Deep learning-assisted development and validation of an algorithm for predicting the growth of persistent pure ground-glass nodules
Highlight box
Key findings
• Deep learning (DL)-assisted algorithm could predict the growth of persistent pure ground-glass nodules (pGGNs).
What is known and what is new?
• DL algorithm has been used to diagnose pulmonary nodules.
• We developed and validated a new algorithm to predict growth of pGGNs.
What is the implication, and what should change now?
• By our algorithm, we could classify high and low risk pGGNs, which may allow clinicians to develop earlier treatment strategies for patients.
Introduction
Lung cancer is the leading cause of cancer mortality worldwide (1). Extensive use of high-resolution computed tomography (CT) has resulted in frequent identification of ground-glass nodules (GGNs) (2,3). Most persistent pure GGNs (pGGNs) represent early-stage lung cancers; in particular, persistent lung GGNs may denote atypical adenomatous hyperplasia, adenocarcinoma in situ (AIS), minimally invasive adenocarcinoma (MIA), and invasive adenocarcinoma (IAC) (4,5). However, pGGNs include some rare cases of metastasis of gastric, colorectal, pancreatic, breast, and cholangiocarcinoma (6-10). Therefore, the careful follow-up and the early detection of the growth of tumor is important.
Although pGGNs have similar characteristics on CT imaging, their biological characteristics vary (11,12). Most pGGNs remain stable for long periods, whereas others are more invasive and prone to rapid growth (4,5,13). Therefore, most clinicians adopt a wait-and-see strategy incorporating regular follow-up. Follow-up has a crucial role in clinical decision-making and determining whether surgery is indicated and is therefore increasingly recommended by thoracic and pulmonary specialists. As with most guidelines, the Fleischner Society Guidelines for the management of pGGNs recommend follow-up intervals ranging from 3 to 6 months initially depending on the nodule characteristics, then every 2 years for a total of 5 years, if stable, and surgery if the pGGN or the solid component grows (14). The increased number of detected pGGNs requiring the long-term follow-up results several problems, such as the economic burden, overuse of medical resources, and overexposure to radiation. Therefore, it is necessary and reasonable to extend the follow-up intervals once stable pGGNs have been identified.
Most previous studies have used quantitative CT imaging to determine the size, density, and volume of GGNs and have estimated their invasiveness based on these characteristics (4,5). However, both the assessment of these characteristics and forecasting of pGGN growth have been inaccurate (3,13).
Artificial intelligence in the form of automatic quantitative chest CT imaging has recently been shown to reliably diagnose pulmonary nodules and accurately predict lymph node metastasis (15-18). Artificial intelligence has proven so versatile and efficient in developing deep learning (DL) algorithms from otherwise uninterpretable neural network systems and medical imaging findings that it has been dubbed “Black Box” medicine. The present study aimed to construct and validate a DL classification model (DLCM) based on chest CT imaging findings for predicting the growth of pGGNs. We present this article in accordance with the TRIPOD reporting checklist (available at https://tlcr.amegroups.com/article/view/10.21037/tlcr-23-666/rc).
Methods
Study design and patients
The study was conducted in accordance with the Declaration of Helsinki (as revised in 2013). This two-institution (Institution 1: Beijing Chaoyang Hospital; Institution 2: People’s Liberation Army General Hospital), retrospective cohort study was approved by the institutional review board of Beijing Chaoyang Hospital (IRB No. 2021-ke-135), and written informed consent for this retrospective analysis was waived. The People’s Liberation Army General Hospital was informed and agreed with this study. None of the patients who participated in this study were previously reported. We initially collected consecutive patients with at least two chest-CT scans for pGGNs performed at Institution 1 or Institution 2 from January 2010 to December 2020.
The inclusion criteria were as follows: (I) patients with persistent stable pGGNs and a follow-up of ≥2 years and (II) growing GGNs, no limitation of the follow-up duration. The exclusion criteria were as follows: (I) a confirmed solid nodule on initial chest CT image; (II) transient pGGNs; (III) disappeared pGGNs and suspicion of interstitial lung disease, fibrotic changes, or bronchiolitis; and (IV) cancer history. A flowchart of participant enrollment is shown in Figure 1.
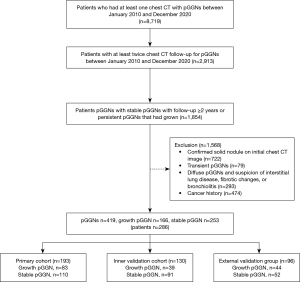
pGGN identification, growth analysis, follow-up time calculation, and region of interest (ROI) placement
pGGNs were identified by one reviewer at two institutions (X.T. and Y.T., 4/12 years of experience of chest images) who was not involved in image interpretation. Consecutive institutional retrospective searches of imaging databases were performed on or before May 31, 2021.
To assess intraobserver reproducibility, two radiologists reviewed all patients’ CT images (initial and last) twice in 1 week [Reader 1 (Tang Y, 12 years of experience of chest images) and Reader 2 (Jin X, 12 years of experience chest images)] and determined whether each pGGN had grown or was stable. Interobserver (Reader 1 vs. Reader 2) reproducibility concerning the status of all pGGNs was also evaluated. The nodules were defined as having grown only when both readers had reached this conclusion twice. Inter- and intraclass correlation coefficients were used to evaluate the agreement between judging that growth had occurred by Reader 1 (twice) and by Reader 2 (twice).
The follow-up time for stable pGGNs was calculated as the interval between the initial and last CT scan. For pGGNs that had grown, the follow-up time was defined as the interval between the initial CT scan and the CT scan in which growth was identified. ROIs the whole pGGNs of all layers, from pGGNs were first segmented by a radiologist (X.T.) by ITK-SNAP software (version 3.6; Penn Image Computing and Science Laboratory at the University of Pennsylvania, Philadelphia, PA, USA; http://www.itksnap.org/pmwiki/pmwiki.php) and later modified by another radiologist (X.J.).
Dataset establishment
All patients in Institution 1 were randomly allocated to the study cohort or inner validation cohorts at a ratio of 4:1, keeping the ratio of stable and growth pGGN data in these cohorts at 1:1. Patients in Institution 2 were all assigned to an independent external validation cohort. In patients who had multiple pGGNs, 40×40 pixel areas of each nodule on each lung CT were extracted separately. Subgroups of patients with pGGNs that had grown in the inner validation cohort were formed based on the duration of follow-up [0–1 year follow-up (D0–1), 1–2 years follow-up (D1–2), and more than 2 years follow-up (D2+)].
Model development, evaluation, and interpretability
We implemented four DLCMs, including Inceptionv3 (19), ResNet18 (20), DenseNet121 (21), and DenseNet_DR. All DLCMs were trained to predict whether pGGNs would grow (22).
We quantified all the DLCM predictive performances by using the receiver operating characteristic (ROC) curve and the area under the ROC curve (AUROC) in the inner validation and external validation cohorts. Moreover, we calculated each model’s AUROC for the following subgroups in the inner validation cohort: D0–1, D1–2, and D2+.
In the testing phase, the DLCM predicted all images of each pGGN and calculated the average predicted probability to obtain the prediction of the growth state of nodules, as shown in Figure 2. By comparing the predictive performance of all models, the model with the best predictive performance was established as the final model in this study. In-depth information about the selected model development is provided in the supplementary material.
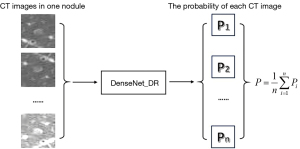
Score-CAM (23) was used to interpret the DLCM. Score-CAM incorporated an increase in confidence in the design of weight for each activation to eliminate the dependence on gradients. The specific process is described in Figure 3.
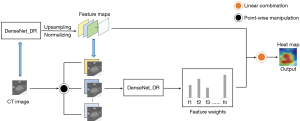
Statistical analysis
Statistical analyses were conducted with R Studio (version 1.3.959; R Project for Statistical Computing, Vienna, Austria; https://www.r-project.org) and Python (version 3.6; Bioinformatics Lab at University of Ljubljana, Ljubljana, Slovenia; https://www.python.org/downloads/release/python-360/), with P<0.05 considered statistically significant. The data are expressed as the means ± standard deviations (SDs) for continuous variables if normally distributed and percentages for categorical variables. Clinical data were analyzed using the Pearson χ2 test for categorical data and the Wilcoxon rank sum test for continuous data. Kappa consistency analysis was used for intra- and intergroup consistency analysis.
Results
Clinical characteristics
We enrolled 286 eligible patients, who had 419 pGGNs, including 253 persistent stable pGGNs and 166 with pGGNs that had grown (Institution 1, primary cohort, 206 patients, 323 pGGNs; Institution 2, external validation cohort, 80 patients, 96 pGGNs). The primary cohort comprised 58 males and 148 females with a mean age of 59.53 (range, 18–93) years, and the external validation cohort comprised 31 males and 49 females with a mean age of 59.26 (range, 33–83) years.
pGGN growth analysis, follow-up time calculation, and ROI placement
Dataset establishment
Among all 419 pGGNs, 168 were classified as growth pGGNs by Reader 1 and 166 by Reader 2; 251 were classified as stable pGGNs by Reader 1 and 253 by Reader 2. In total, two cases with different growth statuses were finally identified as stable. Satisfactory interobserver and intraobserver reproducibility regarding whether growth had occurred was achieved, with the intraobserver and interobserver agreement of 0.96, 0.97, and 0.99, respectively. The follow-up times of stable pGGNs for the primary and external validation cohorts were 3.66 (range, 2.01–10.08) and 4.63 (range, 2.00–9.91) years, respectively.
The maximum diameter, volume, and density of all pGGNs were calculated by the mask placement of the ROI on ITK-SNAP software. The growth pGGN with bigger diameter (8.23 vs. 13.06 mm, P<0.001), larger size (208.52 vs. 830.55 mm3, P<0.001) had a higher CT value [−672.59 vs. −615.89 Hounsfield unit (HU), P<0.001] than the stable pGGN group, similar as previous study (24). Patient characteristics in the two institutions and pGGNs in the study cohort, inner validation cohort, and external validation cohorts are listed respectively in Tables 1,2.
Table 1
Characteristics | Institution 1 (n=206) | Institution 2 (n=80) | Total (n=286) | P value |
---|---|---|---|---|
Age (years) | 59.5±12.7 | 59.3±10.0 | 59.5±12.0 | 0.77 |
Gender | 0.08 | |||
Male | 58 (28.2) | 31 (38.8) | 89 (31.1) | |
Female | 148 (71.8) | 49 (61.3) | 197 (68.9) | |
Surgery | <0.01 | |||
No surgery | 180 (87.4) | 37 (46.3) | 217 (75.9) | |
Surgery | 26 (12.6) | 43 (53.8) | 69 (24.1) | |
Multiple/solitary nodules | 0.01 | |||
Multiple nodule | 48 (23.3) | 8 (10.0) | 56 (19.6) | |
Solitary nodule | 158 (76.7) | 72 (90.0) | 230 (80.4) | |
Nodule number | 1.6±1.6 | 1.2±0.7 | 1.5±1.4 | 0.01 |
CT follow-up times, year | 4.2±2.4 | 3.9±1.8 | 4.1±2.3 | 0.53 |
Values are expressed as n (%) or mean ± SD. Institution 1: Beijing Chaoyang Hospital; Institution 2: People’s Liberation Army General Hospital. CT, computed tomography; SD, standard deviation.
Table 2
Characteristics | Study cohort (n=193) | Inner validation cohort (n=130) | External validation cohort (n=96) | Total (n=419) | P value |
---|---|---|---|---|---|
Follow-up (years) | |||||
Stable pGGNs | 3.59±1.68, n=110 | 3.84±1.41, n=91 | 4.66±2.25, n=52 | 3.90±1.76, n=253 | <0.01 |
Growth pGGNs | 2.80±1.98, n=83 | 2.19±2.40, n=39 | 3.46±2.29, n=44 | 2.83±2.20, n=166 | 0.03 |
Diameter (mm) | 9.4±4.1 | 10.7±15.0 | 10.8±3.9 | 10.1±9.0 | <0.01 |
Volume (mm3) | 452.0±1,165.3 | 417.2±637.4 | 511.9±793.4 | 511.9±793.4 | <0.01 |
CT value (HU) | −654.3±107.6 | −654.4±109.7 | −635.9±108.3 | −635.9±108.3 | 0.14 |
Location | 0.28 | ||||
Left upper lobe | 42 (21.8) | 33 (25.4) | 26 (27.1) | 101 (24.1) | |
Left lower lobe | 19 (9.8) | 23 (17.7) | 7 (7.3) | 49 (11.7) | |
Right upper lobe | 90 (46.6) | 54 (41.5) | 44 (45.8) | 188 (44.9) | |
Right middle lobe | 14 (7.3) | 5 (3.8) | 7 (7.3) | 26 (6.2) | |
Right lower lobe | 28 (14.5) | 15 (11.5) | 12 (12.5) | 55 (13.1) | |
Cell type | <0.01 | ||||
AIS | 4 (2.1) | 4 (3.1) | 4 (4.2) | 12 (2.9) | |
MIA | 1 (0.5) | 1 (0.8) | 10 (10.4) | 12 (2.9) | |
IAC | 9 (4.7) | 7 (5.4) | 31 (32.3) | 47 (11.2) | |
No surgery | 179 (92.7) | 118 (90.8) | 51 (53.1) | 51 (53.1) |
Values are expressed as n (%) or mean ± SD. pGGN, pure ground-glass nodule; CT, computed tomography; HU, Hounsfield unit; AIS, adenocarcinoma in situ; MIA, minimally invasive adenocarcinoma; IAC, invasive adenocarcinoma; SD, standard deviation.
Model establishment and prediction in the internal validation cohort
Four models were used to predict the growth of pulmonary nodules: Inceptionv3 (19), ResNet18 (20), DenseNet121 (21), and DenseNet_DR. The performance of the four feature extractors was as follows: the AUROC was 0.54 for Inceptionv3, 0.63 for ResNet18, 0.76 for DenseNet121, and 0.79 for DenseNet_DR (Figure 4A). The performance of DenseNet_DR was significantly better than that of the other model and established as the predicting model in our study. The detailed number of nodules images used in the training and validation set is provided in the supplementary material.
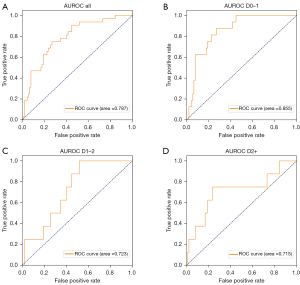
Prediction in subgroups, in external validation cohort, and interpretability of model prediction decision
The use of DenseNet_DR for predicting the growth of pulmonary nodules was more accurate in the D0–1 (AUROC =0.855, Figure 4B) than in the D1–2 (AUROC =0.723, Figure 4C), and D2+ (AUROC =0.715, Figure 4D) subgroups (Table 3). DenseNet_DR achieved an AUROC of 0.70 [95% confidence interval (CI): 0.60, 0.79, Figure 5] in the external validation cohort. DenseNet_DR performed worse in the external validation cohort than in the internal validation cohort, but the AUROC was similar to that for the D2+ subgroup. Feature-weighted activation maps overlaid with CT images showed that DenseNet_DR focused its attention on the pulmonary nodules and periphery of nodules (Figure 6).
Table 3
Subgroup | AUC | ACC | SENS | SPEC | PPV | NPV |
---|---|---|---|---|---|---|
Internal validation cohort | 0.787 | 0.727 | 0.719 | 0.730 | 0.489 | 0.878 |
D0–1 | 0.855 | 0.743 | 0.813 | 0.731 | 0.351 | 0.956 |
D1–2 | 0.723 | 0.711 | 0.500 | 0.730 | 0.143 | 0.942 |
D2+ | 0.715 | 0.732 | 0.750 | 0.730 | 0.200 | 0.970 |
DLCM, deep learning classification model; AUC, area under the curve; ACC, accuracy; SENS, sensitivity; SPEC, specification; PPV, positive predictive value; NPV, negative predictive value; D0–1, 0–1 year follow-up; D1–2, 1–2 years follow-up; D2+, more than 2 years follow-up.
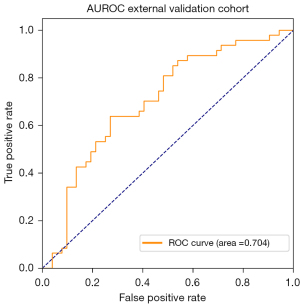
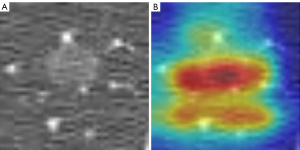
Discussion
In our study, the DLCM accurately predicted the growth of pGGNs in the inner validation cohort (AUROC =0.79) and somewhat less accurately in the external validation cohort (AUROC =0.70). The performance of our proposed DLCM indicates that it could provide an alternative to current prediction methods, which use long-term serial CT findings to assess growth risk in patients with persistent pGGNs.
In this study, the prediction accuracy of subgroup D0–1 was superior to that of the other two subgroups (D0–1 vs. D1–2, D2+; AUROC, 0.855 vs. 0.723, 0.715). The pGGNs can be stable for a long time, and their identifying characteristics may be most obvious when they become unstable or about to become unstable within one year of their identification (4,13,25-29). The CT image characteristics of pGGNs that remain stable over long periods before eventually growing probably differ considerably from the CT characteristics of those that grow soon after detection (5,11,30). This may explain why prediction is more accurate in pGGN growth within a short follow-up than within a long follow-up.
The diagnostic performance of DLCM was lower in the external validation cohort than in the inner validation cohort (AUROC, 0.70 vs. 0.79) but was nonetheless encouraging. Explanations for this discrepancy were different follow-up times and different image parameters between the two cohorts. The average follow-up time of growth pGGNs for the external validation cohort (3.46 years) was longer than for the inner validation cohort (2.19 years), and the diagnostic performance of DLCM is better in pGGN growth within short follow-ups. Differences in image conditions, such as window width and window level, were observed between the inner and external validation cohorts. The window width and level were mostly 1,800 and 800 HU, respectively, in the inner cohort, whereas they were 1,500 and 800 HU, respectively, in the external cohort. The different parameters for CT imaging cause ineradicable differences in baselines for all images inputted into the DLCM. What’s more, the follow-up protocol is not rigid and it is determined by each physician according to the preference.
In this study, we chose 2 years of follow-up as the cutoff for defining stable pGGNs, which is one of the limitations of this study. Despite this, most growth of pGGNs occurs within 2 years (25.5–51.9%) or 3 years (41.0–86.0%) of its detection reported in previous studies (11,27,29-35). In the current study, 157 (62.1%) stable pGGNs were followed up for more than 3 years.
In previous studies, the growth of pGGNs had been defined as at least a 20% increase in volume or ≥2 mm increase in diameter (26,36-38) between follow-ups. However, it is inaccurate in measurement because of the partial volume effect, especially for small pGGNs, which would cause classification errors. In this study, we minimized classification error by defining growth based on independent assessments by two radiologists rather than based on simple increases in volume or diameter. There was 0.99 agreement between the two radiologists, demonstrating high consistency and low classification error.
Most previous studies have excluded patients with multiple pGGNs or chose the largest pGGN to represent all pGGNs in a single individual (5,13,39,40). In the present study, we included multiple pGGNs and evaluated them independently for two reasons. First, multiple pGGNs comprise approximately 35% of newly discovered pGGNs (27,29,41,42). It would therefore result in a large selection bias if we excluded multiple pGGNs. Second, in patients with multiple pGGNs, the largest lesions and those with a high risk of growth are recommended to be simultaneously resected. Therefore, it is important to identify each growing pGGN among multiple pGGNs.
We acknowledge that this study had limitations. First, the CT scan protocol and follow-up times of enrolled patients were heterogeneous because of the nature of the retrospective study. Second, 24.48% of all patients underwent surgical resection, and only 29.8% with growing pGGNs patients were underwent survival resection, which means that most pGGNs were not pathologically diagnosed. What’s more, the rate of surgical procedure is different between inner cohort and external validation cohort. Third, the follow-up period for stable pGGNs was not long enough, which may have resulted in bias.
Conclusions
In conclusion, a DLCM that directly analyzes pGGN identified on chest CT images could facilitate the prediction of the growth of pGGNs. Considering all the limitations and the potential sources of bias, we believe further research and a larger database are required to validate and optimize our model.
Acknowledgments
Funding: This study was funded by
Footnote
Reporting Checklist: The authors have completed the TRIPOD reporting checklist. Available at https://tlcr.amegroups.com/article/view/10.21037/tlcr-23-666/rc
Data Sharing Statement: Available at https://tlcr.amegroups.com/article/view/10.21037/tlcr-23-666/dss
Peer Review File: Available at https://tlcr.amegroups.com/article/view/10.21037/tlcr-23-666/prf
Conflicts of Interest: All authors have completed the ICMJE uniform disclosure form (available at https://tlcr.amegroups.com/article/view/10.21037/tlcr-23-666/coif). The authors have no conflicts of interest to declare.
Ethical Statement: The authors are accountable for all aspects of the work in ensuring that questions related to the accuracy or integrity of any part of the work are appropriately investigated and resolved. The study was conducted in accordance with the Declaration of Helsinki (as revised in 2013). This two-institution, retrospective cohort study was approved by the institutional review board of Beijing Chaoyang Hospital (IRB No. 2021-ke-135), and written informed consent for this retrospective analysis was waived. The People’s Liberation Army General Hospital was informed and agreed with this study.
Open Access Statement: This is an Open Access article distributed in accordance with the Creative Commons Attribution-NonCommercial-NoDerivs 4.0 International License (CC BY-NC-ND 4.0), which permits the non-commercial replication and distribution of the article with the strict proviso that no changes or edits are made and the original work is properly cited (including links to both the formal publication through the relevant DOI and the license). See: https://creativecommons.org/licenses/by-nc-nd/4.0/.
References
- Siegel RL, Miller KD, Jemal A. Cancer statistics, 2019. CA Cancer J Clin 2019;69:7-34. [Crossref] [PubMed]
- Zhang Y, Fu F, Chen H. Management of Ground-Glass Opacities in the Lung Cancer Spectrum. Ann Thorac Surg 2020;110:1796-804. [Crossref] [PubMed]
- Hattori A, Takamochi K, Oh S, et al. Prognostic Classification of Multiple Primary Lung Cancers Based on a Ground-Glass Opacity Component. Ann Thorac Surg 2020;109:420-7. [Crossref] [PubMed]
- Lee JH, Park CM, Lee SM, et al. Persistent pulmonary subsolid nodules with solid portions of 5 mm or smaller: Their natural course and predictors of interval growth. Eur Radiol 2016;26:1529-37. [Crossref] [PubMed]
- Qi LL, Wu BT, Tang W, et al. Long-term follow-up of persistent pulmonary pure ground-glass nodules with deep learning-assisted nodule segmentation. Eur Radiol 2020;30:744-55. [Crossref] [PubMed]
- Ryuko T, Sano Y, Kitazawa R, et al. Lung Metastasis From Thyroid Carcinoma Showing a Pure Ground-Glass Nodule. Ann Thorac Surg 2022;114:e253-6. [Crossref] [PubMed]
- Kunikata S, Yokomura K, Matsui T, et al. A case of metastatic lung tumor with multiple ground glass opacities on chest CT. Nihon Kokyuki Gakkai Zasshi 2010;48:298-302. [PubMed]
- Nagayoshi Y, Yamamoto K, Hashimoto S, et al. An Autopsy Case of Lepidic Pulmonary Metastasis from Cholangiocarcinoma. Intern Med 2016;55:2849-53. [Crossref] [PubMed]
- Kim SB, Lee S, Koh MJ, et al. Ground-glass opacity in lung metastasis from breast cancer: a case report. Tuberc Respir Dis (Seoul) 2013;74:32-6. [Crossref] [PubMed]
- Kundu S, Murphy J, Towers M, et al. Computed tomographic demonstration of very-low-density pulmonary nodules in metastatic gastric carcinoma: case report. Can Assoc Radiol J 1999;50:198-201. [PubMed]
- Aoki T. Growth of pure ground-glass lung nodule detected at computed tomography. J Thorac Dis 2015;7:E326-8. [PubMed]
- Detterbeck FC, Gibson CJ. Turning gray: the natural history of lung cancer over time. J Thorac Oncol 2008;3:781-92. [Crossref] [PubMed]
- Tang EK, Chen CS, Wu CC, et al. Natural History of Persistent Pulmonary Subsolid Nodules: Long-Term Observation of Different Interval Growth. Heart Lung Circ 2019;28:1747-54. [Crossref] [PubMed]
- Bankier AA, MacMahon H, Goo JM, et al. Recommendations for Measuring Pulmonary Nodules at CT: A Statement from the Fleischner Society. Radiology 2017;285:584-600. [Crossref] [PubMed]
- Wang X, Zhao X, Li Q, et al. Can peritumoral radiomics increase the efficiency of the prediction for lymph node metastasis in clinical stage T1 lung adenocarcinoma on CT? Eur Radiol 2019;29:6049-58. [Crossref] [PubMed]
- Ather S, Kadir T, Gleeson F. Artificial intelligence and radiomics in pulmonary nodule management: current status and future applications. Clin Radiol 2020;75:13-9. [Crossref] [PubMed]
- Ding H, Xia W, Zhang L, et al. CT-Based Deep Learning Model for Invasiveness Classification and Micropapillary Pattern Prediction Within Lung Adenocarcinoma. Front Oncol 2020;10:1186. [Crossref] [PubMed]
- Gong J, Liu J, Hao W, et al. A deep residual learning network for predicting lung adenocarcinoma manifesting as ground-glass nodule on CT images. Eur Radiol 2020;30:1847-55. [Crossref] [PubMed]
- Szegedy C, Vanhoucke V, Ioffe S, et al. Rethinking the inception architecture for computer vision. In: Proceedings of the IEEE Conference on Computer Vision and Pattern Recognition; 2016:2818-26.
- He K, Zhang X, Ren S, et al. Deep residual learning for image recognition. In: Proceedings of the IEEE Conference on Computer Vision and Pattern Recognition; 2016:770-8.
- Huang G, Liu Z, Van Der Maaten L, et al. Densely connected convolutional networks. In: Proceedings of the IEEE Conference on Computer Vision and Pattern Recognition; 2017:4700-8.
- Ge G, Zhang J. Feature selection methods and predictive models in CT lung cancer radiomics. J Appl Clin Med Phys 2023;24:e13869. [Crossref] [PubMed]
- Wang H, Wang Z, Du M, et al. Score-CAM: Score-weighted visual explanations for convolutional neural networks. In: Proceedings of the IEEE/CVF Conference on Computer Vision and Pattern Recognition Workshops; 2020:24-5.
- Heidinger BH, Anderson KR, Nemec U, et al. Lung Adenocarcinoma Manifesting as Pure Ground-Glass Nodules: Correlating CT Size, Volume, Density, and Roundness with Histopathologic Invasion and Size. J Thorac Oncol 2017;12:1288-98. [Crossref] [PubMed]
- Kobayashi Y, Sakao Y, Deshpande GA, et al. The association between baseline clinical-radiological characteristics and growth of pulmonary nodules with ground-glass opacity. Lung Cancer 2014;83:61-6. [Crossref] [PubMed]
- Song YS, Park CM, Park SJ, et al. Volume and mass doubling times of persistent pulmonary subsolid nodules detected in patients without known malignancy. Radiology 2014;273:276-84. [Crossref] [PubMed]
- Cho J, Kim ES, Kim SJ, et al. Long-Term Follow-up of Small Pulmonary Ground-Glass Nodules Stable for 3 Years: Implications of the Proper Follow-up Period and Risk Factors for Subsequent Growth. J Thorac Oncol 2016;11:1453-9. [Crossref] [PubMed]
- Kakinuma R, Noguchi M, Ashizawa K, et al. Natural History of Pulmonary Subsolid Nodules: A Prospective Multicenter Study. J Thorac Oncol 2016;11:1012-28. [Crossref] [PubMed]
- Sato Y, Fujimoto D, Morimoto T, et al. Natural history and clinical characteristics of multiple pulmonary nodules with ground glass opacity. Respirology 2017;22:1615-21. [Crossref] [PubMed]
- Eguchi T, Adusumilli PS. Risk stratification for lung nodules: Size isn't everything. J Thorac Cardiovasc Surg 2017;153:1557-62. [Crossref] [PubMed]
- Matsuguma H, Mori K, Nakahara R, et al. Characteristics of subsolid pulmonary nodules showing growth during follow-up with CT scanning. Chest 2013;143:436-43. [Crossref] [PubMed]
- Lim HJ, Ahn S, Lee KS, et al. Persistent pure ground-glass opacity lung nodules ≥10 mm in diameter at CT scan: histopathologic comparisons and prognostic implications. Chest 2013;144:1291-9. [Crossref] [PubMed]
- Lee SW, Leem CS, Kim TJ, et al. The long-term course of ground-glass opacities detected on thin-section computed tomography. Respir Med 2013;107:904-10. [Crossref] [PubMed]
- Chang B, Hwang JH, Choi YH, et al. Natural history of pure ground-glass opacity lung nodules detected by low-dose CT scan. Chest 2013;143:172-8. [Crossref] [PubMed]
- Takahashi S, Tanaka N, Okimoto T, et al. Long term follow-up for small pure ground-glass nodules: implications of determining an optimum follow-up period and high-resolution CT findings to predict the growth of nodules. Jpn J Radiol 2012;30:206-17. [Crossref] [PubMed]
- de Hoop B, Gietema H, van de Vorst S, et al. Pulmonary ground-glass nodules: increase in mass as an early indicator of growth. Radiology 2010;255:199-206. [Crossref] [PubMed]
- Jiang Y, Xiong Z, Zhao W, et al. Pathological components and CT imaging analysis of the area adjacent pleura within the pure ground-glass nodules with pleural deformation in invasive lung adenocarcinoma. BMC Cancer 2022;22:958. [Crossref] [PubMed]
- Wu Y, Chen B, Su L, et al. Diagnostic value of double low-dose targeted perfusion CT imaging for the diagnosis of invasive and preinvasive pulmonary ground-glass nodules: systematic review and meta-analysis. Transl Cancer Res 2022;11:2823-33. [Crossref] [PubMed]
- Elia S, Pompeo E, Santone A, et al. Radiomics and Artificial Intelligence Can Predict Malignancy of Solitary Pulmonary Nodules in the Elderly. Diagnostics (Basel) 2023;13:384. [Crossref] [PubMed]
- Bongiolatti S, Corzani R, Borgianni S, et al. Long-term results after surgical treatment of the dominant lung adenocarcinoma associated with ground-glass opacities. J Thorac Dis 2018;10:4838-48. [Crossref] [PubMed]
- Kobayashi Y, Fukui T, Ito S, et al. How long should small lung lesions of ground-glass opacity be followed? J Thorac Oncol 2013;8:309-14. [Crossref] [PubMed]
- Kim TJ, Goo JM, Lee KW, et al. Clinical, pathological and thin-section CT features of persistent multiple ground-glass opacity nodules: comparison with solitary ground-glass opacity nodule. Lung Cancer 2009;64:171-8. [Crossref] [PubMed]