Predicting disease recurrence in limited disease small cell lung cancer using cell-free DNA-based mutation and fragmentome analyses
Highlight box
Key findings
• This study focuses on predicting disease recurrence in limited disease small cell lung cancer (LD-SCLC) using cell-free DNA (cfDNA) analyses. The researchers employed circulating-tumor DNA (ctDNA)-based genomic and fragmentome analyses to assess the risk of recurrence in patients treated with definitive concurrent chemoradiotherapy (CCRT).
What is known and what is new?
• It’s known that LD-SCLC, even when treated effectively initially, often experiences recurrence.
• The new aspect of this study is the utilization of ctDNA analyses, involving both genomic sequencing and fragmentome analysis, to predict recurrence. The study distinguishes between patients with persistent responses (PeR) and those without (non-PeR) based on recurrence-free survival (RFS).
What is the implication, and what should change now?
• The study found that mutations in TP53 and RB1 genes, as well as high fragmentation ratios in ctDNA, correlated with early disease recurrence. This implies that ctDNA analyses can be a potent tool in predicting early treatment failure, which is critical for making timely clinical decisions for high-risk patients.
• The study suggests the need for integrating ctDNA-based monitoring in clinical practice, specifically in the context of LD-SCLC. This approach may help in identifying patients at high risk of relapse early in the treatment process, thereby facilitating more tailored and potentially more effective treatment strategies. Overall, the study contributes to the growing body of evidence supporting the utility of ctDNA analyses in cancer prognosis and treatment monitoring.
Introduction
Small cell lung cancer (SCLC) comprises 15% of newly diagnosed lung cancers and is characterized by the early development of systemic metastases (1). Approximately one-third of patients with SCLC are initially diagnosed with limited disease (LD), which is confined to the ipsilateral chest and can be encompassed within a radiation field for definitive concurrent chemoradiotherapy (CCRT) (2). Although LD-SCLC is considered a curative disease and shows extremely high sensitivity to definitive CCRT (3), responses are transient, showing a median overall survival (OS) of approximately 2 years because of early recurrence (4).
Owing to the deleterious outcomes of LD-SCLC when it relapses in a systemic manner, investigations have been conducted to detect early recurrence in advance of radiological progression using non-invasive approaches. Representatively, the somatic variant using a frequently mutated gene in SCLC, TP53 were detected in 35.7% of circulating tumor DNA (ctDNA) samples from patients with early-stage SCLC (5). Studying longitudinally collected blood samples from patients with SCLC patients, the panel composed with a panel of 14 frequently altered genes in SCLC detect disease-associated mutation in 85% of population. Using this panel, disease recurrence was identified before radiological tumor progression, based on increased mutational abundances in the follow-up samples (6). Moreover, the ctDNA levels (measured as genomic equivalents) were prognostic in that the subset of patients with low ctDNA abundances showed high 1-year survival probabilities (6). With advances in sequencing techniques, ctDNA-based monitoring methods are now utilized in clinics to identify populations with a high risk of relapse and to support clinical decisions regarding the application of additional treatments in advance to confirm the radiological responses (7,8).
To enhance the sensitivity and utility of ctDNA-based monitoring methods, the clinical implications of findings with off-the-shelf large panels or individually bespoke panels are being researched for different types of early-stage cancer (9,10). In addition, by referring to differences in fragment lengths between normal and tumor cell-free DNA (cfDNA) (11), ctDNA fragmentome-based analysis assisted in early detection of cancer recurrence and has demonstrated independent prognostic value (12).
Considering the high potential of hematogenous systemic spread of SCLC, it can be anticipated that detecting and evaluating the abundance of somatic tumors based on ctDNA may be helpful for predicting disease recurrence in LD-SCLC (13). Here, we evaluated the predictive value of ctDNA-based large-panel sequencing and fragmentome analyses using longitudinal pre-treatment and on-treatment samples from patients with LD-SCLC. We present this article in accordance with the STARD reporting checklist (available at https://tlcr.amegroups.com/article/view/10.21037/tlcr-23-479/rc).
Methods
Study design and population
Sequential blood samples were prospectively collected from histologically confirmed treatment-naive patients with LD-SCLC who received definitive CCRT with curative intent. All patients received two cycles of chemotherapy followed by additional two cycles of chemotherapy concurrently with radiotherapy. Samples were longitudinally collected at two different timepoints (Figure 1A). The first blood sample was collected before treatment. The second blood sample (i.e., the “on-treatment” sample) was collected at the timepoint of the first radiological evaluation, which was after two cycles of chemotherapy. All patients were monitored for radiological disease relapse after the completion of CCRT. All patients signed informed consent to provide samples, and the study was approved by the Samsung Medical Center Institutional Review Board (approval number 2016-08-052). The study was conducted in accordance with the Declaration of Helsinki (as revised in 2013).
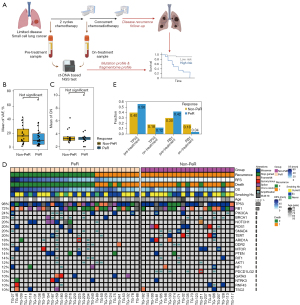
Blood sample collection and cfDNA extraction
Whole blood samples (8–10 mL) were collected in ethylenediaminetetraacetic acid (EDTA) tubes, mixed with Ficoll solution, and centrifuged at 1,500 ×g for 15 minutes. Plasma was then separated by centrifugation at 16,000 ×g for 10 minutes to remove cellular debris, after which 1 mL aliquots were prepared in Eppendorf tubes and stored at −80 ℃ before cfDNA extraction. This protocol was performed within 20 minutes of blood collection to prevent cfDNA degradation and the release of genomic DNA from the blood cells. cfDNA was isolated from 2 to 4 mL plasma using a cfKaptureTM Kit (MagBio Genomics, MD, USA), according to the manufacturer’s instructions, and quantified using a 2200 TapeStation System (Agilent Technologies, CA, USA). Peripheral blood mononuclear cells (PBMCs) were separated, and genomic DNA was isolated from the PBMCs using a QIAamp DNA Mini Kit (Qiagen, Germany).
Targeted deep sequencing and bioinformatics analysis
For the DNA sequencing part of the study, we utilized around 20 ng of cfDNA and 100 ng of DNA from white blood cells for each participant to prepare the sequencing libraries, using the IMBdx next-generation sequencing (NGS) DNA Library Preparation Kit. Target enrichment was carried out using the AlphaLiquid® 100 target capture panel, which focuses on 106 genes related to cancer, at IMBdx, Inc. in Seoul (Tables S1,S2). Sequencing was performed on the Illumina NextSeq 550 system (Illumina, Inc., San Diego, CA, USA) in a paired-end mode with 150 bp reads. We aligned the sequencing data to the human reference genome using the Burrows-Wheeler Aligner’s “mem” algorithm (14). Reads matching the targeted regions of the AlphaLiquid® 100 panel were processed and collapsed using Genecore (15). Variant identification was done using VarDict, followed by IMBdx’s proprietary filters (16). The final list of variants was annotated for functional effects and database tagging using tools like SnpEff (17), SnpSift (18), and VEP (19).
Fragmentome analysis
For fragmentome analysis, read pairs with a mapping quality score of ≥30 were extracted using Samtools. For each patient, the fragment lengths were calculated as the distance between both ends of read pairs spanning patient-specific somatic mutations. Where applicable, read pairs were subdivided into those containing the mutated allele or the wild-type allele. To quantify the potency of the cancer signals, the length distributions were divided according to three metrics: the proportion of fragments in the P1 (100–155 bp) range, the proportion in P2 (160–180 bp), and the ratio between both proportions (P1/P2). Tumor-driven fragments were expected to be more abundant in P1 than in P2 (20,21).
Clinical sub-classification and statistical analysis
The patients were classified into two groups based on the duration of recurrence-free survival (RFS). RFS was defined as the time from the date of initial treatment to the date of radiologically confirmed disease progression (for those with progressive disease) or the last follow-up date (for those without disease progression). Patients who demonstrated an RFS of ≥12 months were assigned to the persistent-response (PeR) group, and the remaining patients were assigned to the non-PeR group. OS was defined as the time from the date of initial treatment to the last follow-up date or the date of death. Cox proportional-hazard models were used to evaluate the hazard ratio (HR) and statistical significance between the groups. Kaplan-Meier curves were used to portray survival differences. Receiver operating characteristic (ROC) curves were drawn to evaluate the predictive values of variables using multiple ROC (https://github.com/cardiomoon/multipleROC), and optimal cut-off values were calculated to predict the non-PeR group. Statistical analysis was conducted using R, version 4.03 (http://www.r-project.org). P values <0.05 were considered statistically significant.
Results
Baseline demographics and sample description
Beginning in February 2017, 100 samples were acquired from 50 patients with LD-SCLC at two different time points (pre-treatment and on-treatment) and were used for analysis (Figure 1A, Table 1). All patients received two cycles of etoposide and carboplatin (EC) followed by two additional cycles of EC concurrently with radiotherapy. The median time interval between sample acquisition was 47 days (range, 31–118 days). The median age of the study population was 68 years (range, 52–83 years). Among the study population, 88% of the patients were either current or past smokers and the rest remaining 12% of patients were non-smokers. The median follow-up duration was 20.1 months [95% confidence interval (CI): 13.9–24.7]. In addition, 29 patients (58.0%) were assigned to the PeR group and 21 patients (42.0%) were assigned to the non-PeR group, with median RFS rates of 15.1 months (95% CI: 12.7–22.6) and 9.1 months (95% CI: 7.6–9.4), respectively.
Table 1
Variables | Patients (n=50) |
---|---|
Age (years) | |
Median [range] | 68 [52–83] |
Sex, n (%) | |
Male | 41 (82.0) |
Female | 9 (18.0) |
Initial stage at diagnosis, n (%) | |
Limited disease | 50 (100.0) |
Smoking history, n (%) | |
Current/previous smoker | 44 (88.0) |
Never smoker | 6 (12.0) |
ECOG PS, n (%) | |
0 | 4 (8.0) |
1 | 43 (86.0) |
2 | 3 (6.0) |
Previous treatment, n (%) | |
Etoposide & platinum with concurrent chemoradiotherapy | 42 (84.0) |
Clinical trials (with maintenance immunotherapy to standard treatment) | 8 (16.0) |
ECOG PS, Eastern Cooperative Oncology Group performance score.
ctDNA-detection rates and overview of sequencing results
The median amount of DNA extracted from pre-treatment samples was 14.83 ng/mL (range, 6.09–194.44 ng/mL) and that from on-treatment sample was 19.96 ng/mL (range, 5.67–145.44 ng/mL). ctDNA was detected in all pre-treatment samples. With the on-treatment sample, ctDNA was detected in 26 patients (52.0%), including 16 patients (55.2%) in the PeR group and 10 patients (47.6%) in the non-PeR group. Among the samples with detectable ctDNA, the median cfDNA levels (ng/mL) were 4.93 (range, 2.53–64.81) and 6.23 (range, 2.03–47.09) in pre-treatment samples from the PeR and non-PeR groups, respectively, and 4.00 (range, 2.53–64.81) and 7.66 (range, 3.21–28.88) in post-treatment samples from the PeR and non-PeR groups, respectively.
In terms of the mutation landscape of the pre-treatment samples, the mean variant-allele frequencies (VAFs) of single-nucleotide variants (SNVs) and insertions and deletions (INDELs) were 9.76% (range, 0.97–69.58%) and 16.31% (range, 1.10–43.94%) in the PeR and non-PeR groups, respectively (Figure 1B). The most frequently altered genes were TP53 (98.0%, n=49), followed by RB1 (72.0%, n=36) and NOTCH1 (18.0%, n=9). Copy-number alterations (CNAs) were detected in 31 patients (62.0%). The mean copy numbers were 3.31 (range, 2.00–10.00) and 3.24 (range, 2.61–6.47) in the PeR and non-PeR groups, respectively (Figure 1C). Amplifications were frequently detected in SMAD4 (20.0%, n=10), PIK3CA (18.0%, n=9), and BRCA1 (18.0%, n=9).
A detailed depiction of the mutation landscape is shown in Figure 1D. The difference in fraction of TP53 and RB1 mutations between pre- and post-treatment were higher in the PeR samples than in the non-PeR samples (Figure 1E).
Fragmentome landscape
The fragment sizes were evaluated using pre-treatment (n=50) and on-treatment (n=26) samples with detectable ctDNA. The distributions of fragments between 0 to 300 bp were analyzed with fragments harboring either mutant alleles or wild-type alleles (Figure 2A). The median size of the fragments with mutant alleles was 149 bp, whereas that of fragments with wild-type alleles was 169 bp. A statistically significant difference was found in the fragment-size distributions of both groups (P=0.019, as determined by performing a two-sample Kolmogorov-Smirnov test). No significant differences were found between the P1 proportion (P=0.452), P2 proportion (P=0.551), and fragmentation ratio (P1/P2) (P=0.587) in the pre-treatment samples, among patients in the non-PeR and PeR groups (Figure 2B). In contrast, the on-treatments sample showed higher P1 proportions (P=0.004) and fragmentation ratios (P<0.001) in the non-PeR group than in the PeR group. However, no difference was found in the P2 proportions between the groups (P=0.060).
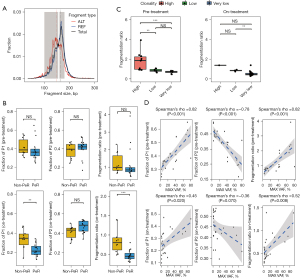
To evaluate correlations between ctDNA VAFs and clonality, we used the maximum VAF value from each patient to calculate the clonality and categorized the patients as having very low clonality, low clonality, or high clonality. With the pre-treatment samples, the distribution of the fragmentation ratio was higher in patients with high clonality than in patients with low (P=0.004) or very low clonality (P<0.001) (Figure 2C, left). A similar trend was observed in the on-treatment samples, although statistical power was not achieved because of the limited number of samples in the high-clonality group (Figure 2C, right). Regarding the associations between fragmentation metrics and the maximum VAF found with pre-treatment samples, Spearman’s rho values of 0.82, −0.78, and 0.82 were observed for the P1 proportion, P2 proportion, and fragmentation ratio, respectively (Figure 2D). The on-treatment samples showed Spearman’s rho values of 0.45, −0.36, and 0.52 for the P1 proportion, P2 proportion, and fragmentation ratio, respectively.
Changes in the fragment distributions in the pre-treatment and on-treatment samples (n=26) were analyzed in a paired manner. After treatment, significant decreases in the P1 proportion (P<0.001) and fragment ratio (P<0.001) and a significant increase in the P2 proportion (P=0.009) were observed in the PeR group (n=16) (Figure S1A-S1C). Once again, the decrease in the P1 proportion of the PeR group showed a more significant fold-change than the P1 proportion of non-PeR group (P=0.036) which has not observed in P2 or fragment ratio (Figure S1D-S1F).
Analysis using genes of interest and determining their predictive values for survival
We evaluated the predictive values of the TP53 and RB1 genes, which were most frequently mutated in SCLC (Figure 3A). The VAFs of both TP53 (P<0.001) and RB1 (P<0.001) significantly decreased after treatment (Figure 3B). Analyzing the TP53 mutation status in the on-treatment samples revealed significant difference in the median RFS between patients with TP53 mutations and wild-type TP53 (11.2 months vs. not reached, respectively, P=0.05) (Figure 3C). Patients with RB1 mutations in their on-treatment samples also showed a significantly shorter median RFS than patients with wild-type RB1 (8.9 months vs. not reached, P=0.0014) (Figure 3D). Exploratory analysis showed that patients with detectable RB1 mutations in their on-treatment samples had significantly shorter OS time (P=0.0018), whereas TP53 mutations showed shorter but not significant OS difference (P=0.083) (Figure S2).
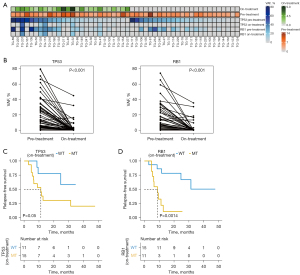
Fragmentome analysis and correlations with survival
ROC analysis was conducted using the on-treatment samples with detectable mutations (n=26) to assess the utility of fragmentome analysis in distinguishing between patients in the PeR and non-PeR groups. Using the pre-treatment samples, the area under the curve (AUC) values for the P1 proportion, P2 proportion, and fragmentation ratio were 0.569, 0.594, and 0.575, respectively (Figure S3A). The on-treatment sample showed comparably higher AUC values with respect to the P1 proportion (0.850), P2 proportion (0.725), and fragmentation ratio (0.900) (Figure 4A).
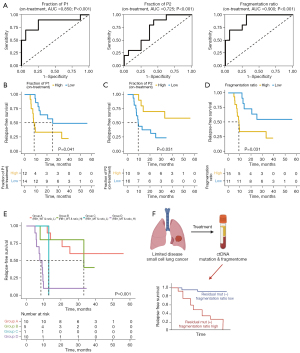
Using the on-treatment samples, survival rates were compared using an optimal cut-off based on the ROC analysis. Analysis using optimal cut-off values for P1 (P=0.041) and P2 (P=0.031) demonstrated statistically significant differences in the RFS (Figure 4B,4C). Patients with a low fragmentation ratio (n=11) showed a longer RFS than patients with a high fragmentation ratio (n=15) (25.2 vs. 7.9 months, P=0.031) (Figure 4D). Combining the fragmentation ratio status with the RB1 mutation status revealed an enhanced survival benefit in the ctDNA-based low-risk group with wild-type RB1 and a low fragmentation ratio (n=10), as the median RFS was not achieved during a median follow-up duration of 20.1 months. This group comprised ten patients with a PeR (100%) and 0 patients with a non-PeR (0%). In contrast, the shortest median RFS (5.0 months) was observed in the ctDNA-based high-risk group with mutant RB1 and a high fragmentation ratio (n=10). This group comprised one patient with a PeR (10.0%) and nine patients with a non-PeR (90.0%). The HR between the ctDNA-based high- and low-risk groups was 7.55 (95% CI: 2.14–26.6, P=0.002) (Figure 4E).
Using pre-treatment samples, the low- and high-risk groups showed no significant differences in the median RFS but not OS, based on the optimal cut-off, with respect to the P1 proportion (P=0.86), P2 proportion (P=0.43), and fragmentation ratio (P=0.71) (Figure S3B-S3G).
Discussion
In this study, we determined the value of analyzing ctDNA samples (collected before and during treatment) for predicting early disease recurrence in patients with LD-SCLC. We analyzed blood-derived cfDNA samples using an off-the-shelf NGS panel for a relatively large set of cancer-related gene (n=106), owing to the very limited access to longitudinal tumor tissue samples in patients with SCLC. Although our study population consisted of patients with LD-SCLC, ctDNA was detectable in all pre-treatment samples and in 52.0% of the on-treatment samples, showing an overall detection rate of 76.0%. Our detection sensitivity was similar to that of a previous report (85%), which was based on assaying for mutations in 14 genes, but included a high proportion of samples from patients with extensive disease (59%) (6). Based on the assumption that the clearance of pre-identified mutations during the treatment period might be related to the degree of treatment efficacy, we evaluated long-term clinical responses based on mutations in two major mutations, TP53 and RB1, which were detected in 98% and 72% of the patients, respectively. Although our on-treatment samples were collected only after two cycles of chemotherapy, the mutation-detection rates of TP53 and RB1 had decreased to 30% and 22%, respectively. Surprisingly, early disease recurrence was observed in patients with persistent mutations identified in their samples even before completing the entire course of CCRT treatment. As mounting data support the correlation of minimal residual disease (MRD) identified by somatic alterations after curative treatment and disease relapse (22), our results were somewhat unique in that they showed a correlation between MRD during the early treatment phase and the time to recurrence.
Because some types of tumors only harbor a limited number of hotspot mutations, detecting residual disease or evaluating the depth of clinical responses using a pre-designed customized sequencing panel is challenging. In addition, it is difficult to construct large gene sets that can be used as a universal definition for MRD. Bypassing the issues associated with individual gene-based MRD concepts, emerging approaches using the ctDNA-fragment length have been investigated for enhancing the ctDNA-detection rate and evaluating the MRD. Similar to a previous observation that the fragment lengths of ctDNAs harboring mutant alleles (132–145 bp) were shorter than those harboring wild-type alleles (165 bp) (11,20), we found that the median length of ctDNA fragments with genomic alterations was 149 bp and that of ctDNA fragments harboring wild-type alleles was 169 bp (Figure 2A). A clear difference between the P1 proportion (which most likely represents ctDNA-derived fragments) was observed based on long-term clinical outcomes in on-treatment samples, not in pre-treatment samples, which also supports the predictive value of fragmentome analysis. The finding was more prominent when the P1:P2 ratio was high, which corresponds to a high proportion of fragments with mutant alleles and a low proportion of fragments with wild-type allele (Figure 4A). In addition, our binary analysis using the optimal ROC cut-offs for P1, P2, and the P1:P2 ratio demonstrated significant differences in the RFS interval (P=0.041, 0.031, 0.031, respectively, Figure 4B-4D). Based on the genomic characteristics of SCLC, combining the RB1 mutation status with the P1:P2 fragment ratio can assist in identifying PeR patients and early treatment failure (P=0.002) (Figure 4E,4F).
In our study, we used a customized NGS panel for 106 genes of similar size that are used for ctDNA-based sequencing with other commercially available products. As an exploratory approach, we tried to conduct survival analyses based on the number mutations identified from the on-treatment samples, but the data were limited because only 8 out of 50 patients harbored ≥4 mutations, and only two patients had ≥5 mutations. Although a sufficient number of mutations was observed in the pre-treatment samples, survival analyses based on cut-offs of ≥4 mutations (n=33) or ≥5 mutations (n=22) showed no survival differences for the low-mutation group (data not shown). This finding suggests a limitation in defining MRD using a somatic mutation-based approach, as it could under detect the MRD because of the composition of the genes in a given panel or the frequencies of different somatic mutations in different types of cancer (Table S3). Nevertheless, this finding supports and suggests the opportunity for evaluating the presence and abundance of ctDNA, considering that the approach could be more sensitive than evaluating specific somatic alterations.
Regarding ctDNA-based analysis, determining how to best handle the clinical outcomes of patients with no detectable ctDNA was challenging. Our results only showed RFS differences for patients who had sufficient ctDNA for analysis in their on-treatment samples (n=26). We hypothesize that the remaining patients (n=24) with undetectable ctDNA in their on-treatment samples could be considered as patients who did not shed DNA from their tumors, due to a prominent clinical response. However, comparing the survival of that group with that of patients with detectable ctDNA revealed no difference in the RFS (P=0.51) (Figure S4A). In contrast, after dividing the ctDNA-positive patients based on their P1:P2 ratios, our analysis showed that the RFS of the low-ratio group was similar to that of the ctDNA-negative group, whereas patients in the high-ratio group showed a significantly shorter RFS (P=0.033) (Figure S4B). This observation indicates that both the abundance of ctDNA fragments and the presence of ctDNA identified with the sequencing panel were useful for relapse prediction.
Since we selected a single time point during treatment for ctDNA analysis, our results are limited in terms of predicting the concordance with radiological changes. In addition, analyzing ctDNA acquired at the time of treatment completion could be more effective than our approach of studying mid-treatment samples; thus, further investigation is needed. Lastly, due to an unidentified confounding effect between mutation analysis from genetic ctDNA and the burden of tumor DNA fragment, there could be a possibility that benefit of combining the two modalities might have little benefit than our assumption. However, to our knowledge, this study represents the one of early research outcome evaluating disease recurrence based on ctDNA analysis for patients with LD-SCLC. In addition, this study adds value to explore the predictive value of fragmentome analysis in terms of disease recurrence with solid tumors which has limited research outcome. The number of analytical tools for fragmentome analysis is increasing. Thus, the utility of fragmentome analysis has expanded from cancer screening to identifying the cancer type, based on distinct transcriptional characteristics, fragment coverage, and transcription factor-binding sites, using cell-free, whole-genome sequencing (12). Considering the aggressive clinical features of LD-SCLC, with disease recurrence eventually occurring in up to 70% of such patients, our approach could support the development of further treatment strategies. For example, our analysis could highlight the necessity for additional consolidation therapy, including prophylactic cranial irradiation or immunotherapy, or serve as a basis for developing and adopting similar approaches for other types of solid tumors (8,23).
Conclusions
In conclusion, the results of our study demonstrated the feasibility and preliminary outcomes of predicting disease recurrence using somatic variations and ctDNA fragments captured from a customized ctDNA-based panel for patients with LD-SCLC. Further investigation using samples from different longitudinal timepoints and correlations with radiological features is ongoing to expand this concept to both LD-SCLC and extended-stage SCLC.
Acknowledgments
The authors acknowledge the Korea Research Environment Open Network (KREONET) service and the usage of the Global Science Experimental Data Hub Center (GSDC) provided by Korea Institute of Science and Technology Information (KISTI). Sequencing analysis based on the AlphaLiquid® 100 was supported by IMBdx.
Funding: This work was supported by
Footnote
Reporting Checklist: The authors have completed the STARD reporting checklist. Available at https://tlcr.amegroups.com/article/view/10.21037/tlcr-23-479/rc
Data Sharing Statement: Available at https://tlcr.amegroups.com/article/view/10.21037/tlcr-23-479/dss
Peer Review File: Available at https://tlcr.amegroups.com/article/view/10.21037/tlcr-23-479/prf
Conflicts of Interest: All authors have completed the ICMJE uniform disclosure form (available at https://tlcr.amegroups.com/article/view/10.21037/tlcr-23-479/coif). S.H.L. serves as an unpaid editorial board member of Translational Lung Cancer Research from October 2023 to September 2025. J.K.K., H.P.K., S.Y.K. and T.Y.K. report the employment in IMBdx, Inc. The other authors have no conflicts of interest to declare.
Ethical Statement: The authors are accountable for all aspects of the work in ensuring that questions related to the accuracy or integrity of any part of the work are appropriately investigated and resolved. The study was conducted in accordance with the Declaration of Helsinki (as revised in 2013). All patients signed informed consent to provide samples, and the study was approved by the Samsung Medical Center Institutional Review Board (approval number 2016-08-052).
Open Access Statement: This is an Open Access article distributed in accordance with the Creative Commons Attribution-NonCommercial-NoDerivs 4.0 International License (CC BY-NC-ND 4.0), which permits the non-commercial replication and distribution of the article with the strict proviso that no changes or edits are made and the original work is properly cited (including links to both the formal publication through the relevant DOI and the license). See: https://creativecommons.org/licenses/by-nc-nd/4.0/.
References
- Siegel RL, Miller KD, Fuchs HE, et al. Cancer Statistics, 2021. CA Cancer J Clin 2021;71:7-33. [Crossref] [PubMed]
- Dingemans AC, Früh M, Ardizzoni A, et al. Small-cell lung cancer: ESMO Clinical Practice Guidelines for diagnosis, treatment and follow-up☆. Ann Oncol 2021;32:839-53. [Crossref] [PubMed]
- Faivre-Finn C, Snee M, Ashcroft L, et al. Concurrent once-daily versus twice-daily chemoradiotherapy in patients with limited-stage small-cell lung cancer (CONVERT): an open-label, phase 3, randomised, superiority trial. Lancet Oncol 2017;18:1116-25. [Crossref] [PubMed]
- Amarasena IU, Chatterjee S, Walters JA, et al. Platinum versus non-platinum chemotherapy regimens for small cell lung cancer. Cochrane Database Syst Rev 2015;2015:CD006849. [Crossref] [PubMed]
- Fernandez-Cuesta L, Perdomo S, Avogbe PH, et al. Identification of Circulating Tumor DNA for the Early Detection of Small-cell Lung Cancer. EBioMedicine 2016;10:117-23. [Crossref] [PubMed]
- Almodovar K, Iams WT, Meador CB, et al. Longitudinal Cell-Free DNA Analysis in Patients with Small Cell Lung Cancer Reveals Dynamic Insights into Treatment Efficacy and Disease Relapse. J Thorac Oncol 2018;13:112-23. [Crossref] [PubMed]
- ClinicalTrials.gov. Phase III Study to Determine the Efficacy of Durvalumab in Combination with Chemotherapy in Completely Resected Stage II-III Non-small Cell Lung Cancer (NSCLC) (MERMAID-1). Available online: https://clinicaltrials.gov/ct2/show/NCT04385368. (accessed 1 April 2022)
- ClinicalTrials.gov. Phase III Study to Determine Efficacy of Durvalumab in Stage II-III Non-small Cell Lung Cancer (NSCLC) After Curative Intent Therapy. (MERMAID-2). Available online: https://clinicaltrials.gov/ct2/show/NCT04642469. (accessed 1 April 2022)
- Lau E, McCoy P, Reeves F, et al. Detection of ctDNA in plasma of patients with clinically localised prostate cancer is associated with rapid disease progression. Genome Med 2020;12:72. [Crossref] [PubMed]
- Chaudhuri AA, Chabon JJ, Lovejoy AF, et al. Early Detection of Molecular Residual Disease in Localized Lung Cancer by Circulating Tumor DNA Profiling. Cancer Discov 2017;7:1394-403. [Crossref] [PubMed]
- Underhill HR, Kitzman JO, Hellwig S, et al. Fragment Length of Circulating Tumor DNA. PLoS Genet 2016;12:e1006162. [Crossref] [PubMed]
- Mathios D, Johansen JS, Cristiano S, et al. Detection and characterization of lung cancer using cell-free DNA fragmentomes. Nat Commun 2021;12:5060. [Crossref] [PubMed]
- Sivapalan L, Iams WT, Belcaid Z, et al. Dynamics of Sequence and Structural Cell-Free DNA Landscapes in Small-Cell Lung Cancer. Clin Cancer Res 2023;29:2310-23. [Crossref] [PubMed]
- Li H, Durbin R. Fast and accurate short read alignment with Burrows-Wheeler transform. Bioinformatics 2009;25:1754-60. [Crossref] [PubMed]
- Chen S, Zhou Y, Chen Y, et al. Gencore: an efficient tool to generate consensus reads for error suppressing and duplicate removing of NGS data. BMC Bioinformatics 2019;20:606. [Crossref] [PubMed]
- Lai Z, Markovets A, Ahdesmaki M, et al. VarDict: a novel and versatile variant caller for next-generation sequencing in cancer research. Nucleic Acids Res 2016;44:e108. [Crossref] [PubMed]
- Cingolani P, Platts A. A program for annotating and predicting the effects of single nucleotide polymorphisms, SnpEff: SNPs in the genome of Drosophila melanogaster strain w1118; iso-2; iso-3. Fly (Austin) 2012;6:80-92. [Crossref] [PubMed]
- Cingolani P, Patel VM, Coon M, et al. Using Drosophila melanogaster as a Model for Genotoxic Chemical Mutational Studies with a New Program, SnpSift. Front Genet 2012;3:35. [Crossref] [PubMed]
- McLaren W, Gil L, Hunt SE, et al. The Ensembl Variant Effect Predictor. Genome Biol 2016;17:122. [Crossref] [PubMed]
- Mouliere F, Chandrananda D, Piskorz AM, et al. Enhanced detection of circulating tumor DNA by fragment size analysis. Sci Transl Med 2018;10:eaat4921. [Crossref] [PubMed]
- Cristiano S, Leal A, Phallen J, et al. Genome-wide cell-free DNA fragmentation in patients with cancer. Nature 2019;570:385-9. [Crossref] [PubMed]
- Pellini B, Chaudhuri AA. Circulating Tumor DNA Minimal Residual Disease Detection of Non-Small-Cell Lung Cancer Treated With Curative Intent. J Clin Oncol 2022;40:567-75. [Crossref] [PubMed]
- Bratman SV, Yang SYC, Iafolla MAJ, et al. Personalized circulating tumor DNA analysis as a predictive biomarker in solid tumor patients treated with pembrolizumab. Nat Cancer 2020;1:873-81. [Crossref] [PubMed]