Microbiota modulate lung squamous cell carcinoma lymph node metastasis through microbiota-geneset correlation network
Highlight box
Key findings
• A novel microbiota-geneset network was developed for modulating the lymph node (LN) metastasis of lung squamous cell carcinoma (LUSC).
What is known and what is new?
• Surgery only provides a good postoperative prognosis for LUSCs without LNs or distant metastases. The tumor-resident microbiota is a newly discovered modulating strategy by reorganizing the migration activity of cancer cells.
• We successfully constructed a microbiota-geneset network to characterize the associations between microbiota and host genes of LUSC.
What is the implication, and what should change now?
• This finding provides a strongly correlation network for LUSC with LN metastasis and improve understanding of interaction between microbiota and the host, which may shed light on etiology of LUSC.
Introduction
Clinical and biological cancer studies have revealed that well-known genetics (1) and environmental exposure (2,3) are two of the main carcinogenic factors. Host-microbiota is another crucial mediator in modulating cancer initiation and progression (4-7). Intra-tumoral microbiota is present in at least 33 major cancer types (8), and its diversity can be distinct across various cancers (9). The co-localization of pan-bacterial markers and immune and epithelial-cell targets suggests that the intra-tumoral microbiota may be intracellular (8). It has already been reported that, in various types of cancer, tumor-resident microbiota is related to cancer risk (10), tumor progression (4,11-13), treatment response (14-16), and chemotherapy resistance (17). Recent studies have also revealed that distant metastasis can be regulated by microbiota; for example, intracellular microbiota can promote breast cancer metastasis by activating the RhoA-ROCK pathway and reorganizing the actin cytoskeleton (12). Fusobacterium nucleatum (18) was found to persist consistently between paired primary colorectal tumor and liver metastasis (19). However, for lung squamous cell carcinoma (LUSC), the specific ways and related signaling pathways in which tumor-resident microbiota affects cancer cells have not been fully revealed.
Lung cancer is classified into non-small cell lung cancer (NSCLC) and small-cell lung cancer, and 80% of lung cancers are NSCLC (20), with LUSC comprised of about 20–30% of NSCLC (21,22). LUSC is characterized by a high rate of genetic mutations and chromosomal instability (22,23). Unlike lung adenocarcinoma, LUSCs originate from the bronchial mucosa; most of the LUSCs are central type, growing along the proximal bronchus and invading large blood vessels (21). Most of the LUSCs are in stages IIIA, IIIB, or IV at the time of diagnosis. Thus, its prognosis is relatively poor. Surgical intervention is currently the mainstay of treatment for LUSCs, even for locally advanced diseases (24,25). However, the indications for surgical intervention remain controversial since many factors, such as the presence of lymph nodes (LNs) and distant metastases, can affect the outcome of surgery. In most cases, surgical intervention only provides a good postoperative prognosis for LUSCs without LNs and distant metastasis.
The lung is a mucosal tissue with the largest surface area in the body, and its mucosal surfaces are exposed to various airborne microbes and environmental insults through inhalation. Moreover, lung microbes’ characteristics vary between tissue/tumor types and partially depend on the microbiota-host interaction (26-28). The literature has a growing body of evidence linking lung microbiomes and NSCLC tumorigenesis. A previous study has confirmed that the diversity of tumor-resident microbiome is related to the clinical stage: compared with stage I–IIIA NSCLCs, the lower airway dysbiotic signature triggered by microbiota was more likely to present with those at advanced-stage (IIIB–IV) (11). In contrast to lung adenocarcinoma, the abundance of acidophilus was positively correlated with TP53 mutation in LUSCs (29).
The literature mentioned above indicates complex interactions between lung microbiota and gene mutations of LUSCs. However, so far, no studies have thoroughly investigated the relationship between tumor-resident microbes and LN metastasis, which is important for clinical decision-making and prediction of a patient’s prognosis. Herein, we aimed to compare the microbiota landscape in LUSC samples with (LN+) and without LN metastasis (LN−) to explore the microbiota biomarkers; and by analyzing multi-omics data, the microbiota-genesets network was built to investigate the underlying regulation signaling pathway of signature microbiota. This novel interactive microbiota-genesets network may serve as a candidate target in the early monitoring and treatment of LUSCs. We present this article in accordance with the STROBE and MDAR reporting checklists (available at https://tlcr.amegroups.com/article/view/10.21037/tlcr-23-357/rc).
Methods
Study design
RNA sequencing (RNA-seq) data of LUSC samples and their matched clinical and genomic information were obtained from the The Cancer Genome Atlas (TCGA) database. The matched proteome and microorganism quantification data were accessed via the cBioPortal database (http://www.cbioportal.org/), which includes the microbial reads generated by Poore et al., re-examining whole-genome and whole-transcriptome sequencing studies in TCGA by Cerami et al. (30,31). In Figure 1, we present a schematic representation of our analysis pipeline. For our comparative microbial analysis between lung cancer with and without LN metastasis, we included only LUSC patients without distant metastasis, i.e., TxNxM0 disease, to minimize confounding effects of pathological type, tumor size and advanced cancer stage on the intratumor microbiota composition (11,32). Samples without available microbial information were excluded. Accordingly, eligible patients were classified into the positive LN metastasis (LN+) group (n=165), defined as the T ≥ 1N > 0M0 disease and negative LN metastasis (LN−) group (n=296), defined as T ≥ 1N0M0 disease. Consent from patients was waived since all data for this study were derived from publicly available data. Based on their quantification, we crossed microorganism abundance and gene expression data at the aliquot level to ensure biological comparability between the datasets (33). The study was conducted in accordance with the Declaration of Helsinki (as revised in 2013).
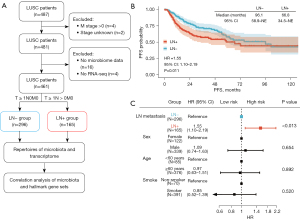
Somatic mutation and mutational signature analysis
Somatic mutations were identified using MuTect2 and summarized by the MAFtools (34). The decomposition of mutational signatures was performed using deconstructSigs (35) with default parameters based on the set of 30 mutational signatures (36) for samples. Cosine similarity analysis (36) was used to measure the similarity between components and signatures, which ranged from 0 to 1, indicating maximal dissimilarity to maximal similarity.
Transcriptome analysis
We used level three pre-processed gene expression data. Briefly, we aligned RNA-seq data by STAR (37), and mapped reads to each gene using HT-SEQ (38). We normalized the read counts using the fragments per kilobase of transcript per million mapped reads (FPKM) calculation, which divides counts by the gene length and the total number of reads mapped to protein-coding genes.
Gene set enrichment analysis (GSEA)
To elucidate expression profiles of hallmark genes during tumor metastasis and progression, we performed GSEA (39) by using the FPKM matrix of genes using clusterProfiler (40). The normalized enrichment scores (NES) of hallmark pathway gene sets were calculated by projecting the matrix of signed multi-omic feature weights (Wsigned) (41). The GSEA workflow was performed with the following parameters: gene.set.database = “h.all.v7.0.symbols.gmt”, sample.norm.type = “rank”, weight =1, statistic = “area.under.RES”, output.score.type = “NES”, nperm =1,000, global.FDR = TRUE, min.overlap =5, correl.type = “z.score”. The differential gene sets obtained from GSEA were used for subsequent microbiota-geneset network construction.
Immune infiltration
Immune infiltration, based on 22 distinct immune cell types, represents the immune landscape of the cancer microenvironment. CIBERSORT (42) was employed to analyze the immune landscape based on the TCGA RNA-seq dataset. The analysis was conducted with 1,000 permutations. The CIBERSORT values generated were defined as immune cell infiltration fraction per sample. Immune and matrix scores were calculated using the ESTIMATE package (43). The LinkET package was used to visualize the degree of correlation between representative microbiota and immune cell infiltration. The immunological scores were measured by five indexes, including cytolytic activity, stromal score, immune score, tumor purity, and ESTIMATE score.
Microbiota abundance and diversity
We used the public microbiota dataset generated by Poore et al. as microorganism abundance data (31). To assess microbial diversity, we extracted bacterial species’ abundance and taxonomic information from the microbial abundance dataset. The microbiota counts, sample metadata, and taxonomy information were imported using the Microeco toolkit (44). The abundant taxon was shown using a stacked bar plot (individual samples and group averages).
For diversity measures, between-group differences in the α-diversity indices (Shannon, Fisher, Simpson, and InvSimpson) and β-diversity (principal-coordinate analysis based on the Bray-Curtis dissimilarity distances) were assessed using one-way analysis of variance (ANOVA) and PERMANOVA (a non-parametric test similar to ANOVA) tests, respectively. LEfSe (45) was used to determine the bacterial groups with significant differences [|linear discriminant analysis (LDA) scores| >3, P<0.05] in abundance from phylum to genus level between the LN+ and LN− groups. The bacterial biomarkers were imported into the follow-up association network.
Microbe-geneset network
To show the connection between microbiota and hallmark, we assessed the correlation between the hallmark genesets and the bacterial biomarkers (genus level) using the cal_cor function of the Microeco (44). Moreover, the correlation was characterized by Spearman. Significant microbe-gene correlation (Cor >0.2, q value <0.05) connections were imported as input data of the correlative network. Gephi software was used to construct a representative microbe-gene network. Cytoscape V3.5.1 software (46) was used to edit the network visually. The size of the visual element indicated the degree of a node. Red and blue lines indicate positive and negative correlations in networks.
Statistical analyses
All statistical analyses were performed using the R program (version 3.6.3). Log-rank tests were used to compare survival distributions in Kaplan-Meier plots. For the baseline characteristic description, binary variables were presented as frequencies and percentages, and the numerical variables as the median and interquartile range. These two variables between LN+ and LN− groups were compared with the Pearson chi-squared (or Fisher exact) and Wilcoxon rank sum test, as appropriate. Log-rank tests were used to compare survival distributions in Kaplan-Meier plots. Significant differences were stated if the two-sided P value <0.05.
Results
Characteristics of the LN+ and LN− patients
According to the schematic representation (Figure 1A), 487 LUSC patients were evaluated for inclusion according to the selection criteria, and 461 patients in total were finally included, with 165 patients in the LN+ group and 296 patients in the LN− group (Figure 1A). The median age of the patients was 68 years. Forty-one patients (25%) over 60 years old were in the LN+ group, which was significantly higher than that in the LN− group. Most baseline characteristics were generally balanced between the groups, including sex, smoke, race, neoadjuvant, radiation (Table 1). We observed that LN metastasis resulted in a shorter progression-free survival (PFS) [hazard ratio (HR): 1.55, 95% confidence interval (CI): 1.10–2.19; P=0.011] (Figure 1B,1C). The median overall survival (OS) was 48.3 months in the LN+ group and 60.5 months in the LN− group (Figure S1), as well as the higher rate of 3-year OS and 5-year OS in the LN− group, representing a similar trend to the PFS.
Table 1
Characteristics | Overall (n=461) | LN+ (n=165) | LN− (n=296) | P value† |
---|---|---|---|---|
Age, n [%] | 0.008 | |||
<60 years | 85 [18] | 41 [25] | 44 [15] | |
≥60 years | 376 [82] | 124 [75] | 252 [85] | |
Sex, n [%] | 0.7 | |||
Female | 122 [26] | 42 [25] | 80 [27] | |
Male | 339 [74] | 123 [75] | 216 [73] | |
Smoke, n [%] | 0.8 | |||
No-smoker | 70 [15] | 26 [16] | 44 [15] | |
Smoker | 391 [85] | 139 [84] | 252 [85] | |
Race, n [%] | 0.079 | |||
Asian | 9 [2.0] | 4 [2.4] | 5 [1.7] | |
Black or African American | 28 [6.1] | 10 [6.1] | 18 [6.1] | |
Not reported | 97 [21.0] | 45 [27.3] | 52 [17.6] | |
White | 327 [70.9] | 106 [64.2] | 221 [74.7] | |
Neoadjuvant, n [%] | 0.8 | |||
No | 454 [98.5] | 163 [98.8] | 291 [98.3] | |
Unknown | 2 [0.4] | 1 [0.6] | 1 [0.3] | |
Yes | 5 [1.1] | 1 [0.6] | 4 [1.4] | |
New tumor event, n [%] | 0.3 | |||
No | 255 [55] | 92 [56] | 163 [55] | |
Unknown | 92 [20] | 38 [23] | 54 [18] | |
Yes | 114 [25] | 35 [21] | 79 [27] | |
Radiation, n [%] | 0.2 | |||
No | 350 [76] | 126 [76] | 224 [76] | |
Unknown | 61 [13] | 26 [16] | 35 [12] | |
Yes | 50 [11] | 13 [7.9] | 37 [13] |
†, Pearson’s Chi-squared test. LN, lymph node.
Microbiota diversity in the LN+ and LN− patients
To evaluate the effects of LN+/LN− status on α-diversity, we examined measures of Shannon, Fisher, Simpson, and InvSimpson index (Figure 2A) and discovered no significant difference between groups. At the same time, unsupervised principal component analysis (PCA) of Bray-Curtis distances (β-diversity) displayed no significant differences (P=0.471) (Figure 2B). The discriminative analysis of microbiota abundance did not identify major taxa differences. The microbiota diversity was similar between the LN+ and LN− groups. We then investigated the relative abundances in each group at various taxonomic levels. At the genus level, Terrabacter (LN+: 22.21%, LN−: 24.25%), Bacteroides (LN+: 11.75%, LN−: 11.69%), Neisseria (LN+: 8.05%, LN−: 7.24%), Listeria (LN+: 4.55%, LN−: 5.06%), and Proteus (LN+: 5.99%, LN−: 5.96%) at the genus level (Figure 2C) were predominant in our included samples. From phylum to genus, we observed no significant difference in the abundance of the top 5 microbiota (Figure 2D).
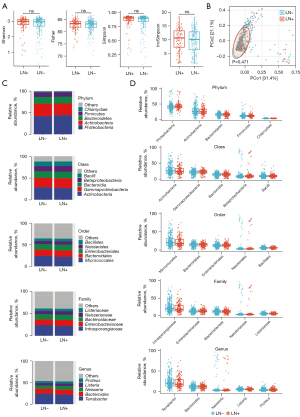
To discover high-dimensional biomarkers, the LEfSe software was applied to identify predominant bacterial taxa associated with different clinical characteristics. We found significant differences in two genera, in which Terrabacter was more abundant in the LN− group relative to the LN+ group (Figure 3A,3B). Contrarily, Neisseria, Bordetella, Shigella, Lactobacillus, Proteus, Aeromonas, and Bacteroides were significantly more abundant in LN+ than in the LN− group (Figure 3A,3B). At the phylum level, Proteobacteria and Bacteroidota were more abundant in the LN+ group relative to the LN− group (Figure S2). On the other hand, Actinobacteria and Firmicutes were significantly more abundant in LN− than in the LN+ group (Figure S2).
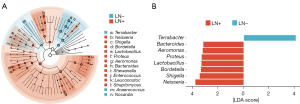
Similar mutation profiles and mutational signatures are shared by LN+ and LN− group
To better understand the association between the mutation landscape and microbiota profiles, we called a total of 156,229 single nucleotide variants (SNVs), 4,559 insertion and deletion (InDel) and 2,039 insertions. The most frequent genes were TP53 (79%), TTN (72%), CSMD3 (42%), MUC16 (39%), and RYR2 (36%) (Figure 4A). There were more ROS1 mutations in the LN− group than in the LN+ group (Figure 4B), but the mutation frequency was low. The others generally known NSCLC driver genes mutation (47) was not found to be associated with LN metastases (Figure 4B). Therefore, the mutation profiles of LN+ and LN− are similar.
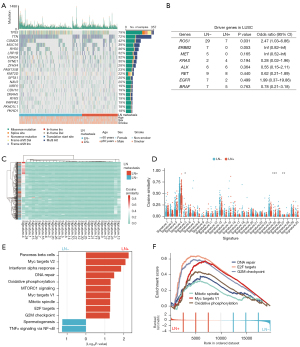
Previous studies have identified more than 30 single-base substitutions (SBS) signatures, some of which are common in lung cancer, for example, ‘aging’ (Signature 1), ‘smoking’ (Signature 4), and ‘APOBEC’ (Signature 13) (48). We performed a systematic identification using the nonnegative matrix factorization algorithm. Signature 4 showed a high cosine similarity value in the LN+ and LN− patients (Figure 4C,4D). However, all three common mutational signatures, including Signature 1, Signature 4, and Signature 13, did not differ significantly between the LN+ and LN−. However, the Signature 5, Signature 25, and Signature 27 showed significant differences; considering the low cosine similarity overall, these signatures might not affect the outcome. Thus, the differential microbiota biomarkers of LN metastases may not be due to the contribution of gene mutations or mutational signatures.
Upregulation of gene sets related to tumor development, cell cycle, and energy metabolism in LN metastases
Recently, a study reported that the bacteria affect host transcript profiles (49). We comprehensively investigated transcriptomes between LN+ and LN− groups. Two hundred and fifteen differentially expressed genes were identified (Figure S3A). Further, the GSEA showed that ten gene sets were enriched in the LN+ group, and two gene sets were enriched in the LN− group (Figure 4E). The highly expressed genes were significantly enriched in the Myc Targets, E2F Targets, G2M Checkpoint, Mitotic Spindle, DNA Repair, and Oxidative Phosphorylation (Figure 4F). According to oncology function, the enriched gene sets are related to tumor development (Myc Targets, E2F Targets), cell cycle (G2M Checkpoint, Mitotic Spindle), energy metabolism (Oxidative Phosphorylation) and DNA Repair. In addition, PCA clustering based on the expression profiles of the enriched gene sets showed a significant difference between LN+ and LN− groups (Figure S3B). No significant differences were observed in the epithelial-mesenchymal transition protein markers (Figure S3C,S3D).
LN metastases induce higher inflammatory response status for the tumor microenvironment
In order to better distinguish the immune characteristics of LN+ and LN− groups, we characterized the immune infiltration profile using a gene expression matrix. The results showed no significant difference in five immunological scores between LN+ and LN− (Figure 5A). Regarding immune cell composition, the LN+ was characterized by a higher inflammatory response status, which was significantly increased in M0 macrophages (Figure 5B).
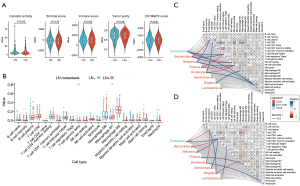
To explore the potential correlation between tumor immune infiltration status and microbiota abundance, we performed the association analysis of immune infiltration and microbiota for LN+ and LN− groups, respectively. We found that macrophage M0 was correlated with Shigella (Figure 5C). We also found that CD8 T cells were correlated with Bacteroides in the LN+ group (Figure 5C). In the meantime, as presented in Figure 5D, the M0 macrophages were associated with Shigella in the LN− group. In summary, these results indicated that a higher inflammatory response status of the LN+ group is potentially correlated with microbiota biomarkers in the LN+ group.
Proteus and Bacteroides negatively correlated with transcripts of energy metabolism and migration-related gene sets
The up-regulated gene sets and microbial markers were identified as significant differences between the LN− and LN+. Therefore, we evaluated the associations between co-occurring taxa and hallmark gene sets. Generally, the microbiota in LN+ and LN− networks were associated with gene sets related to Myc Targets, E2F Targets, G2M Checkpoint, Mitotic Spindle, Oxidative Phosphorylation, and DNA Repair (Figure S4). Compared with the LN− network, two genera exhibited an increasing degree in the LN+ network. Firstly, Proteus and Bacteroides were positively correlated with gene sets, including Oxidative Phosphorylation, Myc Targets, and E2F Targets in the LN+ network (Figure 6A, table available at https://cdn.amegroups.cn/static/public/tlcr-23-357-1.xlsx). However, the LN− network did not show strong connections (Figure 6B, table available at https://cdn.amegroups.cn/static/public/tlcr-23-357-2.xlsx). The LN+ microbiota biomarkers, for example, Proteus and Bacteroides, were positively correlated with gene sets related to tumor development and energy metabolism, which potentially promote LN metastasis.
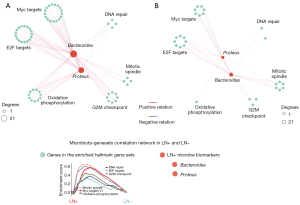
Discussion
To elucidate the role of tumor-resident microbiota in LN metastasis, the present study compared the microbiota profiles of 296 LN− LUSCs samples and 165 LN+ LUSCs samples in the TCGA-LUSC cohort (table available at https://cdn.amegroups.cn/static/public/tlcr-23-357-3.xlsx); and proposed that Proteus and Bacteroides as signature microbiota play crucial roles in LUSCs LN metastasis by correlating the active hallmark gene sets (Figure 6A). Finally, with the analysis of the association between immune infiltration and microbiota abundance in the LN+ and LN− groups, respectively, it was confirmed that the higher inflammatory response status in the LN+ group may be associated with the microbiome markers. To the best of our knowledge, this is the innovative study to identify the relationship between the microbiome and LN metastasis of squamous cell lung carcinoma.
Previously, most studies exploring microbes’ role in cancer development and progression have focused on gut microbes (50). It has been proved that tumor-resident bacteria can modulate local tumor progression independently of the gut microbiome (51). Various reasons can lead to easier colonization of bacteria in tumor tissues (52). For example, (I) tumor blood vessels have incomplete structure and abnormal function compared with normal blood vessels, which may allow circulating bacteria in the blood to enter the tumor tissue; (II) the tumor microenvironment is in an immunosuppressive state, allowing the microbiome to proliferate within the tumor without being easily eliminated by the immune system; (III) most solid tumors are hypoxic internally, providing an environment suitable for the proliferation of facultative and anaerobic bacteria. Previous studies have confirmed that microbiota can activate carcinogenic signaling pathways by directly inducing DNA damage and mutagenesis (53,54) or interacting with the host immune microenvironment (55), thereby affecting tumor genesis and tumor progression. For example, Escherichia coli and Staphylococcus epidermidis in the mammary can promote breast carcinogenesis in susceptible individuals by causing double-stranded DNA breaks in host cells (56). Moreover, Fusobacterium can contribute to tumorigenesis by creating a chronic proinflammatory environment (57). In addition, several known metabolites of microbiota, such as reactive oxygen species, bile acids, butyrate, hydrogen sulfide, and N-nitroso compounds (58) have also been shown to interfere with immune responses, causing cellular inflammation, and induce tumorigenesis (51).
Molecularly, LUSC exhibited a high rate of genomic mutations and structure variants (22,23). Unlike lung adenocarcinoma, LUSCs have high mortality and often derive less benefit from targeted therapy; therefore, surgical treatment, chemotherapy, and immune checkpoint inhibitors are the main treatment strategies for LUSCs (22). In most cases, surgical intervention only provides a good prognosis for LUSCs without LNs and distant metastasis. LN metastasis has also been reported to affect the efficacy of definitive radiotherapy for stage III NSCLC (59). Therefore, it is of great clinical value to explore the influencing factors of LN metastasis in LUSCs. Considering that tumor-resident microbiomes have been reported to be closely associated with TP53 mutation (29) as well as tumor progression (4,11,12) in LUSCs, it is reasonable to hypothesize that there may be a certain relationship between microbiota and LN metastasis of LUSCs.
In the present study, we found that tumor-resident microbiota existed in both LN− and LN+ LUSCs samples (Figure 2C,2D), indicating that it is more likely to be an inherent component of tumor tissues. This is consistent with the previous literature that intra-tumor microbiota can persist during tumor metastasis and passage in breast cancer and colorectal cancer (12,19). Furthermore, the distinct microbiota profiles of LN− LUSC and LN+ LUSC samples allowed us to explore further the underlying mechanism of LN metastasis.
For most solid tumors, LNs are generally the first location of metastasis. LN colonization can also induce tumor-specific immune tolerance that renders distant tissues amenable to metastatic colonization (60). In general, LN metastasis is a multistep process involving the following four steps: VEGF-C/VEGF-D/VEGFR-3 axis-regulated tumor-associated lymph angiogenesis in the vicinity of tumors, chemokine-assisted migration of tumor cells to lymphatic vessels, tumor-induced lymph angiogenesis in LNs, and changes in the morphology and function of blood vessels and lymphatic vessels in LNs (61,62). Here, we elucidated the process of LN metastasis from a new perspective: through the microbiota-geneset network, tumor-resident microbiota promotes the upregulation of cell cycle and energy metabolism pathways, which enhance tumor cell activity and possibly associated with LN metastasis (Figure 3E,3F). Recent studies also support the view that microbiota regulates tumor cell viability by affecting energy metabolism pathways (63,64). More importantly, we demonstrated that in LN+ LUSC samples, the associations between microbiota biomarkers, Proteus and Bacteroides, and hallmark gene sets involving tumor cell development and energy metabolism are more diverse. This is also supported by the microbiota-transcript co-occurrences analysis in stage IIIB–IV NSCLC (11). Our results further presented transcriptomic clues that provide insights into the tumor development and energy metabolism-related gene sets associated with LN metastasis.
By constructing the microbiota-geneset network, we found that Proteus and Bacteroides were closely correlated with LN metastasis of LUSCs. Recent studies have also suggested their association with tumor occurrence, development and metastasis. In gastric cancer, compared with the control group and gastric antrum tissues with intestinal metaplasia, Proteobacteria (especially Proteus) were enriched, and Bacteroidetes (especially S24-7 family) were absent in mucosa of the early-stage gastric cancer group (65). Changes in the abundance of phylum Proteus have also been reported to be significantly associated with gastric cancer development (66,67). Moreover, Proteus mirabilis was associated with LN metastasis in human papillomavirus (HPV)-positive oropharyngeal squamous cell carcinoma (68). This conclusion is consistent with that of the present study. However, this study did not propose the possible mechanism by which Proteus may affect LN metastasis. In colorectal cancer, Bacteroidetes were further divided into two clusters. The abundance of Bacteroidetes Cluster 1 was decreased in colorectal cancer mucosa, while Cluster 2 was increased. The colorectal cancer-associated commensal Bacteroidetes have also been shown to be significantly associated with the expression of host immune-inflammatory genes (69). This literature further supports the conclusions of the present study.
Our study has several numbers of limitations: firstly, tumor-resident commensal microflora can be seen as a complex cellular system, and this study failed to elucidate the complex interrelationships among them; secondly, the baseline characteristic of LN− and LN+ patients were not completely matched (Table 1 and table available at https://cdn.amegroups.cn/static/public/tlcr-23-357-3.xlsx), although we had compared the characteristics of SNV, InDel and insertion mutations and the smoking and age signature between the two groups, the selection bias cannot be excluded; thirdly, the molecular mechanism of how bacteria modulated the transcripts of these enriched hallmark gene sets remains unclear; finally, low levels of the bacterial contaminate are not be able to exclude through this database perfectly, the precise experiments are needed to verify microbe-host interactions.
To sum up, we used a multi-omics approach to analyze the relationship between tumor-resident microbiota and LN metastasis of LUSCs, and we constructed a co-occurrence network connecting diverse microbe spectra in tumor niches and cancer hallmark gene sets. The next step of this study is to understand the mechanisms of how bacteria modulated the transcript level of these hallmark gene set. We plan to use a mouse model with LN metastasis (60) to explore the homeostasis characteristics of bacteria represented by Proteus and Bacteroides in and out of tumor cells, to analyze whether the disorder of tumor microbiota can induce tumor-specific immune tolerance that eventually drives the process of LN metastasis. The answers to these questions will help to promote the application of bacterial-host cell interaction in cancer therapy.
Conclusions
In conclusion, our results revealed the distinct repertoires of tumor-resident microbiota and transcript in LN− and LN+ samples of LUSC. The Proteus and Bacteroides are closely correlated with the highly expressed gene sets involved in tumor development and energy metabolism, which might contribute to LN metastasis. These findings can provide theoretical support for the future application of antimicrobial-based cancer treatment.
Acknowledgments
Funding: This study was supported by
Footnote
Reporting Checklist: The authors have completed the STROBE and MDAR reporting checklists. Available at https://tlcr.amegroups.com/article/view/10.21037/tlcr-23-357/rc
Peer Review File: Available at https://tlcr.amegroups.com/article/view/10.21037/tlcr-23-357/prf
Conflicts of Interest: All authors have completed the ICMJE uniform disclosure form (available at https://tlcr.amegroups.com/article/view/10.21037/tlcr-23-357/coif). W.L. is serving as an Associate Editor-in-Chief of Translational Lung Cancer Research from May 2023 to April 2024. The other authors have no conflicts of interest to declare.
Ethical Statement: The authors are accountable for all aspects of the work in ensuring that questions related to the accuracy or integrity of any part of the work are appropriately investigated and resolved. The study was conducted in accordance with the Declaration of Helsinki (as revised in 2013).
Open Access Statement: This is an Open Access article distributed in accordance with the Creative Commons Attribution-NonCommercial-NoDerivs 4.0 International License (CC BY-NC-ND 4.0), which permits the non-commercial replication and distribution of the article with the strict proviso that no changes or edits are made and the original work is properly cited (including links to both the formal publication through the relevant DOI and the license). See: https://creativecommons.org/licenses/by-nc-nd/4.0/.
References
- Ishaque N, Abba ML, Hauser C, et al. Whole genome sequencing puts forward hypotheses on metastasis evolution and therapy in colorectal cancer. Nat Commun 2018;9:4782. [Crossref] [PubMed]
- Kucab JE, Zou X, Morganella S, et al. A Compendium of Mutational Signatures of Environmental Agents. Cell 2019;177:821-836.e16. [Crossref] [PubMed]
- Lee JJ, Park S, Park H, et al. Tracing Oncogene Rearrangements in the Mutational History of Lung Adenocarcinoma. Cell 2019;177:1842-1857.e21. [Crossref] [PubMed]
- Riquelme E, Zhang Y, Zhang L, et al. Tumor Microbiome Diversity and Composition Influence Pancreatic Cancer Outcomes. Cell 2019;178:795-806.e12. [Crossref] [PubMed]
- Jin C, Lagoudas GK, Zhao C, et al. Commensal Microbiota Promote Lung Cancer Development via γδ T Cells. Cell 2019;176:998-1013.e16. [Crossref] [PubMed]
- Xavier JB, Young VB, Skufca J, et al. The Cancer Microbiome: Distinguishing Direct and Indirect Effects Requires a Systemic View. Trends Cancer 2020;6:192-204. [Crossref] [PubMed]
- Roelands J, Kuppen PJK, Ahmed EI, et al. An integrated tumor, immune and microbiome atlas of colon cancer. Nat Med 2023;29:1273-86. [Crossref] [PubMed]
- Kalaora S, Nagler A, Nejman D, et al. Identification of bacteria-derived HLA-bound peptides in melanoma. Nature 2021;592:138-43. [Crossref] [PubMed]
- Nejman D, Livyatan I, Fuks G, et al. The human tumor microbiome is composed of tumor type-specific intracellular bacteria. Science 2020;368:973-80. [Crossref] [PubMed]
- Hong S, Mok Y, Jeon C, et al. Tuberculosis, smoking and risk for lung cancer incidence and mortality. Int J Cancer 2016;139:2447-55. [Crossref] [PubMed]
- Tsay JJ, Wu BG, Sulaiman I, et al. Lower Airway Dysbiosis Affects Lung Cancer Progression. Cancer Discov 2021;11:293-307. [Crossref] [PubMed]
- Fu A, Yao B, Dong T, et al. Tumor-resident intracellular microbiota promotes metastatic colonization in breast cancer. Cell 2022;185:1356-1372.e26. [Crossref] [PubMed]
- Galeano Niño JL, Wu H, LaCourse KD, et al. Effect of the intratumoral microbiota on spatial and cellular heterogeneity in cancer. Nature 2022;611:810-7. [Crossref] [PubMed]
- Yu T, Guo F, Yu Y, et al. Fusobacterium nucleatum Promotes Chemoresistance to Colorectal Cancer by Modulating Autophagy. Cell 2017;170:548-563.e16. [Crossref] [PubMed]
- Wang M, Rousseau B, Qiu K, et al. Killing tumor-associated bacteria with a liposomal antibiotic generates neoantigens that induce anti-tumor immune responses. Nat Biotechnol 2023; Epub ahead of print. [Crossref]
- Li Y, Xing S, Chen F, et al. Intracellular Fusobacterium nucleatum infection attenuates antitumor immunity in esophageal squamous cell carcinoma. Nat Commun 2023;14:5788. [Crossref] [PubMed]
- Geller LT, Barzily-Rokni M, Danino T, et al. Potential role of intratumor bacteria in mediating tumor resistance to the chemotherapeutic drug gemcitabine. Science 2017;357:1156-60. [Crossref] [PubMed]
- Kostic AD, Chun E, Robertson L, et al. Fusobacterium nucleatum potentiates intestinal tumorigenesis and modulates the tumor-immune microenvironment. Cell Host Microbe 2013;14:207-15. [Crossref] [PubMed]
- Bullman S, Pedamallu CS, Sicinska E, et al. Analysis of Fusobacterium persistence and antibiotic response in colorectal cancer. Science 2017;358:1443-8. [Crossref] [PubMed]
- Duma N, Santana-Davila R, Molina JR. Non-Small Cell Lung Cancer: Epidemiology, Screening, Diagnosis, and Treatment. Mayo Clin Proc 2019;94:1623-40. [Crossref] [PubMed]
- Socinski MA, Obasaju C, Gandara D, et al. Current and Emergent Therapy Options for Advanced Squamous Cell Lung Cancer. J Thorac Oncol 2018;13:165-83. [Crossref] [PubMed]
- Lau SCM, Pan Y, Velcheti V, et al. Squamous cell lung cancer: Current landscape and future therapeutic options. Cancer Cell 2022;40:1279-93. [Crossref] [PubMed]
- Sánchez-Danés A, Blanpain C. Deciphering the cells of origin of squamous cell carcinomas. Nat Rev Cancer 2018;18:549-61. [Crossref] [PubMed]
- Takamochi K, Tsuboi M, Okada M, et al. S-1 + Cisplatin with Concurrent Radiotherapy Followed by Surgery for Stage IIIA (N2) Lung Squamous Cell Carcinoma: Results of a Phase II Trial. Ann Surg Oncol 2022;29:8198-206. [Crossref] [PubMed]
- Zhou Q, Shi Y, Chen J, et al. Long-term survival of personalized surgical treatment of locally advanced non-small cell lung cancer based on molecular staging. Zhongguo Fei Ai Za Zhi. 2011;14:86-106. [Crossref] [PubMed]
- Hosgood HD 3rd, Sapkota AR, Rothman N, et al. The potential role of lung microbiota in lung cancer attributed to household coal burning exposures. Environ Mol Mutagen 2014;55:643-51. [Crossref] [PubMed]
- Marsland BJ, Gollwitzer ES. Host-microorganism interactions in lung diseases. Nat Rev Immunol 2014;14:827-35. [Crossref] [PubMed]
- Yu G, Gail MH, Consonni D, et al. Characterizing human lung tissue microbiota and its relationship to epidemiological and clinical features. Genome Biol 2016;17:163. [Crossref] [PubMed]
- Greathouse KL, White JR, Vargas AJ, et al. Interaction between the microbiome and TP53 in human lung cancer. Genome Biol 2018;19:123. [Crossref] [PubMed]
- Cerami E, Gao J, Dogrusoz U, et al. The cBio cancer genomics portal: an open platform for exploring multidimensional cancer genomics data. Cancer Discov 2012;2:401-4. [Crossref] [PubMed]
- Poore GD, Kopylova E, Zhu Q, et al. Microbiome analyses of blood and tissues suggest cancer diagnostic approach. Nature 2020;579:567-74. [Crossref] [PubMed]
- Gomes S, Cavadas B, Ferreira JC, et al. Profiling of lung microbiota discloses differences in adenocarcinoma and squamous cell carcinoma. Sci Rep 2019;9:12838. [Crossref] [PubMed]
- Uriarte-Navarrete I, Hernández-Lemus E, de Anda-Jáuregui G. Gene-Microbiome Co-expression Networks in Colon Cancer. Front Genet 2021;12:617505. [Crossref] [PubMed]
- Mayakonda A, Lin DC, Assenov Y, et al. Maftools: efficient and comprehensive analysis of somatic variants in cancer. Genome Res 2018;28:1747-56. [Crossref] [PubMed]
- Rosenthal R, McGranahan N, Herrero J, et al. DeconstructSigs: delineating mutational processes in single tumors distinguishes DNA repair deficiencies and patterns of carcinoma evolution. Genome Biol 2016;17:31. [Crossref] [PubMed]
- Alexandrov LB, Nik-Zainal S, Wedge DC, et al. Signatures of mutational processes in human cancer. Nature 2013;500:415-21. [Crossref] [PubMed]
- Dobin A, Davis CA, Schlesinger F, et al. STAR: ultrafast universal RNA-seq aligner. Bioinformatics 2013;29:15-21. [Crossref] [PubMed]
- Anders S, Pyl PT, Huber W. HTSeq--a Python framework to work with high-throughput sequencing data. Bioinformatics 2015;31:166-9. [Crossref] [PubMed]
- Subramanian A, Tamayo P, Mootha VK, et al. Gene set enrichment analysis: a knowledge-based approach for interpreting genome-wide expression profiles. Proc Natl Acad Sci U S A 2005;102:15545-50. [Crossref] [PubMed]
- Yu G, Wang LG, Han Y, et al. clusterProfiler: an R package for comparing biological themes among gene clusters. OMICS 2012;16:284-7. [Crossref] [PubMed]
- Liberzon A, Birger C, Thorvaldsdóttir H, et al. The Molecular Signatures Database (MSigDB) hallmark gene set collection. Cell Syst 2015;1:417-25. [Crossref] [PubMed]
- Newman AM, Liu CL, Green MR, et al. Robust enumeration of cell subsets from tissue expression profiles. Nat Methods 2015;12:453-7. [Crossref] [PubMed]
- Yoshihara K, Shahmoradgoli M, Martínez E, et al. Inferring tumour purity and stromal and immune cell admixture from expression data. Nat Commun 2013;4:2612. [Crossref] [PubMed]
- Liu C, Cui Y, Li X, et al. microeco: an R package for data mining in microbial community ecology. FEMS Microbiol Ecol 2021;97:fiaa255. [Crossref] [PubMed]
- Segata N, Izard J, Waldron L, et al. Metagenomic biomarker discovery and explanation. Genome Biol 2011;12:R60. [Crossref] [PubMed]
- Shannon P, Markiel A, Ozier O, et al. Cytoscape: a software environment for integrated models of biomolecular interaction networks. Genome Res 2003;13:2498-504. [Crossref] [PubMed]
- Wu X, Zhao J, Yang L, et al. Next-generation Sequencing Reveals Age-dependent Genetic Underpinnings in Lung adenocarcinoma. J Cancer 2022;13:1565-72. [Crossref] [PubMed]
- Alexandrov LB, Kim J, Haradhvala NJ, et al. The repertoire of mutational signatures in human cancer. Nature 2020;578:94-101. [Crossref] [PubMed]
- Xue C, Jia J, Gu X, et al. Intratumoral bacteria interact with metabolites and genetic alterations in hepatocellular carcinoma. Signal Transduct Target Ther 2022;7:335. [Crossref] [PubMed]
- Alhinai EA, Walton GE, Commane DM. The Role of the Gut Microbiota in Colorectal Cancer Causation. Int J Mol Sci 2019;20:5295. [Crossref] [PubMed]
- Zhang W, An Y, Qin X, et al. Gut Microbiota-Derived Metabolites in Colorectal Cancer: The Bad and the Challenges. Front Oncol 2021;11:739648. [Crossref] [PubMed]
- Mohammad NS, Nazli R, Zafar H, et al. Effects of lipid based Multiple Micronutrients Supplement on the birth outcome of underweight pre-eclamptic women: A randomized clinical trial. Pak J Med Sci 2022;38:219-26. [Crossref] [PubMed]
- Ramírez-Labrada AG, Isla D, Artal A, et al. The Influence of Lung Microbiota on Lung Carcinogenesis, Immunity, and Immunotherapy. Trends Cancer 2020;6:86-97. [Crossref] [PubMed]
- Garrett WS. Cancer and the microbiota. Science 2015;348:80-6. [Crossref] [PubMed]
- Belkaid Y, Hand TW. Role of the microbiota in immunity and inflammation. Cell 2014;157:121-41. [Crossref] [PubMed]
- Hieken TJ, Chen J, Hoskin TL, et al. The Microbiome of Aseptically Collected Human Breast Tissue in Benign and Malignant Disease. Sci Rep 2016;6:30751. [Crossref] [PubMed]
- Urbaniak C, Gloor GB, Brackstone M, et al. The Microbiota of Breast Tissue and Its Association with Breast Cancer. Appl Environ Microbiol 2016;82:5039-48. [Crossref] [PubMed]
- Fang Y, Yan C, Zhao Q, et al. The roles of microbial products in the development of colorectal cancer: a review. Bioengineered 2021;12:720-35. [Crossref] [PubMed]
- Fernandes AT, Mitra N, Xanthopoulos E, et al. The impact of extent and location of mediastinal lymph node involvement on survival in Stage III non-small cell lung cancer patients treated with definitive radiotherapy. Int J Radiat Oncol Biol Phys 2012;83:340-7. [Crossref] [PubMed]
- Reticker-Flynn NE, Zhang W, Belk JA, et al. Lymph node colonization induces tumor-immune tolerance to promote distant metastasis. Cell 2022;185:1924-1942.e23. [Crossref] [PubMed]
- Csanadi A, Oser A, Aumann K, et al. Overexpression of SLC1a5 in lymph node metastases outperforms assessment in the primary as a negative prognosticator in non-small cell lung cancer. Pathology 2018;50:269-75. [Crossref] [PubMed]
- Achen MG, Stacker SA. Molecular control of lymphatic metastasis. Ann N Y Acad Sci 2008;1131:225-34. [Crossref] [PubMed]
- Garcia-Etxebarria K, Clos-Garcia M, Telleria O, et al. Interplay between Genome, Metabolome and Microbiome in Colorectal Cancer. Cancers (Basel) 2021;13:6216. [Crossref] [PubMed]
- Łaniewski P, Ilhan ZE, Herbst-Kralovetz MM. The microbiome and gynaecological cancer development, prevention and therapy. Nat Rev Urol 2020;17:232-50. [Crossref] [PubMed]
- Png CW, Lee WJJ, Chua SJ, et al. Mucosal microbiome associates with progression to gastric cancer. Theranostics 2022;12:48-58. [Crossref] [PubMed]
- Ferreira RM, Pereira-Marques J, Pinto-Ribeiro I, et al. Gastric microbial community profiling reveals a dysbiotic cancer-associated microbiota. Gut 2018;67:226-36. [Crossref] [PubMed]
- Wang L, Xin Y, Zhou J, et al. Gastric Mucosa-Associated Microbial Signatures of Early Gastric Cancer. Front Microbiol 2020;11:1548. [Crossref] [PubMed]
- Rajasekaran K, Carey RM, Lin X, et al. The microbiome of HPV-positive tonsil squamous cell carcinoma and neck metastasis. Oral Oncol 2021;117:105305. [Crossref] [PubMed]
- Flemer B, Lynch DB, Brown JM, et al. Tumour-associated and non-tumour-associated microbiota in colorectal cancer. Gut 2017;66:633-43. [Crossref] [PubMed]