In-depth proteomic analysis identifies key gene signatures predicting therapeutic efficacy of anti-PD-1/PD-L1 monotherapy in non-small cell lung cancer
Highlight box
Key findings
• The proteomic gene expression profile of MOXD1, PHAF1, KRT7, ANKRD30A, TMEM184A, KIR3DL1, and KCNK4 could better predict the durable response to anti-programmed cell death 1 (PD-1)/programmed cell death ligand 1 (PD-L1) therapy among non-small cell lung cancer (NSCLC) patients.
What is known and what is new?
• PD-L1 is currently a widely accepted biomarker for predicting the efficacy of immunotherapy. Nonetheless, relying solely on PD-L1 as a single biomarker may not be sufficient to identify the suitable treatment population for immunotherapy due to the highly complex tumor microenvironment of NSCLC patients.
• Immunotherapy efficacy-based proteomic gene panel could further improve prediction accuracy for the efficacy of PD-1/PD-L1-based immunotherapy among NSCLC patients.
What is the implication, and what should change now?
• The rationale for using proteomics to develop proteomic gene signatures in predicting NSCLC patients suitable for immunotherapy has been established in this study.
• Future proteomic analyses with larger sample size should be conducted to further confirm the clinical application value of proteomic gene panels in predicting the efficacy of immunotherapy among NSCLC patients.
Introduction
Lung cancer is a malignant tumor that seriously endangers human health (1,2). Non-small cell lung cancer (NSCLC) is the most common type and accounts for about 80–85% of lung cancer (3). With the introduction of immune checkpoint inhibitor (ICI) therapy, the programmed cell death 1 (PD-1)/programmed cell death ligand 1 (PD-L1) blockade prolongs 5-year survival rate from 4% to 16% in patients with pretreated advanced NSCLC (4). To date, anti-PD-1/PD-L1 therapy has become the standard first-line option for driver gene-negative NSCLC (5). Although PD-L1 is currently a widely accepted biomarker for predicting the efficacy of immunotherapy, relying solely on PD-L1 as a single biomarker may not be sufficient to identify the suitable treatment population for immunotherapy due to the highly complex tumor microenvironment (TME). The comprehensive scoring system based on genomics mutation, such as tumor mutational burden (TMB) and T cell-inflamed gene expression profile (GEP), could be excellent supplements to efficacy prediction (6). However, more evidence on transcriptomic or proteomic level is still required to further confirm the immune status of patients since the routinely utilized predictive biomarkers or prediction models are still not accurate enough.
The immune state is dynamic, whereas genetic mutations at the DNA level are relatively stable. Nonetheless, proteins can reflect the changes in the immune system over a period of time, so as to more dynamically track the real-time status of patients. Screening for proteins that can affect the anti-tumoral environment may therefore be the key to exploring biomarkers of therapeutic response to ICIs. To date, some studies have been conducted to investigate such biomarkers at the protein level, including tumor neoantigen and immune checkpoint proteins (ICPs) in the TME. The prediction of neoantigens mainly depends on gene mutation burden (7). In terms of ICPs, similar to PD-L1, they can be utilized to evaluate cellular components or immune status in the TME, but a large number of clinically relevant genes are still neglected (8,9). Therefore, it is imperative to investigate the protein components in the TME through proteomics to identify the potential therapeutic biomarkers of ICIs.
In this study, data were obtained from 50 patients with NSCLC who were treated with anti-PD-1/PD-L1 monotherapy and subjected to high-throughput proteomic/transcriptomic profiling. Among them, the data of 23 NSCLC patients were from our institution, and their primary lung lesions were examined by protein mass spectrometry (MS) (SHFK cohort). Data for the remaining 27 patients were from GSE135222 with the RNA-seq analysis data (GSE135222 cohort) (10). By combining differential gene screening and weighted correlation network analysis (WGCNA), a total of 43 gene modules positively correlated to efficacy were screened. Secondly, the elastic network, a machine learning model, was used to screen the input variables and three key gene modules were obtained. By using gene expression-mutation association analysis, 59 mutated genes coexisting with our selected genes were located. Finally, investigation of the Memorial Sloan-Kettering Cancer Center (MSKCC) ICI cohort confirmed that patients with these gene signatures had prolonged progression-free survival (PFS), indicating a durable clinical response (11,12). Moreover, our patient-specific protein network further confirmed the practically significant protein-protein interaction (PPI) of selected gene panels. We present this article in accordance with the TRIPOD and REMARK reporting checklists (available at https://tlcr.amegroups.com/article/view/10.21037/tlcr-23-713/rc).
Methods
Patients
This work was carried out in accordance with Declaration of Helsinki (as revised in 2013), and was approved by ethics committee of Shanghai Pulmonary Hospital (No. L20-337Y). Written consents were obtained from all enrolled participants. Twenty-three patients included in this study were diagnosed as NSCLC without targetable driver gene mutations, and received anti-PD-1 or anti-PD-L1 monotherapy as second-line or above therapy (Table S1). Samples were collected before immunotherapy. All samples were paraffin-embedded or stored at −80 °C. Patients were hospitalized regularly during treatment for the assessment of therapeutic efficacy and physical status. The response to immunotherapy was determined according to Response Evaluation Criteria in Solid Tumors version 1.1 (RECIST V.1.1) (13). Durable clinical benefit (DCB) was defined as patients with complete response (CR), partial response (PR) or stable disease (SD) for at least six months. PFS was calculated as the time from the first follow-up to the first imaging evidence of disease progression or death.
Protein sample preparation and MS analyses
All lung lesions were collected by fine needle biopsy under computed tomography (CT) guidance and pathologically confirmed. All samples were processed in the same proteomic platform with different pre-processing procedure. For paraffin-embedded sections, samples were de-paraffinized using xylene, and sequentially hydrated for three times with anhydrous ethanol, 90% and 75% ethanol. The frozen samples were rinsed twice with iced phosphate buffer saline (PBS) and grounded in liquid nitrogen. The lysis solution with protease inhibitor (0.1 M Tris-HCL, PH 8.0, 0.1 M DTT) was mixed with sample grinds or de-paraffinized samples, and homogenized on ice. Sodium dodecyl sulfate was added until a final concentration of 4%, followed by incubation at 99 °C for 1 h. The supernatant was centrifuged and added to acetone, followed by incubation at −20 °C overnight. The protein samples were re-dissolved using 8 M urea, followed by reductive alkylation. The samples were then lysed with protease at 37 °C for 16 h. After termination, the samples were desalted using C18 columns and lyophilized for quantification. All samples were assayed using hybrid trapped ion mobility spectrometry (TIMS) coupled with quadrupole time-of-flight MS. MS data were searched in the Swiss-prot database.
Screening for differential genes and construction of a WGCNA network
The WGCNA network was constructed and the differentially expressed genes were analyzed in the SHFK and GSE135222 cohorts, respectively. The protein expression matrix was column-wise normalized before computation. For the construction of gene network, genes with missing expression levels or zero variance were deleted. Hierarchical clustering was performed for the samples and outliers were removed. PFS was used to associate with the gene modules in the weighted network to construct the gene-clinical feature correlation matrix. Since the PFS of patients in the GSE135222 cohort was not available, the DCB and non-durable clinical benefit (NDB) of patients were used as surrogate endpoints. The number 1 was used to represent patients with DCB, indicating a possibly longer PFS. Differentially expressed genes were analyzed by comparing DCB and NDB in each cohort. Genes with an absolute value of LogFC equal to or greater than 1.5 and P-adj less than 0.1 were considered as significantly up-regulated or down-regulated genes. We compared and matched these genes with gene modules, and filtered out the unmatched genes. The R package deseq2 (1.38.3) and WGCNA (1.72-1) were used for analysis.
Gene module screening and The Cancer Genome Atlas (TCGA) cohort validation
A total of 43 gene characteristic modules obtained from the WGCNA network were integrated with seven immune-related genes from the literature (14). The Gene Set Variation Analysis (GSVA) scores of 50 samples were calculated in the cohort, and used as the independent variable to build the elastic network. With the finding of the minimum α value, a stable model was built to predict the efficacy. Three independent gene modules that contributed the most to DCB were selected as the efficacy-dependent variables. The cut-off value was calculated in the cohort. Then, GSVA values were calculated for the TCGA-lung adenocarcinoma (LUAD)/lung squamous cell carcinoma (LUSC) cohort with the above three selected gene modules, followed by survival analysis. At the same time, through Gene Set Cancer Analysis (GSCA) analysis of the three modules, the correlation between the three modules and main signal pathway or immune cell components was calculated (15). Finally, gene modules without statistically significant differences in hazard ratio (HR) were filtered out by Cox regression analysis. We also screened gene modules closely relevant to T cells, B cells, cytotoxic T cells and other immune cells promoting anti-tumor immunity.
Association analysis of gene expression and mutations and further validation in MSKCC ICI cohort
The relationship between gene expression and gene mutations was verified. By using TCGA cohort, the cohort with genes mutation status was first subdivided and the expression differences were determined between the mutant/wild type of these screened genes, by the means of muTarget method (16). Then, the mutant genes in the group of highly expressed genes screened were selected as the gene panel for subsequent MSKCC cohort validation (11,12). Also, these mutation genes were further screened and verified in the MSKCC cohort according to the mutation rates. Data and visualization were acquired via cbioportal (accessed in December 2022).
Patient-specific PPI network construction and modification
Individualized PPI network was constructed to demonstrate the differences between DCB and NDB cohorts, using PPI Cytoscape 3.7.1. TME involves inter-individual differences, and the correlation between each gene pair indicates individual proximity. Briefly, each gene pair between DCB and NDB cohorts was determined by calculating the Pearson correlation index (PCI), as previously reported (17). If the PCI perturbation was significant, we considered that we obtained a solid gene pair. This process was repeated for each gene, eventually yielding a patient-specific network. According to this analysis, a patient-specific protein-pair network was generated for every DCB sample. Each PPI network was curated by proved/existing protein interactions. Four databases of protein interactions were utilized (STRING, PROPER, PREPPI and Biogrid, downloaded in June 2022). Briefly, patients’ gene pairs were compared with the curated PPI datasets. Gene pairs with existing PPI were remained, while others were removed for unknown protein-level interactions.
Statistical analysis and bioinformatics methods
In this study, R (4.1.0) was used for statistical analysis and plotting. Unless otherwise stated, a P value of less than 0.05 was considered statistically significant in this study.
Results
Forty-three gene modules were obtained through joint screening of gene modules
The designed data analysis flow is depicted in Figure 1A. Through WGCNA network and differential gene analysis, a total of 43 differential gene-modules were obtained from two cohorts (tables are available at https://cdn.amegroups.cn/static/public/tlcr-23-713-1.xlsx; https://cdn.amegroups.cn/static/public/tlcr-23-713-2.xlsx; https://cdn.amegroups.cn/static/public/tlcr-23-713-3.xlsx, Figure 1B,1C, Figure 2A,2B). Among these differential genes, the correlation between gene modules and PFS could be inferred by correlation coefficient (Figure 1B,1C). Gene modules were mapped with differentially expressed genes, and only matched genes remained. Of note, in the GSE135222 cohort, due to the lack of PFS corresponding to the original data, the DCB and NDB of patients were used as surrogate endpoints that the corresponding PFS of the DCB and NDB groups were set to 1 and 0, respectively. Therefore, we could approximate the coefficients related to PFS. In the subsequent elastic network modeling, the gene modules negatively related to PFS were not eliminated, because the inclusion of all these relevant gene modules was conducive to further analysis.
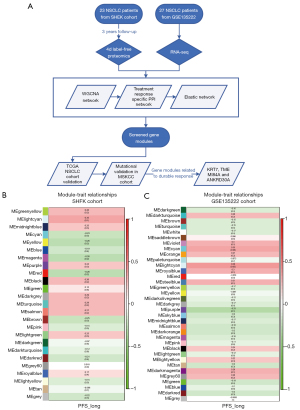
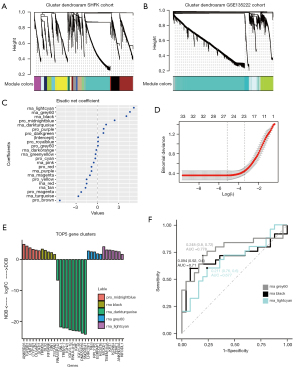
Three gene modules related to DCB could better predict response to ICI
Through the model of elastic network, three gene modules with highest coefficients related to the durable response of ICI were identified, including rna_lightcyan, rna_grey60, and rna_black (Figure 2C,2D). These three gene modules were the first three modules with the largest coefficients in the model, all of which were from the GSE135222 cohort. All three gene modules had positive LogFC values, and were positively related to the DCB group (Figure 2E). The GSVA values of these three gene modules were calculated for both cohorts, and the results showed that these three indicators could predict the therapeutic response. Among them, the rna_grey60 module had the maximum area under the curve (AUC) (Figure 2F). Furthermore, the molecular functions of the signaling pathway corresponding to the three gene modules were confirmed. The signaling pathway enrichment showed that the black and lightcyan modules had significant correlations with the main signaling pathway modules (Figure 3A). In the immune cell enrichment analysis, the lightcyan module was positively related to B cells, CD4+ T cells, memory cells, natural killer (NK) cells, natural killer T (NKT) cells, and macrophages (Figure 3B). Therefore, from the perspective of molecular function, the anti-tumor immune functions corresponding to the lightcyan gene module were more meaningful and effective.
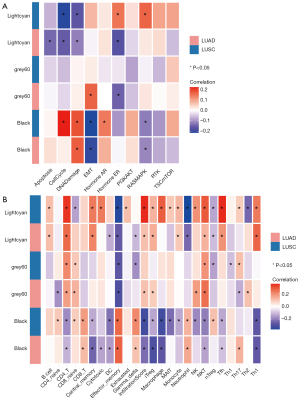
Three gene modules were not related to survival and prognosis
Through the TCGA-NSCLC cohort (LUAD + LUSC), we further confirmed that these three gene modules were not factors affecting the survival of patients. By comparing the patient cohorts, the difference in overall survival was not statistically significant between the high-expression group and low-expression group of these three genes modules (Figure S1A-S1C). Further Cox regression analysis indicated that these three gene modules did not possess significant HRs for the model, but the lightcyan gene module had a relatively smaller confidence interval (Figure S1D). Based on the above results, the lightcyan module was selected for further exploration.
Investigation of the mutated genes related to lightcyan gene module
By exploring the mutated genes associated with high expression of the gene module, the accuracy of gene module in predicting the therapeutic response to ICI was further verified. Based on the muTarget method and TCGA-LUAD/LUSC cohort, the corresponding mutated genes were identified through the high expression of these module genes (tables are available at https://cdn.amegroups.cn/static/public/tlcr-23-713-4.xlsx; https://cdn.amegroups.cn/static/public/tlcr-23-713-5.xlsx). For example, in the comparison of mutant type and wild type of DSTYK gene, the results showed that the expression of ANKRD30A was significantly higher in mutant type of DSTYK gene, and then DSTYK gene would be included into further analysis.
Mutated gene panel predicted durable therapeutic response to ICI in MSKCC cohort
Furthermore, the existence of these 396 mutated genes was further verified in the MSKCC cohort. The list of mutated genes was screened, and the genes without mutation or were related to NDB were removed, finally yielding the list of 59 genes (tables are available at https://cdn.amegroups.cn/static/public/tlcr-23-713-4.xlsx; https://cdn.amegroups.cn/static/public/tlcr-23-713-5.xlsx). Patients with mutations in this gene panel had a longer PFS after ICI treatment, compared with patients with the wild type (Figure 4A). In fact, these mutations were also accompanied by mutations in other conventional genes, such as TP53, TTN, KRAS, etc. (Figure 4B). Compared with patients with this wild-type gene panel, those with the mutant type had significantly increasing mutation load and TMB. Meanwhile, the neoantigen burden in the mutant population was significantly higher than that in the wild-type population (Figure 4C-4F). Therefore, the mutant gene panel associated with the expression profile in the lightcyan gene module could effectively predict the therapeutic response to ICI, which also validated the predictive effect of this gene module.
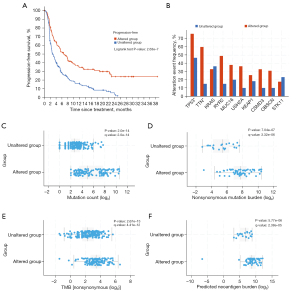
Lightcyan gene module showed interaction network of multiple genes in patient PPI network
The genes in the lightcyan module were introduced into the individualized gene interaction network of DCB patients, in which KRT7, TMEM184A and ANKRD30A were enriched (Figure 5A-5C). With these three genes as the center, an interaction network was formed. These interacting genes were closely related to the three module genes and the durable response to ICI treatment.
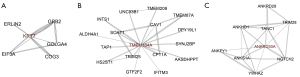
Discussion
NSCLC stands at the frontline of precision oncology. For patients with negative driver gene mutations, anti-PD-1-based immunotherapy is a promising option. Nevertheless, the heterogeneity of cancer patients results in various therapy efficacies and deciding whether to receive ICI is momentous. Previous studies reported that high expression levels of PD-L1 and TMB were related to better response to ICI for NSCLC populations (18,19). However, protein components in TME also play a crucial role in ICI for malignant cancers. Previous prediction models based on clinical characteristics or pathological information of tumors had restrained generalization performance. Consequently, to identify key proteomic gene signatures affecting the efficacy of anti-PD-1/PD-L1 treatment in NSCLC patients, we combined and analyzed the multi-omics data from two clinical cohorts based on the machine learning model, including transcriptomic data from GSE135222 cohort and proteomic data from SHFK cohort. We demonstrated that the prediction model with seven genes, including MOXD1, PHAF1, KRT7, ANKRD30A, TMEM184A, KIR3DL1, and KCNK4, could better predict the durable response to anti-PD-1/PD-L1 therapy, which were also validated in the MSKCC ICI cohort, further indicating its clinical application value.
To date, the predictive value of PD-L1 expression or TMB has been very limited. Due to the heterogeneity of NSCLC, a single efficacy predictor can only summarize the characteristic of TME and potential therapeutic effect from one dimension. In clinical practice, some patients have very high PD-L1 expression (or high TMB levels) but do not have a good response to anti-PD-1/PD-L1 therapy. Conversely, some patients are not positive for these biomarkers but achieve a better response to treatment. Therefore, the detection of immunotherapeutic markers should be further improved. On the translational level, many proteins in the TME are related to anti-tumor immunity, including other immune checkpoints, immune-activating molecules, and other membrane proteins associated with antitumor activity. Molecular markers at the protein level are of practical interest, both for evaluating immune components in the TME and for guiding the therapy combined with other ICIs. In this study, we identified three gene modules that mostly related to therapeutic response from all 43 gene modules obtained by joint screening of proteomic profile and RNA-seq data from two cohorts. Among them, the black and lightcyan modules had significant correlations with the main signaling pathway module while the lightcyan module was found to be further positively related to B cells, CD4+ T cells, memory cells, NK cells, NKT cells and macrophages in the immune cell enrichment analysis. Further investigation centering on the lightcyan module demonstrated that mutated genes related to lightcyan gene module could effectively stratify the PFS among patients receiving ICI treatment in the MSKCC cohort, exhibiting positive associations with mutation counts, nonsynonymous mutation burden, TMB and predicted neoantigen burden. Furthermore, the PPI network analysis showed strong correlation between KRT7, TMEM184A and ANKRD30A in the lightcyan gene module and an interaction network around them was constructed.
Overall, our evidence supported that the lightcyan module, including the expression profile of MOXD1, PHAF1, KRT7, ANKRD30A, TMEM184A, KIR3DL1, and KCNK4, has illustrated its great potential in predicting the response of anti-PD-1 treatment among NSCLC patients, with support by its biological association with immune-related signaling pathways, mutation load, TMB and neoantigen burden. It is of practical significance to explore the biomarkers of ICI treatment from the translational and transcription levels. These ICI-benefits related proteomic gene-signature in lightcyan model primarily enriched in a variety of immune-related cells, including B cells, CD4+ T cells, memory cells, NK cells, NKT cells and macrophages, etc. Immune cells in the TME are important during cancer development, and their biological functions change dynamically with tumor progression. As for specific gene among the module, KRT7 in tumors is involved in metastasis, proliferation and drug resistance, and is closely related to anti-tumor immunity (20). TMEM184A is closely related to heparin-binding signaling pathway and tumor necrosis factor-related pathway (21). ANKRD30A is related to transcription factors and can promote tumor growth and proliferation (22). For these genes, subsequent studies should further explore their functions, which will contribute to an in-depth understanding of the mechanism and signaling pathway underlying the durable response of PD-1-positive tumor. Of note, the sample size was still small in the present study, which also limited further subgroup analyses, such as the comparison of different anti-PD-1/PD-L1 inhibitors. More studies are needed to focus on biomarkers that predict the efficacy of anti-PD-1/PD-L1 therapy and explore their mechanisms. More therapeutic strategies will be developed for the combination therapy of NSCLC with the investigation of immune-related targets.
Conclusions
By combing the multi-omics data from two clinical cohorts, our proteomic gene signature-based prediction model based on machine learning algorithms could estimate the prognosis of patients treated with anti-PD-1/PD-L1 monotherapy in NSCLC. We demonstrated that immunotherapy efficacy-based proteomic gene panel could improve prediction accuracy and our results provided a rationale for using proteomic gene to develop ICI-specific proteomic gene signatures in predicting patients suitable for immunotherapy.
Acknowledgments
Funding: This study was funded by
Footnote
Reporting Checklist: The authors have completed the TRIPOD and REMARK reporting checklists. Available at https://tlcr.amegroups.com/article/view/10.21037/tlcr-23-713/rc
Data Sharing Statement: Available at https://tlcr.amegroups.com/article/view/10.21037/tlcr-23-713/dss
Peer Review File: Available at https://tlcr.amegroups.com/article/view/10.21037/tlcr-23-713/prf
Conflicts of Interest: All authors have completed the ICMJE uniform disclosure form (available at https://tlcr.amegroups.com/article/view/10.21037/tlcr-23-713/coif). C.Z. serves as an Editor-in-Chief of Translational Lung Cancer Research. He also reports that this study was funded by Shanghai Key Clinical Specialty Construction Project—Respiratory Medicine (201912-0552). The other authors have no conflicts of interest to declare.
Ethical Statement: The authors are accountable for all aspects of the work in ensuring that questions related to the accuracy or integrity of any part of the work are appropriately investigated and resolved. This work was carried out in accordance with Declaration of Helsinki (as revised in 2013), and was approved by ethics committee of Shanghai Pulmonary Hospital (No. L20-337Y). Written consents were obtained from all enrolled participants.
Open Access Statement: This is an Open Access article distributed in accordance with the Creative Commons Attribution-NonCommercial-NoDerivs 4.0 International License (CC BY-NC-ND 4.0), which permits the non-commercial replication and distribution of the article with the strict proviso that no changes or edits are made and the original work is properly cited (including links to both the formal publication through the relevant DOI and the license). See: https://creativecommons.org/licenses/by-nc-nd/4.0/.
References
- Sung H, Ferlay J, Siegel RL, et al. Global Cancer Statistics 2020: GLOBOCAN Estimates of Incidence and Mortality Worldwide for 36 Cancers in 185 Countries. CA Cancer J Clin 2021;71:209-49. [Crossref] [PubMed]
- Soerjomataram I, Bray F. Planning for tomorrow: global cancer incidence and the role of prevention 2020-2070. Nat Rev Clin Oncol 2021;18:663-72. [Crossref] [PubMed]
- Siegel RL, Miller KD, Jemal A. Cancer Statistics, 2017. CA Cancer J Clin 2017;67:7-30. [Crossref] [PubMed]
- Gettinger S, Horn L, Jackman D, et al. Five-Year Follow-Up of Nivolumab in Previously Treated Advanced Non-Small-Cell Lung Cancer: Results From the CA209-003 Study. J Clin Oncol 2018;36:1675-84. [Crossref] [PubMed]
- Wu N, Ge W, Quek RG, et al. Trends in real-world biomarker testing and overall survival in US patients with advanced non-small-cell lung cancer. Future Oncol 2022;18:4385-97. [Crossref] [PubMed]
- Kim H, Kwon HJ, Kim ES, et al. Comparison of the Predictive Power of a Combination versus Individual Biomarker Testing in Non-Small Cell Lung Cancer Patients Treated with Immune Checkpoint Inhibitors. Cancer Res Treat 2022;54:424-33. [Crossref] [PubMed]
- Cai W, Zhou D, Wu W, et al. MHC class II restricted neoantigen peptides predicted by clonal mutation analysis in lung adenocarcinoma patients: implications on prognostic immunological biomarker and vaccine design. BMC Genomics 2018;19:582. [Crossref] [PubMed]
- Ayers KL, Ma M, Debussche G, et al. A composite biomarker of neutrophil-lymphocyte ratio and hemoglobin level correlates with clinical response to PD-1 and PD-L1 inhibitors in advanced non-small cell lung cancers. BMC Cancer 2021;21:441. [Crossref] [PubMed]
- Wiesweg M, Mairinger F, Reis H, et al. Machine learning reveals a PD-L1-independent prediction of response to immunotherapy of non-small cell lung cancer by gene expression context. Eur J Cancer 2020;140:76-85. [Crossref] [PubMed]
- Jung H, Kim HS, Kim JY, et al. DNA methylation loss promotes immune evasion of tumours with high mutation and copy number load. Nat Commun 2019;10:4278. [Crossref] [PubMed]
- Hellmann MD, Nathanson T, Rizvi H, et al. Genomic Features of Response to Combination Immunotherapy in Patients with Advanced Non-Small-Cell Lung Cancer. Cancer Cell 2018;33:843-852.e4. [Crossref] [PubMed]
- Rizvi H, Sanchez-Vega F, La K, et al. Molecular Determinants of Response to Anti-Programmed Cell Death (PD)-1 and Anti-Programmed Death-Ligand 1 (PD-L1) Blockade in Patients With Non-Small-Cell Lung Cancer Profiled With Targeted Next-Generation Sequencing. J Clin Oncol 2018;36:633-41. [Crossref] [PubMed]
- Eisenhauer EA, Therasse P, Bogaerts J, et al. New response evaluation criteria in solid tumours: revised RECIST guideline (version 1.1). Eur J Cancer 2009;45:228-47. [Crossref] [PubMed]
- Niu W, Jiang L. A seven-gene prognostic model related to immune checkpoint PD-1 revealing overall survival in patients with lung adenocarcinoma. Math Biosci Eng 2021;18:6136-54. [Crossref] [PubMed]
- Liu CJ, Hu FF, Xie GY, et al. GSCA: an integrated platform for gene set cancer analysis at genomic, pharmacogenomic and immunogenomic levels. Brief Bioinform 2023;24:bbac558. [Crossref] [PubMed]
- Nagy Á, Győrffy B. muTarget: A platform linking gene expression changes and mutation status in solid tumors. Int J Cancer 2021;148:502-11. [Crossref] [PubMed]
- Maron BA, Wang RS, Shevtsov S, et al. Individualized interactomes for network-based precision medicine in hypertrophic cardiomyopathy with implications for other clinical pathophenotypes. Nat Commun 2021;12:873. [Crossref] [PubMed]
- Dong A, Zhao Y, Li Z, et al. PD-L1 versus tumor mutation burden: Which is the better immunotherapy biomarker in advanced non-small cell lung cancer? J Gene Med 2021;23:e3294. [Crossref] [PubMed]
- Gibney GT, Weiner LM, Atkins MB. Predictive biomarkers for checkpoint inhibitor-based immunotherapy. Lancet Oncol 2016;17:e542-51. [Crossref] [PubMed]
- Dum D, Menz A, Völkel C, et al. Cytokeratin 7 and cytokeratin 20 expression in cancer: A tissue microarray study on 15,424 cancers. Exp Mol Pathol 2022;126:104762. [Crossref] [PubMed]
- Farwell SL, Kanyi D, Hamel M, et al. Heparin Decreases in Tumor Necrosis Factor α (TNFα)-induced Endothelial Stress Responses Require Transmembrane Protein 184A and Induction of Dual Specificity Phosphatase 1. J Biol Chem 2016;291:5342-54. [Crossref] [PubMed]
- Varga Z, Theurillat JP, Filonenko V, et al. Preferential nuclear and cytoplasmic NY-BR-1 protein expression in primary breast cancer and lymph node metastases. Clin Cancer Res 2006;12:2745-51. [Crossref] [PubMed]