Prognostic value and immune regulatory role of dynamin 1-like in lung adenocarcinoma
Highlight box
Key findings
• Dynamin 1-like (DNM1L) was overexpressed in lung adenocarcinoma (LUAD) compared to healthy control tissues and significantly promoted the proliferation and migration of LUAD cells, which suggests DNM1L was a potential therapeutic target in LUAD.
What is known and what is new?
• Dynamin-related protein 1, which is encoded by the DNM1L gene, acts as a regulator of mitochondrial fission and plays crucial roles in tumor initiation and progression.
• DNM1L may play an important role in the recruitment of immune cells in LUAD, potentially contributing to the regulation of immune-related signaling pathways and the infiltration of tumor-related immune cells.
What is the implication, and what should change now?
• DNM1L plays a role in regulating the immune infiltration of immune cell subsets in the tumor microenvironment of lung tumors. Further studies are however warranted to define its exact mechanism of action and potential therapeutic significance in LUAD patients.
Introduction
Lung cancer remains the major cause of cancer-related mortality all over the world, with 1.8 million new cases diagnosed and 1.6 million deaths each year. Globally, the incidence of lung cancer continues to rise annually and 5-year survival rate following diagnosis remains at less than 20% (1). Lung adenocarcinoma (LUAD) retains the most common histological subtype of non-small cell lung cancer (NSCLC) and is often diagnosed at an advanced stage, where surgical resection with curative intent is not generally a treatment option for these patients (2). The most recent use of immunotherapies, such as programmed cell death-1 (PD-1), programmed cell death-ligand 1 (PD-L1), cytotoxic T-lymphocyte antigen 4 (CTLA-4) immune checkpoint inhibitors (ICIs), either in first- or second-line setting, have revolutionized the treatment landscape of NSCLC and has led to significant durable responses in many patients (3,4). However, resistance to ICIs is a common phenomenon, leading to disease progression following an initial response to treatment (5,6). A greater understanding of the biological mechanisms underlying the resistance to ICIs may contribute to the development of more effective immunotherapies (7). One possible mechanism that plays in the development of resistance to ICIs is the inhibition of migration and infiltration of T cells to the immune tumor microenvironment (TME), which results in a functionally exhausted phenotype (8). Thus, identifying the underlying biological mechanisms of T cell exhaustion is crucial in improving the response to immunotherapy. The immune cell infiltration in the TME is particularly relevant to the prognosis and chemosensitivity of patients, in particular neutrophils and tumor-associated macrophages (TAMs) (9). Therefore, identifying the tumor immunophenotype and further understanding of the underlying biological mechanisms of immune regulation are warranted to identify novel immunotherapeutic targets in LUAD (10).
The mitochondria play essential roles in a number of essential cellular functions, such as cell growth, proliferation, differentiation, metabolism, and programmed cell death (11). It is now known that mitochondrial dynamics are implicated in the regulation of fundamental processes related to T cell and natural killer (NK) function and tumor immuno-surveillance (12,13). In fact, mitochondrial morphology may directly regulate T cell differentiation through metabolic pathways. Dynamin 1-like (DNM1L)/dynamin-related protein 1 (DRP1) plays important roles in regulating mitochondrial fission and in maintaining the mitochondrial dynamic network, which is important in T cell proliferation, migration, and invasion (14).
The molecular mechanisms whereby DNM1L regulates tumor progression and the immune TME in LUAD remain largely unknown. Herein, we evaluated DNM1L expression in LUAD and the correlation between DNM1L and clinical variables based on publicly available databases. In addition, we comprehensively analyzed the correlation between DNM1L expression and survival outcomes of LUAD patients based on Kaplan-Meier plotter and PrognoScan databases and the association between DNM1L expression and the immune infiltration of cells in the TME using the Tumor IMmune Estimation Resource (TIMER)2.0 database. This study provides novel insights into the potential clinical value of DNM1L in LUAD and highlights, at least in part, the mechanistic pathways whereby DNM1L affects immune cell-tumor interactions. We present this article in accordance with the REMARK reporting checklist (available at https://tlcr.amegroups.com/article/view/10.21037/tlcr-23-685/rc).
Methods
University of The ALabama at Birmingham CANcer data analysis Portal (UALCAN)
UALCAN (https://ualcan.path.uab.edu/index.html) is a comprehensive and interactive online resource for the in-depth analysis of gene expression data based on The Cancer Genome Atlas (TCGA) (15). We evaluated the expression of DNM1L in the “lung adenocarcinoma” dataset using the “Expression Analysis” module. Moreover, we also explored the phosphoprotein expression profiles of DNM1L in LUAD and adjacent normal lung tissues according to clinical variables, including race, age, tumor grade, and cancer stage, in the Clinical Proteomic Tumor Analysis Consortium (CPTAC) database. The prognostic analysis was performed using the Student’s t-test. Statistical significance was determined as a P value <0.05. The study was conducted in accordance with the Declaration of Helsinki (as revised in 2013).
Human Protein Atlas (HPA)
The HPA dataset (https://www.proteinatlas.org/) aims to map human proteins based on proteomics, transcriptomic, and systems biology data (16). It includes data on protein expression in normal and tumor tissues, in addition to their prognostic relevance in the form of survival curves. It also contains the survival curve data of tumor patients. DNM1L protein expression in LUAD and healthy control tissues was evaluated by immunohistochemistry (IHC) and scoring of stained tissues based on staining intensity (negative, weak, moderate, or strong), fraction of stained cells, and subcellular localization (nuclear and/or cytoplasmic/membranous).
Gene expression profiling interactive analysis (GEPIA)
The GEPIA database (http://gepia2.cancer-pku.cn/#index) offers a tool for the analysis of gene expression data related to cancer pathological subtypes, driver genes, alleles, differentially expressed or carcinogenic factors, so as to explore new cancer therapeutic targets and biomarkers based on normal and tumor samples in the TCGA and Genotype-Tissue Expression (GTEx) databases (17). The “Correlation Analysis” module was used to explore the correlation between DNM1L and other genes in the GEPIA database. We analyzed the correlation of DNM1L with other genes with spearman coefficient using the non-log scale for calculation and the log-scale axis for visualization. Statistical significance was determined as a P value <0.05.
PrognoScan
PrognoScan (http://dna00.bio.kyutech.ac.jp/PrognoScan/index.html) is a tool for evaluating the biological correlation between prognosis and gene expression (18). The minimum P value method is used to group patients for survival analysis. This method does not require continuous biological knowledge nor assumptions to determine the best entry point for the continuous gene expression measurement. Empirical grouping of median/quartile/quartile does not necessarily reflect the true biological function of a gene. Therefore, the minimum P value method is a full-scale analysis method to explore the best critical point of risk separation for continuous variables. It has been shown to be highly practical in tumor size analysis, cell cycle measurements, and gene copy number analysis. Herein, we assessed the association between DNM1L and the outcomes of LUAD patients.
Kaplan-Meier plotter
The Kaplan-Meier plotter database (https://kmplot.com/analysis/index.php?p=service&cancer=pancancer_rnaseq) is available for evaluating the correlation of gene expression [microRNA (miRNA), messenger RNA (mRNA), protein] with survival in more than thirty thousand samples of 21 tumor types from the TCGA and Gene Expression Omnibus (GEO) databases. This tool aims to conduct a meta-analysis-based identification and validation of potential survival biomarkers (19,20). We categorize patients based on the automatically selected optimal threshold. When this checkbox is ticked, we calculate all potential threshold values between the lower and upper quartiles of DNM1L expression, and then use the most effective threshold as the cutoff. The results page will show the false discovery rate along with the P value. Herein, the database was applied to evaluate the association between DNM1L expression and patient outcome, and to analyze the effect of both clinical variables and DNM1L on the survival outcome of LUAD patients.
TIMER2.0
The TIMER2.0 database (https://cistrome.shinyapps.io/timer/) provides a platform to comprehensively analyze the immune infiltrates in cancers. The extent of infiltration of immune cell types were evaluated using the TIMER algorithm. This allows researchers to analyze immune cell infiltration in tumor tissues with RNA sequencing (RNA-seq) profiles (21). Using the TIMER “gene” module, we explored the correlation between immune cell infiltration and DNM1L expression in LUAD. After inputting gene symbol for DNM1L, the cancer type for LUAD and the cell type of immune infiltration, we clicked the submit button to visualize a scatter plot, with illustrates the relationship between the estimated infiltrates value and gene expression. We defined the correlation between immune cell infiltration and DNM1L expression based on the following criteria: positive correlation (P<0.05; Pearson correlation coefficient >0); negative correlation (P<0.05; Pearson correlation coefficient <0). In addition, we also evaluated the correlation between DNM1L expression and hub gene sets of different immune cells in the TIMER2.0 database.
Search Tool for the Retrieval of Interacting Genes/Proteins (STRING) analysis
The STRING database (https://cn.string-db.org/) can be used to retrieve known and predicted protein-protein interactions (PPIs). In addition to producing exquisite networks of proteins, it also provides analysis of input proteins, including common functional enrichment analyses and reference publications (22). The goal of STRING is to achieve a full interaction network, including physical interactions (direct interactions) and functional associations (indirect interactions).
GeneMANIA
GeneMANIA (https://genemania.org/) helps the users estimate the value of the target genes or gene sets and to identify additional genes that may have been missed in the screening process or novel genes with specific biological functions based on the function-related data. These include gene-protein interactions, molecular pathways, co-localization, co-expression, and protein domain similarity (23). In our study, the GeneMANIA database was used to develop the gene-gene interaction network involved in DNM1L to explore the potential molecular functions of the genes.
Cell lines
The human LUAD cell lines, including H1299, PC-9, A549, HCC827, H460, and H1975, were purchased from the China Center for Type Culture Collection (CCTCC, Wuhan, China) and were routinely cultured in RPMI 1640 containing 10% fetal bovine serum (FBS; Corning, Manassas, VA, USA) and 1% penicillin-streptomycin liquid (Solarbio, Beijing, China) at 37 ℃ with 5% CO2.
RNA interference
Small interference RNAs (siRNAs) were purchased from RiboBio (Guangzhou, China) and used for DNM1L knockdown in LUAD cells. Three siRNAs were transiently transfected into cells by lipofectamine 2000 (Invitrogen, Carlsbad, CA, USA) at a concentration of 100 nM. The siRNA sequences: (I) 5'-3': GGAACAAAGTATCTTGCTA; (II) 5'-3': GAAGGGTTATTCCAGTCAA; and (III) 5'-3': GGTTGGAGATGGTGTTCAA.
Reverse transcription-quantitative polymerase chain reaction (RT-qPCR)
Total RNA was extracted by FastPure Cell/Tissue Total RNA Isolation Kit V2 (Vazyme, Nanjing, China) according to the manufacturer’s instructions. Reverse transcription was performed with 1 μg of total RNA by HiScript III RT SuperMix for qPCR (Vazyme). A Taq Pro Universal SYBR qPCR Master Mix (Vazyme) was selected for quantification. The relative gene expression was determined by the 2−ΔΔCt method. The gene PCR primers were as follows: β-actin forward: TGGCACCCAGCACAATGAA, β-actin reverse: CTAAGTCATAGTCCGCCTAGAAGCA; DNM1L forward: GCAACTGGAGAGGAATGCTG, DNM1L reverse: GCACATCTAGCAGGTTCACG.
Western blot
Cells were lysed in RIPA Lysis Buffer (Beyotime Biotechnology, Shanghai, China) and proteins were separated by 4–12% gradient polyacrylamide gel electrophoresis (PAGE; YAMAY BIOTECH, Shanghai, China). Subsequently, the proteins were transferred to a PVDF membrane (Millipore Corp., Bedford, MA, USA), and blocked with 5% skimmed milk at room temperature for 2 h. Membranes were washed for three times with TBST (tris-buffered saline Tween-20) and were subsequently immunoblotted with the primary antibodies at 4 ℃ overnight. Then, the secondary antibodies (Beyotime Biotechnology) were incubated with these membranes at room temperature for 2 h. Finally, the immunoreactive bands were developed using an ECL detection system (BLT, PHOTO Technology, Guangzhou, China). The primary antibodies were as follows: β-actin (1:1,000, Abways, Shanghai, China), DNM1L (1:6,000, Proteintech, Wuhan, China).
Cell proliferation assays
Cell growth was detected by the cell counting kit-8 (CCK-8) assays (Dojindo, Kumamoto-ken, Japan). Transient transfected H1299 and PC-9 cells with DNM1L siRNAs were seeded in 96-well plates with a density of 3,000 cells/well. Subsequently, CCK-8 was added to 96-well plates at 0, 24, 48, 72 h and incubated for another 2 h at 37 ℃. A microplate reader (BioTek, Burlington, VT, USA) was used to measure the absorbance at 450 nm.
Colony formation assays
Transient transfected H1299 and PC-9 cells with DNM1L siRNAs were seeded in 6-well plates at a density of 300 cells/well and incubated for 7 days. Then, the cells were fixed in paraformaldehyde for 30 minutes and stained with 0.1% crystal violet for 30 minutes.
Wound healing assays
Transient transfected H1299 and PC-9 cells with siRNAs were seeded in 24-well plates at a density of 2×105 cells/well. A 10 μL tip was used to scribe vertically on the bottom surface of 24-well plates. Subsequently, the cells underwent two rounds of phosphate buffer solution (PBS) washing followed by the addition of serum-free medium. Photographic documentation was conducted at 0, 24, and 48 h, respectively. The width measurements were calculated using Image J software.
Transwell assays
Transient transfected H1299 and PC-9 cells with siRNAs were re-suspended in serum-free medium and seeded 5×104 cells with 200 μL in the upper chamber (Corning, Manassas, VA, USA). For the lower chamber, medium containing 20% FBS was added. After 48 h, the cells in the upper chamber were discarded, while the cells crossing the chamber were fixed with paraformaldehyde and stained with crystal violet.
Statistical analysis
The GEPIA, Kaplan-Meier plotter, PrognoScan, and TIMER2.0 databases were used to generate survival plots. STRING and GeneMANIA databases were used to develop the gene-gene interaction network. Spearman correlation analysis was used to determine the correlations between cohorts. Student t-test was used to determine the discrepancy between groups. For in vitro experiments, each experiment was repeated three times. Data were considered significant where P values were ≤0.05 (*, P<0.05; **, P<0.01; ***, P<0.001).
Results
DNM1L is increased in cancer
The expression of DNM1L in cancer and healthy tissues was evaluated using the TIMER2.0 database. The results indicated that DNM1L was significantly upregulated in tumor tissues compared to normal tissues in almost all the cancer datasets examined, including breast cancer (BRCA), cholangiocarcinoma (CHOL), esophageal carcinoma (ESCA), colon adenocarcinoma (COAD), glioblastoma (GBM), kidney renal clear cell carcinoma (KIRC), head and neck squamous cell carcinoma (HNSCC), LUAD, liver hepatocellular carcinoma (LIHC), lung squamous cell carcinoma (LUSC), thyroid cancer (THCA), and stomach adenocarcinoma (STAD) (P<0.05) (Figure 1A). In addition, we estimated DNM1L mRNA expression between the LUAD and healthy tissues based on the TCGA data in the UALCAN database. Our results demonstrated that DNM1L mRNA was significantly upregulated in LUAD (P<0.05), which is consistent with data from the TIMER2.0 database (Figure 1B). Moreover, phosphoprotein levels of DNM1L in tumor tissue were significantly higher than that in the healthy tissue based on CPTAC data from the UALCAN database (Figure 1C). Similarly, IHC staining data in the HPA database indicate that DNM1L protein expression is increased in LUAD tissues compared to healthy lung tissues (P<0.05) (Figure 1D,1E). These results demonstrated that DNM1L is upregulated in LUAD, suggesting that DNM1L may play essential roles in the tumorigenesis and progression.
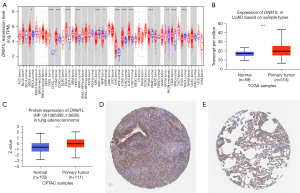
DNM1L expression correlates with clinical and molecular parameters
To further explore the correlation of DNM1L expression with clinical variables, we analyzed the mRNA profiles of DNM1L in subgroups of patients with different clinical variables based on UALCAN database analysis. Data showed that DNM1L protein expression was significantly upregulated in both female and male LUAD tissue relative to adjacent normal lung tissue (P<0.05) (Figure 2A). Additionally, DNM1L was significantly upregulated in LUAD tissue in patients across different age groups: 41–60 and 61–80 years, compared to DNM1L expression in adjacent normal lung tissues (P<0.05) (Figure 2B). Significantly higher expression of DNM1L was observed in grade 2 and grade 3 LUAD tumors in addition to patients with stage 1, 2, and 3 diseases (P<0.05) (Figure 2C,2D). There was also a significantly higher expression of DNM1L protein between grade 3 and grade 2 tumors. DNM1L expression was significantly higher in p53/Rb-related pathway-altered cohorts compared to p53 wild-type (WT) LUAD tumors and adjacent normal lung tissue (P<0.05) (Figure 2E). Furthermore, DNM1L expression was significantly upregulated in both myelocytomatosis oncogene (MYC)/neuroblastoma-derived MYC (MYCN) altered and other (MYC/MYCN WT) LUAD samples compared to adjacent normal lung tissue (P<0.05) (Figure 2F). Overall, these data highlight significant increases in DNM1L protein expression across a variety of clinical and molecular variables in LUAD patients.
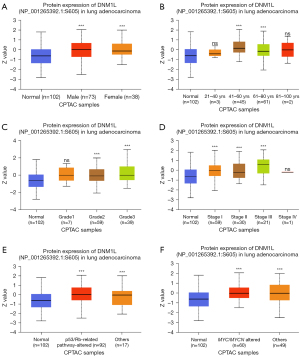
DNM1L is an independent prognostic biomarker in LUAD
The correlation between DNM1L expression and prognosis of LUAD patients was evaluated using the UALCAN database. Kaplan-Meier survival curves showed that high expression of DNM1L was significantly associated with unfavorable outcomes in patients with LUAD (P=0.0033) (Figure 3A). Patients with high expression of DNM1L had significantly worse overall survival (OS) and progression-free survival (PFS) compared to those with low DNM1L expression, based on microarray data of DNM1L expression from the Kaplan-Meier plotter database (P<0.05) (Figure 3B). Furthermore, we validated the predictive value in the independent GEO datasets from the PrognoScan database. Consistent with the above data, these results demonstrated that high expression of DNM1L was significantly associated with worse OS in two independent GEO cohorts (GSE13213 and GSE31210) (P<0.05) (Figure 3C,3D).
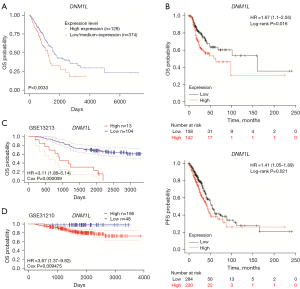
DNM1L interacts with genes involved in mitochondrial, apoptotic, and peroxisomal function
Gene-gene interactions were constructed to evaluate genes that interact with DNM1L in LUAD and to explore the functions of these genes using the GeneMANIA database. A total of 20 genes were significantly associated with DNM1L (Figure 4A). The top 5 candidate genes that correlated with DNM1L gene expression included B cell receptor-associated protein 31 (BCAP31), mitochondrial elongation factor 2 (MIEF2), mitochondrial fission factor (MFF), caspase-8 (CASP8), and secretogranin III (SCG3). Furthermore, functional analysis on the differentially expressed genes (DEGs) between the high and low DNM1L expression cohorts demonstrated that these genes were mainly associated with the following biological processes; microbody, peroxisome organization, regulation of mitochondrial fission, mitochondrial fission, and regulation of mitochondrion organization. To further evaluate the functional mechanisms of the DNM1L gene, its associated PPI network was examined using STRING. The top 11 proteins identified in the DNM1L network were shown (Figure 4B). Among these, the five proteins with the highest degree of centrality were mitochondrial fission 1 (FIS1), huntingtin (HTT), leucine-rich repeat kinase 2 (LRRK2), MFF, and MIEF2. In addition, we further estimated the association between DNM1L and the interacting genes in the GEPIA database. Further experiments found that the expression of MFF in LUAD was increased, while the expression of FIS1 was decreased (Figure S1). Our results demonstrated that DNM1L was significantly associated with CASP8, COPB2, FIS1, HTT, JMJD6, MFN1, MFN2, and PEX11B in LUAD (P<0.05) (Figure 4C-4J).
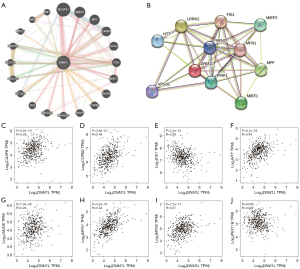
DNM1L expression correlates with immune cell infiltration in LUAD
Lymphocyte infiltration is known to be an independent factor of survival outcomes and sentinel lymph node status. Herein, we explored the correlation between DNM1L expression and the immune cell infiltration in the TME. We performed the Spearman correlation analysis to evaluate the correlation between DNM1L expression and infiltration levels of immune cell subsets based on the data from TCGA-LUAD cohort using ggplot2 R package in R software (4.2.1 version). As shown in Figure 5, the results showed that DNM1L expression was significantly positively associated with the infiltration levels of T helper (Th) cells, especially Th2 cells. while, negatively associated with the infiltration levels of B cells, CD8 T cells, dendritic cells, and mast cells (P<0.05).
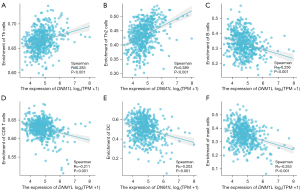
Correlation between immune marker sets and DNM1L expression
We further evaluated the correlation between infiltrating immune cell subtypes and DNM1L, based on immune cell markers using TIMER2.0. Our data showed that DNM1L was significantly associated with numerous immune cell markers (P<0.05) (Table 1). Subsequently, we explored the association of DNM1L expression with diverse populations of functional T cells, such as regulatory T cells (Tregs), effector T cells, Th1, Th2, memory, naïve, and exhausted T cells using the TIMER2.0 database. Of interest, DNM1L expression was significantly associated with 28 of the 41 T cell markers in LUAD (Table 2), further supporting our data that DNM1L expression is positively related to the infiltration of anti-tumor immune cells.
Table 1
Immune cell | Gene marker | Correlation | P value |
---|---|---|---|
CD8+ T cell | CD8A | 0.103 | * |
CD8B | 0.091 | * | |
T cell (general) | CD3D | 0.005 | 0.910 |
CD3E | 0.025 | 0.577 | |
CD2 | 0.006 | 0.892 | |
B cell | CD19 | 0.004 | 0.921 |
CD79A | 0.002 | 0.956 | |
Monocyte | CD86 | 0.116 | ** |
CSF1R | 0.080 | 0.069 | |
TAM | CCL2 | 0.123 | ** |
CD68 | 0.105 | * | |
IL10 | 0.116 | ** | |
M1 | IRF5 | 0.048 | 0.275 |
NOS2 | 0.212 | *** | |
PTGS2 | 0.198 | *** | |
M2 | CD163 | 0.247 | *** |
VSIG4 | 0.074 | 0.094 | |
MS4A4A | 0.082 | 0.062 | |
Neutrophils | CEACAM8 | −0.127 | ** |
ITGAM | 0.063 | 0.155 | |
CCR7 | −0.010 | 0.828 | |
NK cell | KIR2DL1 | 0.062 | 0.160 |
KIR2DL3 | 0.195 | *** | |
KIR2DL4 | 0.238 | *** | |
KIR3DL1 | 0.141 | ** | |
KIR3DL2 | 0.161 | *** | |
KIR2DS4 | 0.091 | * | |
Dendritic cell | HLA-DPB1 | −0.264 | *** |
HLA-DQB1 | −0.229 | *** | |
HLA-DRA | −0.235 | *** | |
HLA-DPA1 | −0.194 | *** | |
CD1C | −0.297 | *** | |
NRP1 | 0.151 | *** | |
ITGAX | 0.103 | * |
Spearman correlation analysis was used to determine correlations, with statistical significance represented as: *, P<0.05; **, P<0.01; ***, P<0.001. DNM1L, dynamin 1-like; TIMER, Tumor IMmune Estimation Resource; TAM, tumor-associated macrophage; NK, natural killer.
Table 2
T cell | Gene marker | Correlation | P value |
---|---|---|---|
Th1 | STAT4 | −0.015 | 0.742 |
STAT1 | 0.346 | *** | |
IFNG | 0.163 | *** | |
TNF | 0.094 | * | |
Th1-like | CXCL13 | 0.034 | 0.280 |
HAVCR2 | 0.075 | * | |
BHLHE40 | 0.105 | * | |
CD4 | 0.061 | 0.166 | |
IL21 | 0.179 | *** | |
Th17 | STAT3 | 0.267 | *** |
IL17A | 0.067 | 0.128 | |
Treg | FOXP3 | 0.138 | *** |
CCR8 | 0.212 | *** | |
STAT5B | 0.287 | *** | |
TGFB1 | −0.035 | 0.424 | |
Effector T cell | FGFBP2 | −0.043 | 0.331 |
FCGR3A | 0.193 | *** | |
Naïve T cell | SELL | 0.038 | 0.219 |
TCF7 | 0.196 | *** | |
Effective memory T cell | PDCD1 | 0.090 | * |
DUSP4 | 0.350 | *** | |
GZMK | 0.032 | 0.470 | |
GZMA | 0.101 | * | |
IFNG | 0.163 | *** | |
Resistant memory T cell | CD69 | −0.049 | 0.267 |
ITGAE | 0.057 | 0.200 | |
CXCR6 | 0.095 | * | |
MYADM | 0.179 | *** | |
Exhausted T cell | HAVCR2 | 0.075 | * |
TIGIT | 0.172 | *** | |
LAYN | 0.107 | * | |
Resting Treg T cell | FOXP3 | 0.138 | *** |
IL2RA | 0.291 | *** | |
Effective Treg T cell | FOXP3 | 0.138 | *** |
CTLA4 | 0.132 | * | |
CCR8 | 0.212 | *** | |
TNFRSF9 | 0.172 | *** | |
General memory T cell | SELL | 0.038 | 0.219 |
IL7R | 0.199 | *** |
Spearman correlation analysis was used to determine correlations, with statistical significance represented as: *, P<0.05; ***, P<0.001. DNM1L, dynamin 1-like; TIMER, Tumor IMmune Estimation Resource; Th, T helper; Treg, regulatory T cell.
DNM1L promotes the proliferation and migration of LUAD cells
The six LUAD cell lines (H1299, PC-9, A549, HCC827, H460 and H1975) selected are all commonly used in lung cancer research. For validation of our predicted tumor promoter role of DNM1L, we first detected the RNA and protein expression of DNM1L in different LUAD cell lines (Figure 6A-6C), and selected two cell lines H1299 and PC-9 with relatively high expression of DNM1L for further experiments. Then, we used siRNAs to inhibit the expression of DNM1L in H1299 and PC-9 cells. In the pre-experiment, all three siRNAs can effectively knock down the DNM1L (Figure 6D,6E), so we use the mixture of three siRNAs to construct the DNM1L-knockdown cell lines, and the silence of DNM1L expression was confirmed by RT-qPCR and western blot (Figure 6F-6I).
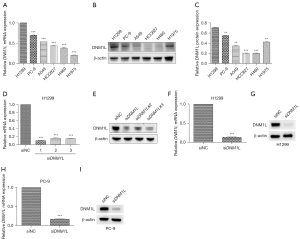
The CCK-8 assays and colony formation assays demonstrated that the knockdown of DNM1L significantly suppressed the proliferation ability of LUAD cells (Figure 7A-7E). Meanwhile, the results of wound healing assays and transwell assays showed that the knockdown of DNM1L expression remarkably decreased the migration capacity of LUAD cells (Figure 7F-7J). These results confirmed that DNM1L is a detrimental factor in LUAD.
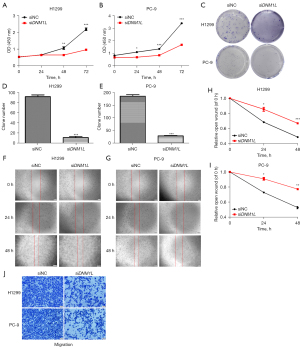
Discussion
The relevant prognostic and therapeutic markers play an important role in the management of LUAD patients (24,25). Dysregulated mitochondrial dynamics are responsible for the progression and metastasis of many cancers. The functional mitochondrial network is regulated by various key proteins, including DNM1L. These mitochondria-shaping proteins play important roles in diverse biological pathways such as cell metabolism, proliferation, migration, cell death, as well as innate and adaptive immunity via its regulation of the dynamic equilibrium between mitochondria fission and fusion. Notably, mitochondrial dynamics are altered, thereby resulting in the inhibition of apoptosis and altered metabolic requirements of cancer cells which help drive tumor initiation and progression.
Accumulating evidence indicates that DNM1L-induced mitochondrial fission acts as an important mechanism in carcinogenesis. Overexpression of DNM1L contributes to disease progression and induces glycolysis through regulation of the FOXM1/MMP12 axis in head and neck cancer (26). Increased DNM1L also promotes autophagy and disease progression of esophageal squamous cell carcinoma (27). It has been reported that DNM1L may be recruited to the mitochondria to promote mitochondrial fission, facilitating cell metastasis in hepatocellular carcinoma cells both in vivo and in vitro (28). It can also promote metabolic changes induced by KRAS to drive the growth of pancreatic tumors (29). In addition, DNM1L-mediated mitochondrial fission participates in the development of chemoresistance in various cancer types (30). DNM1L- and MIEF1/2-mediated mitochondrial fission has been shown to link the extracellular matrix mechano-transduction to metabolism and resistance to chemotherapy in metastatic BRCA (31). These observations point to DNM1L as a potential therapeutic target for cancer treatment and as such, warrants further studies to elucidate its role in this disease.
In view of the important role of elevated DNM1L in stem cell maintenance, cell metabolism, proliferation, invasion, and metastasis, various DNM1L inhibitors have been identified in numerous studies as a potential therapeutic strategy in cancer treatment. Mitochondrial division inhibitor 1 (mdivi-1) is an efficacious DNM1L protein inhibitor, which can inhibit cell proliferation by inducing mitochondrial fusion and changes in oxygen consumption (32). Fang et al. reported that mdivi-1 could induce spindle abnormalities and augment the cytotoxicity of taxol to improve its efficacy in BRCA (33). Furthermore, Wang et al. demonstrated that mdivi-1 could enhance death receptor-mediated apoptosis in human ovarian cancer cells (34). This study also showed that SUMOylated synaptojanin 2 binding protein-cytochrome-c oxidase 16, recruits DNM1L to the mitochondria to enhance SUMOylation and phosphorylation in order to induce mitochondrial fission which could be inhibited by mdivi-1 (35). These findings suggest that treatments directed against the mitochondria via targeting of DNM1L protein, may represent a novel strategy for many diseases, including cancer. Despite this, the relationship between DNM1L and prognosis in LUAD remains largely unexplored, while a link between DNM1L expression and immune cell infiltration in the TME of this NSCLC subtype, remains to be elucidated. Further exploration of the expression patterns of DNM1L in LUAD and its relationship with specific immune cell subtypes is warranted.
In this study, we explored the clinical value and immune regulation of DNM1L in LUAD using data from the TCGA, UALCAN, and TIMER2.0 databases. Our data show that DNM1L is significantly overexpressed in LUAD. High DNM1L expression was associated with various clinical and molecular parameters, including higher tumor grade and stage, in addition to OS and PFS in patients with LUAD, thereby suggesting that DNM1L is an independent prognostic factor in LUAD. Immunotherapy using ICIs aims to enhance natural defenses to eliminate cancer cells. This has revolutionized anti-tumor treatments in the lung cancer setting and yielded unparalleled survival benefits for cancer patients. However, limited efficacy remains a challenge in most clinical settings and only a minority of patients benefit from this treatment, with even fewer achieving durable responses (36). The infiltrating immune cells in the TME are significantly associated with disease progression and therapeutic outcome of cancer patients (37). The TME contains a variety of cell types, including immune cells, stromal cells, and cancer cells as well as secreted factors which are often the targets for anti-tumor therapies (38). Advanced therapeutic strategies targeting the TME in cancer have been developed in recent years (39). Since tumor-infiltrating immune cells, in particular cytotoxic CD8+ T cells play essential roles in immunotherapy, comprehensive research of the immune cells within the TME in tumors is vital, in order to develop new immunotherapeutic strategies and to optimize treatments (40). Notably, the mitochondria are involved in inflammation, immunity and the TME in response to cellular stress, damage, and infection (41). Mitochondrial dynamics are crucial for many cellular processes such as mitochondrial integrity, apoptosis, cell cycle, inflammatory responses, and immunity (42,43). Mitochondrial dynamics regulate the activation, differentiation, and cytokine production of immune cells, including B cells, T cells, dendritic cells, and macrophages, in both innate and adaptive immunity (14). Zheng et al. showed that excessive mitochondrial fission is associated with loss of NK cells and reduced cytotoxicity to evade the surveillance mediated by the NK cells, resulting in unfavorable survival in patients with liver cancer (12). The sustained activation of rapamycin-GTPase DNM1L of NK cells in the hypoxic TME results in excessive mitochondrial fission (12). PD-1 signaling downregulates DNM1L phosphorylation on serine 616 (Ser616) to restrain mitochondrial fission mediated by T cells via the extracellular signal-regulated kinases 1 and 2 (ERK1/2) and mechanistic target of rapamycin (mTOR) pathways (44). Bao et al. demonstrated that overexpressing DNM1L was positively associated with infiltration of TAMs in the TME of hepatocellular carcinomas (45). DNM1L-mediated mitochondrial fission-induced cytosolic mitochondrial DNA (mtDNA) stress figure upregulates C-C motif chemokine 2 (CCL2) via toll-like receptor 9 (TLR9)-mediated nuclear factor-κB (NF-κB) signaling and thus, facilitates the polarization and recruitment of TAMs (45). As a systemic disease, cancer can regulate many functions and components of the immune system. Our data indicate that DNM1L participates in the tumor-immune interactions of LUAD and is associated with immune cell infiltration. We also report that DNM1L is positively associated with the level of infiltration of numerous immune cells in LUAD, including Th cells, B cells, CD8 T cells, dendritic cells, and mast cells. Furthermore, we show that DNM1L is significantly associated with diverse subtypes of T cells, including Th1, Th2, T follicular helper (Tfh), Treg, native, memory, effector, and exhausted T cells, suggesting that DNM1L plays a key role in immunity and therefore, may be a potential therapeutic target in the TME of LUAD patients.
Moreover, our study shows that tumor protein p53 (TP53) WT LUAD tumors had higher DNM1L expression compared to p53-mutated tumors, a finding of which is consistent with previous studies (46,47). Accumulating evidence has indicated that p53 can induce phosphorylation of DNM1L at Ser637 and subsequent mitochondrial dysfunction, thereby providing evidence for its important role in mitochondrial dynamics and function (48). Such findings support the idea that inhibition of DNM1L may be an effective therapeutic strategy for overcoming drug resistance in p53-mutated cancers, including LUAD (46,47).
Limitations
This study had a number of limitations. Firstly, the analyses were conducted based on data from several public databases. We are aware that web-based databases, including UALCAN, HPA, GEPIA, PrognoScan, Kaplan-Meier plotter, TIMER2.0, STRING, and GeneMANIA, lack detailed information about data sources and methodologies, which is a significant limitation in my study. Moreover, in the correlation analysis between DNM1L expression and clinical and molecular parameters, some groups have small sample size, even less than 10. Further research, both in vivo and in vitro, is required to examine the underlying molecular mechanisms of DNM1L in regulating the immune cell infiltration in LUAD. Secondly, future prospective studies with larger sample cohorts are warranted to evaluate the prognostic value of DNM1L. Thirdly, while mitochondrial fission is regulated by several genes, including DNM1L, FIS1, and MFF (49), this study focused primarily on the role of DNM1L which to our knowledge, has not been extensively reported in the context of LUAD.
Conclusions
Taken together, our findings demonstrate DNM1L as a potential prognostic biomarker in LUAD. Furthermore, we report for the first time in LUAD, a role for DNM1L in regulating proliferation and migration of LUAD cells as well as the infiltration of tumor-related immune cells, which suggests DNM1L was a potential therapeutic target in LUAD. Further studies are however warranted to define its exact mechanism of action and potential therapeutic significance in LUAD patients.
Acknowledgments
We are grateful to the contributors to the public databases used in this study.
Funding: This study was supported by
Footnote
Reporting Checklist: The authors have completed the REMARK reporting checklist. Available at https://tlcr.amegroups.com/article/view/10.21037/tlcr-23-685/rc
Peer Review File: Available at https://tlcr.amegroups.com/article/view/10.21037/tlcr-23-685/prf
Conflicts of Interest: All authors have completed the ICMJE uniform disclosure form (available at https://tlcr.amegroups.com/article/view/10.21037/tlcr-23-685/coif). The authors have no conflicts of interest to declare.
Ethical Statement: The authors are accountable for all aspects of the work in ensuring that questions related to the accuracy or integrity of any part of the work are appropriately investigated and resolved. The study was conducted in accordance with the Declaration of Helsinki (as revised in 2013).
Open Access Statement: This is an Open Access article distributed in accordance with the Creative Commons Attribution-NonCommercial-NoDerivs 4.0 International License (CC BY-NC-ND 4.0), which permits the non-commercial replication and distribution of the article with the strict proviso that no changes or edits are made and the original work is properly cited (including links to both the formal publication through the relevant DOI and the license). See: https://creativecommons.org/licenses/by-nc-nd/4.0/.
References
- Siegel RL, Miller KD, Fuchs HE, et al. Cancer statistics, 2022. CA Cancer J Clin 2022;72:7-33. [Crossref] [PubMed]
- Succony L, Rassl DM, Barker AP, et al. Adenocarcinoma spectrum lesions of the lung: Detection, pathology and treatment strategies. Cancer Treat Rev 2021;99:102237. [Crossref] [PubMed]
- Mamdani H, Matosevic S, Khalid AB, et al. Immunotherapy in Lung Cancer: Current Landscape and Future Directions. Front Immunol 2022;13:823618. [Crossref] [PubMed]
- Reck M, Remon J, Hellmann MD. First-Line Immunotherapy for Non-Small-Cell Lung Cancer. J Clin Oncol 2022;40:586-97. [Crossref] [PubMed]
- Passaro A, Brahmer J, Antonia S, et al. Managing Resistance to Immune Checkpoint Inhibitors in Lung Cancer: Treatment and Novel Strategies. J Clin Oncol 2022;40:598-610. [Crossref] [PubMed]
- Schoenfeld AJ, Antonia SJ, Awad MM, et al. Clinical definition of acquired resistance to immunotherapy in patients with metastatic non-small-cell lung cancer. Ann Oncol 2021;32:1597-607. [Crossref] [PubMed]
- Li D, Cheng C, Song WP, et al. Dramatic response to immunotherapy in an epidermal growth factor receptor-mutant non-small cell lung cancer: A case report. World J Clin Cases 2021;9:11419-24. [Crossref] [PubMed]
- Morad G, Helmink BA, Sharma P, et al. Hallmarks of response, resistance, and toxicity to immune checkpoint blockade. Cell 2021;184:5309-37. [Crossref] [PubMed]
- Gajewski TF, Schreiber H, Fu YX. Innate and adaptive immune cells in the tumor microenvironment. Nat Immunol 2013;14:1014-22. [Crossref] [PubMed]
- Dang HH, Ta HDK, Nguyen TTT, et al. Prospective role and immunotherapeutic targets of sideroflexin protein family in lung adenocarcinoma: evidence from bioinformatics validation. Funct Integr Genomics 2022;22:1057-72. [Crossref] [PubMed]
- Chan DC. Mitochondrial Dynamics and Its Involvement in Disease. Annu Rev Pathol 2020;15:235-59. [Crossref] [PubMed]
- Zheng X, Qian Y, Fu B, et al. Mitochondrial fragmentation limits NK cell-based tumor immunosurveillance. Nat Immunol 2019;20:1656-67. [Crossref] [PubMed]
- Simula L, Pacella I, Colamatteo A, et al. Drp1 Controls Effective T Cell Immune-Surveillance by Regulating T Cell Migration, Proliferation, and cMyc-Dependent Metabolic Reprogramming. Cell Rep 2018;25:3059-3073.e10. [Crossref] [PubMed]
- Song J, Yi X, Gao R, et al. Impact of Drp1-Mediated Mitochondrial Dynamics on T Cell Immune Modulation. Front Immunol 2022;13:873834. [Crossref] [PubMed]
- Chandrashekar DS, Bashel B, Balasubramanya SAH, et al. UALCAN: A Portal for Facilitating Tumor Subgroup Gene Expression and Survival Analyses. Neoplasia 2017;19:649-58. [Crossref] [PubMed]
- Uhlen M, Oksvold P, Fagerberg L, et al. Towards a knowledge-based Human Protein Atlas. Nat Biotechnol 2010;28:1248-50. [Crossref] [PubMed]
- Tang Z, Li C, Kang B, et al. GEPIA: a web server for cancer and normal gene expression profiling and interactive analyses. Nucleic Acids Res 2017;45:W98-W102. [Crossref] [PubMed]
- Mizuno H, Kitada K, Nakai K, et al. PrognoScan: a new database for meta-analysis of the prognostic value of genes. BMC Med Genomics 2009;2:18. [Crossref] [PubMed]
- Győrffy B. Discovery and ranking of the most robust prognostic biomarkers in serous ovarian cancer. Geroscience 2023;45:1889-98. [Crossref] [PubMed]
- Nagy Á, Munkácsy G, Győrffy B. Pancancer survival analysis of cancer hallmark genes. Sci Rep 2021;11:6047. [Crossref] [PubMed]
- Li T, Fan J, Wang B, et al. TIMER: A Web Server for Comprehensive Analysis of Tumor-Infiltrating Immune Cells. Cancer Res 2017;77:e108-10. [Crossref] [PubMed]
- Szklarczyk D, Gable AL, Nastou KC, et al. The STRING database in 2021: customizable protein-protein networks, and functional characterization of user-uploaded gene/measurement sets. Nucleic Acids Res 2021;49:D605-12. [Crossref] [PubMed]
- Warde-Farley D, Donaldson SL, Comes O, et al. The GeneMANIA prediction server: biological network integration for gene prioritization and predicting gene function. Nucleic Acids Res 2010;38:W214-20. [Crossref] [PubMed]
- Tran TO, Vo TH, Lam LHT, et al. ALDH2 as a potential stem cell-related biomarker in lung adenocarcinoma: Comprehensive multi-omics analysis. Comput Struct Biotechnol J 2023;21:1921-9. [Crossref] [PubMed]
- Wu X, Zhou H, He Z, et al. Coexistence of a novel CCNY-ALK and ATIC-ALK double-fusion in one patient with ALK-positive NSCLC and response to crizotinib: a case report. Transl Lung Cancer Res 2020;9:2494-9. [Crossref] [PubMed]
- Huang TL, Chang CR, Chien CY, et al. DRP1 contributes to head and neck cancer progression and induces glycolysis through modulated FOXM1/MMP12 axis. Mol Oncol 2022;16:2585-606. [Crossref] [PubMed]
- Li Y, Chen H, Yang Q, et al. Increased Drp1 promotes autophagy and ESCC progression by mtDNA stress mediated cGAS-STING pathway. J Exp Clin Cancer Res 2022;41:76. [Crossref] [PubMed]
- Yu Y, Peng XD, Qian XJ, et al. Fis1 phosphorylation by Met promotes mitochondrial fission and hepatocellular carcinoma metastasis. Signal Transduct Target Ther 2021;6:401. [Crossref] [PubMed]
- Nagdas S, Kashatus JA, Nascimento A, et al. Drp1 Promotes KRas-Driven Metabolic Changes to Drive Pancreatic Tumor Growth. Cell Rep 2019;28:1845-1859.e5. [Crossref] [PubMed]
- Xie L, Shi F, Li Y, et al. Drp1-dependent remodeling of mitochondrial morphology triggered by EBV-LMP1 increases cisplatin resistance. Signal Transduct Target Ther 2020;5:56. [Crossref] [PubMed]
- Romani P, Nirchio N, Arboit M, et al. Mitochondrial fission links ECM mechanotransduction to metabolic redox homeostasis and metastatic chemotherapy resistance. Nat Cell Biol 2022;24:168-80. [Crossref] [PubMed]
- Dai W, Wang G, Chwa J, et al. Mitochondrial division inhibitor (mdivi-1) decreases oxidative metabolism in cancer. Br J Cancer 2020;122:1288-97. [Crossref] [PubMed]
- Fang CT, Kuo HH, Yuan CJ, et al. Mdivi-1 induces spindle abnormalities and augments taxol cytotoxicity in MDA-MB-231 cells. Cell Death Discov 2021;7:118. [Crossref] [PubMed]
- Wang J, Hansen K, Edwards R, et al. Mitochondrial division inhibitor 1 (mdivi-1) enhances death receptor-mediated apoptosis in human ovarian cancer cells. Biochem Biophys Res Commun 2015;456:7-12. [Crossref] [PubMed]
- Wang M, Wei R, Li G, et al. SUMOylation of SYNJ2BP-COX16 promotes breast cancer progression through DRP1-mediated mitochondrial fission. Cancer Lett 2022;547:215871. [Crossref] [PubMed]
- Marin-Acevedo JA, Kimbrough EO, Lou Y. Next generation of immune checkpoint inhibitors and beyond. J Hematol Oncol 2021;14:45. [Crossref] [PubMed]
- Wang JB, Qiu QZ, Zheng QL, et al. Tumor Immunophenotyping-Derived Signature Identifies Prognosis and Neoadjuvant Immunotherapeutic Responsiveness in Gastric Cancer. Adv Sci (Weinh) 2023;10:e2207417. [Crossref] [PubMed]
- Hiam-Galvez KJ, Allen BM, Spitzer MH. Systemic immunity in cancer. Nat Rev Cancer 2021;21:345-59. [Crossref] [PubMed]
- Bejarano L, Jordāo MJC, Joyce JA. Therapeutic Targeting of the Tumor Microenvironment. Cancer Discov 2021;11:933-59. [Crossref] [PubMed]
- Zhang Y, Zhang Z. The history and advances in cancer immunotherapy: understanding the characteristics of tumor-infiltrating immune cells and their therapeutic implications. Cell Mol Immunol 2020;17:807-21. [Crossref] [PubMed]
- Kuo CL, Ponneri Babuharisankar A, Lin YC, et al. Mitochondrial oxidative stress in the tumor microenvironment and cancer immunoescape: foe or friend? J Biomed Sci 2022;29:74. [Crossref] [PubMed]
- Xie JH, Li YY, Jin J. The essential functions of mitochondrial dynamics in immune cells. Cell Mol Immunol 2020;17:712-21. [Crossref] [PubMed]
- Tilokani L, Nagashima S, Paupe V, et al. Mitochondrial dynamics: overview of molecular mechanisms. Essays Biochem 2018;62:341-60. [Crossref] [PubMed]
- Simula L, Antonucci Y, Scarpelli G, et al. PD-1-induced T cell exhaustion is controlled by a Drp1-dependent mechanism. Mol Oncol 2022;16:188-205. [Crossref] [PubMed]
- Bao D, Zhao J, Zhou X, et al. Mitochondrial fission-induced mtDNA stress promotes tumor-associated macrophage infiltration and HCC progression. Oncogene 2019;38:5007-20. [Crossref] [PubMed]
- Jang JE, Hwang DY, Eom JI, et al. DRP1 Inhibition Enhances Venetoclax-Induced Mitochondrial Apoptosis in TP53-Mutated Acute Myeloid Leukemia Cells through BAX/BAK Activation. Cancers (Basel) 2023;15:745. [Crossref] [PubMed]
- Kim YY, Um JH, Yoon JH, et al. p53 regulates mitochondrial dynamics by inhibiting Drp1 translocation into mitochondria during cellular senescence. FASEB J 2020;34:2451-64. [Crossref] [PubMed]
- Phadwal K, Tang QY, Luijten I, et al. p53 Regulates Mitochondrial Dynamics in Vascular Smooth Muscle Cell Calcification. Int J Mol Sci 2023;24:1643. [Crossref] [PubMed]
- Kleele T, Rey T, Winter J, et al. Distinct fission signatures predict mitochondrial degradation or biogenesis. Nature 2021;593:435-9. [Crossref] [PubMed]