SLC22A3 that encodes organic cation transporter-3 is associated with prognosis and immunogenicity of human lung squamous cell carcinoma
Highlight box
Key findings
• SLC22A3 gene expression was associated with worse prognoses in lung squamous cell carcinoma (LSCC) patients and intense levels of inflammation in the LSCC tumor microenvironment (TME).
What is known and what is new?
• SLC22A3 has been linked to the prognoses of several types of cancer.
• This study found that high SLC22A3 expression is associated with poor prognosis and positively correlated with immune-related pathways such as inflammatory response and abundance of infiltrating immune cells in the TME.
What is the implication, and what should change now?
• SLC22A3 may become as a biomarker for prognosis in LSCC.
• SLC22A3 may play a role in the development of inflammatory microenvironment in LSCC.
Introduction
For decades, lung cancer has consistently ranked the deadliest cancer globally (1). Lung cancer has two main types: small cell lung cancer (SCLC) and non-small cell lung cancer (NSCLC), which account for 85% and 15% of all cases, respectively (www.cancer.org, accessed on July 24, 2023). Based on the histological characteristics, NSCLC is further classified as lung adenocarcinoma (LUAD), lung squamous cell carcinoma (LSCC), large cell (undifferentiated) carcinoma, and others. LSCC accounts for nearly 20% of all lung cancer (2).
The 5-year relative survival rate of patients with LSCC increased over time but remained poor, reaching only 24.2% in 2020 (3). Although the introduction of targeted therapy in recent years has shown remarkable improvements in survival outcomes for LUAD patients, LSCC patients have seen modest benefits from these advanced therapies, even detrimental (4). This is partly due to the differences in the biology and genetics of the two types of lung cancer. LSCC has a limited number of genetic alterations that can be targeted, such as EGFR, KRAS mutations, and ALK rearrangements, making it challenging to develop effective therapeutic strategies. In addition, although LSCC treatment has revolutionized thanks to immune checkpoint blockade therapy, a sizable portion of LSCC patients is resistant to immune checkpoint inhibitors (5,6). To tackle these challenges in developing therapeutic remedies for LSCC patients, it is essential to gain a deeper understanding of molecular abnormalities underlying LSCC tumors.
SLC22A3 gene encodes organic cation transporter (OCT)-3—a member of the OCT family which transports a range of exogenous and endogenous organic cations, including norepinephrine, dopamine, histamine, and certain drugs, across plasma membranes. OCT-3 is found in many types of tissues throughout the body, including the lung, liver, kidney, small intestine, and others (7,8). Several studies have linked OCT-3 to the prognosis of various cancer types with varying impacts. While on the one hand, overexpression of SLC22A3 was associated with prolonged survival in patients with pancreatic cancer (9) and glioblastoma multiforme (10); on the other hand, colorectal and cervical cancer patients experienced unfavorable outcomes when SLC22A3 was highly expressed (11,12). Furthermore, alteration in SLC22A3 expression has been shown to influence the sensitivity of tumor cells to chemotherapeutic medication in kidney carcinoma, colorectal cancer, and head and neck squamous cell cancer (13-15). These studies implied that SLC22A3 may influence cancer prognosis and treatment responsiveness.
To the best of our knowledge, the impact of SLC22A3 expression on LSCC has not been addressed elsewhere. Therefore, the current work sought to evaluate gene expression, DNA methylation, and clinicopathological characteristics data of the first sample of primary LSCC retrieved from The Cancer Genome Atlas - Lung Squamous Cell Carcinoma (TCGA-LUSC) project, exclusively consisting of LSCC patients. We present this article in accordance with the REMARK reporting checklist (available at https://tlcr.amegroups.com/article/view/10.21037/tlcr-23-334/rc).
Methods
Data processing
TCGA-LUSC (n=504) is one of the largest projects in the TCGA program (The Cancer Genome Atlas, National Cancer Institute, USA), exclusively consists of LSCC patients. We accessed the TCGA-LUSC and retrieved data about gene expression, DNA methylation, and clinicopathological characteristics. First, we established the criteria for a sample to represent a case in our study as the first sample of the primary tumor. Normal tissue, later samples of the primary tumor, or recurrent tumors were not included (Figure 1). Patients were involved in this study regardless of treatment strategy. Then, we extracted raw data from gene expression and DNA methylation.
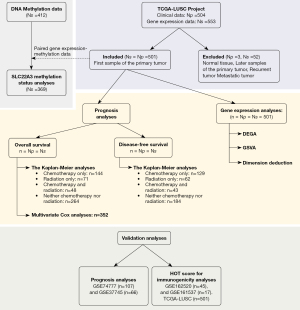
Regarding gene expression data, we applied the following layers of filter: (I) data category of transcriptome profiling; (II) data type of gene expression quantification; and (III) workflow type of STAR—counts. A total of 60,660 read counts within 553 samples comprise the expression matrix. After applying the sample criteria, 501 samples of primary tumors, each of which corresponds to an LSCC case, were included in our analyses.
Regarding DNA methylation data, we used (I) data type of methylation beta value; (II) workflow type of SeSAMe methylation beta estimation; and (III) illumina human methylation 450. A report of methylation status in 450,000 CpG sites within 412 samples was collected. Using the mentioned sample criteria, 369 samples with available DNA methylation information remained.
Finally, we retrieved the clinicopathological parameters, such as age, gender, race, TNM stages, the American Joint Committee on Cancer (AJCC) stages, chemotherapy, radiation therapy, overall survival (OS) time, OS status, disease-free survival (DFS) time, and DFS status. OS time is calculated as the time interval in months from LSCC diagnosis to death, regardless of LSCC or other causes. DFS is the time-period in months between LSCC diagnosis and clinical relapse. The study was conducted in accordance with the Declaration of Helsinki (as revised in 2013).
DNA methylation analysis
We employed the ChAMP package (16) to perform differential methylation position (DMP) analysis. The primary purpose of this analysis section was to examine the methylation status of CpG sites within the SLC22A3 gene, including the gene promoter and body. Therefore, other methylation regions were not accounted for in the present study.
Differentially expressed gene analysis (DEGA) and pathway analysis
In the DEGA pipeline, we initially employed three panels, including nCounter Human PanCancer Pathways (CHPP), nCounter Human PanCancer Immune Profile (CHIP), and nCounter Human PanCancer Progression (CHPPr). Such panels consist of 770, 772, and 770 genes of interest, among which there are 40, 40, and 30 internal reference genes, respectively. These reference genes were discarded before further analyses. Cancer-related genes involved in 13 classic cancer pathways comprise the CHPP panel, while CHIP includes genes from different immune cell types, common checkpoint inhibitors, CT antigens, and adaptive and innate immune responses. Conversely, the CHPPr panel consists of genes related to angiogenesis, extracellular matrix remodeling, and epithelial-to-mesenchymal transition (EMT). CHPP, CHIP, and CHPPr theoretically provide insights about cancer pathways, tumor immunity, and progression landscapes of LSCC. We employed the DESeq2 pipeline (17) to perform DEGA and explore the differential expressed genes in the three panels separately.
For the pathway analysis, we used gene set variation analysis (GSVA) (18) to investigate the enrichment score (ES) of all the pathways included in The Molecular Signatures Database (MSigDB) Hallmark Gene Set Collection (19). ES of a pathway indicates its activity within each sample. GSVA is a variant of single-sample gene set enrichment analysis (GSEA) (20). We aimed to focus on (I) different biological processes, including signaling, proliferation, pathway, metabolic, immune, DNA damage, development, cellular components, and others (21) and (II) cell type-specific signals (22), which describe the tumor microenvironment (TME). The pathways of the aforementioned biological processes originate from the Hallmark gene set, while the cell-type specific signals are markers from the previous study, which includes 24 different cell types (22).
Validation analyses
We downloaded GSE74777, GSE37745, GSE162520, and GSE161537 from Gene Expression Omnibus (GEO) database and extracted gene expression and clinical data of LSCC cases (n=107, 66, 45, and 17, respectively) to validate the prognosis and immunogenicity significance of SLC22A3 on LSCC.
We conducted Kaplan-Meier OS analyses on GSE74777 and GSE37745 separately and on the combined cohort of these datasets, which were generated using the value-binning technique. Value-binning is a commonly used technique in data analysis that discretizes data into a predefined number (B) of bins, thereby facilitating cross-platform data management (23). We applied a simple value-binning method to categorize SLC22A3 gene expression values into B bins, discretizing expression values from 1 to B. We selected B =107 (corresponding to the sample number of the larger dataset) because each value can be assigned to a gene expression value in both datasets. This process can be deemed non-parametric scaling. Our goal was to remove the “magnitude” effect, which is easily confounded by cross-platform differences, and normalize the expression value, which creates the same range of value in both datasets for further non-parametric analyses. This technique was also performed by another study (24). We used the maximally selected rank statistics (MSRS) technique to determine the survival cutpoint of the SLC22A3 expression in validation data, which is also a non-parametric analysis, to find the most significant prognostic cut-off in each dataset. The cut-off bins of GSE37745 and GSE74777 were 40 and 95, respectively. This result indicated the differences in clinicopathological characteristics of the two examined cohorts. Therefore, we used the corresponding cut-off of each dataset to divide the sample into SLC22A3-high and SLC22A3-low samples.
We validated the immunogenicity impact of SLC22A3 on LSCC by utilizing the Hot Oral Tumor (HOT) score described below. Because the unit of read count was inconsistent between TCGA and validation data, it is impossible to utilize the cut-off of FPKM (fragments per kilobase of exon per million mapped fragments) ≥5 to determine high expression in the validation data. Therefore, we used the MSRS technique to determine the survival cutpoint of the SLC22A3 expression in validation data. Based on the HOT score, samples in the TCGA-LUSC cohort were defined as “hot” or “cold” tumors. SLC22A3 gene expression was compared between the “hot” and “cold” tumor groups in TCGA-LUSC as well as in validation datasets: GSE162520 and GSE161537 [these two datasets were used in the original study on developing HOT score (25)]. Additionally, we conducted OS Kaplan-Meier analyses of SLC22A3-low and SLC22A3-high LSCC samples in the GSE162520 dataset. We excluded GSE161537 in this survival analysis since the patients of this cohort were treated with immunotherapy, which may confound the result.
HOT score
The HOT score was calculated by using GSVA of the 27-gene list in the original study (25), including CCL19, CCR2, CCR4, CCR5, CD27, CD40LG, CD8A, CXCL10, CXCL11, CXCL13, CXCL9, CXCR3, CXCR6, FASLG, FGL2, GZMA, GZMH, IDO1, IFNG, IRF8, LAG3, LYZ, MS4A1, PDCD1, TBX21, TLR7, and TLR8. LSCC tumors with −1< ES <0 or 0< ES <1 were classified as “cold” or “hot” tumors, respectively. The calculation procedure was performed as in the previous study (25).
Statistical analysis
FPKM, a type of normalized read count, was used as the gene expression unit in this study. By applying a cut-off of 5 FPKM, we divided the LSCC patients into two subgroups based on their SLC22A3 expression levels: individuals with high expression (SLC22A3-high) and those with low expression (SLC22A3-low). To assess the prognosis effect of SLC22A3, we employed both non-parametric and parametric analyses, including univariate and multivariate Cox analyses of OS and the Kaplan-Meier curves of OS and DFS rates. The Kaplan-Meier curves compared the OS and DFS rates among patient subgroups categorized by SLC22A3 expression, chemotherapy, and radiation therapy. The Bioconductor packages, including DESeq2, GSVA, and ChAMP, were employed for bioinformatic analyses using R software version 4.2.2 (The R Foundation, Vienna, Austria). Hypothesis tests were considered significant when P<0.05 or adjusted P (if available) <0.05. Samples/patients with related missing values were excluded from the corresponding analysis section.
Results
A total of 17.8% LSCCs possess high expression (FPKM ≥5) of the SLC22A3 gene
We first investigated the distribution of SLC22A3 read counts in the first non-treated samples of primary LSCC using data retrieved from the TCGA-LUSC project (n=501), exclusively consisting of LSCC patients (Figure 2A). The mean, median, and standard deviation of the SLC22A3 expression were 3.3, 1.4, and 6.2, respectively. Choosing the FPKM cut-off to regard low and high gene expression is controversial, depending on the analysis context. Given the range and variation in SLC22A3 expression, we arbitrarily set FPKM ≥5 as a high expression. Using this cut-off, we found 17.8% of SLC22A3-high LSCCs. For comparison, the read count distribution of SLC22A1 and SLC22A2, which encode other members of the OCT family (OCT1 and OCT2, respectively) in the first samples of primary LSCC, was also examined (Figure S1). We found almost no expression of SLC22A1 and SLC22A2 genes in the entire studied cohort.
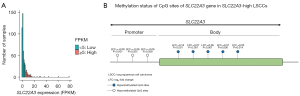
We then asked which mechanism was involved in the differential expressions of the SLC22A3 gene in LSCC. DMP analysis indicated the hypomethylation of the SLC22A3 gene promoter and hypermethylation of the SLC22A3 gene body in SLC22A3-high tumors (Figure 2B). These results implied that the level of SLC22A3 gene expression in LSCC tumors was perhaps partially attributable to the DNA methylation mechanism.
Clinicopathological analysis showed worse prognoses in SLC22A3-high patients of TCGA-LUSC cohort
Table 1 illustrates the difference in clinical characteristics (age, gender, race, stage, treatment) of SLC22A3-low and SLC22A3-high LSCC. Only two different variables, T stage (P=0.004) and chemotherapy (P=0.01), were different between SLC22A3-low and SLC22A3-high LSCC. The SLC22A3-high tumor showed a higher distribution of T1 (33.7% vs. 20.4%), T3 (18.0% vs. 13.3%), and T4 (6.7% vs. 4.1%) stages while the T2 stage is more dominant in SLC22A3-low compared to SLC22A3-high LSCCs (62.1% vs. 41.6%). SLC22A3-high patients (15.7%) received chemotherapy less frequently than SLC22A3-low patients (31.8%).
Table 1
Variables | SLC22A3-low (n=412) | SLC22A3-high (n=89) | P value |
---|---|---|---|
Age (years), median [range] | 69 [39–90] | 67 [40–85] | 0.459 |
Gender, n (%) | 0.521 | ||
Women | 104 (25.2) | 26 (29.2) | |
Men | 308 (74.8) | 63 (70.9) | |
Race, n (%) | 0.073 | ||
Asian | 5 (1.2) | 4 (4.5) | |
Black | 25 (6.1) | 5 (5.6) | |
White | 283 (68.7) | 14 (15.7) | |
Not reported | 99 (24.0) | 66 (74.2) | |
T stage, n (%) | 0.004 | ||
T1 | 84 (20.4) | 30 (33.7) | |
T2 | 256 (62.1) | 37 (41.6) | |
T3 | 55 (13.3) | 16 (18.0) | |
T4 | 17 (4.1) | 6 (6.7) | |
N stage, n (%) | 0.072 | ||
N0 | 257 (62.4) | 62 (69.7) | |
N1 | 116 (28.2) | 15 (16.9) | |
N2 | 32 (7.8) | 8 (9.0) | |
N3 | 4 (1.0) | 1 (1.1) | |
NX | 3 (0.7) | 3 (3.4) | |
M stage, n (%) | 0.668 | ||
M0 | 341 (82.8) | 70 (78.7) | |
M1 | 5 (1.2) | 2 (2.2) | |
MX | 63 (15.3) | 16 (18.0) | |
Not reported | 3 (0.7) | 1 (1.1) | |
AJCC stage, n (%) | 0.498 | ||
I | 197 (47.8) | 47 (52.8) | |
II | 140 (34.0) | 22 (24.7) | |
III | 67 (16.3) | 17 (19.1) | |
IV | 5 (1.2) | 2 (2.2) | |
Not reported | 3 (0.7) | 1 (1.1) | |
Chemotherapy, n (%) | 0.01 | ||
No | 233 (56.6) | 61 (68.5) | |
Yes | 131 (31.8) | 14 (15.7) | |
Not reported | 48 (11.7) | 14 (15.7) | |
Radiation therapy, n (%) | 0.811 | ||
No | 303 (73.5) | 64 (71.9) | |
Yes | 59 (14.3) | 12 (13.5) | |
Not reported | 50 (12.1) | 13 (14.6) | |
Overall survival (years), median [range] | 1.9 [0.0–13.1] | 1.5 [0.0–14.5] | 0.459 |
LSCC, lung squamous cell carcinoma; AJCC, The American Joint Committee on Cancer.
In survival analysis, we performed both non-parametric and parametric analyses. In the non-parametric method, the Kaplan-Meier curves compared the OS and DFS patterns (Figure 3) of patients stratified by SLC22A3 expression, chemotherapy, and radiation therapy. OS curves showed that high expression of SLC22A3 was associated with worse prognoses regardless of chemotherapy and radiation (P<0.05) (Figure 3A-3E). DFS curves also revealed a substantial difference between SLC22A3-high and SLC22A3-low individuals, particularly in groups treated solely with radiation (P<0.001) (Figure 3F-3J). Staging-stratified Kaplan-Meier curves based on the AJCC staging system showed that SLC22A3-high was associated with worse outcomes in stage II and IV patients (Figure 3K-3N).
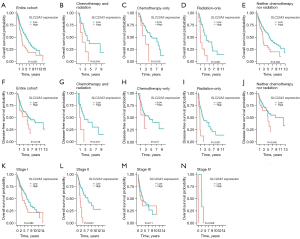
In the parametric method, we performed univariate and multivariate Cox analyses of OS to determine the prognostic effects of SLC22A3 expression (Table 2). Univariate Cox analysis suggested that SLC22A3-high, T3 and T4 of T stage, distant metastatic status, AJCC stage III and IV, and radiation therapy status all had a detrimental impact on LSCC patients’ outcomes (P<0.05). Only significant variables in univariate analysis were included in the subsequent multivariate section. Overall, SLC22A3 expression was a prognostic factor in both univariate [hazard ratio (HR) =1.82; 95% confidence interval (95% CI): 1.32–2.52; P<0.001] and multivariate (HR =2.47; 95% CI: 1.65–3.71; P<0.001) evaluations. Additionally, the 5-year OS rates of patients with SLC22A3-low and SLC22A3-high LSCC were 51.5% (95% CI: 45.4–58.4%) and 22.9% (95% CI: 13.0–40.6%), respectively. The 5-year DFS rates of SLC22A3-low and SLC22A3-high LSCC were 52.6% (95% CI: 45.3–61.0%) and 35.2% (95% CI: 18.4–67.2%). These results demonstrated that SLC22A3-high LSCC had poor prognoses compared to SLC22A3-low LSCC.
Table 2
Variables | Univariate | Multivariate | |||||
---|---|---|---|---|---|---|---|
HR | 95% CI | P value | HR | 95% CI | P value | ||
SLC22A3 expression | |||||||
Low | 1 | 1 | |||||
High | 1.82 | 1.32–2.52 | <0.001 | 2.47 | 1.65–3.71 | <0.001 | |
Age (years) | 1.02 | 1.00–1.03 | 0.046 | 1.03 | 1.01–1.06 | 0.003 | |
Gender | |||||||
Women | 1 | ||||||
Men | 1.2 | 0.87–1.65 | 0.264 | ||||
Race | |||||||
Asian | 1 | ||||||
Black | 0.87 | 0.30–2.59 | 0.808 | ||||
White | 0.568 | 0.21–1.54 | 0.12 | ||||
Not reported | 0.44 | 0.16–1.24 | 0.266 | ||||
T stage | |||||||
T1 | 1 | 1 | |||||
T2 | 1.24 | 0.87–1.75 | 0.232 | 1.17 | 0.76–1.79 | 0.476 | |
T3 | 1.8 | 1.15–2.82 | 0.01 | 1.32 | 0.70–2.49 | 0.389 | |
T4 | 2.31 | 1.24–4.31 | 0.008 | 1.23 | 0.47–3.22 | 0.672 | |
LNM | |||||||
No | 1 | ||||||
Yes | 1.14 | 0.86–1.51 | 0.358 | ||||
Distant metastasis | |||||||
No | 1 | 1 | |||||
Yes | 3.07 | 1.26–7.51 | 0.014 | 4.04 | 1.53–10.70 | 0.005 | |
AJCC stage | |||||||
I | 1 | 1 | |||||
II | 1.12 | 0.82–1.55 | 0.471 | 1.4 | 0.94–2.10 | 0.1 | |
III | 1.54 | 1.08–2.20 | 0.017 | 1.11 | 0.62–1.98 | 0.737 | |
IV | 3.27 | 1.32–8.06 | 0.01 | N/A | N/A | N/A | |
Chemotherapy | |||||||
No | 1 | ||||||
Yes | 0.846 | 0.61–1.17 | 0.309 | ||||
Radiation therapy | |||||||
No | 1 | 1 | |||||
Yes | 1.5 | 1.03–2.10 | 0.032 | 0.37 | 0.23–1.65 | 0.1 |
LSCC, lung squamous cell carcinoma; HR, hazard ratio; 95% CI, 95% confidence interval; LNM, lymph node metastasis; N/A, not applicable.
Dimension reduction analyses showed SLC22A3 expression patterns were associated with tumor pathway, immune, and progression landscapes
We performed t-distributed stochastic neighbor embedding (t-SNE) dimension reduction on CHPP, CHIP, and CHPPr gene matrices to explore the distribution of SLC22A3 expression as both continuous (FPKM) and binary (low/high) variables. In the CHPP panel, we found that SLC22A3-high LSCC tended to locate in a locus belonging to the lower left quarter of the graph rather than being evenly distributed (Figure 4A). Our subjective observation was proven by the correlation analysis between each t-SNE dimension and SLC22A3 expression (P<0.001, Figure 4B, and P=0.003, Figure 4C). The same logic is applied to interpreting the results in the CHIP (Figure 4D-4F) and CHPPr (Figure 4G-4I) panels. These results can be interpreted as a possible link between SLC22A3 expression patterns in LSCC and cancer pathways, cancer-immune interaction, and cancer progression.
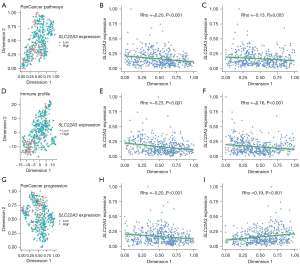
Pathway analyses showed vastly different oncogenic and TME signals in SLC22A3-high LSCCs
We compared the ES between SLC22A3-low and SLC22A3-high LSCCs in the pathway analysis using Hallmark gene sets (Figure 5A) and TME-related analyses (Figure 5B). Figure 5C shows the skewed distribution of SLC22A3-high samples in TME space.
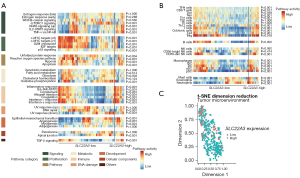
Hallmark gene sets were categorized into eight groups: signaling, proliferation, pathway, metabolic, immune, DNA damage, development, and cellular components, which were used in a previous study (21). SLC22A3-high LSCC were characterized by high activity in immune-related pathways such as inflammatory response, IL6-JAK-STAT3, complement, allograft rejection, coagulation, IFN-α, and IFN-γ response (adjusted P<0.001). Other pathways also exhibited high activity in SLC22A3-high LSCC were KRAS signaling (up), IL2/STAT5, TNFα via NF-κB, EMT, angiogenesis, and TGF-β signaling (adjusted P<0.001). On the other hand, the pathways related to proliferation, such as c-MYC targets, G2M checkpoint, and E2F targets, were downregulated in SLC22A3-high LSCC (adjusted P<0.001).
In TME-related analyses, SLC22A3-high LSCC showed enriched for almost all adaptive immune cells, including B-cells, T-cells, and T-cell subpopulations [T helper 1 (Th1), T gamma delta (Tgd), CD8+ T, T central memory (Tcm), T effector memory (Tem), and T follicular helper (Tfh), regulatory T (Treg) cells], except T helper 2 (Th2) cells. Innate immune cells such as C56-dim natural killer (NK), dendritic cells (DC), macrophages, mast cells, eosinophils, and neutrophils were abundant in SLC22A3-high LSCC. These findings indicated that SLC22A3-high LSCC is associated with high activity of immune-related pathways and an intense inflammatory microenvironment compared to SLC22A3-low LSCC.
DEAs illustrated many significant DEGs between SLC22A3-low and SLC22A3-high tumors
In CHPP, CHIP, and CHPPr, there were numerous differentially expressed genes between SLC22A3-high and SLC22A3-low LSCC. Notably, many genes encoding immune checkpoint molecules were upregulated in the SLC22A3-high LSCC. In particular, in CHIP, SLC22A3-high LSCC expressed higher levels of many immune inhibitory receptors genes, namely: PDCD1, CTLA-4, TIGIT, HAVCR2, and BTLA, which encode programmed cell death protein 1 (PD-1), cytotoxic T-lymphocyte-associated protein 4 (CTLA-4), T-cell immunoreceptor with Ig and immunoreceptor tyrosine-based inhibitory motif (ITIM) domains (TIGIT), T-cell immunoglobulin and mucin domain-containing protein 3 (TIM-3), and B and T lymphocyte attenuator (BTLA), respectively (Table 3). The up-regulation of many genes encoding checkpoint inhibitory molecules in the SLC22A3-high LSCC might be related to the poor prognosis. The full results of DESeq2 analyses are detailed in tables available at https://cdn.amegroups.cn/static/public/tlcr-23-334-1.xlsx.
Table 3
Gene name | LFC | Adjusted P value |
---|---|---|
TNFRSF14 | 0.505 | <0.001 |
PDCD1 | 0.751 | <0.001 |
HAVCR2 | 0.544 | <0.001 |
TIGIT | 0.678 | <0.001 |
CD86 | 0.463 | <0.001 |
CTLA4 | 0.701 | <0.001 |
CD80 | 0.665 | <0.01 |
BTLA | 0.813 | <0.01 |
CD160 | −0.192 | >0.05 |
LAG3 | 0.271 | >0.05 |
CD274 | 0.068 | >0.05 |
LFC, log2 fold change.
Validation on prognostic and immunogenic effects of SLC22A3 gene expression in LSCC
When validating the prognostic significance of SLC22A3 in LSCC patients from GSE37745 and GSE74777 cohorts separately, we observed a tendency towards poorer prognoses in SLC22A3-high patients compared to SLC22A3-low patients, albeit without statistical significance (P=0.078 and 0.07, respectively) (Figure S2A,S2B). These results may be influenced by the relatively limited sample sizes within these cohorts. Therefore, we employed the value-binning technique to merge these two datasets (please refer to the Methods for more details). The combined cohort’s Kaplan-Meier OS curves showed that SLC22A3-high expression was linked to poorer prognoses (P<0.001) (Figure 6A).
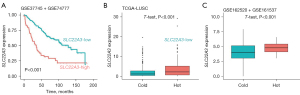
Our study also found that SLC22A3-high tumors were positively correlated with immune-related pathways and an abundance of infiltrating immune cells in the TME. To validate this finding, we utilized the HOT score (25), an interesting metric developed by Foy et al. specifically designed to identify tumors with high immunological activity, suggesting potential benefits from immunotherapies. We found that SLC22A3 expression was consistently higher in “hot” tumors compared to “cold” tumors in both TCGA and validation datasets (Figure 6B,6C). Despite not being statistically significant (P=0.22), the results of OS Kaplan-Meier analyses indicated a trend towards poorer prognosis in SLC22A3-high patients compared to SLC22A3-low patients in GSE162520 dataset (Figure S2C).
Discussion
Our present study found that SLC22A3 expression negatively correlated with OS and DFS rates in LSCC patients. Dimension reduction analysis revealed the link between SLC22A3 expression and cancer pathway, immune landscape, and cancer progression. Pathway analysis showed that, compared to SLC22A3-low, SLC22A3-high LSCC had higher activity in immune-related pathways such as inflammatory response and IL6-JAK-STAT3 and possessed a bustling TME, with numerous innate/adaptive immune cells infiltrating. There were a lot of differentially expressed genes between SLC22A3-high and SLC22A3-low LSCC. Notably, many genes encoding immune checkpoint inhibitory molecules were upregulated in the SLC22A3-high group. Furthermore, the “hot” LSCC tumor phenotype, as classification based on the HOT score, expressed a higher SLC22A3 level. These findings indicated that SLC22A3-high LSCC is associated with poor prognosis and positively correlated with immune-related pathways such as inflammatory response and abundance of infiltrating immune cells in the TME.
SLC22A3-high LSCC was associated with worse outcomes in LSCC patients (Figure 3). This found result is consistent with earlier studies linking SLC22A3 expression to unfavorable outcomes in cervical and colorectal cancer. Notably, multivariate Cox analysis solidified SLC22A3-high as an independent prognostic factor. In addition, DFS curves suggested that SLC22A3-high tumors were particularly resistant to radiotherapy (P<0.001, Figure 3I). Thus, there is a need for more studies to determine the importance of SLC22A3 in the pathophysiology and treatment responses of LSCC.
Hallmark gene sets (Figure 5A) and TME-related analyses (Figure 5B) showed that, in comparison to SLC22A3-low LSCC, SLC22A3-high LSCC is associated with increased activity of immune-related pathways, such as inflammatory response and IL6-JAK-STAT3, and infiltration of almost all innate and adaptive immune cells. These findings suggested that SLC22A3-high LSCC undergoes inflammatory signals from the microenvironment where numerous immune cells are infiltrated. The link between inflammation and cancer has become widely accepted and identified as one of cancer’s ten hallmarks (26). Chronic inflammation is either the underlying mechanism of carcinogenesis or the consequence of genetic alterations in tumor cells (27). In addition, inflammation and lung cancer have been reported to be closely related (28). Inflammation promotes tumor initiation and progression by providing an array of growth and survival factors for tumor cells as well as mediators inducing angiogenesis and the EMT process (27). Of note, angiogenesis and EMT-related pathways activity were also upregulated in SLC22A3-high LSCC, which may contribute to the aggressive behavior of these tumors. Interestingly, Li et al. have demonstrated that knocking down SLC22A3 suppressed LPS-induced inflammation in THP-1 cells (29). Further research is needed to fully uncover the role of SLC22A3 in controlling inflammatory responses, particularly in the tumor context.
On the other hand, the TME-related analyses (Figure 5B) suggested that SLC22A3-high LSCC is likely “hotter” (which means tumors with abundant immune cell infiltration) than SLC22A3-low LSCC, which was further evaluated using the HOT score—a metric that was specifically designed to identify tumors with high immunological activity. This finding may be a bit surprising since hot tumors are generally assumed to have better outcomes, but our results showed that SLC22A3-high LSCC patients had lower OS and DFS rates than SLC22A3-low LSCC patients. This contradiction, perhaps, is brought about by the intricate interaction between tumor cells, infiltrating immune cell networks, and soluble components in the TME. T-cell subpopulations, including CD8+ T-cells, Th1, Tem, Tcm, Tgd, and Tfh, as well as other tumor-infiltrating lymphocytes such as NK cells, B-cells, and DCs, are commonly known to have tumor-protective properties (30-36). However, the presence of these beneficial cells does not guarantee efficient tumor eradication. A broad spectrum of immunosuppressive mechanisms presenting in the TME can impede immune cell function (34,37). These mechanisms, in the context of our study, consist of (I) high expression of multiple immune checkpoint inhibitory molecules; (II) persistence of immunosuppressive cell types such as Tregs; and (III) high activity of the immunosuppressive cytokine TGF-β signaling pathway, which possibly confer SLC22A3-high tumors with immune evasion ability.
Our study is among the first studies investigating the clinicopathological implications of SLC22A3 in LSCC. However, there were several limitations. First, our study has not been validated by in-house experiments. Further in vitro and in vivo studies are needed to examine the causal relationships between biological processes and confirm our observations. Second, the inherent bias of the TCGA database cannot be avoided, including selection bias. For example, most patients are of Caucasian race. Therefore, it is important to extend the population characteristics to other races. Lastly, our study findings are based on gene expression. It is of value to examine the protein expression of SLC22A3. Studies using Western-blot experiments and immunohistochemistry of SLC22A3 are needed to confirm its value at the protein level and how this protein contributes to the clinical landscape of SLC22A3-high LSCC.
Conclusions
This study found that SLC22A3-high LSCC had worse prognoses than SLC22A3-low LSCC. Moreover, SLC22A3-high LSCC was closely associated with high activity in immune-related pathways and a bustling TME infiltrated by immune cells. These findings suggest that high expression of SLC22A3 that encodes OCT3 in LSCC is associated with poor prognosis and immunogenicity of the tumor. Understanding the functional implications of SLC22A3 in LSCC and how it interacts with the immune system may help improve LSCC patient stratification for optimizing immune checkpoint inhibitor therapy treatment, thereby potentially improving outcomes for LSCC patients.
Acknowledgments
Funding: This research was funded by a grant-in-aid for scientific research from
Footnote
Reporting Checklist: The authors have completed the REMARK reporting checklist. Available at https://tlcr.amegroups.com/article/view/10.21037/tlcr-23-334/rc
Peer Review File: Available at https://tlcr.amegroups.com/article/view/10.21037/tlcr-23-334/prf
Conflicts of Interest: All authors have completed the ICMJE uniform disclosure form (available at https://tlcr.amegroups.com/article/view/10.21037/tlcr-23-334/coif). The authors have no conflicts of interest to declare.
Ethical Statement: The authors are accountable for all aspects of the work in ensuring that questions related to the accuracy or integrity of any part of the work are appropriately investigated and resolved. The study was conducted in accordance with the Declaration of Helsinki (as revised in 2013).
Open Access Statement: This is an Open Access article distributed in accordance with the Creative Commons Attribution-NonCommercial-NoDerivs 4.0 International License (CC BY-NC-ND 4.0), which permits the non-commercial replication and distribution of the article with the strict proviso that no changes or edits are made and the original work is properly cited (including links to both the formal publication through the relevant DOI and the license). See: https://creativecommons.org/licenses/by-nc-nd/4.0/.
References
- Sung H, Ferlay J, Siegel RL, et al. Global Cancer Statistics 2020: GLOBOCAN Estimates of Incidence and Mortality Worldwide for 36 Cancers in 185 Countries. CA Cancer J Clin 2021;71:209-49. [Crossref] [PubMed]
- Zheng M. Classification and Pathology of Lung Cancer. Surg Oncol Clin N Am 2016;25:447-68. [Crossref] [PubMed]
- SEER*Explorer: An interactive website for SEER cancer statistics [Internet]. Surveillance Research Program, National Cancer Institute; 2023 Apr 19. [updated: 2023 Jun 8; cited 2023 Oct 12]. Available online: https://seer.cancer.gov/statistics-network/explorer/. Data source(s): SEER Incidence Data, November 2022 Submission (1975-2020), SEER 22 registries.
- Niu Z, Jin R, Zhang Y, et al. Signaling pathways and targeted therapies in lung squamous cell carcinoma: mechanisms and clinical trials. Signal Transduct Target Ther 2022;7:353. [Crossref] [PubMed]
- Reck M, Rodríguez-Abreu D, Robinson AG, et al. Updated Analysis of KEYNOTE-024: Pembrolizumab Versus Platinum-Based Chemotherapy for Advanced Non-Small-Cell Lung Cancer With PD-L1 Tumor Proportion Score of 50% or Greater. J Clin Oncol 2019;37:537-46. [Crossref] [PubMed]
- Sezer A, Kilickap S, Gümüş M, et al. Cemiplimab monotherapy for first-line treatment of advanced non-small-cell lung cancer with PD-L1 of at least 50%: a multicentre, open-label, global, phase 3, randomised, controlled trial. Lancet 2021;397:592-604. [Crossref] [PubMed]
- Ingoglia F, Visigalli R, Rotoli BM, et al. Functional characterization of the organic cation transporters (OCTs) in human airway pulmonary epithelial cells. Biochim Biophys Acta 2015;1848:1563-72. [Crossref] [PubMed]
- Nishimura M, Naito S. Tissue-specific mRNA expression profiles of human ATP-binding cassette and solute carrier transporter superfamilies. Drug Metab Pharmacokinet 2005;20:452-77. [Crossref] [PubMed]
- Mohelnikova-Duchonova B, Brynychova V, Hlavac V, et al. The association between the expression of solute carrier transporters and the prognosis of pancreatic cancer. Cancer Chemother Pharmacol 2013;72:669-82. [Crossref] [PubMed]
- Lian Q, Xiao S, Wang Y, et al. Expression and clinical significance of organic cation transporter family in glioblastoma multiforme. Ir J Med Sci 2022;191:1115-21. [Crossref] [PubMed]
- Huo X, Zhou X, Peng P, et al. Identification of a Six-Gene Signature for Predicting the Overall Survival of Cervical Cancer Patients. Onco Targets Ther 2021;14:809-22. [Crossref] [PubMed]
- Ren A, Sun S, Li S, et al. Genetic variants in SLC22A3 contribute to the susceptibility to colorectal cancer. Int J Cancer 2019;145:154-63. [Crossref] [PubMed]
- Hsu CM, Lin PM, Chang JG, et al. Upregulated SLC22A3 has a potential for improving survival of patients with head and neck squamous cell carcinoma receiving cisplatin treatment. Oncotarget 2017;8:74348-58. [Crossref] [PubMed]
- Gu J, Dong D, Long E, et al. Upregulated OCT3 has the potential to improve the survival of colorectal cancer patients treated with (m)FOLFOX6 adjuvant chemotherapy. Int J Colorectal Dis 2019;34:2151-9. [Crossref] [PubMed]
- Shnitsar V, Eckardt R, Gupta S, et al. Expression of human organic cation transporter 3 in kidney carcinoma cell lines increases chemosensitivity to melphalan, irinotecan, and vincristine. Cancer Res 2009;69:1494-501. [Crossref] [PubMed]
- Morris TJ, Butcher LM, Feber A, et al. ChAMP: 450k Chip Analysis Methylation Pipeline. Bioinformatics 2014;30:428-30. [Crossref] [PubMed]
- Love MI, Huber W, Anders S. Moderated estimation of fold change and dispersion for RNA-seq data with DESeq2. Genome Biol 2014;15:550. [Crossref] [PubMed]
- Hänzelmann S, Castelo R, Guinney J. GSVA: gene set variation analysis for microarray and RNA-seq data. BMC Bioinformatics 2013;14:7. [Crossref] [PubMed]
- Liberzon A, Birger C, Thorvaldsdóttir H, et al. The Molecular Signatures Database (MSigDB) hallmark gene set collection. Cell Syst 2015;1:417-25. [Crossref] [PubMed]
- Subramanian A, Tamayo P, Mootha VK, et al. Gene set enrichment analysis: a knowledge-based approach for interpreting genome-wide expression profiles. Proc Natl Acad Sci U S A 2005;102:15545-50. [Crossref] [PubMed]
- Satpathy S, Krug K, Jean Beltran PM, et al. A proteogenomic portrait of lung squamous cell carcinoma. Cell 2021;184:4348-4371.e40. [Crossref] [PubMed]
- Bindea G, Mlecnik B, Tosolini M, et al. Spatiotemporal dynamics of intratumoral immune cells reveal the immune landscape in human cancer. Immunity 2013;39:782-95. [Crossref] [PubMed]
- Jung S, Bi Y, Davuluri RV. Evaluation of data discretization methods to derive platform independent isoform expression signatures for multi-class tumor subtyping. BMC Genomics 2015;16:S3. [Crossref] [PubMed]
-
Cui H Wang C Maan H scGPT: Towards Building a Foundation Model for Single-Cell Multi-omics Using Generative AI. doi: . - Foy JP, Karabajakian A, Ortiz-Cuaran S, et al. Immunologically active phenotype by gene expression profiling is associated with clinical benefit from PD-1/PD-L1 inhibitors in real-world head and neck and lung cancer patients. Eur J Cancer 2022;174:287-98. [Crossref] [PubMed]
- Hanahan D, Weinberg RA. Hallmarks of cancer: the next generation. Cell 2011;144:646-74. [Crossref] [PubMed]
- Mantovani A, Allavena P, Sica A, et al. Cancer-related inflammation. Nature 2008;454:436-44. [Crossref] [PubMed]
- Gomes M, Teixeira AL, Coelho A, et al. The role of inflammation in lung cancer. Adv Exp Med Biol 2014;816:1-23. [Crossref] [PubMed]
- Li L, He M, Zhou L, et al. A solute carrier family 22 member 3 variant rs3088442 G→A associated with coronary heart disease inhibits lipopolysaccharide-induced inflammatory response. J Biol Chem 2015;290:5328-40. [Crossref] [PubMed]
- Wang SS, Liu W, Ly D, et al. Tumor-infiltrating B cells: their role and application in anti-tumor immunity in lung cancer. Cell Mol Immunol 2019;16:6-18. [Crossref] [PubMed]
- Villegas FR, Coca S, Villarrubia VG, et al. Prognostic significance of tumor infiltrating natural killer cells subset CD57 in patients with squamous cell lung cancer. Lung Cancer 2002;35:23-8. [Crossref] [PubMed]
- Stevens D, Ingels J, Van Lint S, et al. Dendritic Cell-Based Immunotherapy in Lung Cancer. Front Immunol 2020;11:620374. [Crossref] [PubMed]
- Basu A, Ramamoorthi G, Albert G, et al. Differentiation and Regulation of T(H) Cells: A Balancing Act for Cancer Immunotherapy. Front Immunol 2021;12:669474. [Crossref] [PubMed]
- Thommen DS, Schumacher TN. T Cell Dysfunction in Cancer. Cancer Cell 2018;33:547-62. [Crossref] [PubMed]
- Kennedy R, Celis E. Multiple roles for CD4+ T cells in anti-tumor immune responses. Immunol Rev 2008;222:129-44. [Crossref] [PubMed]
- Raskov H, Orhan A, Christensen JP, et al. Cytotoxic CD8(+) T cells in cancer and cancer immunotherapy. Br J Cancer 2021;124:359-67. [Crossref] [PubMed]
- Harlin H, Kuna TV, Peterson AC, et al. Tumor progression despite massive influx of activated CD8(+) T cells in a patient with malignant melanoma ascites. Cancer Immunol Immunother 2006;55:1185-97. [Crossref] [PubMed]