferroptosis of tumour neutrophils causes immune suppression in cancer. Nature 2022;612:338-46. [Crossref] [PubMed]
- Fisher AB, Vasquez-Medina JP, Dodia C, et al. Peroxiredoxin 6 phospholipid hydroperoxidase activity in the repair of peroxidized cell membranes. Redox Biol 2018;14:41-6. [Crossref] [PubMed]
- Magtanong L, Ko PJ, To M, et al. Exogenous Monounsaturated Fatty Acids Promote a Ferroptosis-Resistant Cell State. Cell Chem Biol 2019;26:420-432.e9. [Crossref] [PubMed]
- Zhou N, Bao J. FerrDb: a manually curated resource for regulators and markers of ferroptosis and ferroptosis-disease associations. Database (Oxford) 2020;2020:baaa021. [Crossref] [PubMed]
- Newman AM, Liu CL, Green MR, et al. Robust enumeration of cell subsets from tissue expression profiles. Nat Methods 2015;12:453-7. [Crossref] [PubMed]
- Wang W, Green M, Choi JE, et al. CD8(+) T cells regulate tumour ferroptosis during cancer immunotherapy. Nature 2019;569:270-4. [Crossref] [PubMed]
- Stockwell BR, Friedmann Angeli JP, Bayir H, et al. Ferroptosis: A Regulated Cell Death Nexus Linking Metabolism, Redox Biology, and Disease. Cell 2017;171:273-85. [Crossref] [PubMed]
- Jiang X, Stockwell BR, Conrad M. Ferroptosis: mechanisms, biology and role in disease. Nat Rev Mol Cell Biol 2021;22:266-82. [Crossref] [PubMed]
- Lei G, Zhuang L, Gan B. Targeting ferroptosis as a vulnerability in cancer. Nat Rev Cancer 2022;22:381-96. [Crossref] [PubMed]
- Friedmann Angeli JP, Krysko DV, Conrad M. Ferroptosis at the crossroads of cancer-acquired drug resistance and immune evasion. Nat Rev Cancer 2019;19:405-14. [Crossref] [PubMed]
- Lei G, Zhang Y, Koppula P, et al. The role of ferroptosis in ionizing radiation-induced cell death and tumor suppression. Cell Res 2020;30:146-62. [Crossref] [PubMed]
- Hoy AJ, Nagarajan SR, Butler LM. Tumour fatty acid metabolism in the context of therapy resistance and obesity. Nat Rev Cancer 2021;21:753-66. [Crossref] [PubMed]
- Chen X, Kang R, Kroemer G, et al. Broadening horizons: the role of ferroptosis in cancer. Nat Rev Clin Oncol 2021;18:280-96. [Crossref] [PubMed]
- Zhang C, Liu X, Jin S, et al. Ferroptosis in cancer therapy: a novel approach to reversing drug resistance. Mol Cancer 2022;21:47. [Crossref] [PubMed]
- Lewis CE, Pollard JW. Distinct role of macrophages in different tumor microenvironments. Cancer Res 2006;66:605-12. [Crossref] [PubMed]
- Chan JM, Quintanal-Villalonga Á, Gao VR, et al. Signatures of plasticity, metastasis, and immunosuppression in an atlas of human small cell lung cancer. Cancer Cell 2021;39:1479-1496.e18. [Crossref] [PubMed]
- Liu L, Li H, Wang J, et al. Leveraging macrophages for cancer theranostics. Adv Drug Deliv Rev 2022;183:114136. [Crossref] [PubMed]
- Patil NS, Nabet BY, Müller S, et al. Intratumoral plasma cells predict outcomes to PD-L1 blockade in non-small cell lung cancer. Cancer Cell 2022;40:289-300.e4. [Crossref] [PubMed]
- Singh R, Letai A, Sarosiek K. Regulation of apoptosis in health and disease: the balancing act of BCL-2 family proteins. Nat Rev Mol Cell Biol 2019;20:175-93. [Crossref] [PubMed]
- Yang WS, Kim KJ, Gaschler MM, et al. Peroxidation of polyunsaturated fatty acids by lipoxygenases drives ferroptosis. Proc Natl Acad Sci U S A 2016;113:E4966-75. [Crossref] [PubMed]
- Jiang T, Zhou B, Li YM, et al. ALOX12B promotes carcinogenesis in cervical cancer by regulating the PI3K/ERK1 signaling pathway. Oncol Lett 2020;20:1360-8. [Crossref] [PubMed]
- Rhee SG, Chae HZ, Kim K. Peroxiredoxins: a historical overview and speculative preview of novel mechanisms and emerging concepts in cell signaling. Free Radic Biol Med 2005;38:1543-52. [Crossref] [PubMed]
- Kumar R, Coronel L, Somalanka B, et al. Mitochondrial uncoupling reveals a novel therapeutic opportunity for p53-defective cancers. Nat Commun 2018;9:3931. [Crossref] [PubMed]
- Lu B, Chen XB, Hong YC, et al. Identification of PRDX6 as a regulator of ferroptosis. Acta Pharmacol Sin 2019;40:1334-42. [Crossref] [PubMed]
- Doll S, Proneth B, Tyurina YY, et al. ACSL4 dictates ferroptosis sensitivity by shaping cellular lipid composition. Nat Chem Biol 2017;13:91-8. [Crossref] [PubMed]
- Yang M, Chen P, Liu J, et al. Clockophagy is a novel selective autophagy process favoring ferroptosis. Sci Adv 2019;5:eaaw2238. [Crossref] [PubMed]
- Bensaad K, Favaro E, Lewis CA, et al. Fatty acid uptake and lipid storage induced by HIF-1α contribute to cell growth and survival after hypoxia-reoxygenation. Cell Rep 2014;9:349-65. [Crossref] [PubMed]
- Dierge E, Debock E, Guilbaud C, et al. Peroxidation of n-3 and n-6 polyunsaturated fatty acids in the acidic tumor environment leads to ferroptosis-mediated anticancer effects. Cell Metab 2021;33:1701-1715.e5. [Crossref] [PubMed]
- Li Z, Jiang L, Chew SH, et al. Carbonic anhydrase 9 confers resistance to ferroptosis/apoptosis in malignant mesothelioma under hypoxia. Redox Biol 2019;26:101297. [Crossref] [PubMed]
- Tian Q, Zhou Y, Zhu L, et al. Development and Validation of a Ferroptosis-Related Gene Signature for Overall Survival Prediction in Lung Adenocarcinoma. Front Cell Dev Biol 2021;9:684259. [Crossref] [PubMed]
- Liu Y, Ouyang L, Mao C, et al. PCDHB14 promotes ferroptosis and is a novel tumor suppressor in hepatocellular carcinoma. Oncogene 2022;41:3570-83. [Crossref] [PubMed]
(English Language Editor: B. Draper)