Impact of time-to-treatment on survival for early-stage non-small cell lung cancer in The Netherlands—a nationwide observational cohort study
Highlight box
Key findings
• Clinical stage II patients benefit from fast initiation of surgical treatment, mainly because of those patients who clinically appear to be stage II but are pathologically still stage I. Expected is that these patients are more vulnerable to stage shift before treatment starts due to presence of lymph node- or distant micrometastasis.
What is known and what is new?
• Previous research reported varied outcomes on the impact of time-to-treatment on survival for early-stage non-small cell lung cancer. Contrary to others, this study stratified analyses for stage and type of therapy and, defined time-to-treatment as the time between first outpatient visit to start of treatment.
What is the implication, and what should change now?
• Efforts should be made to optimize diagnostic workup and decision-making strategy. Added, further study is needed on characterizing these patients and the presence and significance of lymph node- or distant micrometastasis in guiding time-to-treatment and especially treatment strategy.
Introduction
Lung cancer is the second most commonly diagnosed cancer and the leading cause of cancer mortality with an estimated 1.8 million deaths worldwide (18%) in 2020 (1). Especially non-small cell lung cancer (NSCLC), which represents approximately 85% of all lung cancers, is a considerable public health problem (2). In The Netherlands, 28% of the NSCLC patients were diagnosed with clinical stage I and II between 2014–2019 (3). For early-stage NSCLC, surgery is still the golden standard for treatment (4,5). Although up to 70% of these patients survive 5 years or longer (6,7), there is a subset of patients who are inoperable due to comorbidities, or who refuse surgery. Curative radiotherapy is established for these patients as an alternative curative treatment option (5,6,8).
Optimal management of early-stage lung cancer is a complex process that requires multidisciplinary collaboration from various medical specialists. Longer waiting times between diagnostics and start of treatment is unwanted as this causes significant emotional distress, including anxiety and depression, that is related to poorer health-related quality of life for patients (9,10). To monitor and improve timeliness, several countries developed clinical practice guidelines setting goals for maximum time intervals related to diagnosis and treatment of (lung) cancer (8,11,12).
Prolonged time-to-treatment has been suspected to be associated with lower survival and poorer clinical outcomes. Previous research reported varied outcomes on the impact of time-to-treatment on survival for early-stage NSCLC (13). Some studies showed that longer waiting times, e.g., time-to-surgery, decreased survival (14-19), while subset analysis presented that a shorter time interval was related to a higher mortality (17). Varying definitions of time-to-treatment might (partly) explain these different findings.
The incidence of early-stage NSCLC diagnosis is likely to rise with more and more pulmonary nodules detected on an increasing number of chest computed tomography (CT) scans (20,21). Increasing lung screening efforts will further accelerate this effect. Understanding time-to-treatment and its impact on survival is therefore crucial to allocate clinical resources efficiently (22-24). The objective of this study was to: (I) examine the association between extended time-to-treatment and overall survival (OS) for stage and type of therapy and (II) identify factors associated with extended time-to-treatment. We tested the hypothesis that extended time-to-treatment would negatively impact survival of early-stage NSCLC patients. We present this article in accordance with the STROBE reporting checklist (available at https://tlcr.amegroups.com/article/view/10.21037/tlcr-23-256/rc).
Methods
This study applied an observational retrospective cohort design. Information regarding early-stage lung cancer patients was retrieved from the population-based Netherlands Cancer Registry (NCR) after approval by the privacy review board of the Dutch Cancer Registry (approval number: K20.051) and analyzed for the period 2014–2019. All data were collected before the COVID-19 pandemic. This study was conducted in accordance with the Declaration of Helsinki (as revised in 2013). In accordance with the regulations of the Central Committee on Research Involving Human Subjects, this type of study does not require approval from an ethics committee in The Netherlands. Writtent informed consent was also waived due the retrospective nature of the study. The NCR collects data on all cancer patients diagnosed in The Netherlands, based on notification of newly diagnosed malignancies by the national automated pathological archive and of hospital discharge diagnoses. Information on demographics, diagnosis, staging and treatment is extracted routinely from the medical records by specially trained NCR personnel. A computerized link with the national civil registry is used to update information on survival status and date of death annually. Performance status was registered since 2015 and, information on comorbidities, lung function tests and smoking status was not available.
Patient selection
From 2014 to 2019, the NCR recorded 23,428 patients diagnosed with clinical stage I or II lung cancer from 109 hospitals (general, teaching and academic hospitals) in The Netherlands. Stages were stratified using baseline clinical staging based upon radiological imaging only. Cases were considered eligible when patient age was more than 18 years and treatment was surgery or radiotherapy. Time-to-treatment was defined as the number of days between the first recorded outpatient visit with a pulmonologist for suspected lung cancer and the recorded start of treatment (8). From the eligible cases, we excluded patients in whom the registered time-to-treatment could not be calculated because of missing data or considered unreliable, namely <0 or >180 days. Negative time-to-treatment intervals were, for instance, observed in patients who had multiple hospital appointments for different treatment modalities or second opinion, and in whom the first outpatient visit was only recorded after treatment at another hospital. A maximum cutoff value of 180 days was used as longer waiting times may have been the result of unusual, less well-controlled circumstances. We also excluded patients who underwent mediastinoscopy because they were clinically suspected for a N2-status and pathological stage III, inherent to a different prognosis (25). Also, other timeliness are recommended by the Dutch guidelines when receiving a mediastinoscopy compared to a normal diagnostic work-up (<49 vs. <35 days). Further, we excluded those patients that entered the clinical pathway differently than via an outpatient visit, e.g., emergency department. Focusing on patients that followed a normal diagnostic workup in secondary care according to the guidelines, enabled us to reduce confounding factors. Patients who enter the clinical pathway differently have a wide variety of underlying factors that may influence time-to-treatment and survival. After exclusion, 9,536 patients were available for analyses (Figure 1). All patients were followed from index (first recorded outpatient visit) to death or February 2020.
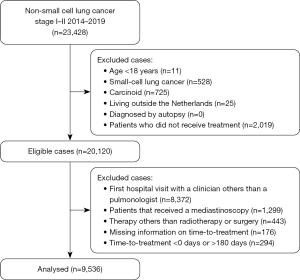
Study variables
Time-to-treatment
We categorized time-to-treatment based on data-driven quartiles (1st: 0–33 days, 2nd: 34–47 days, 3rd: 48–64 days, 4th: 65–180 days). The 1st quartile (0–33 days) was used as a reference, all other quartiles were interpreted as extended time-to-treatment. Data-driven quartiles enabled equal distribution of patients across groups and optimal statistical power. The cut-off for the reference group, 33 days, felt clinically reasonable because it is similar to the 35 days that is used in the Dutch national NSCLC guidelines as the time by which treatment of NSCLC should have started (8).
Primary outcomes
Main outcomes were OS in relation to time-to-treatment and factors associated with longer waiting times. OS was defined as the time in days from start of treatment until day of death or 1 February 2020.
Covariates
We selected those variables from the dataset of the cancer registry that could affect timing of treatment and patient survival: age at diagnosis, gender, socioeconomic status, history of other cancer, year of diagnosis, clinical stage, performance status, histology, staging procedures [endoscopic ultrasound (EUS), endobronchial ultrasound (EBUS), transthoracic biopsy, surgical exploration] and treatment type. Age was categorized as 18–60, 61–70, 71–80 and 80+ years. History of other cancer was defined as previous cancer diagnosis, other than NSCLC, in the five years before NSCLC diagnosis. Socioeconomic status was provided by the Netherlands Institute for Social Research at an aggregated level for each postal code and determined from the average income, proportions with low income, low educational level, and unemployment, categorized as low [1–4], mid [5–7] and high [8–10]. Year of diagnosis was grouped in 2014, 2015–2016 and 2017+. We based categorization of year of diagnosis on therapeutic evolvements (i.e., introduction of immunotherapy in 2015) and introduction of the eight version of the TNM classification in 2017. Stage information was recorded according to the 7th (until 2016) and 8th (from 2017) edition of the TNM Classification of Malignant Tumors from the International Union Against Cancer (26). Performance scores were registered prior to starting treatment and indicated in the dataset by the Eastern Cooperative Oncology Group Performance Status (ECOG PS) and the Karnofsky scale [10–100]. Karnofsky scores were converted to ECOG PS and grouped in 0, 1, 2, 3–4 and unknown (27). Histology was coded according to the third edition of the International Classification of Disease for Oncology (ICD-O-3) and categorized in four subgroups: adenocarcinoma, squamous cell carcinoma, large cell carcinoma and clinical diagnosis wherever pathological confirmation was not possible. Type of treatment was categorized as radiotherapy and surgery. Surgical procedures included (bi)lobectomy, pneumonectomy, segmental resection, and wedge resection. Radiotherapy included stereotactic body radiation therapy (SBRT) and conventional radiotherapy of the primary tumor.
Statistical analysis
Statistical analyses were performed using SAS, version 9.4 (SAS Institute, Cary, NC, USA).
The proportions of patients for whom treatment was initiated within 33 days and beyond 33 days were tabulated (Table 1). The Pearson’s chi-squared test and Cochran-Armitage trend test were used to test whether distributions of the dependent variable differed across the (ordered) categories of each parameter. All statistical tests were two-sided and the significance level for the analysis was set at a P value <0.05.
Table 1
Characteristics | Patient cohort stage I-II (n=9,536) | |||
---|---|---|---|---|
Total* | Time-to-treatment: 0–33 days | Time-to-treatment: >33 days | P value** | |
Age (years) | <0.0001 | |||
0–60 | 1,646 | 478 (29%) | 1,168 (71%) | |
61–70 | 3,349 | 883 (26%) | 2,466 (74%) | |
71–80 | 3,509 | 712 (20%) | 2,797 (80%) | |
>80 | 1,032 | 197 (19%) | 835 (81%) | |
Gender | 0.5897 | |||
Male | 4,994 | 3,794 (76%) | 3,794 (76%) | |
Female | 4,542 | 3,472 (76%) | 3,472 (76%) | |
Socioeconomic status | 0.0090 | |||
1–4 (low) | 5,335 | 1,223 (23%) | 4,112 (77%) | |
5–7 (mid) | 2,255 | 544 (24%) | 1,711 (76%) | |
8–10 (high) | 1,946 | 503 (26%) | 1,443 (74%) | |
History of other cancer | 0.3649 | |||
Yes | 1,635 | 375 (23%) | 1,260 (77%) | |
No | 7,901 | 1,895 (24%) | 6,006 (76%) | |
Year of diagnosis | <0.0001 | |||
2014 | 1,113 | 308 (28%) | 805 (72%) | |
2015 | 1,612 | 420 (26%) | 1,192 (74%) | |
2016 | 1,741 | 479 (28%) | 1,262 (72%) | |
2017 | 1,719 | 414 (24%) | 1,305 76%) | |
2018 | 1,760 | 329 (19%) | 1,431 (81%) | |
2019 | 1,591 | 320 (20%) | 1,271 (80%) | |
Clinical stage | 0.2532 | |||
I | 7,455 | 1,755 (24%) | 5,700 (76%) | |
II | 2,081 | 515 (25%) | 1,566 (75%) | |
Performance status | 0.8344 | |||
0 | 2,667 | 705 (26%) | 1,962 (74%) | |
1 | 2,268 | 486 (21%) | 1,782 (79%) | |
2 | 760 | 133 (18%) | 627 (82%) | |
3 | 162 | 35 (22%) | 127 (78%) | |
4 | 13 | 0 (0%) | 13 (100%) | |
Unknown | 3,666 | 911 (25%) | 2,755 (75%) | |
Histology | 0.0025 | |||
Adenocarcinoma | 4,166 | 1,071 (26%) | 3,095 (74%) | |
Squamous cell | 2,214 | 498 (22%) | 1,716 (78%) | |
Large cell | 486 | 111 (23%) | 375 (77%) | |
Other1 | 2,670 | 590 (22%) | 2,080 (78%) | |
EUS | 0.0004 | |||
Yes | 312 | 48 (15%) | 264 (85%) | |
No | 9,224 | 2,222 (24%) | 7,002 (76%) | |
EBUS | <0.0001 | |||
Yes | 1,601 | 276 (17%) | 1,325 (83%) | |
No | 7,935 | 1,994 (25%) | 5,941 (75%) | |
Surgical exploration | 0.2080 | |||
Yes | 12 | 1 (8%) | 11 (92%) | |
No | 9,524 | 2,269 (24%) | 7,255 (76%) | |
Transthoracic biopsy | <0.0001 | |||
Yes | 1,478 | 176 (12%) | 1,302 (88%) | |
No | 3,592 | 887 (25%) | 2,705 (75%) | |
Unknown | 4,466 | 1,207 (27%) | 3,259 (73%) | |
Therapy | <0.0001 | |||
Radiotherapy | 4,763 | 797 (17%) | 3,966 (83%) | |
Surgery | 4,773 | 1,473 (31%) | 3,300 (69%) |
*, Percentages for time-to-treatment ≤33 and >33 days were horizontally calculated for each subcategory. **, P values from the Pearson’s chi-squared test and Cochran-Armitage trend test. The Cochran-Armitage trend test was applied on age, social economic status, year of diagnosis, performance status. 1, Other: rare histological subtypes, clinical diagnosis. NSCLC, non-small cell lung cancer; EUS, endoscopic ultrasound; EBUS, endobronchial ultrasound.
Time-to-treatment and survival
Cox proportional hazards (PH) models were used to evaluate the relation between longer time-to-treatment intervals and OS per stage and type of therapy. Time-to-treatment was tested for quartiles and, later for the first quartile versus the others. Prior to analysis, the PH assumption was examined to determine whether the hazard ratio (HR) for any parameter was constant over time. Non-proportionality was detected graphically and by proportionality tests. Other covariates were selected by use of backward and forward stepwise regression, parameters with P values >0.05 were eliminated from the model. Covariates adjusted in the analyses included age at diagnosis, gender, socioeconomic status, history of other cancer, clinical stage, and histology. Separate models were built for type of therapy to account for effect modification. Especially as treatment outcomes might not be equivalent for stage II compared to stage I. Analyses were primarily based on clinical staging. Additionally, we evaluated the impact of pathological up- and downstaging on the survival of surgical patients. The association was determined by adjusted hazard ratios (aHRs), 95% confidence intervals (CIs) and P values. An aHR >1.0 with a P value <0.05 represented an increased hazard of death.
Factors associated with extended time-to-treatment
Factors associated with a time-to-treatment interval beyond 33 days were identified using multivariable analyses by multilevel logistic regression per stage of early disease. To account for clustering of patients, hospital of diagnosis was included as a random-effect parameter (28). The statistical significance of covariates was evaluated in backward and forward stepwise regression, where parameters with a P value >0.05 were eliminated from the model. Adjusted covariates included age at diagnosis, histology, diagnostic staging procedures (EUS, EBUS, transthoracic biopsy) and type of therapy. Analyses were also stratified for type of hospital (general, teaching, academic) to explore whether effects differ between these types of hospitals. The goodness of fit was assessed with use of the Bayesian Information Criterion (BIC) and area under the curve (AUC). The intraclass correlation coefficient (ICC) quantified the relatedness of clustered data by comparing the variance within clusters with the variance between clusters. The ICC ranges from 0 to 1 and a smaller ICC indicates a lower cluster effect (29).
Results
Patient population
In total, 9,536 early-stage patients were included in the analyses (Figure 1). Seventy-eight percent was diagnosed with clinical stage I and 22% with clinical stage II. Most of the characteristics were comparable for stage I and II (Table S1); 52% were male and 48% female (Table 1). The median age was 70 years and 83% of the population was >60 years. Adenocarcinoma was more common than squamous cell carcinoma and large cell carcinoma (44% vs. 23% vs. 5% respectively). However, squamous cell carcinoma was more common in stage II than in stage I, 36% vs. 20% respectively. Fifty-four percent of stage I patients were treated with radiotherapy versus 36% in stage II. Twenty-one percent of the surgical patients who were clinically diagnosed as stage I had a higher pathological stage after surgery. Twenty percent of the surgical patients clinically staged as II had a lower and 23% a higher pathological stage after surgery. Other demographic and clinical characteristics are listed in Table 1.
Time-to-treatment
The median time-to-treatment was 47 days for stage I [interquartile range (IQR): 34–65] and 46 days for stage II (IQR: 33–62). Two percent of the study population received treatment within seven days. Time-to-treatment with radiotherapy had a median of 51 days for both stage I and II (IQR: 37–72 vs. 38–71, respectively), and 83% of patients experienced a delay (>33 days). Surgical treatment started after a median interval of 42 days for both stage I and II (IQR: 30–57 vs. 32–57), whereby 68% of stage I and 71% of stage II patients experienced a delay (>33 days). Figures 2,3 present the distribution of time-to-radiotherapy and time-to-surgery per stage of disease.
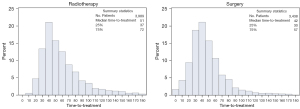
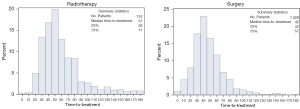
The association between time-to-treatment and survival
The median follow-up for survival was 62 months (65 months for stage I and 45 months for stage II). The 1-, 3- and 5-year survival rate was 90%, 69% and 53% for clinical stage I and 81%, 53% and 39% for clinical stage II, respectively. Patients who received surgery had better survival rates (log-rank P<0.0001) (Figure 4). In clinical stage I, subset analyses for radiotherapy and surgery showed no significant association between time-to-treatment and OS. In clinical stage II, subset analyses showed no association between prolonged time-to-radiotherapy and OS. Longer time-to-surgery in clinical stage II cancers, however, was significantly associated with an increased hazard of death when compared to the first quartile (<33 days) (aHR 34–47 days: 1.32, 95% CI: 1.02–1.70; aHR 48–64 days: 1.39, 95% CI: 1.05–1.85; aHR 64–180 days: 1.41, 95% CI: 1.04–1.92). Table 2 shows the aHRs of extended time-to-treatment per clinical stage and type of therapy. Table S2 gives an overview of covariates selected for multivariable analysis.
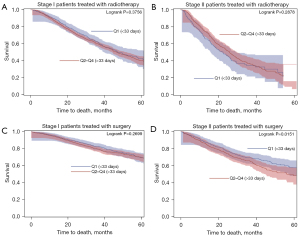
Table 2
Time-to-treatment quartiles | Stage I | Stage II | |||||||||
---|---|---|---|---|---|---|---|---|---|---|---|
Radiotherapy (N=3,989) | Surgery (N=3,438) | Radiotherapy (N=747) | Surgery (N=1,325) | ||||||||
aHR1 (95% CI) | P value | aHR2 (95% CI) | P value | aHR3 (95% CI) | P value | aHR4 (95% CI) | P value | ||||
Q1 (0–33 d) | Ref | – | Ref | – | Ref | – | Ref | – | |||
Q2 (34–47 d) | 1.02 (0.87–1.20) | 0.797 | 1.08 (0.88–1.33) | 0.441 | 1.03 (0.76–1.40) | 0.829 | 1.32 (1.02–1.70) | 0.034 | |||
Q3 (48–64 d) | 1.07 (0.90–1.26) | 0.453 | 0.99 (0.79–1.24) | 0.943 | 0.87 (0.65–1.18) | 0.382 | 1.39 (1.05–1.85) | 0.022 | |||
Q4 (65–180 d) | 1.12 (0.96–1.31) | 0.153 | 1.03 (0.81–1.31) | 0.807 | 0.87 (0.65–1.17) | 0.360 | 1.41 (1.04–1.92) | 0.027 | |||
Q2–Q4 (34–180 d) | 1.07 (0.93–1.23) | 0.336 | 1.04 (0.88–1.23) | 0.655 | 0.91 (0.71–1.19) | 0.517 | 1.36 (1.09–1.70) | 0.007 |
1, time-to-treatment adjusted for clinical stage (Ia/Ib), age, gender, history of other cancer, socioeconomic status, performance status; 2, time-to-treatment adjusted for clinical stage (Ia/Ib), age, gender, history of other cancer, socioeconomic status, performance status, histology; 3, time-to-treatment adjusted for age, performance status; 4, time-to-treatment adjusted for year of diagnosis, gender, performance status. aHR, adjusted hazard ratio; CI, confidence interval; d, days.
We further studied the relation to the pathological stage in clinical stage II patients treated with surgery. We found a significant effect of extended time-to-treatment on OS for those clinical stage II patients who were down-staged to a pathological stage I after surgery (n=259, aHR 34–47 days: 2.29, 95% CI: 1.08–4.84; aHR 48–64 days: 2.46, 95% CI: 1.15–5.28; aHR 64–180 days: 2.77, 95% CI: 1.25–6.15). However, we found no significant relation between time-to-treatment and OS in clinical stage II patients who were correctly staged or up-staged to stage III or IV at pathology (n=303) (Table 3). Figure 5 shows Kaplan-Meier plots of clinical stage II/pathological stage I and clinical stage II/pathological stage II by Q1 vs. Q2–Q4 of time-to-treatment. Patients clinically staged II and post-surgery as stage I had better survival when time intervals were <33 days (log-rank P=0.0070).
Table 3
Time-to-treatment quartiles | Pathological stage I (N=259) | Pathological stage II (N=763) | Pathological stage III–IV (N=303) | |||||
---|---|---|---|---|---|---|---|---|
aHR1 (95% CI) | P value | aHR2 (95% CI) | P value | aHR3 (95% CI) | P value | |||
Q1 (0–33 d) | Ref | – | Ref | – | Ref | – | ||
Q2 (34–47 d) | 2.29 (1.08–4.84) | 0.031 | 1.22 (0.86–1.72) | 0.266 | 1.00 (0.63–1.60) | 0.986 | ||
Q3 (48–64 d) | 2.46 (1.15–5.28) | 0.021 | 1.24 (0.83–1.85) | 0.305 | 1.33 (0.81–2.18) | 0.267 | ||
Q4 (65–180 d) | 2.77 (1.25–6.15) | 0.012 | 1.32 (0.86–2.03) | 0.201 | 1.20 (0.69–2.08) | 0.515 | ||
Q2–Q4 (34–180 d) | 2.47 (1.30-4.69) | 0.006 | 1.25 (0.92-1.69) | 0.162 | 1.14 (0.76-1.70) | 0.527 |
1, time-to-treatment adjusted for gender; 2, time-to-treatment adjusted for year of incidence, social economic status, age; 3, time-to-treatment adjusted for performance status and histology. aHR, adjusted hazard ratio; CI, confidence interval; d, days.
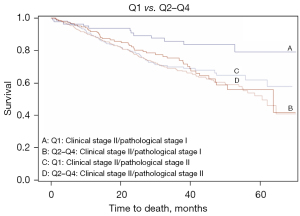
Factors associated with extended time-to-treatment
Diagnostic procedures such as EUS, EBUS and transthoracic biopsy significantly increased the odds for longer time-to-treatment (EUS: OR stage I: 1.80, 95% CI: 1.17–2.76; OR stage II: 2.13, 95% CI: 1.23–3.71) (EBUS: OR stage I: 1.90, 95% CI: 155–2.32; OR stage II: 2.20, 95% CI: 1.68–2.88) (transthoracic biopsy: OR stage I: 2.50, 95% CI: 2.00–3.12; OR stage II: 3.03, 95% CI: 2.06–4.47). Also, radiotherapeutic strategies showed significantly increased odds when adjusted for other covariates (SBRT OR stage I: 3.70, 95% CI: 3.05–4.48; OR stage II: 2.45, 95% CI: 1.73–3.48) (Conventional radiotherapy OR stage I: 3.41, 95% CI: 2.58–4.49; OR stage II: 2.08, 95% CI: 1.55–2.78). Factors associated with extended time-to-treatment are detailed in Tables 4,5. The AUC of the fitted model was 0.72 for stage I and 0.72 for stage II. The clustering effect accounted for approximately 6–7% of the total variation in the probability of extended time-to-treatment. This leaves 93–94% of the variability to be accounted for measured or unmeasured factors that are not related to the hospital, such as patient and tumor characteristics.
Table 4
Factor | OR | 95% CI | P value |
---|---|---|---|
Age at diagnosis (years) | |||
61–70 vs. 18–60 | 1.07 | 0.91–1.25 | 0.4267 |
71–80 vs. 18–60 | 1.32* | 1.11–1.55 | 1.11–1.55 |
80+ vs. 18–60 | 1.23 | 0.96–1.56 | 0.96–1.56 |
Histology | |||
Clinical diagnosis1vs. pathological diagnosis2 | 0.53* | 0.44–0.65 | <0.0001 |
EUS | |||
Yes vs. no | 1.80* | 1.17–2.76 | 0.0080 |
EBUS | |||
Yes vs. no | 1.90* | 1.55–2.32 | <0.0001 |
Transthoracic biopsy | |||
Yes vs. no | 2.50* | 2.00–3.12 | <0.0001 |
Unknown vs. no | 0.89 | 0.78–1.01 | 0.0769 |
Type of therapy | |||
Pneumonectomy vs. lobectomy | 1.71 | 0.85–3.42 | 0.1313 |
Segment resection vs. lobectomy | 0.78 | 0.53–1.13 | 0.1906 |
Wedge resection vs. lobectomy | 0.78 | 0.60–1.03 | 0.0762 |
SBRT vs. lobectomy | 3.70* | 3.05–4.48 | <0.0001 |
Conventional radiotherapy vs. lobectomy | 3.41* | 2.58–4.49 | <0.0001 |
Other3vs. lobectomy | 0.41* | 0.18–0.94 | 0.0341 |
*, odds ratios that reached the level of significance. The hospitals accounted for approximately 7% of the variability in time-to-treatment for stage I [ICC: 𝜏00 =0.2260; z(107) =3.41, P=0.003] (median ORlowest-highest: 0.4–2.6). 1, diagnosis based on imaging and not pathologically confirmed. 2, histological subtypes adenocarcinoma, squamous cell carcinoma, large cell carcinoma, other. NSCLC, non-small cell lung cancer; EUS, endoscopic ultrasound; EBUS, endobronchial ultrasound; SBRT, stereotactic body radiotherapy; ICC, intraclass correlation coefficient.
Table 5
Factor | OR | 95% CI | P value |
---|---|---|---|
EUS | |||
Yes vs. no | 2.13* | 1.23–3.71 | 0.0082 |
EBUS | |||
Yes vs. no | 2.20* | 1.68–2.88 | <0.0001 |
Transthoracic biopsy | |||
Yes vs. no | 3.03* | 2.06–4.47 | <0.0001 |
Unknown vs. no | 0.96 | 0.76–1.22 | 0.7448 |
Type of therapy | |||
Pneumonectomy vs. lobectomy | 0.76 | 0.45–1.26 | 0.2794 |
Segment resection vs. lobectomy | 0.81 | 0.22–2.89 | 0.7395 |
Wedge resection vs. lobectomy | 1.85 | 0.71–4.79 | 0.2059 |
SBRT vs. lobectomy | 2.45* | 1.73–3.48 | <0.0001 |
Conventional radiotherapy vs. lobectomy | 2.08* | 1.55–2.78 | <0.0001 |
Other3vs. lobectomy | 0.56 | 0.12–2.66 | 0.4635 |
*, odds ratios that reached the level of significance. The hospitals accounted for approximately 6% of the variability in time-to-treatment for stage II [ICC: 𝜏00 =0.2364; z(104) =4.58, P<0.0001] (median ORlowest-highest: 0.4–1.8) (29). 3, other: electrocauterization, laser coagulation, local surgery, organ surgery, local tumor destruction. NSCLC, non-small cell lung cancer; EUS, endoscopic ultrasound; EBUS, endobronchial ultrasound; SBRT, stereotactic body radiotherapy; ICC, intraclass correlation coefficient.
Discussion
We studied the relation between time-to-treatment and survival in early-stage NSCLC in this retrospective cohort study based on a nation-wide registry from The Netherlands, and identified factors associated with extended time-to-treatment. We found no significant effect of an extended time-to-treatment up to 180 days after initial outpatient visit on OS in all clinical stage I patients and in those clinical stage II patients treated with radiotherapy. This finding persists after correcting for confounding factors such as patient demographics, performance status and socioeconomic status. Adjusted analyses for extended time-to-treatment identified staging procedures, older age and treatment with radiotherapy to cause diagnostic delay. The non-significant results of longer time-to-radiotherapy on survival are probably due to careful patient selection, taking patients’ fitness and comorbidities into consideration. Stratification of the analyses for type of hospital showed that effects were similar, irrespective of type of hospital.
In clinical stage II NSCLC patients treated with surgery, however, an extended time-to-treatment beyond 33 days was significantly associated with a more than 30% increased risk of death (see Table 2). Subgroup analysis showed that this increased mortality risk mainly came from clinical stage II patients who were over-staged and turned out to be a pathological stage I after surgery. Their HR for mortality increased relatively from 2.26 in the 2nd quartile to 2.77 in the 4th quartile (64–180 d time-to-treatment) compared to patients who were treated within 33 days. We didn’t find an explanation for this finding. Potentially, this group with suspected radiological stage II disease, based on enlarged or FDG positive lymph nodes, is misclassified as pathological stage I due to incomplete mediastinal lymphadenectomy or because pathological evaluation in NSCLC does not include description of isolated tumor cells. We suspect that some of these pathologic stage I cancers may actually be pathological stage II with lymph node micrometastasis not detected in the resected material because of current clinical methods or distant micrometastasis. Lymph node micrometastasis are usually found in clinical N0/N1 NSCLC and with tumors smaller than 3 cm (30), which is associated with a poor prognosis (31-36). Ideally, detection should be done rapidly to shorten the diagnostic interval and fasten treatment (37,38). This hypothesis is supported by the fact that the survival curves of patients with clinical stage II/pathological stage I who were treated within 33 days was better than that of those patients who were treated later. Their survival curves became more similar to those of clinical stage II/pathological stage II cancers. Early detection of occult disease could help to identify those patients who might benefit from neoadjuvant therapy. Results of a previous study indicate a place for limited biomarker testing (EGFR, ALK and PD-L1) in the initial workup of early-stage NSCLC (37). During the study period, however, biomarker testing in early stages was only performed in clinical trials.
No association was found between timing of radiotherapy and survival. Higher percentages of patients aged 70 years or older, or with reduced clinical performances scores were found in the subgroup that received radiotherapy (Table S1). Also presence of lymph node metastases (N1) seems to affect the association with survival since higher numbers of patients that received surgery were staged 2B (Table S1). For most of these patients, surgery is preferred over extending the radiotherapeutic field. These patients are more likely to benefit from earlier timing of treatment as metastasized disease tends to progress faster (38). We did not further assess the potential for upstaging for patients with a pathological status who underwent radiation rather than surgery.
We also found a significant decrease of OS in patients who waited longer for surgery (14,17,19,39,40). One study suggests that mortality risk differs meaningfully across patients subgroups when stratified by stage and specific histologic subtype (ie, adenocarcinoma and squamous cell carcinoma) (19). We did not repeat this multivariable analysis for due to small subgroups but further research should incorporate this stratification. The other results are not in line with previous studies that found no effect (14,18,41), or significant worse survival for shorter time intervals (17). We believe that the main reason for these conflicting results is the variability in definitions, e.g., time-to-treatment and cutoff values for extended time-to-treatment. But also, stratification for therapy and stage, the use of either clinical or pathological tumor stage, and the prognostic factors selected for multivariable analysis such as performance status and comorbidity. Previous timeframes that have been evaluated vary from dichotomous to categorical, e.g., 0–7, 8–14, 15–60, >61 days, but also <42 vs. ≥42 days, or 1–37 vs. >37 days (14,18,40). We have chosen to use the time between first outpatient visit to start of treatment as a measure of time-to-treatment because it also includes any delay due to the diagnostic process. A sensitivity analysis investigating the effect of using a different definition of time-to-treatment did not change our conclusion. Others used the time between diagnosis and treatment, leading to shorter time intervals, which can even become 0 days if the diagnosis is made during surgery. Shorter time intervals are less likely to show any significant findings as chances on tumor progression are reduced.
Moreover, most studies based cutoff values for extended time-to-treatment on guidelines. This way, patients are distributed non-equally across the time intervals and more likely to cause significant findings. Questioned can be, however, if significance is based on the effect of timing of treatment or due to differences in numbers of patients. At the same time, most studies included comorbidities but did not adjust multivariable analysis for performance status. Our dataset did not provide information of comorbidity but we corrected for performance status and age. Both are defined as important predictors for surgery and prognostic factors in previous research (18). Added, we used the type of treatment as an effect modifier in the link between time-to-treatment and survival as these parameters have a different impact on survival. This was done by stratified analysis, while others did not (14,15).
Our study has several major strengths. First, the national coverage of the database allowed for a large sample size and therefore made it possible to draw conclusions based on an entire population in an European country. Our data represents the current clinical situation by focusing on patients diagnosed and treated in 2014–2019. Since health care coverage is universal in The Netherlands, effects of inadequate coverage on outcome could be excluded. We used the first consultation with a pulmonologist as starting point of the time-to-treatment interval. This includes all delays in the diagnostic process, in decision making and in waiting times to commencement of treatment. In addition, we reduced the heterogeneity in underlying prognostic factors by excluding patients that received a mediastinoscopy and entered the medical pathway differently. We have chosen to use data-driven quartiles to equally distribute the patients across the different categories to ensure sufficient power in all categories. Using data-driven quartiles provided evidence-based cut-offs that could be of value in future recommendations. Last, with use of multilevel analyses, we accounted for many confounding factors including health status, interhospital variability and nested data. We performed extensive adjustments for case mix and confounding factors, including performance and socioeconomic status, which was rarely done in other studies.
Our study also has limitations. First, detailed information on comorbidity index, chronic lung disease, tobacco use and lung function test were not available in the NCR, limiting exploration of the potential impact. Also, not all information was available on what happened within the time-to-treatment interval, such as the types of diagnostic procedures [e.g., positron emission tomography (PET), CT, PET-CT], the dates at which they were conducted, if a patient received multiple diagnostic tests and if the patient was referred to another hospital for therapy. As well as information on time intervals before visiting a pulmonologist. We believe that the time interval from primary care to secondary care is clinically very relevant. Availability of such data would have put us in a better position to explain variations in time-to-treatment. Next, information on parameters such as type of resection and histologic grade lacked. However, we only included variables that were expected to impact time-to-treatment. By stratifying data for type of therapy and correcting for histology, we have tried to caught some potential confounding. The role of adjuvant treatment was not explored because we believe that this parameter is of less relevance in the association between timing of primary treatment and survival. Further, we reduced our sample size as we focused on a more ‘routine’ referral pathway according to the guidelines. We included patients who were referred by a general practitioner and had a first hospital visit with a pulmonologist. However, it is the nature of lung cancer that a proportion of patients will present as an emergency or through alternative referral pathway. We preferred our selection as this enabled us to reduce confounding. This because patients who enter the clinical pathway differently have a wide range of underlying factors that may influence time-to-treatment and survival. It is difficult to correct for all these underlying factors, especially as most are not registered. Also, Dutch practice patterns might differ from other countries as lung cancer patients are primarily seen by pulmonologists. As our results were retrieved in a country with a healthcare system equally accessible to all inhabitants, these can only be generalized to countries with a similar healthcare system. Furthermore, we excluded patients who received therapy others than radiotherapy and surgery as this subgroup was underpowered to do further stratification. However, the role of multi-modality neo-adjuvant and adjuvant medical treatments such as chemotherapy and immunotherapy is likely to rise with current evolvements. Therefore, it would be of great value to expand future databases with such clinical data. Besides, information on variables as performance status and transthoracic biopsy was missing for some patients as the NCR started this registration since 2015 and 2017, respectively. We decided to classify the status as unknown rather than exclude them from analysis as these subgroups consisted of relatively high numbers. Excluding these patients from the analysis however still provided similar results. Last, information on cause of death was not available, hampering the distinction between due to progressive disease or other causes.
Conclusions
Clinical stage II patients benefit from fast initiation of surgical treatment, mainly because of those patients who clinically appear to be stage II but are pathologically still stage I. Expected is that these patients are more vulnerable to stage shift before treatment starts due to presence of lymph node- or distant micrometastasis. Efforts should be made to optimize diagnostic workup and decision-making strategy. Added, further study is needed on characterizing these patients and the presence and significance of lymph node- or distant micrometastasis in guiding time-to-treatment and especially treatment strategy.
Acknowledgments
We would like to thank the registration team of the Netherlands Comprehensive Cancer Organization (IKNL) for the collection of data as well as IKNL staff for scientific advice. Special thanks to Ronald Damhuis and Matteo Cellamare, also both working at IKNL, for their advice on the statistical analyses.
Funding: None.
Footnote
Reporting Checklist: The authors have completed the STROBE reporting checklist. Available at https://tlcr.amegroups.com/article/view/10.21037/tlcr-23-256/rc
Peer Review File: Available at https://tlcr.amegroups.com/article/view/10.21037/tlcr-23-256/prf
Conflicts of Interest: All authors have completed the ICMJE uniform disclosure form (available at https://tlcr.amegroups.com/article/view/10.21037/tlcr-23-256/coif). The authors have no conflicts of interest to declare.
Ethical Statement: The authors are accountable for all aspects of the work in ensuring that questions related to the accuracy or integrity of any part of the work are appropriately investigated and resolved. This study was conducted in accordance with the Declaration of Helsinki (as revise in 2013). Information regarding early-stage lung cancer patients was retrieved from the population-based Netherlands Cancer Registry (NCR) after approval by the privacy review board of the Dutch Cancer Registry (approval number: K20.051). In accordance with the regulations of the Central Committee on Research Involving Human Subjects, this type of study does not require approval from an ethics committee in the Netherlands. Written informed consent was also waived due the retrospective nature of the study.
Open Access Statement: This is an Open Access article distributed in accordance with the Creative Commons Attribution-NonCommercial-NoDerivs 4.0 International License (CC BY-NC-ND 4.0), which permits the non-commercial replication and distribution of the article with the strict proviso that no changes or edits are made and the original work is properly cited (including links to both the formal publication through the relevant DOI and the license). See: https://creativecommons.org/licenses/by-nc-nd/4.0/.
References
- Sung H, Ferlay J, Siegel RL, et al. Global Cancer Statistics 2020: GLOBOCAN Estimates of Incidence and Mortality Worldwide for 36 Cancers in 185 Countries. CA Cancer J Clin 2021;71:209-49. [Crossref] [PubMed]
- Alberg A, Brock M, Samet J. Chapter 52: epidemiology of lung cancer. Murray & Nadel's textbook of respiratory medicine. 6. Philadelphia: Saunders Elsevier; 2016
- NCR, Dutch Lung Cancer Statistics 2019. Available online: https://nkr-cijfers.iknl.nl/viewer
- Postmus PE, Kerr KM, Oudkerk M, et al. Early and locally advanced non-small-cell lung cancer (NSCLC): ESMO Clinical Practice Guidelines for diagnosis, treatment and follow-up. Ann Oncol 2017;28:iv1-iv21. [Crossref] [PubMed]
- Hopstaken JS, de Ruiter JC, Damhuis RAM, et al. Stage I non-small cell lung cancer: Treatment modalities, Dutch daily practice and future perspectives. Cancer Treat Res Commun 2021;28:100404. [Crossref] [PubMed]
- Zierhut D, Bettscheider C, Schubert K, et al. Radiation therapy of stage I and II non-small cell lung cancer (NSCLC). Lung Cancer 2001;34:S39-43. [Crossref] [PubMed]
- Walters S, Maringe C, Coleman MP, et al. Lung cancer survival and stage at diagnosis in Australia, Canada, Denmark, Norway, Sweden and the UK: a population-based study, 2004-2007. Thorax 2013;68:551-64. [Crossref] [PubMed]
- NVALT, Niet kleincellig longcarcinoom: Landelijke richtlijn versie 2.3 [Non-small cell lung carcinoma: National guideline version 2.3. Oncoline 2015. Available online: https://www.venvn.nl/richtlijnen/alle-richtlijnen/richtlijn-niet-kleincellig-longcarcinoom/
- Paul C, Carey M, Anderson A, et al. Cancer patients' concerns regarding access to cancer care: perceived impact of waiting times along the diagnosis and treatment journey. Eur J Cancer Care (Engl) 2012;21:321-9. [Crossref] [PubMed]
- Brocken P, van der Heijden EH, Oud KT, et al. Distress in suspected lung cancer patients following rapid and standard diagnostic programs: a prospective observational study. Psychooncology 2015;24:433-41. [Crossref] [PubMed]
- Asch SM, Kerr EA, Hamilton EG, et al. Quality of Care for Oncologic Conditions and HIV: A Review of the Literature and Quality Indicators. . RAND Corporation 2000:133-171.
- NHS, Cancer waiting times UK. 2019. Available online: https://www.england.nhs.uk/statistics/statistical-work-areas/cancer-waiting-times/
- Hall H, Tocock A, Burdett S, et al. Association between time-to-treatment and outcomes in non-small cell lung cancer: a systematic review. Thorax 2022;77:762-8. [Crossref] [PubMed]
- Tsai CH, Kung PT, Kuo WY, et al. Effect of time interval from diagnosis to treatment for non-small cell lung cancer on survival: a national cohort study in Taiwan. BMJ Open 2020;10:e034351. [Crossref] [PubMed]
- Gomez DR, Liao KP, Swisher SG, et al. Time to treatment as a quality metric in lung cancer: Staging studies, time to treatment, and patient survival. Radiother Oncol 2015;115:257-63. [Crossref] [PubMed]
- Khorana AA, Tullio K, Elson P, et al. Time to initial cancer treatment in the United States and association with survival over time: An observational study. PLoS One 2019;14:e0213209. [Crossref] [PubMed]
- Anggondowati T, Ganti AK, Islam KMM. Impact of time-to-treatment on overall survival of non-small cell lung cancer patients-an analysis of the national cancer database. Transl Lung Cancer Res 2020;9:1202-11. [Crossref] [PubMed]
- Samson P, Patel A, Garrett T, et al. Effects of Delayed Surgical Resection on Short-Term and Long-Term Outcomes in Clinical Stage I Non-Small Cell Lung Cancer. Ann Thorac Surg 2015;99:1906-12; discussion 1913. [Crossref] [PubMed]
- Yang CJ, Wang H, Kumar A, et al. Impact of Timing of Lobectomy on Survival for Clinical Stage IA Lung Squamous Cell Carcinoma. Chest 2017;152:1239-50. [Crossref] [PubMed]
- Setio AA, Jacobs C, Gelderblom J, et al. Automatic detection of large pulmonary solid nodules in thoracic CT images. Med Phys 2015;42:5642-53. [Crossref] [PubMed]
- Setio AA, Ciompi F, Litjens G, et al. Pulmonary Nodule Detection in CT Images: False Positive Reduction Using Multi-View Convolutional Networks. IEEE Trans Med Imaging 2016;35:1160-9. [Crossref] [PubMed]
- National Lung Screening Trial Research Team. Reduced lung-cancer mortality with low-dose computed tomographic screening. N Engl J Med 2011;365:395-409. [Crossref] [PubMed]
- Janssen-Heijnen ML, Maas HA, Houterman S, et al. Comorbidity in older surgical cancer patients: influence on patient care and outcome. Eur J Cancer 2007;43:2179-93. [Crossref] [PubMed]
- Cone EB, Marchese M, Paciotti M, et al. Assessment of Time-to-Treatment Initiation and Survival in a Cohort of Patients With Common Cancers. JAMA Netw Open 2020;3:e2030072. [Crossref] [PubMed]
- NVALT, Richtlijn Niet Kleincellig Longcarcinoom Mediastinale Stadiëring. Federatie Medisch Specialisten, 2020. Available online: https://richtlijnendatabase.nl/richtlijn/niet_kleincellig_longcarcinoom/startpagina_-_niet-kleincellig_longcarcinoom.html
- Goldstraw P, Chansky K, Crowley J, et al. The IASLC Lung Cancer Staging Project: Proposals for Revision of the TNM Stage Groupings in the Forthcoming (Eighth) Edition of the TNM Classification for Lung Cancer. J Thorac Oncol 2016;11:39-51. [Crossref] [PubMed]
- Ma C, Bandukwala S, Burman D, et al. Interconversion of three measures of performance status: an empirical analysis. Eur J Cancer 2010;46:3175-83. [Crossref] [PubMed]
- Austin PC. A Tutorial on Multilevel Survival Analysis: Methods, Models and Applications. Int Stat Rev 2017;85:185-203. [Crossref] [PubMed]
- Mihaela Ene, E.A.L. Genine L. Blue, Bethany A. Bell, Multilevel Models for Categorical Data Using SAS® PROC GLIMMIX: The Basics. SAS Global Forum Proceedings, 2015.
- Beyaz F, Verhoeven RLJ, Schuurbiers OCJ, et al. Occult lymph node metastases in clinical N0/N1 NSCLC; A single center in-depth analysis. Lung Cancer 2020;150:186-94. [Crossref] [PubMed]
- Sun J, Wu S, Jin Z, et al. Lymph node micrometastasis in non-small cell lung cancer. Biomed Pharmacother 2022;149:112817. [Crossref] [PubMed]
- Dai C, Xie H, Kadeer X, et al. Relationship of Lymph Node Micrometastasis and Micropapillary Component and Their Joint Influence on Prognosis of Patients With Stage I Lung Adenocarcinoma. Am J Surg Pathol 2017;41:1212-20. [Crossref] [PubMed]
- Cui Y, Wang W, Yao S, et al. Relationship between circulating lung-specific X protein messenger ribonucleic acid expression and micrometastasis and prognosis in patients with early-stage nonsmall cell lung cancer. J Cancer Res Ther 2020;16:1641-7. [Crossref] [PubMed]
- Deng XF, Jiang L, Liu QX, et al. Lymph node micrometastases are associated with disease recurrence and poor survival for early-stage non-small cell lung cancer patients: a meta-analysis. J Cardiothorac Surg 2016;11:28. [Crossref] [PubMed]
- Jeong JH, Kim NY, Pyo JS. Prognostic roles of lymph node micrometastasis in non-small cell lung cancer. Pathol Res Pract 2018;214:240-4. [Crossref] [PubMed]
- Lafuente-Sanchis A, Olmo A, Carretero J, et al. Clinical significance of epithelial-mesenchymal transition-related markers expression in the micrometastatic sentinel lymph node of NSCLC. Clin Transl Oncol 2020;22:381-91. [Crossref] [PubMed]
- Felip E, Altorki N, Zhou C, et al. Adjuvant atezolizumab after adjuvant chemotherapy in resected stage IB-IIIA non-small-cell lung cancer (IMpower010): a randomised, multicentre, open-label, phase 3 trial. Lancet 2021;398:1344-57. [Crossref] [PubMed]
- Yuan P, Cao JL, Rustam A, et al. Time-to-Progression of NSCLC from Early to Advanced Stages: An Analysis of data from SEER Registry and a Single Institute. Sci Rep 2016;6:28477. [Crossref] [PubMed]
- Kanarek NF, Hooker CM, Mathieu L, et al. Survival after community diagnosis of early-stage non-small cell lung cancer. Am J Med 2014;127:443-9. [Crossref] [PubMed]
- Mayne NR, Elser HC, Darling AJ, et al. Estimating the Impact of Extended Delay to Surgery for Stage I Non-small-cell Lung Cancer on Survival. Ann Surg 2021;273:850-7. [Crossref] [PubMed]
- Diaconescu R, Lafond C, Whittom R. Treatment delays in non-small cell lung cancer and their prognostic implications. J Thorac Oncol 2011;6:1254-9. [Crossref] [PubMed]