Exploration of a novel prognostic model based on nomogram in non-small cell lung cancer patients with distant organ metastasis: implications for immunotherapy
Highlight box
Key findings
• A prognostic model based on common clinical characteristics is promising for non-small cell lung cancer (NSCLC) patients with distant organ metastasis.
What is known and what is new?
• Advanced NSCLC patients present with metastases, and their prognosis seems to decline sharply despite undergoing immunotherapy. Accessible clinical parameters have unique advantages in terms of accuracy and clinical practicability, which can reflect the overall immune landscape of patients.
• Five indicators (Eastern Cooperative Oncology Group performance status, age-adjusted Charlson comorbidity index, body mass index, absolute neutrophil count, and lactate dehydrogenase) may be correlated with the prognosis of NSCLC following immunotherapy. A feasible and practical model has been developed to predict the prognosis of NSCLC patients with distant organ metastasis prior to immunotherapy.
What is the implication, and what should change now?
• This novel model holds great promise in screening the potential benefits of immunotherapy patients in routine clinical practice while novel biomarkers are developed.
Introduction
According to Global Cancer Statistics 2022, lung cancer is the second most common malignancy with the highest mortality rate worldwide (1). Approximately 85% of cases are of non-small cell lung cancer (NSCLC) (2), and since its early clinical manifestations and signs are not obvious, 40–55% of patients with the disease are first diagnosed at an advanced stage (1,3). The most common distant metastatic sites of advanced NSCLC are the brain, bone, and liver, and in advanced patients presenting metastases, prognoses decline sharply, and the median survival period is only 3–6 months (1,4,5). Therefore, it is important to prevent and evaluate the occurrence and development of metastatic NSCLC. The emergence of immune checkpoint inhibitors (ICIs) is a milestone in the history of cancer drug therapy, which has improved the 5-year survival rate of patients with advanced NSCLC to 16–23% (6-8). While first-line anti-programmed death 1 (anti-PD-1) pembrolizumab treatment for advanced NSCLC results in long-term survival with a 5-year overall survival (OS) rate of 31.9% (9), only 6.2% to 17.5% of asymptomatic or stably metastatic NSCLC patients are included in relevant clinical trials on ICI (10,11). Direct evidence of ICI efficacy in metastatic NSCLC patients remains scarce. Moreover, rapidly increasing cases requiring clinical immunotherapy and associated adverse reaction rates necessitate the identification of predictive biomarkers to assist clinical decision-making.
Previous studies mainly focused on tumor microenvironment (TME), genome, tumor mutation burden (TMB), and other aspects (12,13). However, programmed death legend-1 (PD-L1), TMB, and other classical biomarkers showed heterogeneous expression in different stages of metastasis and in different organs. Genome studies with generation sequencing revealed that mutations detected in samples from metastatic tumors were not recognized in more than 50% of matched primary lung lesions (10,14,15). At the same time, the expensive cost of genomic analysis and unavoidable invasive procedures have severely limited their widespread use in clinical practice. In contrast, easy-to-obtain clinical parameters have unique advantages in terms of accuracy and clinical practicability (16,17). For example, peripheral blood parameters have the advantages of low risk, non-invasive, repeated sampling, and can reflect the overall immune status of patients, enabling researchers to explore the prognosis of patients. Among clinical factors, poor Eastern Cooperative Oncology Group performance status (ECOG PS) (≥2), body mass index (BMI), and lymphocyte and neutrophil counts and relative have been proved to predict outcomes of NSCLC patients receiving ICI therapy in clinical model (18-20). However, previous models incorporating analyzed clinical variables are not comprehensive, with many baseline confounders and limited efficiency (21,22). Furthermore, the prognostic power of above clinical factors in distant metastatic NSCLC patients remains largely unclear. Taken together, the present study aimed to assess the predictive-prognostic model based on clinical parameters in distant metastatic NSCLC patients who received ICI therapy to assist in clinical decision-making. We present this article in accordance with the TRIPOD reporting checklist (available at https://tlcr.amegroups.com/article/view/10.21037/tlcr-23-480/rc).
Methods
Study population
A total of 188 patients treated with ICIs in Xijing Hospital between June 2018 and June 2021 were examined retrospectively. The inclusion criteria were as follows: (I) age ≥18 years; (II) lung cancer diagnosed histologically or cytologically; (III) distant organ metastasis diagnosed pathologically or clinically prior to immunotherapy; (IV) primary treatment for monotherapy or combination therapy; (V) lesions were detected according to Response Evaluation Criteria in Solid Tumors (RECIST) (version 1.1) by at least one evaluation; and (VI) complete clinical and pathological data and follow-up information were available. Patients with other malignant tumors, infections, blood diseases, or autoimmune diseases were excluded. After screening, 100 patients who met the inclusion criteria were enrolled. The study was conducted in accordance with the Declaration of Helsinki (as revised in 2013). The study was approved by the Ethics Committee of Xijing Hospital of Air Force Medical University (approval No. KY20212214-C-1; approval date: 2022.03.16) and informed consent was taken from all individual participants.
Data acquisition
The clinical pathological characteristics of patients were extracted from the electronic inpatient medical record system, and the treatment and follow-up information data were obtained through electronic medical records or telephonic follow-up. The clinical records contained information on gender, age, BMI, smoking status, ECOG PS score, pathological type, clinical stage, number of distant organ metastasis, type of driver gene mutation, treatment plan and line number, age-adjusted Charlson comorbidity index (ACCI), baseline blood cell count and its ratio [neutrophil-to-lymphocyte ratio (NLR), derived NLR (dNLR), platelet-to-lymphocyte ratio (PLR), and monocyte-to-lymphocyte ratio (MLR)], lactate dehydrogenase (LDH), cytokeratin fragment antigen 21-1 (CYFRA21-1), carcinoembryonic antigen (CEA), other serological parameters, and tumor marker indicators. All data were finally updated in June 2022.
Treatment and efficacy assessment
Among the 100 patients who received immune monotherapy or combined therapy, 85 received immune combined chemotherapy, anti-vascular therapy, or a triple regimen. According to the criteria for evaluating the efficacy of solid tumors (RECIST, version 1.1), CT scanning was performed every two cycles or at the stage of tumor progression to assess the prognosis. A follow-up evaluation was conducted every 3 months until the end of the study. OS was defined as the time from the first administration of ICIs to death from any cause or the last follow-up, the primary end-point of our study. PFS was a secondary endpoint, defined as the time from the initiation of immunotherapy to the first record of tumor progression or death from any cause, whichever occurred first. Patients without clinical or imaging progress at the last follow-up were classified as “censored”.
Statistical analysis
For descriptive analysis, the normal and non-normal distributions of continuous variables were expressed by mean ± standard deviation (SD) and median [interquartile range (IQR)], respectively, while categorical variables were presented as percentages. Kaplan-Meier (K-M) curves and a log-rank test were used to calculate OS, and Cox univariate and multivariate survival analyses were used to determine the effect of baseline clinical parameters on OS following immunotherapy. The potential non-linear relationship between clinical factors and outcomes was assessed by restricted cubic spline (RCS), and the optimal cutoff value was selected as the categorical variable and included in the univariate analysis. Statistically significant (P<0.05) variables in univariate analysis were used for further stepwise multivariate Cox regression analysis. A dynamic nomogram model for the prognosis of NSCLC patients with distant metastasis before immunotherapy was constructed based on the screened clinical factors. In the process of model verification and evaluation, the internal validation was performed by the 500 bootstrap resampling method, and a calibration plot was drawn to measure the consistency between the predicted and actual results, indicating the accuracy of the model. Concordance index (C-index) and time-receiver operating characteristic (ROC) curve analyses were conducted to evaluate the prediction accuracy and discriminability of the model, respectively, and area under the curve (AUC) >0.7 suggested a good prediction and discrimination. Finally, K-M curves were used to evaluate the prognosis of patients among three risk subgroups (high, middle, and low). All statistical analyses were performed using R software (version 4.2.0), while the optimal threshold values of age, BMI, and ACCI were calculated using the X-tile software application. All statistical tests were two-sided and P<0.05 was considered statistically significant.
Results
Patient characteristics
A total of 100 NSCLC patients with distant organ metastasis who underwent monotherapy or combination therapy were included (Figure 1). Among them, 24 patients (24%) were over 70 years old, 81 (81%) were male, and 74 (74%) had a history of smoking. In 85 cases (85%), PS was 0 or 1 at the initial stage of immunotherapy. Adenocarcinoma was diagnosed in 65 cases (65%) and squamous cell carcinoma in 33 cases (33%). According to the eighth edition of the tumor-node-metastasis (TNM) classification, 41% of the patients were in stage IVB, and an ACCI score >8 was observed in 71 patients (71%). From the date of the initial immunotherapy to the last follow-up, 77 patients showed progression, 42 died, and the median follow-up time was 18 months [95% confidence interval (CI): 15–19.9]. The median PFS and OS of patients were 8 months (95% CI: 5.6–10.4) and 21 months (95% CI: 8.9–33.1), respectively. Immunotherapy was used as first-line treatment in 61 patients (61%), while 85 (85%) underwent combined immunotherapy. Immune-related adverse effects (irAEs) were seen in 26 (26%) patients. Details of other baseline clinical variables are listed in Table 1.
Table 1
Variables | Value |
---|---|
Gender | |
Female | 19 (19.0) |
Male | 81 (81.0) |
Age | |
≤70 years | 76 (76.0) |
>70 years | 24 (24.0) |
BMI | |
≤23.77 kg/m2 | 71 (71.0) |
>23.77 kg/m2 | 29 (29.0) |
Smoking status | |
Never | 26 (26.0) |
Current | 18 (18.0) |
Former | 56 (56.0) |
Liver metastasis | |
No | 82 (82.0) |
Yes | 18 (18.0) |
Brain metastasis | |
No | 72 (72.0) |
Yes | 18 (18.0) |
Bone metastasis | |
No | 60 (60.0) |
Yes | 40 (40.0) |
ECOG PS | |
0 or 1 | 85 (85.0) |
≥2 | 15 (15.0) |
Histology | |
Adenocarcinoma | 65 (65.0) |
Squamous | 33 (33.0) |
Other NSCLC | 2 (2.0) |
Mutation type | |
No | 71 (71.0) |
EGFR | 8 (8.0) |
KRAS | 13 (13.0) |
Other | 8 (8.0) |
Clinical stage | |
IVA | 59 (59.0) |
IVB | 41 (41.0) |
Line of treatment | |
1 | 61 (61.0) |
≥2 | 39 (39.0) |
ICI drug | |
Pembrolizumab | 47 (47.0) |
Camrelizumab | 7 (7.0) |
Tislelizumab | 9 (9.0) |
Sintilimab | 29 (29.0) |
Nivolumab | 8 (8.0) |
Mono or combo therapy | |
Mono therapy | 15 (15.0) |
Combo therapy | 85 (85.0) |
irAEs | |
No | 74 (74.0) |
Yes | 26 (26.0) |
PD-L1 TPS% | |
<1% | 53 (53.0) |
1–49% | 28 (28.0) |
≥50% | 19 (19.0) |
ACCI | |
≤8 | 29 (29.0) |
>8 | 71 (71.0) |
LDH | P=0.129 |
≤222 U/L | 47 (47.0) |
>222 U/L | 53 (53.0) |
Laboratory parameters | |
Alb (g/L) | 38.08±5.33 |
ANC (×109/L) | 4.68±1.73 |
PLT (×109/L) | 236.51±69.82 |
WBC count (×109/L) | 6.86±2.02 |
AMC (×109/L) | 0.56±0.16 |
ProGRP (pg/mL) | 38.91±10.16 |
ALC (×109/L) | 1.31 (0.93, 1.76) |
AEC (×109/L) | 0.11 (0.05, 0.20) |
LMR | 2.40 (1.79, 3.14) |
NLR | 3.43 (2.43, 4.78) |
dNLR | 2.16 (1.53, 2.75) |
ALI | 241.58 (159.63, 389.68) |
PLR | 183.02 (136.54, 236.72) |
CEA (ng/mL) | 5.32 (3.12, 22.03) |
CYFRA21-1 (ng/mL) | 7.24 (4.14, 20.02) |
SCC (ng/mL) | 1.45 (0.80, 3.95) |
NSE (ng/mL) | 17.12 (12.73, 24.75) |
IL-6 (pg/mL) | 15.99 (7.73, 30.57) |
PCT (ng/mL) | 0.06 (0.04, 0.14) |
Values are presented as n (%), mean ± SD, or median (Q1, Q3). BMI, body mass index; ECOG PS, Eastern Cooperative Oncology Group performance status; NSCLC, non-small cell lung cancer; EGFR, endothelial growth factor receptor; KRAS, Kirsten rat sarcoma viral oncogene homolog; ICI, immune checkpoint inhibitor; irAEs, immune-related adverse effects; PD-L1, programmed death legend-1; TPS, total point score; ACCI, age-adjusted Charlson comorbidity index; LDH, lactate dehydrogenase; Alb, albumin; ANC, absolute neutrophil count; PLT, platelet; WBC, white blood cell; AMC, absolute monocyte count; proGRP, progastrin-releasing peptide; ALC, absolute lymphocyte count; AEC, absolute eosinophil count; LMR, lymphocyte-to-monocyte ratio; NLR, neutrophil-to-lymphocyte ratio; dNLR, derived NLR; ALI, advanced lung cancer inflammatory index; PLR, platelet-to-lymphocyte ratio; CEA, carcinoembryonic antigen; CYFRA21-1, cytokeratin fragment antigen 21-1; SCC, squamous cell carcinoma antigen; NSE, neuron-specific enolase; IL-6, interleukin-6; PCT, procalcitonin; SD, standard deviation.
Relationship between LDH and survival
To avoid direct discarding of several continuous variables in the univariate analysis due to the lack of statistical significance (P<0.05) and ensure statistical rigor, we analyzed the linear relationship between all continuous variables and survival outcomes. This revealed LDH as the only non-linear related indicator. Figure 2 shows a significant non-linear relationship between LDH and OS in NSCLC patients with distant metastasis, and the risk of death increases sharply when LDH reaches 222 U/L (non-linear P=0.0023). The optimal cut-off value of 222 U/L was determined by RCS analysis.
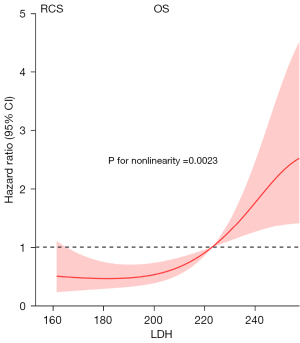
Univariate and multivariate Cox analyses for OS
After screening the prognostic variables from univariate analysis, age, BMI, ECOG PS, clinical stage, ACCI, white blood cell (WBC) count, absolute neutrophil count (ANC), progastrin-releasing peptide (proGRP), interleukin-6 (IL-6), and LDH correlated significantly with OS (P<0.05) (Figure 2; Table S1). After Akaike information criterion (AIC)-based stepwise regression (P<0.05), ECOG PS ≥2 (P<0.001), BMI >23.77 kg/m2 (P=0.003), ACCI >8 (P=0.004), LDH >222 U/L (P=0.012), and ANC (P=0.007) showed statistically significant differences (P<0.05) (Figure 3; Table S1). Among these, ECOG PS exerted the greatest impact on OS, followed by annual ACCI and LDH levels [ECOG PS: hazard ratio (HR) =4.752, 95% CI: 2.073–10.891, P<0.001; ACCI: HR =3.816, 95% CI: 1.538–9.469, P=0.004; LDH: HR =2.461, 95% CI: 1.219–4.970, P=0.012). Further subgroups analysis using K-M curves confirmed BMI was a protective factor for prognosis, while ECOG PS, ACCI, and LDH were associated with shorter OS (Figure 4A-4D). The above results suggest these factors may be independent predictors of immunotherapeutic benefits in NSCLC patients with distant metastasis.
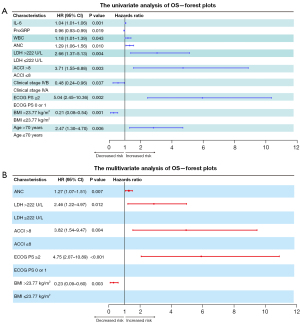

Establishment and validation of OS probability using a dynamic nomogram model
Based on the estimated coefficients in multivariate Cox regression analysis, a survival nomogram model comprising ECOG PS, BMI, ACCI, ANC, and LDH was fit to estimate the 6-, 12-, and 18-month OS probability in the included patients (Figure 5A). The ECOG PS was the most significant influencing factor, and an ECOG PS ≥2 meant a higher risk of death. In detail, each prediction parameter had a corresponding number of risk points obtained by drawing a vertical line from the prediction factor to the original point, and the risk points corresponding to each parameter were summed up to obtain the total score. Finally, a vertical line from the total score axis to the OS probability axis was drawn to obtain the 6-, 12-, and 18-month OS probability. The risk index (RI) was calculated using the following formula: 1.479 × BMI (kg/m2) + 1.558 × ECOG PS + 1.339 × ACCI + 0.900 × LDH (U/L) + 0.237 × ANC (109/L). Additionally, a dynamic nomograph application for predicting OS probability was constructed (https://lucky-wm-nomogram.shinyapps.io/DynNomapp/) (Figure 5B), which can be conveniently available to patients and physicians in the clinical practice. By using the application, the patient’s risk probability plus 95% CI of metastasis could be identified when the information of five variables was input. To assess the predictive ability of models, the C-index for the fitted model was 0.81 (95% CI: 0.778–0.842), and the calibration plot showed no significant deviation between the actual and predicted probabilities (Figure 5C-5E). Moreover, the AUCs of the time-ROC analysis for patients at 6, 12, and 18 months were 0.934, 0.829, and 0.846, indicating a favorable discrimination of the model (Figure 6). In summary, the nomogram for the NSCLC patients with distant organ metastasis had considerable discriminative and calibrating abilities.
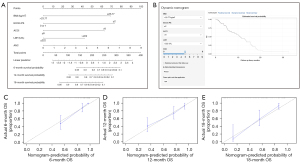
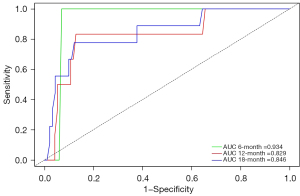
Performance of the dynamic nomogram in stratifying patient risk
X-tile software was used to determine the best cutoff value of the total point score (TPS) in the nomogram, and patients were divided into high (TPS >258; 11 cases), middle (204< TPS ≤258; 23 cases), and low (TPS ≤204; 66 cases) risk subgroups (Figure 7A). The final K-M curves analysis revealed substantial survival disparities among the three subgroups, with patients in the high-risk group showing significantly shorter survival and a worsening tendency (median OS: 4.8 vs. 13.0 vs. 32 months) (Figure 7B).
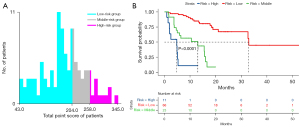
Discussion
According to the Cancer ImmunoAtlas published in Science in 2016, the efficacy of tumor immunotherapy is affected by seven major factors: tumor homology, general immune status of the body, immune cell infiltration, PD-L1 expression, tumor metabolism, ICI solubility, and tumor cell susceptibility to immune effector molecules (23). Recently, much attention has been paid on developing more effective immunotherapies based on the characteristics of TME. The sustained tumor antigen stimulation and immune activation response lead to exhaustion or reshaping of the effector cells in the microenvironment, rendering them unable to perform normal functions or even promoting malignant characteristics of the tumor, resulting in an immunosuppressive microenvironment (24). PD-1 and cytotoxic T lymphocyte-associated protein 4 (CTLA-4) monoclonal antibodies, as classical representatives of immune checkpoint blockade, can restore T cell activation by correcting the immune suppression of the TME which can be considered as a potential cure for some patients with advanced solid tumors (25). Compared to a median survival time of only 12 months for metastatic NSCLC patients a decade ago, patients who received immunotherapy achieved a 5-year survival rate of up to 20% (26). Although visceral metastasis can greatly weaken the body’s anti-tumor immune response, the application of immunotherapy, especially in combination regimens, can effectively improve the PFS and OS of patients with liver and brain metastases (6,27). Clinical studies on bone metastasis are extremely limited, but the combination of bone-targeted therapy and immunotherapy seems to have a synergistic effect (28,29), potentially partially restoring tumor immunogenicity and the bone immune microenvironment. However, these results need to be confirmed in larger-scale prospective clinical trials. Overall, in current clinical practice, the selection of the optimal treatment strategy for different patients remains challenging due to the lack of non-placebo-controlled clinical trials and extreme heterogeneity of metastatic tumor. Utilizing specific predictive biomarkers and establishing effective models may provide valuable guidance for mNSCLC patients in specific therapy regimens (20).
In this study, we comprehensively evaluated various clinical parameters and five indicators (ECOG PS, ACCI, BMI, ANC, and LDH) which were correlated with the prognosis following immunotherapy, emphasizing their potential roles as biomarkers. We then integrated these independent clinical factors to innovatively develop an interactive dynamic nomogram model and transformed it into an application to facilitate its clinical application. The application possessed the unique advantage of intuitively obtaining individual risk based on the personalized parameters of patients, demonstrating its feasibility, good predictive accuracy, and discriminative ability. Different from previous studies (30,31), all patients in our study were confirmed to have distant organ metastases before immunotherapy. However, this group of patients often has poor prognosis and needs more clinical attention. Compared with the reported prognostic model with C-index of 0.74 (95% CI: 0.622–0.860) for NSCLC patients, our model achieved a C-index of 0.81 (95% CI: 0.778–0.842), and the time-ROC analysis of patients at 6, 12, and 18 months showed that the AUC value reached 0.934, 0.829 and 0.846, respectively, which was significantly higher than previous studies (22,32). In addition, we have innovatively transformed it into a mobile device-based predictive web page that can be easily operated by clinicians for clinical application. It should be noted that due to being a single-center study, the representativeness of the patients is limited and the model lacks external validation. The applicability of this model in mNSCLC patients from other sources still needs to be tested in future research.
Although ECOG PS is a negative prognostic factor for lung cancer survival, limited data are available on the safety and efficacy of immunotherapy in NSCLC patients with distant organ metastasis (19,33). CheckMate 153 trials in 1,375 metastatic NSCLC patients revealed the 1-year survival rate of the subgroup with ECOG PS 2 was lower than that in the subgroup with ECOG PS 0–1 (17% vs. 44%) (34). Another retrospective study comprising 75 elderly advanced NSCLC patients showed significant OS differences between ECOG PS 2 and ECOG PS 0–1 (3.8 vs. 13.7 months) subgroups (35). Our findings indicate patients with PS 2 have poor tolerance and responsiveness to ICI treatment compared to those with ECOG PS 0–1, in line with the above conclusions. In patients with distant organ metastasis, restrictions to physical activity may further lead to impaired immune function and an immunosuppressive status (36). However, as a single biomarker, ECOG PS is not sufficient to predict survival. Friedlaender et al. (37) suggest ECOG PS alone is affected by the subjectivity of the evaluator and the differences in Karnofsky scores. The patient’s physical condition is influenced by many factors, such as age, reluctance to walk, medications use (opioids, antidepressants), progression of neoplastic disease (emaciation, weakness), and concomitant disease, such as venous insufficiency of the lower extremities (38). Patients with ECOG PS ≥2 also constitute a largely heterogeneous group. The poor performance status can be caused by the existence of complications or cancer itself, and there are also significant differences between the two conditions in terms of PFS and OS (39). Therefore, other indicators to better assess the prognosis of patients necessitate further studies.
In multivariate analysis, age was not a statistically significant factor. Age alone is often not enough to reflect the reserve capacity of organs and the risk-benefit ratio of immunotherapy. ACCI is an integrative indicator following the quantification of multiple comorbidities and weighted age score, which better reflects the overall functional status of patients. ACCI is mainly used in the context of chemotherapy, and surgery (40,41), and evidence for immunotherapy is relatively limited. Further, no studies currently address the predictive significance of ACCI in NSCLC patients with distant organ metastasis following immunotherapy. A previous study suggests complications in lung cancer patients follow similar pathogenic factors and signal transduction mechanisms, exerting synergistic effects and leading to the deterioration of the basic status of patients and decline in treatment efficacy (42). For real-world clinical settings, our findings incorporated ACCI to determine its potential relationship with the patient’s prognosis, and relative to ACCI ≤8, the ACCI >8 subgroup showed a significantly poorer prognosis. Although there is evidence that complications may affect the progression of aggressive cancers, patients often die of cancer before comorbidities affect survival. Our findings further suggest complications do significantly affect survival in these patients. In our study, tumor stage showed an adverse relationship for OS in the univariate analysis but was non-significant in the multivariate analysis, which suggests tumor stage may not be an independent predictor of OS in this population. Correlation between the ACCI score and prognosis of NSCLC patients with distant metastasis was more significant than tumor stage. In 2017, a retrospective study pointed out for the first time that the Charlson comorbidity index (CCI) score was closely related to the prognosis of patients with advanced NSCLC after chemotherapy, and the cut-off value of CCI was determined by this study as 9 (43). After considering age as a risk factor, the threshold value was further reduced to 8, which has similar results to the previous study, further confirming the reliability of the current study.
Although obesity is the main risk factor for some malignant tumors, its impact on immunotherapy for lung cancer remains controversial. Wang et al. (44) demonstrated increased T cell exhaustion and dysfunction through leptin signaling in obese mice with high PD-1 expression. PD-1-mediated T cell dysfunction in obesity facilitates markedly higher tumor responsiveness to ICIs. Our results indicate a high baseline BMI is a prognostic protective factor in line with several recent findings. Kichenadasse et al. (45) report that immunotherapy confers a better prognosis than chemotherapy in patients with high BMI with a high survival benefit in the PD-L1 positive subgroup. A multicenter study of metastatic NSCLC patients with PD-L1 expression >50% reported that compared to patients with normal weight, obese patients had significantly higher ORR, longer PFS, and OS (18). More importantly, this result was not observed in patients undergoing chemotherapy, which constituted the control group. In our study, regardless of the PD-L1 expression, a higher baseline BMI correlated positively with the patient’s prognosis. Thus, following ICI administration, the microenvironment within metastases in the obese state may lead to greater activation and function of T cells. Whether obesity affects the patient’s prognosis through other immune cells or non-immune factors in metastatic sites requires further evaluation.
We also conducted a comprehensive assessment of the peripheral blood parameters and found elevated baseline ANC correlated significantly with poor OS (P=0.02), which is in accordance with previous studies on the treatment of melanoma with ipilimumab (46,47). Patients with higher baseline ANC serum levels showed higher disease progression with an increased risk of death (HR =3.38, 95% CI: 2.62–4.36; HR =2.52, 95% CI: 1.97–3.21). Neutrophils can maintain tumor stem cells, promoting immune escape, contributing to tumor-related inflammation, and inducing immunotherapy resistance (48). In addition to hematological parameters, immature neutrophils, (CD10 and CD16 or CD15+CD16− according to flow cytometry), are related to the rapid progression in patients with advanced NSCLC undergoing treatment with ICIs (49). Many composite indicators derived from neutrophils, such as NLR and dNLR, are risk factors for disease progression and low survival in several solid tumors (50,51). However, in our study population, no such association was observed. This may be due to the selection of the cutoff value and a more complex immune microenvironment due to metastasis. In conclusion, targeting myeloid-derived immune cells such as neutrophils in the future may provide insights for designing new drug combinations with ICIs.
Similar to neutrophils, as an inflammatory indicator, high levels of LDH result in the production of lactic acid and the acidification of the extracellular matrix, which increases tumor cell aggressiveness (52). LDH is related to the prognosis of melanoma patients treated with ICIs (53), and several retrospective studies show the PFS and OS of NSCLC patients with high LDH following immunotherapy are significantly shorter than those with normal LDH levels (54,55). However, the effect of LDH on the benefit of ICI treatment in advanced NSCLC patients, especially those with distant organ metastasis, remains unclear. Takada et al. (56) found that among patients with advanced NSCLC treated with nivolumab, PFS and OS decreased significantly more in those with LDH >222 U/L than in those with LDH ≤222 U/L. Similarly, our RCS analysis also showed baseline LDH >222 U/L was associated with a poor OS before ICI therapy. However, our current understanding of the importance of LDH remains nascent, which may stem from the lack of a unified cut-off value. LDH is also a marker of large dynamic changes, and in the future, dynamic monitoring of LDH levels in patients undergoing ICI treatment may be valuable for evaluating their prognoses. Of note, the well-known tumor marker PD-L1 was excluded from the nomogram due to no significant difference (P=0.487). While PD-L1 expression can be used to predict the prognosis of patients without organ metastasis prior to immunotherapy, due to its heterogeneity between metastatic and primary sites, whether PD-L1 can serve as prognostic indicators for patients with distant organ metastasis remains unconfirmed.
This study has some other limitations. First, the data used in the analysis were collected retrospectively from a single center, and there is a possibility of bias since the sample size was relatively small. Second, although we conducted internal validation using bootstrap resampling methods, external validation is lacking. Third, various immunotherapeutic agents may exert different effects, and additional stratified analyses based on the use of ICI drugs should be performed in the future. Finally, prospective research needs to be conducted by expanding the sample size to optimize the prognostic model in the future.
Conclusions
Based on common clinical indicators and hematological parameters, we developed a practical and cost-effective prognostic model which can better predict the individual OS probability in NSCLC patients with distant organ metastasis after receiving immunotherapy. These findings may aid clinicians in accurately discriminating the response and prognosis of NSCLC patients in the future.
Acknowledgments
We are grateful to Bullet Edits Limited for their work on the manuscript’s linguistic editing.
Funding: This study was funded by
Footnote
Reporting Checklist: The authors have completed the TRIPOD reporting checklist. Available at https://tlcr.amegroups.com/article/view/10.21037/tlcr-23-480/rc
Data Sharing Statement: Available at https://tlcr.amegroups.com/article/view/10.21037/tlcr-23-480/dss
Peer Review File: Available at https://tlcr.amegroups.com/article/view/10.21037/tlcr-23-480/prf
Conflicts of Interest: All authors have completed the ICMJE uniform disclosure form (available at https://tlcr.amegroups.com/article/view/10.21037/tlcr-23-480/coif). The authors have no conflicts of interest to declare.
Ethical Statement: The authors are accountable for all aspects of the work in ensuring that questions related to the accuracy or integrity of any part of the work are appropriately investigated and resolved. The study was conducted in accordance with the Declaration of Helsinki (as revised in 2013). The study was approved by the Ethics Committee of Xijing Hospital of Air Force Medical University (approval No. KY20212214-C-1; approval date: 2022.03.16) and informed consent was taken from all individual participants.
Open Access Statement: This is an Open Access article distributed in accordance with the Creative Commons Attribution-NonCommercial-NoDerivs 4.0 International License (CC BY-NC-ND 4.0), which permits the non-commercial replication and distribution of the article with the strict proviso that no changes or edits are made and the original work is properly cited (including links to both the formal publication through the relevant DOI and the license). See: https://creativecommons.org/licenses/by-nc-nd/4.0/.
References
- Siegel RL, Miller KD, Fuchs HE, et al. Cancer statistics, 2022. CA Cancer J Clin 2022;72:7-33. [Crossref] [PubMed]
- Ettinger DS, Wood DE, Aisner DL, et al. Non-Small Cell Lung Cancer, Version 3.2022, NCCN Clinical Practice Guidelines in Oncology. J Natl Compr Canc Netw 2022;20:497-530. [Crossref] [PubMed]
- Schabath MB, Cote ML. Cancer Progress and Priorities: Lung Cancer. Cancer Epidemiol Biomarkers Prev 2019;28:1563-79. [Crossref] [PubMed]
- Lamba N, Wen PY, Aizer AA. Epidemiology of brain metastases and leptomeningeal disease. Neuro Oncol 2021;23:1447-56. [Crossref] [PubMed]
- da Silva GT, Bergmann A, Thuler LCS. Incidence and Risk Factors for Bone Metastasis in Non-Small Cell Lung Cancer. Asian Pac J Cancer Prev 2019;20:45-51. [Crossref] [PubMed]
- Borghaei H, Gettinger S, Vokes EE, et al. Five-Year Outcomes From the Randomized, Phase III Trials CheckMate 017 and 057: Nivolumab Versus Docetaxel in Previously Treated Non-Small-Cell Lung Cancer. J Clin Oncol 2021;39:723-33. [Crossref] [PubMed]
- Garon EB, Hellmann MD, Rizvi NA, et al. Five-Year Overall Survival for Patients With Advanced Non-Small-Cell Lung Cancer Treated With Pembrolizumab: Results From the Phase I KEYNOTE-001 Study. J Clin Oncol 2019;37:2518-27. [Crossref] [PubMed]
- Antonia SJ, Borghaei H, Ramalingam SS, et al. Four-year survival with nivolumab in patients with previously treated advanced non-small-cell lung cancer: a pooled analysis. Lancet Oncol 2019;20:1395-408. [Crossref] [PubMed]
- Reck M, Rodríguez-Abreu D, Robinson AG, et al. Five-Year Outcomes With Pembrolizumab Versus Chemotherapy for Metastatic Non-Small-Cell Lung Cancer With PD-L1 Tumor Proportion Score ≥50. J Clin Oncol 2021;39:2339-49. [Crossref] [PubMed]
- Vilariño N, Bruna J, Bosch-Barrera J, et al. Immunotherapy in NSCLC patients with brain metastases. Understanding brain tumor microenvironment and dissecting outcomes from immune checkpoint blockade in the clinic. Cancer Treat Rev 2020;89:102067. [Crossref] [PubMed]
- El Rassy E, Botticella A, Kattan J, et al. Non-small cell lung cancer brain metastases and the immune system: From brain metastases development to treatment. Cancer Treat Rev 2018;68:69-79. [Crossref] [PubMed]
- Wang Z, Duan J, Cai S, et al. Assessment of Blood Tumor Mutational Burden as a Potential Biomarker for Immunotherapy in Patients With Non-Small Cell Lung Cancer With Use of a Next-Generation Sequencing Cancer Gene Panel. JAMA Oncol 2019;5:696-702. [Crossref] [PubMed]
- Hulsbergen AFC, Mammi M, Nagtegaal SHJ, et al. Programmed Death Receptor Ligand One Expression May Independently Predict Survival in Patients With Non-Small Cell Lung Carcinoma Brain Metastases Receiving Immunotherapy. Int J Radiat Oncol Biol Phys 2020;108:258-67. [Crossref] [PubMed]
- Kim R, Keam B, Kim S, et al. Differences in tumor microenvironments between primary lung tumors and brain metastases in lung cancer patients: therapeutic implications for immune checkpoint inhibitors. BMC Cancer 2019;19:19. [Crossref] [PubMed]
- Téglási V, Pipek O, Lózsa R, et al. PD-L1 Expression of Lung Cancer Cells, Unlike Infiltrating Immune Cells, Is Stable and Unaffected by Therapy During Brain Metastasis. Clin Lung Cancer 2019;20:363-369.e2. [Crossref] [PubMed]
- Tian Y, He Y, Li X, et al. Novel nomograms to predict lymph node metastasis and distant metastasis in resected patients with early-stage non-small cell lung cancer. Ann Palliat Med 2021;10:2548-66. [Crossref] [PubMed]
- Deng K, Li S, Zhang J, et al. Prognostic value of thoracic tumor staging and volume parameters in non-small cell lung cancer patients with synchronous solitary bone metastasis. J Thorac Dis 2022;14:1130-8. [Crossref] [PubMed]
- Cortellini A, Ricciuti B, Tiseo M, et al. Baseline BMI and BMI variation during first line pembrolizumab in NSCLC patients with a PD-L1 expression ≥50%: a multicenter study with external validation. J Immunother Cancer 2020;8:e001403. [Crossref] [PubMed]
- Ready NE, Audigier-Valette C, Goldman JW, et al. First-line nivolumab plus ipilimumab for metastatic non-small cell lung cancer, including patients with ECOG performance status 2 and other special populations: CheckMate 817. J Immunother Cancer 2023;11:e006127. [Crossref] [PubMed]
- West H. Prognostic models for immunotherapy: emerging factors for an evolving treatment landscape. Transl Lung Cancer Res 2021;10:9-12. [Crossref] [PubMed]
- Botticelli A, Salati M, Di Pietro FR, et al. A nomogram to predict survival in non-small cell lung cancer patients treated with nivolumab. J Transl Med 2019;17:99. [Crossref] [PubMed]
- Zeng H, Huang WW, Liu YJ, et al. Development and Validation of a Nomogram for Predicting Prognosis to Immune Checkpoint Inhibitors Plus Chemotherapy in Patients With Non-Small Cell Lung Cancer. Front Oncol 2021;11:685047. [Crossref] [PubMed]
- Blank CU, Haanen JB, Ribas A, et al. Cancer Immunology. The "cancer immunogram". Science 2016;352:658-60. [Crossref] [PubMed]
- Turley SJ, Cremasco V, Astarita JL. Immunological hallmarks of stromal cells in the tumour microenvironment. Nat Rev Immunol 2015;15:669-82. [Crossref] [PubMed]
- Murciano-Goroff YR, Warner AB, Wolchok JD. The future of cancer immunotherapy: microenvironment-targeting combinations. Cell Res 2020;30:507-19. [Crossref] [PubMed]
- Cali Daylan AE, Halmos B. Long-term benefit of immunotherapy in metastatic non-small cell lung cancer: the tale of the tail. Transl Lung Cancer Res 2023;12:1636-42. [Crossref] [PubMed]
- Passiglia F, Caglevic C, Giovannetti E, et al. Primary and metastatic brain cancer genomics and emerging biomarkers for immunomodulatory cancer treatment. Semin Cancer Biol 2018;52:259-68. [Crossref] [PubMed]
- Del Conte A, De Carlo E, Bertoli E, et al. Bone Metastasis and Immune Checkpoint Inhibitors in Non-Small Cell Lung Cancer (NSCLC): Microenvironment and Possible Clinical Implications. Int J Mol Sci 2022;23:6832. [Crossref] [PubMed]
- Asano Y, Yamamoto N, Demura S, et al. The Therapeutic Effect and Clinical Outcome of Immune Checkpoint Inhibitors on Bone Metastasis in Advanced Non-Small-Cell Lung Cancer. Front Oncol 2022;12:871675. [Crossref] [PubMed]
- Chen Y, Zhang Q, Lv Y, et al. Prognostic factors of survival in patients with non-small cell lung cancer: a competing risk model using the SEER database. Transl Cancer Res 2022;11:3974-85. [Crossref] [PubMed]
- Chai R, Fan Y, Zhao J, et al. Prognostic nomogram on clinicopathologic features and serum indicators for advanced non-small cell lung cancer patients treated with anti-PD-1 inhibitors. Ann Transl Med 2020;8:1078. [Crossref] [PubMed]
- Sheng Y, Tong L, Geyu L. An immune risk score with potential implications in prognosis and immunotherapy of metastatic melanoma. Int Immunopharmacol 2020;88:106921. [Crossref] [PubMed]
- Felip E, Ardizzoni A, Ciuleanu T, et al. CheckMate 171: A phase 2 trial of nivolumab in patients with previously treated advanced squamous non-small cell lung cancer, including ECOG PS 2 and elderly populations. Eur J Cancer 2020;127:160-72. [Crossref] [PubMed]
- Spigel DR, McCleod M, Jotte RM, et al. Safety, Efficacy, and Patient-Reported Health-Related Quality of Life and Symptom Burden with Nivolumab in Patients with Advanced Non-Small Cell Lung Cancer, Including Patients Aged 70 Years or Older or with Poor Performance Status (CheckMate 153). J Thorac Oncol 2019;14:1628-39.
- Muchnik E, Loh KP, Strawderman M, et al. Immune Checkpoint Inhibitors in Real-World Treatment of Older Adults with Non-Small Cell Lung Cancer. J Am Geriatr Soc 2019;67:905-12. [Crossref] [PubMed]
- Duggal NA, Niemiro G, Harridge SDR, et al. Can physical activity ameliorate immunosenescence and thereby reduce age-related multi-morbidity? Nat Rev Immunol 2019;19:563-72. [Crossref] [PubMed]
- Friedlaender A, Banna GL, Buffoni L, et al. Poor-Performance Status Assessment of Patients with Non-small Cell Lung Cancer Remains Vague and Blurred in the Immunotherapy Era. Curr Oncol Rep 2019;21:107. [Crossref] [PubMed]
- Badaoui S, Shahnam A, McKinnon RA, et al. The predictive utility of patient-reported outcomes and performance status for survival in metastatic lung cancer patients treated with chemoimmunotherapy. Transl Lung Cancer Res 2022;11:432-9. [Crossref] [PubMed]
- Facchinetti F, Mazzaschi G, Barbieri F, et al. First-line pembrolizumab in advanced non-small cell lung cancer patients with poor performance status. Eur J Cancer 2020;130:155-67. [Crossref] [PubMed]
- Charlson ME, Carrozzino D, Guidi J, et al. Charlson Comorbidity Index: A Critical Review of Clinimetric Properties. Psychother Psychosom 2022;91:8-35. [Crossref] [PubMed]
- Kellokumpu I, Kairaluoma M, Mecklin JP, et al. Impact of Age and Comorbidity on Multimodal Management and Survival from Colorectal Cancer: A Population-Based Study. J Clin Med 2021;10:1751. [Crossref] [PubMed]
- Kaseda K, Hishida T, Masai K, et al. Clinicopathological and prognostic features of operable non-small cell lung cancer patients with diabetes mellitus. J Surg Oncol 2021;123:332-41. [Crossref] [PubMed]
- Zhao L, Leung LH, Wang J, et al. Association between Charlson comorbidity index score and outcome in patients with stage IIIB-IV non-small cell lung cancer. BMC Pulm Med 2017;17:112. [Crossref] [PubMed]
- Wang Z, Aguilar EG, Luna JI, et al. Paradoxical effects of obesity on T cell function during tumor progression and PD-1 checkpoint blockade. Nat Med 2019;25:141-51. [Crossref] [PubMed]
- Kichenadasse G, Miners JO, Mangoni AA, et al. Association Between Body Mass Index and Overall Survival With Immune Checkpoint Inhibitor Therapy for Advanced Non-Small Cell Lung Cancer. JAMA Oncol 2020;6:512-8. [Crossref] [PubMed]
- Ferrucci PF, Ascierto PA, Pigozzo J, et al. Baseline neutrophils and derived neutrophil-to-lymphocyte ratio: prognostic relevance in metastatic melanoma patients receiving ipilimumab. Ann Oncol 2018;29:524. [Crossref] [PubMed]
- Soyano AE, Dholaria B, Marin-Acevedo JA, et al. Peripheral blood biomarkers correlate with outcomes in advanced non-small cell lung Cancer patients treated with anti-PD-1 antibodies. J Immunother Cancer 2018;6:129. [Crossref] [PubMed]
- Sangaletti S, Ferrara R, Tripodo C, et al. Myeloid cell heterogeneity in lung cancer: implication for immunotherapy. Cancer Immunol Immunother 2021;70:2429-38. [Crossref] [PubMed]
- Mezquita L, Preeshagul I, Auclin E, et al. Predicting immunotherapy outcomes under therapy in patients with advanced NSCLC using dNLR and its early dynamics. Eur J Cancer 2021;151:211-20. [Crossref] [PubMed]
- Bryant AK, Sankar K, Strohbehn GW, et al. Prognostic and predictive value of neutrophil-to-lymphocyte ratio with adjuvant immunotherapy in stage III non-small-cell lung cancer. Lung Cancer 2022;163:35-41. [Crossref] [PubMed]
- Cortellini A, Ricciuti B, Borghaei H, et al. Differential prognostic effect of systemic inflammation in patients with non-small cell lung cancer treated with immunotherapy or chemotherapy: A post hoc analysis of the phase 3 OAK trial. Cancer 2022;128:3067-79. [Crossref] [PubMed]
- Koukourakis MI, Giatromanolaki A, Sivridis E, et al. Lactate dehydrogenase-5 (LDH-5) overexpression in non-small-cell lung cancer tissues is linked to tumour hypoxia, angiogenic factor production and poor prognosis. Br J Cancer 2003;89:877-85. [Crossref] [PubMed]
- Desai AD, Chinta S, Yeh C, et al. An analysis of lactate dehydrogenase (LDH) levels in advanced stage IV melanoma of the skin: prognostic capabilities and demographic variability. Arch Dermatol Res 2023;315:799-806. [Crossref] [PubMed]
- Tjokrowidjaja A, Lord SJ, John T, et al. Pre- and on-treatment lactate dehydrogenase as a prognostic and predictive biomarker in advanced non-small cell lung cancer. Cancer 2022;128:1574-83. [Crossref] [PubMed]
- Wang S, Lv J, Lv J, et al. Prognostic value of lactate dehydrogenase in non-small cell lung cancer patients with brain metastases: a retrospective cohort study. J Thorac Dis 2022;14:4468-81. [Crossref] [PubMed]
- Takada K, Takamori S, Matsubara T, et al. Clinical significance of preoperative inflammatory markers in non-small cell lung cancer patients: A multicenter retrospective study. PLoS One 2020;15:e0241580. [Crossref] [PubMed]