Biomarkers of lung cancer for screening and in never-smokers—a narrative review
Introduction
Lung cancer
Lung cancer is the leading cause of cancer-related mortality worldwide (1). In 2018, lung cancer accounted for an estimated 1,761,000 deaths, 18.4% of all cancer deaths worldwide (2). While overall cancer survival has improved in the last 30 years, with 70% of all individuals with cancer surviving 5 years after diagnosis, only 20% of individuals with lung cancer survive past 5 years following diagnosis (3). This high mortality rate can be attributed to late-stage diagnosis, with 75% of lung cancer diagnoses presenting at stages III and IV (4). In order to successfully treat individuals with lung cancer and reduce disease mortality, improvements must be made in early disease detection, screening, and prevention.
Overview of biomarkers
Biomarkers are biologically significant molecules that reflect the homeostatic state of the host tissue and can be used for identifying and characterising disease states, notably cancer (5). Biomarkers have a wide range of applications from disease diagnosis and screening, to predicting patient outcomes and responses to treatment (6). Biologically significant markers of disease have long been assessed for their applicability in disease management. Biomarkers can be classified as nucleic acid markers, protein markers, or cellular markers (Figure 1), referring to the functional level at which they operate (7). Recent interest in biomarkers of lung cancer has seen the identification of a wide range of markers with significant clinical applications, most prominently in cancer characterisation, with the identification of a range of targetable driver mutations which have significantly improved lung cancer treatment (8-10). Focus is now beginning to shift towards markers for early detection that work to either complement or potentially replace current diagnostic and screening methods with the aim of identifying lung cancer in its early stages while curative treatment options are still viable (11). Biomarkers of lung cancer are a promising area of research for screening, as various markers have shown promise in helping to identify individuals who are at higher risk of developing lung cancer and in distinguishing between benign and malignant nodules. In particular, biomarkers targeting never-smokers, who have a distinct pathogenesis of lung cancer, could be especially valuable. Therefore, this article will discuss the current state of biomarker research for lung cancer, specifically in screening and in never-smokers, and its potential applications in clinical practice.
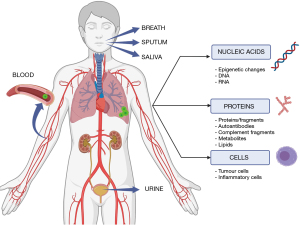
Biomarkers for lung cancer screening (LCS)
LCS using computed tomography (CT) has been validated as an effective method for diagnosing lung cancer. The National Lung Screening Trial and the Dutch-Belgian Randomized Lung Cancer Screening Trial have demonstrated an improvement in the diagnostic efficacy of CT screening compared to chest radiography or no screening (12,13). When detected in its earliest stage (stage IA), individuals with lung cancer have a 5-year survival rate of 92%, in contrast to later disease stages (stage IV) with only 10% of individuals surviving longer than 5 years (4). Thus, LCS aims to improve mortality by diagnosing early-stage lung cancer in high-risk populations, and in this way, allowing early treatment.
While LCS is advantageous for its simplicity and high sensitivity, limitations include high number of false positive results and radiation hazard, amongst others (13,14). As a consequence, older individuals tend to be selected, possibly excluding others that would benefit (15). To complement CT screening and overcome its limitations, body fluid biomarkers could be used as a minimally invasive approach (16,17) to better identify those most likely to benefit from screening and those who need more frequent monitoring or more aggressive intervention once in a screening program. This approach could help minimize healthcare cost by reducing interventions and invasive procedures in individuals at a lower risk of lung cancer who have less to gain from screening but similar exposure to potential harms (18).
Biomarkers of lung cancer in never-smokers (LCINS)
Lung cancer occurs most commonly as a result of cigarette smoking (19). As such, the focus on screening individuals with a significant smoking history for lung cancer is justified, however those with negligible smoking histories who may still be at risk fall through the gaps of screening efforts (20). LCINS represents a growing subgroup of individuals, up to 25% of lung cancer diagnoses worldwide, and is a significant contributor to cancer-related mortality (21-23). Common risk factors other than cigarette smoke include exposure to coal dust, asbestos, indoor and outdoor pollutants, environmental radon, nickel, chromium, and ionising radiation, as well as pre-existing respiratory conditions and genetic abnormalities (22,24-27). Radon exposure is considered the leading cause of LCINS—and the second leading cause of lung cancer overall—with individuals becoming exposed to high levels of radon when living or working in buildings with poor ventilation in areas of high environmental radon (28,29). While reported rates vary, 60–90% of lung cancers in people who have never smoked are adenocarcinomas (30,31). Although mortality rates greatly vary in LCINS, it is characterized by longer survival times than smoking-related lung cancer, despite typically being diagnosed at later disease stage (30,32). To reduce the high disease burden and mortality rate associated with LCINS, improved methods of cancer screening and early detection are required, and numerous biomarkers of LCINS have been identified and investigated over the last decade.
Focussed aims
Although lung cancer biomarkers are the subject of intense research worldwide, there remains comparatively little information on their clinical efficacy and how this compares to current LCS strategies. Further, LCINS has been identified as molecularly, histologically, and pathologically distinct from tobacco smoking-related lung cancers and as such, requires distinctive characterisation (33,34). In this review we provide a focussed up to date summary on:
- Body-fluid biomarker research for LCS and how it may supplement screening strategies, and
- Circulating biomarkers of LCINS lending attention to applications for lung cancer detection.
We present this article in accordance with the Narrative Review reporting checklist (available at https://tlcr.amegroups.com/article/view/10.21037/tlcr-23-291/rc).
Methods
Search strategy
The search strategy is presented in Table 1. Briefly, in August 2022 we performed two systematic literature searches of English-language publications using the terms “lung cancer”, “biomarker”, and “CT screening”, as well as “never-smoking”, “lung cancer”, and “biomarkers” on MEDLINE, CINAHL, Embase, and Web of Science databases.
Table 1
Items | Lung cancer screening | Lung cancer in never-smokers |
---|---|---|
Date of search | 18th–24th of August 2022 | 18th–24th of August 2022 |
Databases and other sources searched | MEDLINE, CINAHL, Embase, and Web of Science databases | MEDLINE, CINAHL, Embase, and Web of Science databases |
Search terms used | Detailed example on Table S1 | Detailed example on Table S2 |
Timeframe | Studies published since 2010 | Studies published since 2012 |
Inclusion and exclusion criteria | Inclusion criteria: | Inclusion criteria: |
• People undergoing lung cancer screening by computed tomography | • Never-smokers diagnosed with lung cancer | |
• Biomarkers in body fluids used as intervention | • Biomarker in body fluids and breath used as intervention | |
• Improved participant selection for lung cancer screening, or improved nodule management after screen detection | • Early-stage lung cancer detection | |
• English-language articles | • English-language articles | |
Exclusion criteria: | Exclusion criteria: | |
• Non-human studies | • Non-human studies | |
• Metastatic lung cancers | • Metastatic lung cancers | |
• Editorials, reviews, and case reports | • Editorials, reviews, and case reports | |
• No full text available | • No full-text available | |
Selection process | Studies obtained from our search strategy were imported into systematic review management software “Covidence”. After removing duplicates, one researcher (Jazmin Guayco Sigcha) evaluated relevance of all titles and abstract following the inclusion criteria for this review | Studies obtained from our search strategy were imported into systematic review management software “Covidence”. After removing duplicates, two researchers (Edward Stephens and Kenneth Lopez-Loo) independently evaluated relevance of all titles and abstract following the inclusion criteria for this review |
Eligibility criteria and study selection
Identified publications were de-duplicated and assessed for relevance based on title and abstract screening. Full texts of potentially relevant articles were retrieved for final eligibility check followed by data extraction (Covidence systematic review software, Melbourne). The NHMRC Evidence Hierarchy and the NIH Early Detection Research Network Five-Phase Approach were used to rank the level of evidence and development phase of the identified biomarkers. Biomarkers from all non- and minimally-invasive biological sources were eligible (Figure 1).
Results
Our literature search yielded 1,433 and 958 articles for LCS and LCINS, respectively. For LCS, our literature search yielded 1,433 articles: 569 from MEDLINE, 141 from CINAHL, 436 from Embase and 287 from Web of Science. For LCINS, our literature resulted on 958 articles: 457 from MEDLINE, 54 from CINAHL, 215 from Embase and 232 from Web of Science. After removing duplicates, articles that do not cover the topic of body-fluid biomarkers for LCS or circulating biomarkers in never-smokers were excluded. Following screening, these were reduced to 22 and 91 total studies, respectively (Figures S1,S2). Table 2 comprises a list of the biomarkers included in this review. Although we searched for studies without discrimination based on sample type (Table 1), our review yielded blood- and sputum-borne markers only. We discuss our findings in the following paragraphs according to biomarker type.
Table 2
Biomarker | Biomarker source | Advantages | Disadvantages | Development phase | Reference |
---|---|---|---|---|---|
Nucleic acid markers | |||||
DNA markers | |||||
Microsatellite instability/loss of heterozygosity and plasma DNA | Sputum/plasma | High sensitivity, minimally invasive | Moderate specificity | Phase 3 | (35) |
DNA methylation | Sputum | High sensitivity, minimally invasive | Low sensitivity | Phase 3 | (36) |
MicroRNA markers | |||||
MicroRNA-155 | Plasma | High sensitivity and specificity, minimally invasive, detects NSCLC at early-stage | No large-scale validation | Phase 3 | (37-41) |
Multiple microRNA panels | Plasma/serum | High sensitivity, stable in serum in plasma, specific to lung cancer, minimally invasive | No large-scale validation | Phase 4 | (42-45) |
Protein markers | |||||
Osteopontin | Plasma | Can be used for both risk and nodule stratification, minimally invasive | Not unique to lung cancer, evidence is still in early stage (control-case) | Phase 2 | (46) |
Carcinoembryonic antigen | Serum | Minimally invasive, high sensitivity | Low sensitivity | Phase 2 | (47,48) |
Combination proteins | Serum | Can be used for both risk and nodule stratification, cost-effective, minimally invasive | Overlap with other cancers and inflammatory diseases | Phase 2 | (49,50) |
Complement fragments | Plasma | Minimally invasive | Discrepancies in results between studies, quantification of C4d is not standardised | Phase 3 | (51-53) |
T-cell receptors | Peripheral blood | Stable in pathological subtypes, minimally invasive | Only studied in stage I lung cancer, limited to Asian population | Phase 3 | (54) |
Autoantibodies | Peripheral blood | Panel compensate for cancer heterogeneity, assessed in randomized control trial, minimally invasive | Moderate sensitivity | Phase 5 | (55) |
Metabolites | Serum | Panel has high sensitivity and specificity, minimally invasive | Biomarkers are still in exploratory stage | Phase 4 | (56-58) |
Circulating lipids | Serum | High sensitivity and specificity, minimally invasive, detects NSCLC at early-stage | Confounded by systemic pathology, validated in female only cohort | Phase 3 | (59) |
Cellular markers | |||||
Circulating tumour cells | Bloodstream | High detection rate by CellCollector, minimally invasive | Confounded by systemic pathology, non-specific | Phase 4 | (60,61) |
Circulating inflammatory cells | Bloodstream | Minimally invasive | Confounded by systemic pathology, non-specific | Phase 3 | (62,63) |
The NIH Early Detection Research Network Five-Phase Approach was used to rank biomarker development. NSCLC, non-small cell lung cancer; LCS, lung cancer screening.
Nucleic acid markers of lung cancer
Gradual accumulation of genetic and epigenetic changes in the cell nucleus can be used to detect lung cancer formation, progression, and metastasis (64). While the disease is primarily driven by somatic alterations, typically linked to smoking exposure, germline mutations could also predispose individuals to lung cancer development (65,66). Emerging as promising biomarkers, nucleic acid markers for lung cancer such as cell-free DNA (cfDNA) and circulating RNA are significantly advancing lung cancer diagnosis through the immense potential of liquid biopsy detection methods. The advent of real-time polymerase chain reaction (PCR) and next-generation sequencing has enhanced the sensitivity and specificity of circulating nucleic acid analysis, making it a valuable asset in the arsenal of lung cancer detection methods (67,68).
DNA
Microsatellite instability/loss of heterozygosity and cfDNA
Lung cancer contains highly altered chromosomal regions, strongly influenced by smoking habits, although not all are specific to lung cancer (69-71). Furthermore, cfDNA concentration in individuals with lung cancer is usually several times higher than in healthy individuals, most likely due to necrosis/apoptosis of cancerous tissue or circulating cancer cells (72). The ITALUNG biomarker panel measured microsatellite instability/loss of heterozygosity and cfDNA in plasma and retrospectively discriminated lung cancer with high sensitivity (90%) and moderate specificity (62%) in 154 individuals with screen-detected cancers and 486 screening controls (35).
When circulating DNA (cirDNA) is detected in the blood, it typically exhibits a distinctive size profile due to its high degree of fragmentation (73,74). This emerging field of research is known as fragmentomics and has recently gained attention for its applicability in pan-cancer screening, and involves the analysis of both transcriptional and topological features of cirDNA (75). Corroborating the utility of this approach, a study using a five circulating cfDNA feature fragmentomic model successfully distinguished between healthy participants and patients with lung cancer across three validation cohorts with high sensitivity (91.4%, 84.7%, and 92.5%) and specificity (95.7%, 98.6%, and 94.2%) in all three groups; additionally, the model showed sensitivity of 83.2% when identifying stage I lung cancer (76). While promising, to further build on these findings, validation in asymptomatic screening cohorts is required.
A different approach to cancer screening are multi-cancer early detection (MCED) tests, which aim to detect different types of cancer at an early stage for further diagnosis. Numerous MCED tests have successfully discriminated multiple cancers from healthy individuals, but most of these findings have been reported in clinical rather than screening population (77). In an exclusively female screening program, the CancerSEEK test—which used a multi-analyte gene mutation and protein panel—demonstrated high specificity (99.6%) when discriminating 9 targeted types of cancer, including lung cancer, from healthy controls when used in conjunction with positron emission tomography (PET)-CT (78). Still, participants enrolled on MCED studies are not reflective of LCS populations. Furthermore, the inability to determine the type of cancer in patients who have tested positive for MCED may lead to difficult clinical follow-ups. Future studies should focus on determining the feasibility of these tests in LCS populations alone by assessing their diagnostic performance in asymptomatic individuals at a high risk of developing lung cancer.
Methylation
Among epigenetic changes, DNA hypomethylation and hypermethylation of specific 5'-C-phosphate-G-3 (CpG)-rich regions in the promoter region of tumour suppressor genes are early events in carcinogenesis, making them markers of interest for early lung cancer detection (79-81). In the context of LCS, a retrospective study analysing DNA hypermethylation in sputum found that a panel of Ras association domain-containing protein 1 (RASSF1), 3-O-sulphotransferase 2 and PR/SET Domain 14 hypermethylation was able to discriminate high-risk screened individuals with (n=65) and without (n=99) lung cancer with specificity of 90%, although it showed a sensitivity of just 28%. When focusing on individual biomarkers, RASSF1 hypermethylation demonstrated the best diagnostic performance with 93% specificity but only 17% sensitivity (36). Further, MCED biomarkers assessed in the PanSeer screening program—which analysed 11,787 CpG sites across 595 regions in the genome from plasma DNA—successfully discriminated 95% [confidence interval (CI): 89–98%] of patients with eight different cancers, including lung cancer, from healthy controls (82). Nevertheless, longitudinal studies are required to validate these results and, as previously stated, future studies need to take into account the specific characteristics of individuals at a high risk of developing lung cancer in order to be of relevance for LCS.
DNA methylation has been linked to environmental exposures and comorbidities other than lung cancer, adding a layer of complexity to its potential use as a biomarker for lung cancer detection. Research has found correlations between methylation patterns and exposure to traffic-related pollutants, polycyclic aromatic hydrocarbons, and particulate matter rich in metals, affecting genes associated with immune responses and other processes (83-85). Moreover, methylation has been tied to medical conditions such as osteoporosis, obesity and chronic obstructive pulmonary disease (COPD), with studies showing distinct methylation profiles in individuals with these diseases compared to healthy controls (86-88). Obesity, in particular, has been associated with alterations in DNA methylation, influencing the likelihood of developing diseases like type 2 diabetes (87). Factors such as the intrauterine environment, physical activity, and diet can also impact both obesity and DNA methylation (89). Therefore, when investigating methylation as a potential biomarker for lung cancer, it is crucial to consider these additional influences.
MicroRNAs (miRNAs)
miRNAs are small noncoding RNA molecules that modulate gene activity and are aberrantly expressed in cancer (90). Protected from degradation by encapsulation in exosomes, circulating RNA remains stable in cancer patients, even amid an increase in ribonuclease activity (91). This stability facilitates cancer detection and characterization through microarray technologies or quantitative PCR (92). As a result of their small size and stability, miRNAs can be detected in fluids such as plasma and serum (93) and have been shown to successfully differentiate prostate, colon, and lung cancer (94-96). A retrospective study of a panel consisting of 24 plasma-derived miRNAs, the miRNA signature classifier, showed high sensitivity (87%) and specificity (81%) in 58 screen-detected lung cancers and 594 screening controls (42). Likewise, a prospective study of a panel consisting of 34 serum-derived miRNAs demonstrated high sensitivity (71%) and specificity (90%) when discriminating 34 screen-detected lung cancers from 30 screening controls (43). Refinement of this panel resulted in a panel of 13-miRNAs with high sensitivity (77.8%) and specificity (74.8%) when discriminating 48 screen-detected cancers from 1,067 screening controls (44). Further, a signature composed of 15 miRNAs was not only able to discriminate 16 screen-detected cancers from 10 screening controls with high sensitivity (80%) and specificity (90%), but could also predict risk of lung cancer, up to 8–9 months before conventional diagnostic methods (45). High sensitivity and specificity shown in these studies illustrate the potential of miRNAs in LCS, but future studies need to validate the diagnostic performance in different populations.
miR-155
While the aforementioned miRNA panels successfully discriminated screen-detected cancers from healthy controls, never-smokers were omitted from these cohorts. A potential miRNA candidate for early cancer detection in never-smokers is miR-155, an miRNA known to function as a mediator of immune response and the immune system, however, when dysregulated, is widely implicated in immune-centric diseases including chronic inflammation, auto-immunity, and cancer, among others (37). A 2014 study identified the up-regulation of miR-155 in plasma was predictive of early stage (stage I and II) LCINS (n=37) compared to healthy controls (n=60) with a sensitivity of 91%, a specificity of 93% (38). Further, studies investigating the role of miR-155 in non-small cell lung cancer (NSCLC) identified its involvement in cellular apoptosis, drug and chemo-resistance, as well as being linked with reduced overall survival (39-41). Interestingly, these studies did not report any differences in overall survival or chemoresistance associated with smoking status, potentially indicating miR-155 as a pan-NSCLC biomarker.
Protein markers of lung cancer
Proteins mediate homeostatic as well as pathological processes and are potential biomarkers (97). Cancer-induced aberrations result in a marked difference in protein translation and expression between normal and cancer cells (98,99). Furthermore, monitoring of cancer-related metabolites in blood has been an emerging approach to detect different malignancies in recent years (100).
Osteopontin (OPN)
The secreted phosphoprotein 1 gene (SPP1) encodes the protein OPN. SPP1 is upregulated in the cancer tissue of early-stage and relapsed NSCLC individuals as well as in LCINS (101-105). OPN is of interest as a lung cancer biomarker as its activity leads to numerous downstream processes associated with cancer progression and cellular transformation (106) by promoting cell migration, invasion, and adhesion, as well as playing an important role in immune cell recruitment, wound healing, and tissue remodelling (107-109). In a case-control study, change-over time of plasma OPN levels (OPN velocity or OPNV) were linked to a statistically significant increase in lung cancer risk [area under the curve (AUC) of 0.88] in 10 screen-detected incident cancers matched to 1–4 screening controls each. Furthermore, when analysis was limited to cancer cases and controls presenting ground glass opacities or stable solitary nodules, OPNV could differentiate between malignant and benign nodules with an AUC of 0.91 (46). Still, validation studies using larger LCS cohorts are necessary to confirm the diagnostic value of plasma OPN.
Carcinoembryonic antigen (CEA) and combination markers
CEA is a glycoprotein produced during foetal development and is usually absent in healthy adults (110); however, it is widely reported as a marker of lung cancer with potential as a predictor of disease prognosis. A South-East Asian study investigating the relationship between serum biomarkers and residential radon levels in never and former (>15 years) smokers, described a significant increase in serum CEA and cytokeratin 19 fragment (CYFRA21-1) in individuals with lung cancer, compared to heathy controls with high and low radon exposure. Interestingly, an increase in CEA (P=0.009) and CYFRA21-1 (P=0.0031) was also observed in healthy controls with high radon exposure when compared to low, potentially indicating high serum CEA as a biomarker for lung cancer development in never-smokers. Receiver operating characteristic analyses of CEA and CYFRA21-1 for diagnosing lung cancer illustrate high specificity (98% and 94% respectively) but inadequate sensitivity (57.3% and 58.6% respectively), which similarly has been reported in other studies investigating CEA as a biomarker of NSCLC and mutational status (48,111,112).
CEA has also been assessed in a panel with other protein markers such as CYFRA21-1, progastrin-releasing peptide (ProGRP), and squamous cell carcinoma (SCC) antigen. CYFRA21-1 is abundant in pulmonary tissue, with concentrations particularly elevated in SCC where it correlates with tumour size, lymph node status and cancer stage (113,114). In a prospective cohort of older smokers (n=634), participants with a positive or indeterminate CT scan result (n=92) were tested for CEA and CYFRA21-1. In the 17 cases with screened-lung cancer, positivity in either biomarker yielded a higher AUC than CT alone (CEA 0.75; CEA/CYFRA21-1 0.76; CT 0.68) (50). In a different prospective case-control study, ProGRP, SCC, CEA, and CYFRA21-1 showed acceptable discrimination (AUC =0.719) between screen-detected lung cancers and screening controls (n=715; China). However, when analysis was limited to cancer cases and controls presenting benign nodules, discrimination was very limited in the validation data set (AUC =0.5836) (49). When considering never-smokers, the combination of CEA and CYFRA21-1 may be more clinically informative than when assessed independently, however further research is required to evaluate the efficacy of this in a clinical setting (47,111,115-117).
Immunological markers
The complement system is a central component of innate immunity and plays an essential role in immune surveillance and homeostasis. The C4d split-product, is a passive indicator of complement pathway activation that is found in higher concentrations in biological fluids of individuals with lung cancer (118). In an independent cohort study, plasma C4d levels were linked to increased lung cancer risk in 32 screen-detected incident cancers compared to 158 screening controls (AUC =0.735) (51), however, its use as a marker for LCS was not validated in a study of 20 screen-detected incident cancers matched to two screening controls each [odds ratio (OR) =1.53; 95% CI: 0.93–2.51; P=0.079] (52). Unlike C4d, C4c is released to the extracellular milieu upon fragmentation during activation of the classical pathway of complement, thus making it more detectable in bodily fluids, such as plasma (119). In a case-control study the panel of plasma C4c plus CYFRA 21-1 and C-reactive protein discriminated screen-detected cancers (n=32) from screening controls (n=93) with a sensitivity of 73% and specificity of 70% (53).
T-cell receptors (TCRs)
A prior investigation found that TCRs present in the bloodstream can differentiate between individuals with lung cancer and healthy controls (120). In the context of LCS, a retrospective study indicated that lung cancer-associated TCRs in peripheral blood could distinguish stage I screened-cancers (n=52) from screening controls (n=94) with high sensitivity (72%) and specificity (91%). In addition, the sensitivity was stable in pathological subtypes, being 73% in lung SCC and 71% in lung adenocarcinoma (54).
Autoantibodies
Autoantibodies develop in response to an abnormal cancer antigen in individuals with lung cancer, often well before symptoms arise or screening detection is possible (121,122). Autoantibodies are usually absent or found in low levels not only in those without cancer but also in many individuals with the disease, thus they are likely to be specific but not sensitive (123). A randomised trial evaluating the EarlyCDT-Lung test for up to 2 years indicated a high specificity (90.4%) but low sensitivity (32.1%) for detecting lung cancer in 127 screen-detected cancers and 11,610 controls (55). Tumour-induced suppression of immune responses can induce a reduction of autoantibody production and detection, which would explain the low sensitivity of the test at 2 years (124). Still, EarlyCDT-lung test could be used in combination with CT to ensure a high detection rate of stage I/II lung cancer cases. A limitation of this study is the absence of CT scans for EarlyCDT-Lung test negative participants and control arm participants, necessary to evaluate the test’s effectiveness in LCS.
Metabolites
Serum and plasma sampling has revealed metabolites with the power to discriminate individuals with lung cancer from healthy individuals and individuals with benign cancers (125-128). When investigating metabolites used in LCS, one prospective study showed significantly elevated levels of serum metabolites, particularly, L-(+)-glucose, cysteinyl-glutamine, phosphatidylethanolamine (PE) [22:2(13Z,16Z)/15:0] and threonine-glutamine, in 34 individuals with ground glass opacities compared to 39 healthy controls (56). Similarly, a cross-sectional study demonstrated that PE (34:2), PE (36:2) and PE (38:4) have modest accuracy (69%, 71% and 67%, respectively) when discriminating screen-detected lung cancers (n=29) from screen-detected benign nodules (n=25); though additional analyses are required to validate the diagnostic value of the compounds discovered in this study (57). When examining panels, a classifier including nine serum metabolites allowed the discrimination of 31 screen-detected lung cancers from 92 matched screening controls with 100% sensitivity and 95% specificity (58).
Lipid profiling
Carcinogenesis disturbs normal lipid metabolism, thus making lipidomics a promising tool for early identification of lung and other cancers (59,129). A proposed marker for the early detection of LCINS is a combinational serum marker of three lipid products of fatty acid (FA) metabolism; FA (20:4), FA (22:0), and lysophosphatidylethanolamine (20:4). Together, these markers successfully distinguished never-smoking females with NSCLC (discovery set n=39, validation set n=25) from healthy controls (discovery set n=46, validation set n=17) with high sensitivity (discovery set 0.949, validation set 1.000) and specificity (discovery set 1.000, validation set 1.000), including those with early-stage disease (59). In addition to this, lipid metabolites have also been identified as potential markers for lung cancer detection in smoking-related NSCLC however these findings have also not been validated in larger cohort studies (130,131).
Cellular markers of lung cancer
Cellular changes promoted by cancer development can be assessed using various markers routinely measured in common blood tests or as ratios derived from these measurements (132). The association between blood cell ratios related to systemic inflammation and cancer risk shows potential as a biomarker for earlier identification of the disease.
Circulating tumour cells (CTCs)
CTCs are metastatic tumour cells that have emerged from the primary tumour site into the bloodstream to form secondary tumours at distinct sites (133). These cells present notable advantages for use as biomarkers as they can be sourced minimally-invasively and provide diagnostic and prognostic information (134,135). CTC collection is not standardised but uses minimally- or non-invasive methods that avoid tissue biopsy (136,137). The isolation by size of epithelial tumour cells (ISET) test uses fixed blood samples and vertical filtration to capture rare cells and CTCs, thus preserving the integrity of the cells for subsequent analysis (138); as a result, many clinical investigations have opted to employ ISET technology for CTC isolation (139). However, a prospective cohort study evaluating the performance of CTCs isolated by ISET test in a high-risk population with COPD found the sensitivity (26.3%) was too low for LCS (61). This study deliberately chose individuals with COPD, as this population is considered at a high risk of developing lung cancer independent of cigarette smoking and so it should be of interest for LCS (140,141). On the other hand, CellCollector, an in-vivo isolation method based on epithelial-cell adhesion molecule (EpCAM) recognition, was found to be able to discriminate screen-detected cancers (n=24) from matched screening controls (n=72) with 62.5% sensitivity and 100% specificity (60). This method involves inserting a wire with EpCAM antibodies on its surface into the cubital vein through a cannula, leaving it there for 30 minutes, and then removing and identifying the captured cells on the wire via immunofluorescence staining (60,142). However, its implementation in the clinic is limited as it requires manual screening for the detection of CTCs and is more invasive for individuals being screened compared to other methods (143). Different technologies for combined enrichment, detection, and characterisation of CTCs will need to be explored in order to use CTCs as a LCS marker.
Systemic markers of inflammation
Systemic inflammation is widely acknowledged to play a key role in carcinogenesis and cancer progression: inflammatory cells are recruited via cytokines and can enhance carcinogenesis and cancer progression; platelets release factors that aid tumour growth, invasion and angiogenesis; lymphocytes play a vital part in the production of cytokines, limiting cancer cell growth and causing cytotoxic cell death (144-149). As such, circulating immune cells can be used as markers of disease states. In one study, elevated pre-diagnosis neutrophil-to-lymphocyte ratio (NLR) in heavy smokers was associated with an increased risk of developing small cell lung cancer, but not NSCLC (150). While never-smokers were not represented in this study, increased NLR has been identified as a risk factor for lung cancer independent of smoking history (151), however its efficacy as a biomarker for lung cancer detection has not been tested in a cohort or randomised study. When looking into systemic inflammation used as biomarkers in LCS, a retrospective study evaluating the annual change of NLR and the platelet-to-lymphocyte ratio (PLR)—another marker of systemic inflammation—indicated that an increase in PLR was significantly associated with lung cancer risk in 32 individuals with screen-detected cancer and 103 screening controls (62). A different retrospective study assessed the systemic immune-inflammation index (SII), NLR and PLR in a Chinese population comprised of screen-detected cancers (n=569) and screening controls (n=95,907), and discovered that high PLR and SII have a significant association with lung cancer, while NLR exhibited a U-shaped association (63). While it is evident markers of systemic inflammation hold diagnostic significance, it should be taken into account that neutrophil, lymphocyte, and platelet counts are nonspecific parameters for lung cancer and may be influenced by concurrent comorbidities (152). Furthermore, considering that both COPD and the extent of airway obstruction are linked to elevated levels of systemic inflammation, future research must take these variables into account while evaluating the differences in inflammation markers between cancer and non-cancer individuals (153,154).
Conclusions
Lung cancer is the leading cause of cancer mortality worldwide largely as a result of late-stage diagnoses. To address this, improvements must be made to current screening measures to identify cancer early, as to allow for the best possible outcomes for the patient. Biomarkers are playing an emerging role in the early detection and management of lung cancer, with applications in screening and detection.
We identified numerous biomarkers for LCS with varying levels of supporting evidence. Metabolites have shown the highest sensitivity and specificity to lung cancer when compared to healthy controls, however, abnormal circulating metabolites are known to be indicative of systemic pathologies and may not be lung cancer specific. Similarly, cellular markers of disease such PLR and SII also occur as result of comorbid disease. While valuable, these markers are likely not applicable for specific LCS strategies for early cancer detection. As such, it is likely that a panel of tumour-specific circulating proteins or nucleic acids would be the most viable for targeted screening strategies. We described recent evidence around miRNA panels for LCS; while the evidence for these markers was not as strong, these markers are less likely to be confounded by other systemic pathologies and, as such, are likely would make more effective markers for LCS. Further, fragmentomics is an emerging area with great potential for biomarker discovery due to its ability to identify numerous tumour-derived changes in cirDNA. However, current detection models lack clinical validation. Despite this, circulating cfDNA remains an attractive target for early lung cancer detection.
LCINS is becoming increasingly prevalent, appearing to be clinicopathologically distinct to smoking-related lung cancers, and therefore unique approaches to its management may be required. Of the markers identified, lipid panels demonstrated the highest sensitivity and specificity when discriminating LCINS compared to healthy controls, however, abnormal lipid levels are known to be indicative of systemic pathologies and may not be lung cancer specific. Thus, nucleic acids are likely the most viable biomarker for LCINS, the most efficacious of which is miR-155. Evidence suggests miR-155 may be a valid pan-NSCLC marker however confirmation in large, randomised studies is required.
Several markers in various stages of development are currently available for LCS and LCINS, and further advancement in terms of external validation and impact assessment is in progress. Randomised trials are considered to be the gold standard for external validation (155). However, proving ultimate evidence of mortality benefits is challenging and may take a significant amount of time. As a result, more time- and cost-effective models are increasingly being used to complement clinical decision-making with the aim of improving patient outcomes (156,157). Such models have already been implemented to compare the effectiveness of certain biomarkers in LCS (158).
To conclude, significant advancements have been made in the field of lung cancer biomarker research, with numerous biomarkers of lung cancer displaying varying levels of clinical efficacy and showing improvement in diagnostic accuracy over standard clinical workflow in LCS and LCINS. The priority now should be the validation of existing candidate markers in appropriate clinical contexts to integrate these into clinical practice. To do this, randomized controlled trials or similar methods of validation should be designed to test the efficacy of these biomarkers. This will positively impact lung cancer diagnosis and treatment, and help to reduced lung cancer mortality worldwide.
Acknowledgments
We would like to acknowledge The Prince Charles Hospital Library team for performing the literature search of MEDLINE, CINAHL, Embase, and Web of Science databases.
Funding: None.
Footnote
Reporting Checklist: The authors have completed the Narrative Review reporting checklist. Available at https://tlcr.amegroups.com/article/view/10.21037/tlcr-23-291/rc
Peer Review File: Available at https://tlcr.amegroups.com/article/view/10.21037/tlcr-23-291/prf
Conflicts of Interest: All authors have completed the ICMJE uniform disclosure form (available at https://tlcr.amegroups.com/article/view/10.21037/tlcr-23-291/coif). KMF serves as an unpaid editorial board member of Translational Lung Cancer Research from August 2023 to July 2025. KMF has received competitive Research Grant Funding from MRFF and NHMRC in the past 36 months, honoraria or royalties from UpToDate and Cochrane Clinical Answers, travel support funding from WCLC, ATS, PCCP, and in kind equipment funding from Olympus and Mevis Veolity. KMF is the Former President of the Asia Pacific Society of Respirology. The other authors have no conflicts of interest to declare.
Ethical Statement: The authors are accountable for all aspects of the work in ensuring that questions related to the accuracy or integrity of any part of the work are appropriately investigated and resolved.
Open Access Statement: This is an Open Access article distributed in accordance with the Creative Commons Attribution-NonCommercial-NoDerivs 4.0 International License (CC BY-NC-ND 4.0), which permits the non-commercial replication and distribution of the article with the strict proviso that no changes or edits are made and the original work is properly cited (including links to both the formal publication through the relevant DOI and the license). See: https://creativecommons.org/licenses/by-nc-nd/4.0/.
References
- Sung H, Ferlay J, Siegel RL, et al. Global Cancer Statistics 2020: GLOBOCAN Estimates of Incidence and Mortality Worldwide for 36 Cancers in 185 Countries. CA Cancer J Clin 2021;71:209-49. [Crossref] [PubMed]
- Thandra KC, Barsouk A, Saginala K, et al. Epidemiology of lung cancer. Contemp Oncol (Pozn) 2021;25:45-52. [Crossref] [PubMed]
- Welfare AIoHa. Cancer in Australia 2021. Available online: https://www.aihw.gov.au/reports/cancer/cancer-in-australia-2021/summary
- Kalinke L, Thakrar R, Janes SM. The promises and challenges of early non-small cell lung cancer detection: patient perceptions, low-dose CT screening, bronchoscopy and biomarkers. Mol Oncol 2021;15:2544-64. [Crossref] [PubMed]
- Strimbu K, Tavel JA. What are biomarkers? Curr Opin HIV AIDS 2010;5:463-6. [Crossref] [PubMed]
- Henry NL, Hayes DF. Cancer biomarkers. Mol Oncol 2012;6:140-6. [Crossref] [PubMed]
- Seijo LM, Peled N, Ajona D, et al. Biomarkers in Lung Cancer Screening: Achievements, Promises, and Challenges. J Thorac Oncol 2019;14:343-57. [Crossref] [PubMed]
- Chevallier M, Borgeaud M, Addeo A, et al. Oncogenic driver mutations in non-small cell lung cancer: Past, present and future. World J Clin Oncol 2021;12:217-37. [Crossref] [PubMed]
- Vijayalakshmi R, Krishnamurthy A. Targetable "driver" mutations in non small cell lung cancer. Indian J Surg Oncol 2011;2:178-88. [Crossref] [PubMed]
- John A, Shah RA, Wong WB, et al. Value of Precision Medicine in Advanced Non-Small Cell Lung Cancer: Real-World Outcomes Associated with the Use of Companion Diagnostics. Oncologist 2020;25:e1743-52. [Crossref] [PubMed]
- Dama E, Colangelo T, Fina E, et al. Biomarkers and Lung Cancer Early Detection: State of the Art. Cancers (Basel) 2021;13:3919. [Crossref] [PubMed]
- Ru Zhao Y, Xie X, de Koning HJ, et al. NELSON lung cancer screening study. Cancer Imaging 2011;11 Spec No A:S79-84.
- Aberle DR, Adams AM, et al. Reduced lung-cancer mortality with low-dose computed tomographic screening. N Engl J Med 2011;365:395-409. [Crossref] [PubMed]
- Brenner DJ, Hall EJ. Computed tomography--an increasing source of radiation exposure. N Engl J Med 2007;357:2277-84. [Crossref] [PubMed]
- Ten Haaf K, Jeon J, Tammemägi MC, et al. Risk prediction models for selection of lung cancer screening candidates: A retrospective validation study. PLoS Med 2017;14:e1002277. [Crossref] [PubMed]
- Mamdani H, Ahmed S, Armstrong S, et al. Blood-based tumor biomarkers in lung cancer for detection and treatment. Transl Lung Cancer Res 2017;6:648-60. [Crossref] [PubMed]
- Lone SN, Nisar S, Masoodi T, et al. Liquid biopsy: a step closer to transform diagnosis, prognosis and future of cancer treatments. Mol Cancer 2022;21:79. [Crossref] [PubMed]
- Atwater T, Cook CM, Massion PP. The Pursuit of Noninvasive Diagnosis of Lung Cancer. Semin Respir Crit Care Med 2016;37:670-80. [Crossref] [PubMed]
- Barta JA, Powell CA, Wisnivesky JP. Global Epidemiology of Lung Cancer. Ann Glob Health 2019;85:8. [Crossref] [PubMed]
- Kerpel-Fronius A, Tammemägi M, Cavic M, et al. Screening for Lung Cancer in Individuals Who Never Smoked: An International Association for the Study of Lung Cancer Early Detection and Screening Committee Report. J Thorac Oncol 2022;17:56-66. [Crossref] [PubMed]
- Cheng ES, Weber MF, Steinberg J, et al. Evaluating risk factors for lung cancer among never-smoking individuals using two Australian studies. J Cancer Res Clin Oncol 2022;148:2827-40. [Crossref] [PubMed]
- Rivera GA, Wakelee H. Lung Cancer in Never Smokers. Adv Exp Med Biol 2016;893:43-57. [Crossref] [PubMed]
- Zhang T, Joubert P, Ansari-Pour N, et al. Genomic and evolutionary classification of lung cancer in never smokers. Nat Genet 2021;53:1348-59. [Crossref] [PubMed]
- Samet JM, Avila-Tang E, Boffetta P, et al. Lung cancer in never smokers: clinical epidemiology and environmental risk factors. Clin Cancer Res 2009;15:5626-45. [Crossref] [PubMed]
- Spyratos D, Zarogoulidis P, Porpodis K, et al. Occupational exposure and lung cancer. J Thorac Dis 2013;5:S440-5. [Crossref] [PubMed]
- Corrales L, Rosell R, Cardona AF, et al. Lung cancer in never smokers: The role of different risk factors other than tobacco smoking. Crit Rev Oncol Hematol 2020;148:102895. [Crossref] [PubMed]
- Chiou YH, Liou SH, Wong RH, et al. Nickel may contribute to EGFR mutation and synergistically promotes tumor invasion in EGFR-mutated lung cancer via nickel-induced microRNA-21 expression. Toxicol Lett 2015;237:46-54. [Crossref] [PubMed]
- Martin-Gisbert L, Ruano-Ravina A, Varela-Lema L, et al. Lung cancer mortality attributable to residential radon: a systematic scoping review. J Expo Sci Environ Epidemiol 2023;33:368-76. [Crossref] [PubMed]
- Rizo-Maestre C, Echarri-Iribarren V. Radon Gas in the City of Alicante. High Risk of Low Indoor Air Quality in Poorly Ventilated Buildings. Int J Environ Res Public Health 2020;17:8762. [Crossref] [PubMed]
- Casal-Mouriño A, Valdés L, Barros-Dios JM, et al. Lung cancer survival among never smokers. Cancer Lett 2019;451:142-9. [Crossref] [PubMed]
- Dubin S, Griffin D. Lung Cancer in Non-Smokers. Mo Med 2020;117:375-9.
- de Groot PM, Wu CC, Carter BW, et al. The epidemiology of lung cancer. Transl Lung Cancer Res 2018;7:220-33. [Crossref] [PubMed]
- Govindan R, Ding L, Griffith M, et al. Genomic landscape of non-small cell lung cancer in smokers and never-smokers. Cell 2012;150:1121-34. [Crossref] [PubMed]
- Karlsson A, Ringnér M, Lauss M, et al. Genomic and transcriptional alterations in lung adenocarcinoma in relation to smoking history. Clin Cancer Res 2014;20:4912-24. [Crossref] [PubMed]
- Carozzi FM, Bisanzi S, Carrozzi L, et al. Multimodal lung cancer screening using the ITALUNG biomarker panel and low dose computed tomography. Results of the ITALUNG biomarker study. Int J Cancer 2017;141:94-101. [Crossref] [PubMed]
- Hubers AJ, Heideman DA, Duin S, et al. DNA hypermethylation analysis in sputum of asymptomatic subjects at risk for lung cancer participating in the NELSON trial: argument for maximum screening interval of 2 years. J Clin Pathol 2017;70:250-4. [Crossref] [PubMed]
- Alivernini S, Gremese E, McSharry C, et al. MicroRNA-155-at the Critical Interface of Innate and Adaptive Immunity in Arthritis. Front Immunol 2018;8:1932. [Crossref] [PubMed]
- Geng Q, Fan T, Zhang B, et al. Five microRNAs in plasma as novel biomarkers for screening of early-stage non-small cell lung cancer. Respir Res 2014;15:149. [Crossref] [PubMed]
- Shao C, Yang F, Qin Z, et al. The value of miR-155 as a biomarker for the diagnosis and prognosis of lung cancer: a systematic review with meta-analysis. BMC Cancer 2019;19:1103. [Crossref] [PubMed]
- Van Roosbroeck K, Fanini F, Setoyama T, et al. Combining Anti-Mir-155 with Chemotherapy for the Treatment of Lung Cancers. Clin Cancer Res 2017;23:2891-904. [Crossref] [PubMed]
- Zang YS, Zhong YF, Fang Z, et al. MiR-155 inhibits the sensitivity of lung cancer cells to cisplatin via negative regulation of Apaf-1 expression. Cancer Gene Ther 2012;19:773-8. [Crossref] [PubMed]
- Sozzi G, Boeri M, Rossi M, et al. Clinical utility of a plasma-based miRNA signature classifier within computed tomography lung cancer screening: a correlative MILD trial study. J Clin Oncol 2014;32:768-73. [Crossref] [PubMed]
- Bianchi F, Nicassio F, Marzi M, et al. A serum circulating miRNA diagnostic test to identify asymptomatic high-risk individuals with early stage lung cancer. EMBO Mol Med 2011;3:495-503. [Crossref] [PubMed]
- Montani F, Marzi MJ, Dezi F, et al. miR-Test: a blood test for lung cancer early detection. J Natl Cancer Inst 2015;107:djv063. [Crossref] [PubMed]
- Boeri M, Verri C, Conte D, et al. MicroRNA signatures in tissues and plasma predict development and prognosis of computed tomography detected lung cancer. Proc Natl Acad Sci U S A 2011;108:3713-8. [Crossref] [PubMed]
- Joseph S, Harrington R, Walter D, et al. Plasma osteopontin velocity differentiates lung cancers from controls in a CT screening population. Cancer Biomark 2012;12:177-84. [Crossref] [PubMed]
- Gu J, Xu S, Huang L, et al. Value of combining serum carcinoembryonic antigen and PET/CT in predicting EGFR mutation in non-small cell lung cancer. J Thorac Dis 2018;10:723-31. [Crossref] [PubMed]
- Gao Y, Song P, Li H, et al. Elevated serum CEA levels are associated with the explosive progression of lung adenocarcinoma harboring EGFR mutations. BMC Cancer 2017;17:484. [Crossref] [PubMed]
- Yang D, Zhang X, Powell CA, et al. Probability of cancer in high-risk patients predicted by the protein-based lung cancer biomarker panel in China: LCBP study. Cancer 2018;124:262-70. [Crossref] [PubMed]
- Triphuridet N, Vidhyarkorn S, Worakitsitisatorn A, et al. Screening values of carcinoembryonic antigen and cytokeratin 19 fragment for lung cancer in combination with low-dose computed tomography in high-risk populations: Initial and 2-year screening outcomes. Lung Cancer 2018;122:243-8. [Crossref] [PubMed]
- Ajona D, Pajares MJ, Corrales L, et al. Investigation of complement activation product c4d as a diagnostic and prognostic biomarker for lung cancer. J Natl Cancer Inst 2013;105:1385-93. [Crossref] [PubMed]
- Ajona D, Okrój M, Pajares MJ, et al. Complement C4d-specific antibodies for the diagnosis of lung cancer. Oncotarget 2018;9:6346-55. [Crossref] [PubMed]
- Ajona D, Remirez A, Sainz C, et al. A model based on the quantification of complement C4c, CYFRA 21-1 and CRP exhibits high specificity for the early diagnosis of lung cancer. Transl Res 2021;233:77-91. [Crossref] [PubMed]
- Li M, Zhang C, Deng S, et al. Lung cancer-associated T cell repertoire as potential biomarker for early detection of stage I lung cancer. Lung Cancer 2021;162:16-22. [Crossref] [PubMed]
- Sullivan FM, Mair FS, Anderson W, et al. Earlier diagnosis of lung cancer in a randomised trial of an autoantibody blood test followed by imaging. Eur Respir J 2021;57:2000670. [Crossref] [PubMed]
- Li JZ, Lai YY, Sun JY, et al. Metabolic profiles of serum samples from ground glass opacity represent potential diagnostic biomarkers for lung cancer. Transl Lung Cancer Res 2019;8:489-99. [Crossref] [PubMed]
- Fahrmann JF, Grapov D, DeFelice BC, et al. Serum phosphatidylethanolamine levels distinguish benign from malignant solitary pulmonary nodules and represent a potential diagnostic biomarker for lung cancer. Cancer Biomark 2016;16:609-17. [Crossref] [PubMed]
- Roś-Mazurczyk M, Wojakowska A, Marczak Ł, et al. Panel of serum metabolites discriminates cancer patients and healthy participants of lung cancer screening - a pilot study. Acta Biochim Pol 2017;64:513-8. [Crossref] [PubMed]
- Noreldeen HAA, Du L, Li W, et al. Serum lipidomic biomarkers for non-small cell lung cancer in nonsmoking female patients. J Pharm Biomed Anal 2020;185:113220. [Crossref] [PubMed]
- He Y, Shi J, Schmidt B, et al. Circulating Tumor Cells as a Biomarker to Assist Molecular Diagnosis for Early Stage Non-Small Cell Lung Cancer. Cancer Manag Res 2020;12:841-54. [Crossref] [PubMed]
- Marquette CH, Boutros J, Benzaquen J, et al. Circulating tumour cells as a potential biomarker for lung cancer screening: a prospective cohort study. Lancet Respir Med 2020;8:709-16. [Crossref] [PubMed]
- Sanchez-Salcedo P, de-Torres JP, Martinez-Urbistondo D, et al. The neutrophil to lymphocyte and platelet to lymphocyte ratios as biomarkers for lung cancer development. Lung Cancer 2016;97:28-34. [Crossref] [PubMed]
- Tian T, Lu J, Zhao W, et al. Associations of systemic inflammation markers with identification of pulmonary nodule and incident lung cancer in Chinese population. Cancer Med 2022;11:2482-91. [Crossref] [PubMed]
- Wadowska K, Bil-Lula I, Trembecki Ł, et al. Genetic Markers in Lung Cancer Diagnosis: A Review. Int J Mol Sci 2020;21:4569. [Crossref] [PubMed]
- Roland M, Rudd RM. Genetics and pulmonary medicine. 7. Somatic mutations in the development of lung cancer. Thorax 1998;53:979-83. [Crossref] [PubMed]
- Yamamoto H, Yatabe Y, Toyooka S. Inherited lung cancer syndromes targeting never smokers. Transl Lung Cancer Res 2018;7:498-504. [Crossref] [PubMed]
- Vogelstein B, Kinzler KW. Digital PCR. Proc Natl Acad Sci U S A 1999;96:9236-41. [Crossref] [PubMed]
- Leary RJ, Kinde I, Diehl F, et al. Development of personalized tumor biomarkers using massively parallel sequencing. Sci Transl Med 2010;2:20ra14. [Crossref] [PubMed]
- Czarnecka KH, Migdalska-Sęk M, Antczak A, et al. Allelic imbalance in 1p, 7q, 9p, 11p, 12q and 16q regions in non-small cell lung carcinoma and its clinical association: a pilot study. Mol Biol Rep 2013;40:6671-84. [Crossref] [PubMed]
- Xia S, Huang CC, Le M, et al. Genomic variations in plasma cell free DNA differentiate early stage lung cancers from normal controls. Lung Cancer 2015;90:78-84. [Crossref] [PubMed]
- Liloglou T, Maloney P, Xinarianos G, et al. Sensitivity and limitations of high throughput fluorescent microsatellite analysis for the detection of allelic imbalance: application in lung tumors. Int J Oncol 2000;16:5-14. [Crossref] [PubMed]
- Esposito A, Criscitiello C, Trapani D, et al. The Emerging Role of "Liquid Biopsies," Circulating Tumor Cells, and Circulating Cell-Free Tumor DNA in Lung Cancer Diagnosis and Identification of Resistance Mutations. Curr Oncol Rep 2017;19:1. [Crossref] [PubMed]
- Mouliere F, Robert B, Arnau Peyrotte E, et al. High fragmentation characterizes tumour-derived circulating DNA. PLoS One 2011;6:e23418. [Crossref] [PubMed]
- Snyder MW, Kircher M, Hill AJ, et al. Cell-free DNA Comprises an In Vivo Nucleosome Footprint that Informs Its Tissues-Of-Origin. Cell 2016;164:57-68. [Crossref] [PubMed]
- Thierry AR. Circulating DNA fragmentomics and cancer screening. Cell Genom 2023;3:100242. [Crossref] [PubMed]
- Wang S, Meng F, Li M, et al. Multidimensional Cell-Free DNA Fragmentomic Assay for Detection of Early-Stage Lung Cancer. Am J Respir Crit Care Med 2023;207:1203-13. [Crossref] [PubMed]
- Hackshaw A, Clarke CA, Hartman AR. New genomic technologies for multi-cancer early detection: Rethinking the scope of cancer screening. Cancer Cell 2022;40:109-13. [Crossref] [PubMed]
- Lennon AM, Buchanan AH, Kinde I, et al. Feasibility of blood testing combined with PET-CT to screen for cancer and guide intervention. Science 2020;369:eabb9601. [Crossref] [PubMed]
- Calabrese F, Lunardi F, Pezzuto F, et al. Are There New Biomarkers in Tissue and Liquid Biopsies for the Early Detection of Non-Small Cell Lung Cancer? J Clin Med 2019;8:414. [Crossref] [PubMed]
- Ehrlich M. DNA hypermethylation in disease: mechanisms and clinical relevance. Epigenetics 2019;14:1141-63. [Crossref] [PubMed]
- Ehrlich M. DNA hypomethylation in cancer cells. Epigenomics 2009;1:239-59. [Crossref] [PubMed]
- Chen X, Gole J, Gore A, et al. Non-invasive early detection of cancer four years before conventional diagnosis using a blood test. Nat Commun 2020;11:3475. [Crossref] [PubMed]
- Alegría-Torres JA, Barretta F, Batres-Esquivel LE, et al. Epigenetic markers of exposure to polycyclic aromatic hydrocarbons in Mexican brickmakers: a pilot study. Chemosphere 2013;91:475-80. [Crossref] [PubMed]
- Bind MA, Baccarelli A, Zanobetti A, et al. Air pollution and markers of coagulation, inflammation, and endothelial function: associations and epigene-environment interactions in an elderly cohort. Epidemiology 2012;23:332-40. [Crossref] [PubMed]
- Tarantini L, Bonzini M, Tripodi A, et al. Blood hypomethylation of inflammatory genes mediates the effects of metal-rich airborne pollutants on blood coagulation. Occup Environ Med 2013;70:418-25. [Crossref] [PubMed]
- Cheishvili D, Parashar S, Mahmood N, et al. Identification of an Epigenetic Signature of Osteoporosis in Blood DNA of Postmenopausal Women. J Bone Miner Res 2018;33:1980-9. [Crossref] [PubMed]
- Wahl S, Drong A, Lehne B, et al. Epigenome-wide association study of body mass index, and the adverse outcomes of adiposity. Nature 2017;541:81-6. [Crossref] [PubMed]
- Chen X, Yan F, Lin X, et al. DNA Methylation in Chronic Obstructive Pulmonary Disease. Adv Exp Med Biol 2020;1255:83-98. [Crossref] [PubMed]
- Nilsson E, Ling C. DNA methylation links genetics, fetal environment, and an unhealthy lifestyle to the development of type 2 diabetes. Clin Epigenetics 2017;9:105. [Crossref] [PubMed]
- Esquela-Kerscher A, Slack FJ. Oncomirs - microRNAs with a role in cancer. Nat Rev Cancer 2006;6:259-69. [Crossref] [PubMed]
- Orozco AF, Lewis DE. Flow cytometric analysis of circulating microparticles in plasma. Cytometry A 2010;77:502-14. [Crossref] [PubMed]
- O'Driscoll L, Kenny E, Mehta JP, et al. Feasibility and relevance of global expression profiling of gene transcripts in serum from breast cancer patients using whole genome microarrays and quantitative RT-PCR. Cancer Genomics Proteomics 2008;5:94-104.
- Cortez MA, Bueso-Ramos C, Ferdin J, et al. MicroRNAs in body fluids--the mix of hormones and biomarkers. Nat Rev Clin Oncol 2011;8:467-77. [Crossref] [PubMed]
- Ng EK, Chong WW, Jin H, et al. Differential expression of microRNAs in plasma of patients with colorectal cancer: a potential marker for colorectal cancer screening. Gut 2009;58:1375-81. [Crossref] [PubMed]
- Hu Z, Chen X, Zhao Y, et al. Serum microRNA signatures identified in a genome-wide serum microRNA expression profiling predict survival of non-small-cell lung cancer. J Clin Oncol 2010;28:1721-6. [Crossref] [PubMed]
- Mitchell PS, Parkin RK, Kroh EM, et al. Circulating microRNAs as stable blood-based markers for cancer detection. Proc Natl Acad Sci U S A 2008;105:10513-8. [Crossref] [PubMed]
- Zamay TN, Zamay GS, Kolovskaya OS, et al. Current and Prospective Protein Biomarkers of Lung Cancer. Cancers (Basel) 2017;9:155. [Crossref] [PubMed]
- Zhang H, Cao J, Li L, et al. Identification of urine protein biomarkers with the potential for early detection of lung cancer. Sci Rep 2015;5:11805. [Crossref] [PubMed]
- Huang H, Yang Y, Zhu Y, et al. Blood protein biomarkers in lung cancer. Cancer Lett 2022;551:215886. [Crossref] [PubMed]
- Spratlin JL, Serkova NJ, Eckhardt SG. Clinical applications of metabolomics in oncology: a review. Clin Cancer Res 2009;15:431-40. [Crossref] [PubMed]
- Hu Z, Lin D, Yuan J, et al. Overexpression of osteopontin is associated with more aggressive phenotypes in human non-small cell lung cancer. Clin Cancer Res 2005;11:4646-52. [Crossref] [PubMed]
- Blasberg JD, Pass HI, Goparaju CM, et al. Reduction of elevated plasma osteopontin levels with resection of non-small-cell lung cancer. J Clin Oncol 2010;28:936-41. [Crossref] [PubMed]
- Tang H, Chen J, Han X, et al. Upregulation of SPP1 Is a Marker for Poor Lung Cancer Prognosis and Contributes to Cancer Progression and Cisplatin Resistance. Front Cell Dev Biol 2021;9:646390. [Crossref] [PubMed]
- Zhang W, Fan J, Chen Q, et al. SPP1 and AGER as potential prognostic biomarkers for lung adenocarcinoma. Oncol Lett 2018;15:7028-36. [Crossref] [PubMed]
- Zheng Y, Hao S, Xiang C, et al. The Correlation Between SPP1 and Immune Escape of EGFR Mutant Lung Adenocarcinoma Was Explored by Bioinformatics Analysis. Front Oncol 2021;11:592854. [Crossref] [PubMed]
- O'Regan A. The role of osteopontin in lung disease. Cytokine Growth Factor Rev 2003;14:479-88. [Crossref] [PubMed]
- Ashkar S, Weber GF, Panoutsakopoulou V, et al. Eta-1 (osteopontin): an early component of type-1 (cell-mediated) immunity. Science 2000;287:860-4. [Crossref] [PubMed]
- Liaw L, Birk DE, Ballas CB, et al. Altered wound healing in mice lacking a functional osteopontin gene (spp1). J Clin Invest 1998;101:1468-78. [Crossref] [PubMed]
- Bellahcène A, Castronovo V, Ogbureke KU, et al. Small integrin-binding ligand N-linked glycoproteins (SIBLINGs): multifunctional proteins in cancer. Nat Rev Cancer 2008;8:212-26. [Crossref] [PubMed]
- Grunnet M, Sorensen JB. Carcinoembryonic antigen (CEA) as tumor marker in lung cancer. Lung Cancer 2012;76:138-43. [Crossref] [PubMed]
- Autsavapromporn N, Klunklin P, Chitapanarux I, et al. A Potential Serum Biomarker for Screening Lung Cancer Risk in High Level Environmental Radon Areas: A Pilot Study. Life (Basel) 2021;11:1273. [Crossref] [PubMed]
- Liu J, Zhao YQ, Han X, et al. Correlation between pre-treatment serum carcinoembryonic antigen levels and genotypes in a large population of Chinese people with advanced lung adenocarcinoma. Intern Med J 2019;49:634-43. [Crossref] [PubMed]
- Molina R, Agusti C, Filella X, et al. Study of a new tumor marker, CYFRA 21-1, in malignant and nonmalignant diseases. Tumour Biol 1994;15:318-25. [Crossref] [PubMed]
- Sertić Milić H, Franjević A, Bubanović G, et al. Size, edge, and stage of NSCLC determine the release of CYFRA 21-1 in bloodstream. Wien Klin Wochenschr 2015;127:465-71. [Crossref] [PubMed]
- Abbas M, Kassim SA, Habib M, et al. Clinical Evaluation of Serum Tumor Markers in Patients With Advanced-Stage Non-Small Cell Lung Cancer Treated With Palliative Chemotherapy in China. Front Oncol 2020;10:800. [Crossref] [PubMed]
- Sone K, Oguri T, Ito K, et al. Predictive Role of CYFRA21-1 and CEA for Subsequent Docetaxel in Non-small Cell Lung Cancer Patients. Anticancer Res 2017;37:5125-31. [Crossref] [PubMed]
- Dall'Olio FG, Abbati F, Facchinetti F, et al. CEA and CYFRA 21-1 as prognostic biomarker and as a tool for treatment monitoring in advanced NSCLC treated with immune checkpoint inhibitors. Ther Adv Med Oncol 2020;12:1758835920952994. [Crossref] [PubMed]
- Pio R, Corrales L, Lambris JD, editors. The Role of Complement in Tumor Growth. Tumor Microenvironment and Cellular Stress; 2014. New York, NY: Springer New York, 2014.
- Law SK, Dodds AW. The internal thioester and the covalent binding properties of the complement proteins C3 and C4. Protein Sci 1997;6:263-74. [Crossref] [PubMed]
- Liu YY, Yang QF, Yang JS, et al. Characteristics and prognostic significance of profiling the peripheral blood T-cell receptor repertoire in patients with advanced lung cancer. Int J Cancer 2019;145:1423-31. [Crossref] [PubMed]
- Li Y, Karjalainen A, Koskinen H, et al. p53 autoantibodies predict subsequent development of cancer. Int J Cancer 2005;114:157-60. [Crossref] [PubMed]
- Robertson J, Chapman C, Cheung KL, et al. Autoantibodies in early breast cancer. J Clin Oncol 2005;23:549.
- Qin J, Zeng N, Yang T, et al. Diagnostic Value of Autoantibodies in Lung Cancer: a Systematic Review and Meta-Analysis. Cell Physiol Biochem 2018;51:2631-46. [Crossref] [PubMed]
- Zaenker P, Gray ES, Ziman MR. Autoantibody Production in Cancer--The Humoral Immune Response toward Autologous Antigens in Cancer Patients. Autoimmun Rev 2016;15:477-83. [Crossref] [PubMed]
- Deja S, Porebska I, Kowal A, et al. Metabolomics provide new insights on lung cancer staging and discrimination from chronic obstructive pulmonary disease. J Pharm Biomed Anal 2014;100:369-80. [Crossref] [PubMed]
- Rocha CM, Carrola J, Barros AS, et al. Metabolic signatures of lung cancer in biofluids: NMR-based metabonomics of blood plasma. J Proteome Res 2011;10:4314-24. [Crossref] [PubMed]
- Guo Y, Wang X, Qiu L, et al. Probing gender-specific lipid metabolites and diagnostic biomarkers for lung cancer using Fourier transform ion cyclotron resonance mass spectrometry. Clin Chim Acta 2012;414:135-41. [Crossref] [PubMed]
- Hori S, Nishiumi S, Kobayashi K, et al. A metabolomic approach to lung cancer. Lung Cancer 2011;74:284-92. [Crossref] [PubMed]
- Santos CR, Schulze A. Lipid metabolism in cancer. FEBS J 2012;279:2610-23. [Crossref] [PubMed]
- Klupczynska A, Plewa S, Kasprzyk M, et al. Serum lipidome screening in patients with stage I non-small cell lung cancer. Clin Exp Med 2019;19:505-13. [Crossref] [PubMed]
- Chen Y, Ma Z, Shen X, et al. Serum Lipidomics Profiling to Identify Biomarkers for Non-Small Cell Lung Cancer. Biomed Res Int 2018;2018:5276240. [Crossref] [PubMed]
- Nøst TH, Alcala K, Urbarova I, et al. Systemic inflammation markers and cancer incidence in the UK Biobank. Eur J Epidemiol 2021;36:841-8. [Crossref] [PubMed]
- Fiorelli A, Accardo M, Carelli E, et al. Circulating Tumor Cells in Diagnosing Lung Cancer: Clinical and Morphologic Analysis. Ann Thorac Surg 2015;99:1899-905. [Crossref] [PubMed]
- Yu N, Zhou J, Cui F, et al. Circulating tumor cells in lung cancer: detection methods and clinical applications. Lung 2015;193:157-71. [Crossref] [PubMed]
- Zhang W, Xia W, Lv Z, et al. Liquid Biopsy for Cancer: Circulating Tumor Cells, Circulating Free DNA or Exosomes? Cell Physiol Biochem 2017;41:755-68. [Crossref] [PubMed]
- Hanssen A, Loges S, Pantel K, et al. Detection of Circulating Tumor Cells in Non-Small Cell Lung Cancer. Front Oncol 2015;5:207. [Crossref] [PubMed]
- Zhang Y, Tang Y, Sun S, et al. Single-cell codetection of metabolic activity, intracellular functional proteins, and genetic mutations from rare circulating tumor cells. Anal Chem 2015;87:9761-8. [Crossref] [PubMed]
- Vona G, Sabile A, Louha M, et al. Isolation by size of epithelial tumor cells: a new method for the immunomorphological and molecular characterization of circulatingtumor cells. Am J Pathol 2000;156:57-63. [Crossref] [PubMed]
- Ferreira MM, Ramani VC, Jeffrey SS. Circulating tumor cell technologies. Mol Oncol 2016;10:374-94. [Crossref] [PubMed]
- Gonzalez J, Marín M, Sánchez-Salcedo P, et al. Lung cancer screening in patients with chronic obstructive pulmonary disease. Ann Transl Med 2016;4:160. [Crossref] [PubMed]
- Maldonado F, Bartholmai BJ, Swensen SJ, et al. Are airflow obstruction and radiographic evidence of emphysema risk factors for lung cancer? A nested case-control study using quantitative emphysema analysis. Chest 2010;138:1295-302. [Crossref] [PubMed]
- Theil G, Boehm C, Fischer K, et al. In vivo isolation of circulating tumor cells in patients with different stages of prostate cancer. Oncol Lett 2021;21:357. [Crossref] [PubMed]
- Costa C, Dávila-Ibáñez AB. Methodology for the Isolation and Analysis of CTCs. Adv Exp Med Biol 2020;1220:45-59. [Crossref] [PubMed]
- Coussens LM, Werb Z. Inflammation and cancer. Nature 2002;420:860-7. [Crossref] [PubMed]
- Elinav E, Nowarski R, Thaiss CA, et al. Inflammation-induced cancer: crosstalk between tumours, immune cells and microorganisms. Nat Rev Cancer 2013;13:759-71. [Crossref] [PubMed]
- Grivennikov SI, Greten FR, Karin M. Immunity, inflammation, and cancer. Cell 2010;140:883-99. [Crossref] [PubMed]
- Powell DR, Huttenlocher A. Neutrophils in the Tumor Microenvironment. Trends Immunol 2016;37:41-52. [Crossref] [PubMed]
- Contursi A, Grande R, Dovizio M, et al. Platelets in cancer development and diagnosis. Biochem Soc Trans 2018;46:1517-27. [Crossref] [PubMed]
- Fogar P, Sperti C, Basso D, et al. Decreased total lymphocyte counts in pancreatic cancer: an index of adverse outcome. Pancreas 2006;32:22-8. [Crossref] [PubMed]
- Grieshober L, Graw S, Barnett MJ, et al. Pre-diagnosis neutrophil-to-lymphocyte ratio and mortality in individuals who develop lung cancer. Cancer Causes Control 2021;32:1227-36. [Crossref] [PubMed]
- Kang J, Chang Y, Ahn J, et al. Neutrophil-to-lymphocyte ratio and risk of lung cancer mortality in a low-risk population: A cohort study. Int J Cancer 2019;145:3267-75. [Crossref] [PubMed]
- Li J, Chen Q, Luo X, et al. Neutrophil-to-Lymphocyte Ratio Positively Correlates to Age in Healthy Population. J Clin Lab Anal 2015;29:437-43. [Crossref] [PubMed]
- Thomsen M, Ingebrigtsen TS, Marott JL, et al. Inflammatory biomarkers and exacerbations in chronic obstructive pulmonary disease. JAMA 2013;309:2353-61. [Crossref] [PubMed]
- Gan WQ, Man SF, Senthilselvan A, et al. Association between chronic obstructive pulmonary disease and systemic inflammation: a systematic review and a meta-analysis. Thorax 2004;59:574-80. [Crossref] [PubMed]
- Hariton E, Locascio JJ. Randomised controlled trials - the gold standard for effectiveness research: Study design: randomised controlled trials. BJOG 2018;125:1716. [Crossref] [PubMed]
- Moons KG, Kengne AP, Grobbee DE, et al. Risk prediction models: II. External validation, model updating, and impact assessment. Heart 2012;98:691-8. [Crossref] [PubMed]
- Strayhorn JM Jr. Virtual controls as an alternative to randomized controlled trials for assessing efficacy of interventions. BMC Med Res Methodol 2021;21:3. [Crossref] [PubMed]
- Kong CY, Sheehan DF, McMahon PM, et al. Combined Biomarker and Computed Tomography Screening Strategies for Lung Cancer: Projections of Health and Economic Tradeoffs in the US Population. MDM Policy Pract 2016;1:2381468316643968. [Crossref] [PubMed]