Recurrence prediction of lung adenocarcinoma using an immune gene expression and clinical data trained and validated support vector machine classifier
Highlight box
Key findings
• Immune genes’ expression data improves the accuracy of lung cancer recurrence prediction.
What is known and what is new?
• Combining immune gene expression data with clinicopathologic prognostic factors improves the accuracy of tumor recurrence prediction.
• ML methods help produce stronger prognostic predictors.
What is the implication, and what should change now?
• More precise immune biomarkers are needed to boost the performance of recurrence prediction.
Introduction
Lung cancer is the leading cause of cancer death worldwide, and it is estimated to contribute 21% of the total cancer deaths in 2023 (1). The most common subtype of lung cancer is lung adenocarcinoma (LUAD), which accounts for about 50% of all lung cancer cases (2). Lung cancer is potentially curable if detected early and treated with surgery (3,4). However, the long-term post-resection survival rate for stage IA2–IIIC is only approximately 17.8%, and recurrence develops in approximately 25% of patients, even when the tumor has been completely resected (5). Additionally, LUAD patients within the same stage have different patterns of disease progression (6). In recent studies, several clinicopathologic prognostic factors have shown correlation with tumor recurrence, such as tumor-node-metastasis (TNM) stage (7), size of non-lepidic invasive pattern (8,9), histological subtype (10-12), lymphovascular invasion (LVI) (13), and visceral pleural invasion (VPI) (14). Based on these variables, recurrence prediction models have been built in recent studies. Yu et al. (15) constructed a nomogram model based on smoking, solid nodules, mucinous LUAD, and micropapillary component. The internal and external validation C-indexes of the nomogram were 0.822 [95% confidence interval (CI): 0.751–0.891] and 0.812, respectively. Genetic predisposition is also involved in tumor recurrence. Single nucleotide variants of MSH5, MMP9, and CYP2D6 were found significantly associated with early-stage LUAD presenting with ground-glass nodules (GGNs) (16). In a study by Janik et al., graph machine learning (ML) achieved 68% accuracy for early-stage non-small cell lung cancer (NSCLC) (17). Incorporating clinical, pathological, and biological aspects into the prediction model, the predictive value of the model [receiver operating characteristic (ROC) curve area under the curve (AUC) =0.723] is better than that of single independent risk factors (18). Bacterial biomarkers also played a role in predicting the survival of lung cancer patients. The relative abundances of bacteria were significantly different between the recurrence group and non-recurrence group (19).
Growing evidence emphasizes that the tumor immune microenvironment has a significant impact on tumor progression. Tumor-infiltrating immune cells (TIICs) have been found to be associated with prognosis in patients with early-stage LUAD (20). Nevertheless, few studies have investigated the link between TIICs and recurrence in NSCLC patients. In addition, the rapid development of molecular biology techniques including whole genome sequencing, as well as current bioinformatics methods have provided evidence of differentially expressed genes (DEGs) related to survival outcome (21). A gene expression deconvolution algorithm [Cell-type Identification by Estimating Relative Subsets of RNA Transcripts (CIBERSORT)] has been developed to computationally dissect the relative proportions of 22 TIIC subtypes (22). ML methods have been widely used in a variety of aspects in cancer research including imaging analysis, biomarker identification, and disease stage classification (23-25). These mathematical methods and powerful computing resources provide an opportunity to improve prediction accuracy of cancer susceptibility, recurrence, and survival.
In the present study, clinicopathological parameters, immune gene expression data of tumor tissues, and blood samples were used for analysis. The landscape of TIICs was estimated by CIBERSORT. A total of 4 support vector machine (SVM)-based models for the prediction of LUAD recurrence were proposed with calculation of accuracy, sensitivity, and specificity as well as the AUC. We present this article in accordance with the STARD reporting checklist (available at https://tlcr.amegroups.com/article/view/10.21037/tlcr-23-473/rc).
Methods
Study population
The study was conducted in accordance with the Declaration of Helsinki (as revised in 2013). The Institutional Review Board (IRB) of New York University Langone Medical Center (New York, NY, USA) approved this single-institutional retrospective study (No. i8896_CR24), and informed consent was obtained using IRB approved protocol 8896 for collection and archiving of tissue and blood samples from patients with presumed or diagnosed lung cancer. Samples were prospectively collected from LUAD patients who were diagnosed and underwent surgery at New York University Langone Medical Center between June 2007 and October 2014. The inclusion criteria were as follows: (I) patients were pathologically confirmed as having LUAD after undergoing complete video-assisted thoracoscopic surgery (VATS) resection at New York University Langone Medical Center; (II) patients were diagnosed as early-stage NCSLC (stage IA–IIB); (III) patients had snap-frozen tumor tissue and buffy coat collected at the time of resection; (IV) patients were actively followed-up; and (V) the quantity and quality of extracted RNA met the criteria for NanoString experiment. A total of 41 patients were eligible for the study and were divided into a with recurrence group (n=16) and a without recurrence group (n=25) according to their follow-up information. Tissue and blood samples of these 41 patients were used for RNA extraction. The extracted RNA was then assessed by NanoString assay to profile the expression of immune genes.
RNA extraction
Tissue RNA was extracted from frozen tumor tissue using the RNeasy Mini Kit (Qiagen, Hilden, Germany). Buffy coat RNA was extracted using Quick-RNA Kits (ZYMO Research, Irvine, CA, USA). A total of 125 ng of RNA was used for the NanoString immunoncology assay. RNA quantity and quality were assessed by Nanodrop One© (Thermo Fisher Scientific, Waltham, MA, USA).
Immune profiling
We used NanoString technology to profile 730 immune oncogenes. The hybridization reaction involved 5 µL (25 ng/µL) of sample RNA, 3 µL of Reporter CodeSet, 5 µL of hybridization buffer, and 2 µL of Capture ProbeSet. The mixture was incubated at 65 ℃ for 16–20 hours. After hybridization, the samples were transferred to a gene-specific probes-coated cartridge using nCounter Prep Station (NanoString, Seattle, WA, USA) and then scanned for gene expression using a NanoString Digital Analyzer at high fields of visualization (550 FOV).
Quantification of gene expression values
The gene expression data were checked for quality control (QC) and normalized using the nSolver 3.0 software (NanoString). Any QC failed samples were removed from the analysis. The gene expression counts were normalized to the internal housekeeping genes to correct any differences in physiological experimental conditions and background signal across the runs. The list of the housekeeping genes was provided in Table S1. The corrected gene expression counts were used for the following TIICs evaluation and model construction.
Evaluation of TIICs
CIBERSORT is an analytical tool used to quantify the abundance of specific cell types in a mixed cell population using a gene expression-based approach. In this study, we used the LM22 signature file which was based on 547 genes to define the 22 subtypes of TIICs, including T cells, B cells, natural killer (NK) cells, macrophages, dendritic cells, monocytes, mast cells, eosinophils, neutrophils, and plasma cells. Using the median of the proportion of each cell subtype, we divided the patients into high- and low-density groups. The association of TIICs and corresponding overall survival (OS) was analyzed by Kaplan-Meier (KM) curves using the log-rank test.
Integration of the protein-protein interaction (PPI) network and KEGG enrichment analysis of DEGs
The Search Tool for the Retrieval of Interacting Genes/Proteins (STRING) version 10.0 (https://string-db.org/) was used for the exploration of potential DEG interactions at the protein level. Hub genes were identified using the Cytohubba plugin of cytoscape. Kyoto Encyclopedia of Genes and Genomes (KEGG) pathway analysis was conducted using the R clusterProfiler package to identify DEGs at the biologically functional level. P<0.05 was considered to indicate a statistically significant difference.
SVM with recursive feature elimination (SVM-RFE) data analysis
The entire research design is shown in Figure S1. To pre-select the DEGs, DESeq2 was used to model the dispersion of the gene expression data on the mean first, and then reduce the influence of outliers. After that, a Bayesian inference approach was used to estimate the dispersion of each gene and generate posterior probabilities and false discovery rates for DEGs. ML methods could be used in both biomarkers’ discovery and disease category classification. In this study, we implemented supervised disease classification using SVM with the linear kernel (SVM-Linear). Each type of data (clinical data, gene expression data of tumor tissue, and buffy coat) of progressors and non-progressors were randomly distributed into a training set (75%) and a validation set (25%). First, we used the data from the training set to train the models, then we applied the trained models to the validation dataset to further confirm the accuracy, specificity, and sensitivity. For the model training iterations, we employed a 25-fold cross-validation approach with folds resampled 15 times.
For each split of the expression data, the top 45 genes from tumor tissue and top 47 genes from buffy coat were selected according to the rank generated by Bayesian inference. The SVM-Linear model was trained on 9-fold and then tested on the remaining folds. Each model evaluated the importance of the input genes separately, and the final evaluation score was calculated by the contribution of each gene to the model. For each feature selection iteration, features with the least absolute model weights and contributions were eliminated. ROC curve was plotted using the pROC package in R, and AUC, sensitivity, and specificity were computed. Ninety-five percent CIs for sensitivity, specificity, and AUC were computed using bootstrapping techniques with the boot package in R. The final list of gene and clinical variables had the minimum number of features which maintained an AUC within 1% of the AUC achieved by the original 45 genes from the solid tissue samples and 47 genes from the buffy coat panel. Independent validation datasets were used to test the validity of the generated classifiers.
Results
Characteristics of the study participants
The clinical and demographic variables of 41 LUAD patients are listed in Table 1. Patients with recurrence had larger tumor size (2.9 vs. 2.0 cm, P=0.003) and more VPI (56.3% vs. 16.0%, P=0.007), but age, gender, and smoking history were not significantly different. The proportion of micropapillary/solid subtype (50.0% vs. 36.0%), positive lymph node (18.8% vs. 8.0%), stage IIB disease (25.0% vs. 8.0%), and segmentectomy (12.5% vs. 8.0%) tended to be higher in patients with recurrence, and although there were less lymph nodes resected (>9 lymph nodes resected: 25.0% vs. 48.0%) in this group, all of them were not statistically significant.
Table 1
Characteristics | With recurrence (n=16) | Without recurrence (n=25) | P value |
---|---|---|---|
Age (years), median (IQR) | 69.5 (62.8–79.5) | 65.0 (59.5–71.0) | 0.133 |
Gender, n (%) | 0.565 | ||
Male | 6 (37.5) | 6 (24.0) | |
Female | 10 (62.5) | 19 (76.0) | |
Smoking, n (%) | 0.754 | ||
≤25 pack-year | 11 (68.8) | 16 (64.0) | |
>25 pack-year | 5 (31.3) | 9 (36.0) | |
Tumor size (cm), median (IQR) | 2.9 (2.03–3.43) | 2.0 (1.50–2.50) | 0.003 |
Histology, n (%) | 0.375 | ||
Lepedic/acinar/papillary | 8 (50.0) | 16 (64.0) | |
Micropapillary/solid | 8 (50.0) | 9 (36.0) | |
VPI, n (%) | 0.007 | ||
No | 7 (43.8) | 21 (84.0) | |
Yes | 9 (56.3) | 4 (16.0) | |
Positive lymph node, n (%) | 0.591 | ||
No | 13 (81.3) | 23 (92.0) | |
Yes | 3 (18.8) | 2 (8.0) | |
Stage, n (%) | 0.294 | ||
IA/IB | 12 (75.0) | 23 (92.0) | |
IIB | 4 (25.0) | 2 (8.0) | |
Surgical procedure, n (%) | 1.000 | ||
Lobectomy/pneumonectomy | 14 (87.5) | 23 (92.0) | |
Segmentectomy | 2 (12.5) | 2 (8.0) | |
Lymph nodes resected, n (%) | 0.141 | ||
≤9 | 12 (75.0) | 13 (52.0) | |
>9 | 4 (25.0) | 12 (48.0) |
IQR, interquartile range; VPI, visceral pleural invasion.
During a follow-up period with a median length of 67.1 months, there were 10 patients with locoregional recurrence, and 6 patients with systemic recurrence. The frequency of recurrence was 39%. A total of 10 patients died during the follow-up.
The distribution of TIICs in progressors and non-progressors and their prognostic value in LUAD
Figure 1A summarizes the composition of 22 TIICs in the 41 included patients. The relative abundances of TIICs according to the progression status of patients were evaluated by t-test (Figure 1B). Recruitment of T CD4+ memory resting cells, activated NK cells, M0 macrophages, and M1 macrophages was higher in patients with progression than in those without progression (P<0.001, P=0.0089, P<0.001, and P=0.0016, respectively). Meanwhile, higher recruitment of M2 macrophages and mast cells resting were identified in patients without progression (both P<0.001).
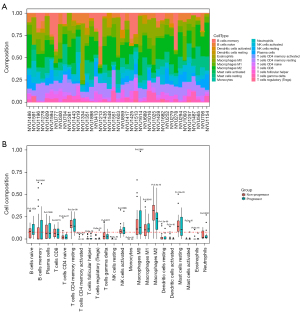
We analyzed the correlation between immune cell infiltrates and corresponding OS among the enrolled patients. Only TIICs with a proportion of ≥5% and a significant difference between progressors and non-progressors were included in the analysis. Using the median percentage as a cut-off value for each type of TIIC, patients were divided into low and high groups accordingly. We found that a higher proportion of M2 macrophages was associated with a better prognosis (mean OS time, 3,675 vs. 2,179 days, P=0.048). There were no differences between the low proportion and high proportion of the other TIICs (Figure 2).
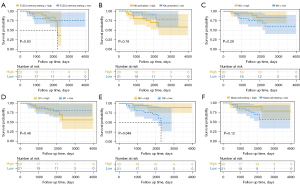
Clinical parameter-based ML classifier
The clinical prognostic variables include age, gender, race, smoking history, tumor size, histology, LVI, VPI, positive lymph nodes, extent of resection, and number of resected lymph nodes. The ranking of these top 12 variables is shown in Figure 3A. An SVM-Linear model built with these clinical data showed low prediction accuracy. The AUC for the training set was 62.7% (95% CI: 56.3–69.1%), and the AUC for the validation set was 58.3% (95% CI: 17.9–98.8%) (Figure 3B). It was indicated that using these clinical parameters to predict the recurrence of LUAD is far from ideal (Table 2).
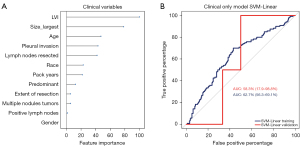
Table 2
Dataset | Performance metric | Tumor gene expression | Blood gene expression | Clinical | Tumor + clinical | Blood + clinical | All combined |
---|---|---|---|---|---|---|---|
Training | Accuracy (%) | 37.4 | 67.0 | 67.0 | 82.4 | 87.9 | 91.2 |
Sensitivity (%) | 81.1 | 90.5 | 86.3 | 78.4 | 88.4 | 89.5 | |
Specificity (%) | 7.5 | 23.3 | 24.2 | 55.8 | 65.0 | 62.5 | |
AUC (%) | 65.4 | 59.7 | 62.7 | 68.6 | 84.2 | 92.0 | |
95% CI (%) | 59.2–71.5 | 52.8–66.5 | 56.3–69.1 | 62.4–74.7 | 79.8–88.5 | 89.0–95.0 | |
Validation | Accuracy (%) | 60.0 | 60.0 | 50.0 | 70.0 | 70.0 | 90.0 |
Sensitivity (%) | 0.0 | 0.0 | 0.0 | 75.0 | 50.0 | 75.0 | |
Specificity (%) | 100.0 | 100.0 | 83.3 | 66.7 | 83.3 | 100.0 | |
PPV (%) | NA | NA | 0.0 | 60.0 | 66.7 | 100.0 | |
NPV (%) | 60.0 | 60.0 | 55.6 | 80.0 | 71.4 | 85.7 |
SVM-Linear, SVM with the linear kernel; SVM, support vector machine; ML, machine learning; AUC, area under the curve; CI, confidence interval; PPV, positive predictive value; NA, not applicable; NPV, negative predictive value.
Hub genes and KEGG pathway analysis
A total of 92 differential expression genes were screened out by Bayesian inference. In a PPI network containing 89 nodes and 663 edges, ten hub genes (TNF, IL6, CD8A, GZMB, CXCL8, TBX21, PRF1, KLRK1, IRF4, and CD247) were identified. Among them, the score of TNF and IL6 were more than 60 (Table S2). KEGG pathway analysis revealed ‘Cytokine-cytokine receptor interaction’, ‘Natural killer cell mediated cytotoxicity’, ‘NF-kappa B signaling pathway’, ‘T cell receptor signaling pathway’, ‘Toll-like receptor signaling pathway’, ‘PI3K-Akt signaling pathway’, ‘JAK-STAT signaling pathway’, ‘TNF signaling pathway’, ‘NOD-like receptor signaling pathway’ and ‘FoxO signaling pathway’ were enriched significantly (Figure S2).
ML classifiers based on gene expression data
In total, 730 immune genes were tested. To address the issue of data overfitting and to test the generality of the classification model, we split the data into a training set (75%) for analysis and a validation set (25%) for validation. The gene expression profiles from the training set were used to train the ML classifier to predict the risk of recurrence. We applied an SVM-RFE and 25-fold cross-validation to rank the features based on their performance to classify progressive and non-progressive cancer. The lower ranking genes were removed. Eventually, a set of the top 45 genes from tumor tissue and a set of the top 47 genes from buffy coat demonstrated the optimized model fitting. Figure 4 lists all these top genes according to their contribution to the models.
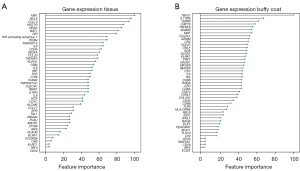
The following analyses were carried out with the 45-gene classifier and 47-gene classifier respectively. As shown in Figure 5A,5B, the SVM-Linear classifier based on tumor tissue expression data showed an AUC of 65.4% (95% CI: 59.2–71.5%), the SVM-Linear classifier based on buffy coat data showed an AUC of 59.7% (95% CI: 52.8–66.5%). Similar accuracies were found in the two validation sets: the AUC for tumor tissue was 83.3% (95% CI: 55.7–100.0%) and the AUC for buffy coat was 75.0% (95% CI: 42.1–100.0%). The two models showed good accuracies for distinguishing progressors from non-progressors (Table 2).
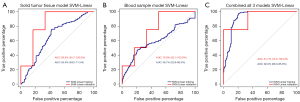
Combo-classifier (combination of clinical variables and/or gene expression data)
In order to improve prediction accuracy, we combined clinical data with gene expression data from both tumor tissue and buffy coat (Table 2). The final combo-classifier showed a significant increase in accuracy, sensitivity, and specificity. The AUC in the training set reached 92.0% (95% CI: 89.0–95.0%), whereas that in the validation set reached 91.7% (95% CI: 72.3–100.0%) (Figure 5C).
Discussion
Lung cancer is the leading cause of cancer-related death, with dismal 5-year survival rates (1). Even patients in the early stage of disease exhibit wide variation in prognosis, and some develop tumor recurrence and die of the disease despite curative surgical resection (26). Factors associated with recurrence include histological, clinical, and population-based characteristics (27,28). However, these parameters do not provide sufficient information for making robust decisions for treatment. Increasing evidence indicates that the immune profile of the tumor microenvironment plays a major role in the development and progression of cancer (20,29). Karasaki et al. (30) assessed the expression of genes related to cancer immunity and constructed three immunogram patterns, which can be used as integrated biomarkers. The cellular composition of infiltrating immune cells in lung cancer tissue has also been found to be related to tumor progression and prognosis (31). In this study, we quantified the expression level of 730 immune genes in both tumor tissue and buffy coat first. Then, the top 45 genes from tumor tissue and top 47 genes from buffy coat were selected to build recurrence prediction classifiers. Prediction accuracy was compared between different classifiers, among which the combo-classifier had the best prediction accuracy, achieving 91.2% in the training set and 90.0% in the validation set.
We analyzed the composition of TIICs and identified a higher abundance of M0 and M1 macrophages in progressive patients and a higher abundance of M2 macrophages in non-progressive patients. Our findings contrast with the results from other studies. Dong et al. (32) demonstrated that M0 macrophages had a higher infiltration level in the metastatic group and might differentiate into M2 macrophages (33). M2 is the main subtype macrophage in advanced lung cancer, and related with resistance to osimertinib (34). However, whether it is the same in early-stage LUAD remains unclear. Furthermore, the phagocytic activity of macrophage is suppressed by the interaction between CD24 on tumor cells and Siglec-10 on macrophages (35). In addition to phagocytosis, macrophages also play a critical role in inflammation. M2 macrophages function to promote angiogenesis, tissue remodeling, and repair, altogether shifting the immune response to an anti-inflammatory response (36). Phu et al. (37) observed that M2 macrophage serve to control inflammation in the liver and adipose tissue of obese mice.
As a popular mathematical tool, ML can improve the accuracy of cancer prediction by 15–20% (23). In the present study, by using an SVM-Linear algorithm, we established an optimized combo-classifier for predicting recurrence in surgically treated early-stage LUAD patients. The accuracy, specificity, and sensitivity of this combo-classifier based on the training set were 91.2%, 62.5%, and 89.5% respectively, and the AUC was 92.0% in the training set and 91.7% in the validation set. There is a big jump in the performance of the individual classifiers and the combo-classifier. AUC for the clinical model was 58.3% in the validation set. AUC for the gene models was 83.3% (tumor tissue) and 75.0% (buffy coat) respectively. The clinical model based mostly on the staging system, which is too broad to predict prognosis precisely and help guide treatment. While more and more evidence show that gene-related biomarkers improve prediction accuracy. Expression of genes changes throughout the development of cancer, it provides more information than clinical features. Forty-five genes from tumor tissue and 47 genes from buffy coat consisted all gene features for the combo-model, which included sufficient information. Compared with Huang et al.’s (38) integrated deep learning evaluation score (AUC =81.7%), our combo-classifier showed better prediction accuracy. However, the specificity of circulating tumor DNA (ctDNA) detection in Gale et al.’s (39) study was 98.7%, which is far better than ours (62.5%). indicating that the combo-classifier still needs further investigation and development.
According to the gene expression data, we identified MR1, BCL6, and CCL13 as the top 3 genes which were significantly differentially expressed in tumor tissue between patients with and without recurrence. TBX21, IL-17RB, and GZMB were identified as the top 3 genes in buffy coat. Most of these immune genes had been shown to have a role in cancer in previous studies. MR1 is a non-polymorphic major histocompatibility complex (MHC) I-like protein which can be recognized by T cell receptor (TCR). Cancer cells carrying the surface molecule MR1 can thus be killed by MR1 T cells (40). This offers a new front of targets for adoptive T cell immunotherapy (41). BCL6 is a critical protooncogene that regulates the growth of B-lymphocytes. It also promotes tumor progression and contributes to malignant behavior in lymphomas, breast cancer, gastric cancer, ovarian cancer, NSCLC, and glioblastoma (42,43). In NSCLC patients, BCL6 is amplified in 40% of squamous cell carcinomas [197/501, The Cancer Genome Atlas (TCGA)] and in 2.2% of adenocarcinomas (5/230, TCGA). The major role of BLC6 in NSCLC could be mediating the repression of DNA damage genes and sustaining genomic instability (44). MCP-4, also called CCL13, is a ligand for 3 different G protein coupled receptors, CCR2, CCR3, and CCR5. Yang et al. (45) assessed the serum level of chemokines in NSCLC patients and found the levels of GCP-2, IL-18-BPa, and MCP-4 were significantly higher in patients than in the healthy volunteers. Okugawa et al. (46) also assessed the serum levels of MCP-4 in colorectal cancer patients and found that elevated MCP-4 was a significant and independent prognostic factor of disease-free survival and OS. The type 1 T helper (Th1) cell-specific transcription factor TBX21 was found to maintain cancer stemness. Zhao et al.’s (47) study of LUAD showed that the TBX21-IL-4 pathway could promote tumor initiation, tumor growth, and expression of stemness markers. It could be used to construct a prognostic model that could distinguish LUAD patients with high or low risk of survival. IL-17B is a member of the IL-17 family, and it is lowly expressed in various tissues. The overexpression of IL-17RB was found to be strongly correlated with postoperative metastasis in pancreatic cancer patients (48). It upregulates cell stemness through activating the AKT/β-catenin pathway in gastric cancer (49). In colorectal cancers, long-term ablation of IL-17RB expressing cancer stem cells strongly suppressed the tumor growth in vivo (50). GZMB is stored in secretory granules of cytotoxic T lymphocytes (CTLs) and NK cells and used by these two types of cells to eliminate harmful target cells including allogeneic, virally infected, and tumor cells (51). In melanoma, GZMB expression in myeloid-derived suppressor cells (MDSCs) is another means to promote tumor growth (52). In NSCLC cells, RocA has been shown to inhibit autophagy and restore the level of NK cell-derived GZMB, therefore increasing their susceptibility to NK cell-mediated killing (53).
This study had some limitations. Further studies are needed to confirm the combo-recurrence classifier we identified in the present study based on the following factors: (I) the sample size is quite small, only 41 samples were included, which may not be sufficient to draw a firm conclusion; (II) too many genes were included in the combo-classifier, which may make it inconvenient and costly in clinical application. Thus, future development of a simpler combination of genes which does not sacrifice accuracy would be preferable; and (III) we haven’t test our models in a test group of patients to prove its prediction accuracy. Moreover, the supervised ML method also has a few but well-known limitations. One is the lack of interpretability, which means a suitable explanation of how the predicted results were related to the genes and clinical factors. Another limitation is the lack of repeatability. As it was apparent in this study, there is a lack high-quality, accurate, and sufficient data to train the ML models. The prediction relies on the weight of selected genes and subjectively observed clinical variables. However, the clinical variable may vary according to different measurement methods and subjective choices, and the NanoString data also relies on the platform and analysis pipeline used for the data processing.
Conclusions
Early-stage LUAD patients are diverse with varying risk of recurrence. A novel combo-ML classifier was built based on both clinicopathological parameters and gene expression features, and it was shown to outperform the standard clinical classifier in accuracy, specificity, and sensitivity.
Acknowledgments
Funding: This work was supported by
Footnote
Reporting Checklist: The authors have completed the STARD reporting checklist. Available at https://tlcr.amegroups.com/article/view/10.21037/tlcr-23-473/rc
Data Sharing Statement: Available at https://tlcr.amegroups.com/article/view/10.21037/tlcr-23-473/dss
Peer Review File: Available at https://tlcr.amegroups.com/article/view/10.21037/tlcr-23-473/prf
Conflicts of Interest: All authors have completed the ICMJE uniform disclosure form (available at https://tlcr.amegroups.com/article/view/10.21037/tlcr-23-473/coif). The authors have no conflicts of interest to declare.
Ethical Statement: The authors are accountable for all aspects of the work in ensuring that questions related to the accuracy or integrity of any part of the work are appropriately investigated and resolved. The study was conducted in accordance with the Declaration of Helsinki (as revised in 2013). The Institutional Review Board (IRB) of New York University Langone Medical Center (New York, NY, USA) approved this single-institutional retrospective study (No. i8896_CR24), and informed consent was obtained using IRB approved protocol 8896 for collection and archiving of tissue and blood samples from patients with presumed or diagnosed lung cancer.
Open Access Statement: This is an Open Access article distributed in accordance with the Creative Commons Attribution-NonCommercial-NoDerivs 4.0 International License (CC BY-NC-ND 4.0), which permits the non-commercial replication and distribution of the article with the strict proviso that no changes or edits are made and the original work is properly cited (including links to both the formal publication through the relevant DOI and the license). See: https://creativecommons.org/licenses/by-nc-nd/4.0/.
References
- Siegel RL, Miller KD, Wagle NS, et al. Cancer statistics, 2023. CA Cancer J Clin 2023;73:17-48. [Crossref] [PubMed]
- Torre LA, Siegel RL, Jemal A. Lung Cancer Statistics. Adv Exp Med Biol 2016;893:1-19. [Crossref] [PubMed]
- Zappa C, Mousa SA. Non-small cell lung cancer: current treatment and future advances. Transl Lung Cancer Res 2016;5:288-300. [Crossref] [PubMed]
- Berman AT, Jabbour SK, Vachani A, et al. Empiric Radiotherapy for Lung Cancer Collaborative Group multi-institutional evidence-based guidelines for the use of empiric stereotactic body radiation therapy for non-small cell lung cancer without pathologic confirmation. Transl Lung Cancer Res 2019;8:5-14. [Crossref] [PubMed]
- Xu W, Jia G, Davie JR, et al. A 10-Gene Yin Yang Expression Ratio Signature for Stage IA and IB Non-Small Cell Lung Cancer. J Thorac Oncol 2016;11:2150-60. [Crossref] [PubMed]
- Lee CY, Shim HS, Lee S, et al. Prognostic effect of matrix metalloproteinase-9 in patients with resected Non small cell lung cancer. J Cardiothorac Surg 2015;10:44. [Crossref] [PubMed]
- Bucciarelli PR, Tan KS, Chudgar NP, et al. BRMS1 Expression in Surgically Resected Lung Adenocarcinoma Predicts Future Metastases and Is Associated with a Poor Prognosis. J Thorac Oncol 2018;13:73-84. [Crossref] [PubMed]
- Hwang S, Han J, Choi M, et al. Size of Non-lepidic Invasive Pattern Predicts Recurrence in Pulmonary Mucinous Adenocarcinoma: Morphologic Analysis of 188 Resected Cases with Reappraisal of Invasion Criteria. J Pathol Transl Med 2017;51:56-68. [Crossref] [PubMed]
- Su H, Dai C, She Y, et al. Which T descriptor is more predictive of recurrence after sublobar resection: whole tumour size versus solid component size? Eur J Cardiothorac Surg 2018;54:1028-36. [Crossref] [PubMed]
- Yoshida Y, Nitadori JI, Shinozaki-Ushiku A, et al. Micropapillary histological subtype in lung adenocarcinoma of 2 cm or less: impact on recurrence and clinical predictors. Gen Thorac Cardiovasc Surg 2017;65:273-9. [Crossref] [PubMed]
- Ujiie H, Kadota K, Chaft JE, et al. Solid Predominant Histologic Subtype in Resected Stage I Lung Adenocarcinoma Is an Independent Predictor of Early, Extrathoracic, Multisite Recurrence and of Poor Postrecurrence Survival. J Clin Oncol 2015;33:2877-84. [Crossref] [PubMed]
- Qian F, Yang W, Wang R, et al. Prognostic significance and adjuvant chemotherapy survival benefits of a solid or micropapillary pattern in patients with resected stage IB lung adenocarcinoma. J Thorac Cardiovasc Surg 2018;155:1227-1235.e2. [Crossref] [PubMed]
- Kiankhooy A, Taylor MD, LaPar DJ, et al. Predictors of early recurrence for node-negative t1 to t2b non-small cell lung cancer. Ann Thorac Surg 2014;98:1175-83. [Crossref] [PubMed]
- Seok Y, Lee E. Visceral Pleural Invasion Is a Significant Prognostic Factor in Patients with Partly Solid Lung Adenocarcinoma Sized 30 mm or Smaller. Thorac Cardiovasc Surg 2018;66:150-5. [Crossref] [PubMed]
- Yu S, You C, Yan R, et al. Establishment and validation of a nomogram model for predicting postoperative recurrence-free survival in stage IA3 lung adenocarcinoma: a retrospective cohort study. Transl Lung Cancer Res 2022;11:2275-88. [Crossref] [PubMed]
- Fu R, Zhang JT, Chen RR, et al. Identification of heritable rare variants associated with early-stage lung adenocarcinoma risk. Transl Lung Cancer Res 2022;11:509-22. [Crossref] [PubMed]
- Janik A, Torrente M, Costabello L, et al. Machine Learning-Assisted Recurrence Prediction for Early-Stage Non-Small-Cell Lung Cancer Patients. arXiv preprint arXiv:2211.09856. 2022. Available online: https://arxiv.org/abs/2211.09856
- Yu Q, Du X, Fang Z, et al. Predictive Risk Factors for Early Recurrence of Stage pIIIA-N2 Non-Small Cell Lung Cancer. Cancer Manag Res 2021;13:8651-61. [Crossref] [PubMed]
- Yuan X, Wang Z, Li C, et al. Bacterial biomarkers capable of identifying recurrence or metastasis carry disease severity information for lung cancer. Front Microbiol 2022;13:1007831. [Crossref] [PubMed]
- Liu X, Wu S, Yang Y, et al. The prognostic landscape of tumor-infiltrating immune cell and immunomodulators in lung cancer. Biomed Pharmacother 2017;95:55-61. [Crossref] [PubMed]
- Zhong J, Chen JM, Chen SL, et al. Constructing a Risk Prediction Model for Lung Cancer Recurrence by Using Gene Function Clustering and Machine Learning. Comb Chem High Throughput Screen 2019;22:266-75. [Crossref] [PubMed]
- Newman AM, Liu CL, Green MR, et al. Robust enumeration of cell subsets from tissue expression profiles. Nat Methods 2015;12:453-7. [Crossref] [PubMed]
- Kourou K, Exarchos TP, Exarchos KP, et al. Machine learning applications in cancer prognosis and prediction. Comput Struct Biotechnol J 2015;13:8-17. [Crossref] [PubMed]
- Vural S, Wang X, Guda C. Classification of breast cancer patients using somatic mutation profiles and machine learning approaches. BMC Syst Biol 2016;10:62. [Crossref] [PubMed]
- Yu L, Tao G, Zhu L, et al. Prediction of pathologic stage in non-small cell lung cancer using machine learning algorithm based on CT image feature analysis. BMC Cancer 2019;19:464. [Crossref] [PubMed]
- Uramoto H, Tanaka F. Recurrence after surgery in patients with NSCLC. Transl Lung Cancer Res 2014;3:242-9. [Crossref] [PubMed]
- Jeong WG, Choi H, Chae KJ, et al. Prognosis and recurrence patterns in patients with early stage lung cancer: a multi-state model approach. Transl Lung Cancer Res 2022;11:1279-91. [Crossref] [PubMed]
- Yang HC, Kim HR, Jheon S, et al. Recurrence Risk-Scoring Model for Stage I Adenocarcinoma of the Lung. Ann Surg Oncol 2015;22:4089-97. [Crossref] [PubMed]
- Anichini A, Tassi E, Grazia G, et al. The non-small cell lung cancer immune landscape: emerging complexity, prognostic relevance and prospective significance in the context of immunotherapy. Cancer Immunol Immunother 2018;67:1011-22. [Crossref] [PubMed]
- Karasaki T, Nagayama K, Kuwano H, et al. An Immunogram for the Cancer-Immunity Cycle: Towards Personalized Immunotherapy of Lung Cancer. J Thorac Oncol 2017;12:791-803. [Crossref] [PubMed]
- Chen F, Yang Y, Zhao Y, et al. Immune Infiltration Profiling in Nonsmall Cell Lung Cancer and Their Clinical Significance: Study Based on Gene Expression Measurements. DNA Cell Biol 2019;38:1387-401. [Crossref] [PubMed]
- Dong B, Wu C, Huang L, et al. Macrophage-Related SPP1 as a Potential Biomarker for Early Lymph Node Metastasis in Lung Adenocarcinoma. Front Cell Dev Biol 2021;9:739358. [Crossref] [PubMed]
- Liu J, Luo R, Wang J, et al. Tumor Cell-Derived Exosomal miR-770 Inhibits M2 Macrophage Polarization via Targeting MAP3K1 to Inhibit the Invasion of Non-small Cell Lung Cancer Cells. Front Cell Dev Biol 2021;9:679658. [Crossref] [PubMed]
- Liang L, He H, Jiang S, et al. TIAM2 Contributes to Osimertinib Resistance, Cell Motility, and Tumor-Associated Macrophage M2-like Polarization in Lung Adenocarcinoma. Int J Mol Sci 2022;23:10415. [Crossref] [PubMed]
- Altevogt P, Sammar M, Hüser L, et al. Novel insights into the function of CD24: A driving force in cancer. Int J Cancer 2021;148:546-59. [Crossref] [PubMed]
- Conway EM, Pikor LA, Kung SH, et al. Macrophages, Inflammation, and Lung Cancer. Am J Respir Crit Care Med 2016;193:116-30. [Crossref] [PubMed]
- Phu TA, Ng M, Vu NK, et al. IL-4 polarized human macrophage exosomes control cardiometabolic inflammation and diabetes in obesity. Mol Ther 2022;30:2274-97. [Crossref] [PubMed]
- Huang P, Illei PB, Franklin W, et al. Lung Cancer Recurrence Risk Prediction through Integrated Deep Learning Evaluation. Cancers (Basel) 2022;14:4150. [Crossref] [PubMed]
- Gale D, Heider K, Ruiz-Valdepenas A, et al. Residual ctDNA after treatment predicts early relapse in patients with early-stage non-small cell lung cancer. Ann Oncol 2022;33:500-10. [Crossref] [PubMed]
- Lepore M, Kalinichenko A, Calogero S, et al. Functionally diverse human T cells recognize non-microbial antigens presented by MR1. Elife 2017;6:e24476. [Crossref] [PubMed]
- Guo T, Chamoto K, Hirano N, Adoptive T. Cell Therapy Targeting CD1 and MR1. Front Immunol 2015;6:247. [Crossref] [PubMed]
- Song W, Wang Z, Kan P, et al. Knockdown of BCL6 Inhibited Malignant Phenotype and Enhanced Sensitivity of Glioblastoma Cells to TMZ through AKT Pathway. Biomed Res Int 2018;2018:6953506. [Crossref] [PubMed]
- Green MR, Vicente-Dueñas C, Romero-Camarero I, et al. Transient expression of Bcl6 is sufficient for oncogenic function and induction of mature B-cell lymphoma. Nat Commun 2014;5:3904. [Crossref] [PubMed]
- Marullo R, Ahn H, Cardenas M, et al. The transcription factor BCL6 is a rational target in non-small cell lung cancer (NSCLC). Cancer Res 2016;76:1271.
- Yang D, Zhou J, Zeng T, et al. Serum chemokine network correlates with chemotherapy in non-small cell lung cancer. Cancer Lett 2015;365:57-67. [Crossref] [PubMed]
- Okugawa Y, Toiyama Y, Tanaka K, et al. Elevated serum monocyte chemotactic protein 4 (MCP4) as a novel noninvasive prognostic and predictive biomarker for detection of metastasis in colorectal cancer. Cancer Res 2016;76:5016. [Crossref] [PubMed]
- Zhao S, Shen W, Yu J, et al. TBX21 predicts prognosis of patients and drives cancer stem cell maintenance via the TBX21-IL-4 pathway in lung adenocarcinoma. Stem Cell Res Ther 2018;9:89. [Crossref] [PubMed]
- Wu HH, Hwang-Verslues WW, Lee WH, et al. Targeting IL-17B-IL-17RB signaling with an anti-IL-17RB antibody blocks pancreatic cancer metastasis by silencing multiple chemokines. J Exp Med 2015;212:333-49. [Crossref] [PubMed]
- Bie Q, Sun C, Gong A, et al. Non-tumor tissue derived interleukin-17B activates IL-17RB/AKT/β-catenin pathway to enhance the stemness of gastric cancer. Sci Rep 2016;6:25447. [Crossref] [PubMed]
- Goto N, Fukuda A, Yamaga Y, et al. Lineage tracing and targeting of IL17RB(+) tuft cell-like human colorectal cancer stem cells. Proc Natl Acad Sci U S A 2019;116:12996-3005. [Crossref] [PubMed]
- Rousalova I, Krepela E. Granzyme B-induced apoptosis in cancer cells and its regulation Int J Oncol 2010;37:1361-78. (review). [Crossref] [PubMed]
- Dufait I, Pardo J, Escors D, et al. Perforin and Granzyme B Expressed by Murine Myeloid-Derived Suppressor Cells: A Study on Their Role in Outgrowth of Cancer Cells. Cancers (Basel) 2019;11:808. [Crossref] [PubMed]
- Yao C, Ni Z, Gong C, et al. Rocaglamide enhances NK cell-mediated killing of non-small cell lung cancer cells by inhibiting autophagy. Autophagy 2018;14:1831-44. [Crossref] [PubMed]