Systemic immune index predicts tumor-infiltrating lymphocyte intensity and immunotherapy response in small cell lung cancer
Highlight box
Key findings
• The prognostic nutritional index (PNI)-based immune risk system is an independent prognostic factor for small-cell lung cancer (SCLC) patients. The PNI-high extensive-stage SCLC patients also achieved better clinical response and survival from programmed cell death 1 ligand 1 blockade therapy.
What is known and what is new?
• Tumor immune microenvironment has been well known for its key role on the tumor progression and local immunologic response to certain treatments.
• We showed a strong relationship between systemic immune index and tumor-infiltrating lymphocyte intensity, patient survival and immunotherapy efficacy.
What is the implication, and what should change now?
• This study provides a method to investigate the prognostic value of overall immune status by combining the PNI with local immune biomarkers in SCLC.
Introduction
Background
Small-cell lung cancer (SCLC) is an exceptionally lethal malignancy and the limited survival improvement by conventional therapies has prompted the urgent search for innovative strategies (1,2). Despite that immune checkpoint blockade (ICB) therapy sheds light on a breakthrough of SCLC treatment recently, the survival benefits are still modest (3-6). In addition, although immune checkpoints such as programmed cell death 1 ligand 1 (PD-L1) are extremely valuable prognostic biomarkers across different cancer types including non-small-cell lung cancer (NSCLC), few biomarkers are available in SCLC for accurate survival prediction and ICB therapy selection.
Tumor immune microenvironment (TIME), compositing several immune cell groups such as tumor-infiltrating lymphocytes (TILs), has been well known for its key role on the tumor progression and local immunologic response to certain treatments (7). However, one must be kept in mind that both local tumor immune contexture and treatment response can also be influenced by systemic immune status, i.e., the host systemic tumor immune environment (STIE) (8). STIE consists of circulating blood immune cells and molecules which can be easily assessed by routine blood tests. A prominent example is prognostic nutritional index (PNI), an indicator of nutritional status and systemic immune competence, the prognostic value of which has been revealed in various types of cancers (9-12). Moreover, various studies have illustrated the local TIME as determined by TIL status is significantly related to the PNI score (10,13).
Rationale and knowledge gap
For SCLC, it is still unknown regarding the complex interaction between STIE and TIME and whether they are valuable for predicting the long-term outcomes and immunotherapy responses of SCLC patients.
Objective
Therefore, we comprehensively evaluated the prognostic value of several systemic immune biomarkers in a relatively large SCLC cohort, determined their correlation with TILs, and further integrated systemic immune status with a local immune score system (LISS) constructed by an eXtreme Gradient Boosting (XGBoost) machine learning model to improve the prognostic accuracy in SCLC patients. In addition, another independent SCLC cohort receiving PD-L1 blockade therapy was used to test the value of PNI score in efficacy prediction and beneficiary selection. We present this article in accordance with the TRIPOD reporting checklist (available at https://tlcr.amegroups.com/article/view/10.21037/tlcr-23-696/rc).
Methods
Patients
The study included two main SCLC patient cohorts (Figure 1A), the limited-stage surgically resected SCLC (LS-SCLC) cohort (N=191) from November 2006 to April 2021, and the extensive-stage immunotherapy SCLC (ES-SCLC) cohort (N=91) who received PD-L1 blockade combined with chemotherapy [atezolizumab 1,200 mg every three weeks (q3w) or durvalumab 1,500 mg q3w] from May 2020 to April 2021 at Fudan University Shanghai Cancer Center (FUSCC). This study was approved by the Institutional Review Board of FUSCC (IRB No. 2008223-9). Informed consents were waived because it was a retrospective study. The study was conducted in accordance with the Declaration of Helsinki (as revised in 2013).
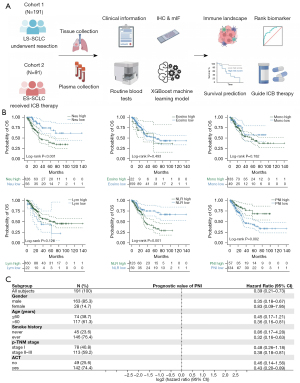
Data and tissue specimens
Data on clinicopathological variables were obtained by reviewing patient medical records specifically for this study purpose. These characteristics included age, sex, smoking history, surgery type, resection type, tumor size, pathological tumor stage, visceral pleural invasion (VPI) and lymphovascular invasion (LVI) status, treatment regimens, sodium level and lactate dehydrogenase (LDH) levels, and disease-free survival (DFS), progression-free survival (PFS) and overall survival (OS). All responses to ICBs were assessed using the Response Evaluation Criteria in Solid Tumors guidelines (14). Durable clinical benefit (DCB) was defined as the proportion of patients achieving objective response or stable disease (SD) lasting ≥6 months. The PNI was calculated as 10× serum albumin (g/dL) + 5× total lymphocytes count (/nL). The latest laboratory data within 1 month before operation or immunotherapy were used. Another inflammation-based prognostic score, the preoperative neutrophil-lymphocyte ratio (NLR) was also obtained. X-tile was used to determine the cut-off points. After independent review of all hematoxylin and eosin (HE) slides and assessment of formalin-fixed paraffin-embedded (FFPE) block quality, 129 cases in LS-SCLC cohort were constructed into tumor tissue microarray (TMA) and 16 cases were analyzed in slides. No cases in ES-SCLC cohort were evaluated with TMA.
TMA construction
SCLC TMA was constructed in the Department of Pathology, Fudan University Shanghai Cancer Center using the automated TMA Grand Master (3DHISTECH, Budapest, Hungary). The HE slides were reviewed to select the most viable tumor and uninvolved lung tissue areas. Finally, 260 cores (two or three cores per case) were punched in corresponding tumor areas with 2 mm in diameter.
Immunohistochemistry
TMAs and slides were stained for subtype-defined markers of SCLC including ASCL1 (clone 24B72D11.1, dilution 1:100, BD Biosciences, New York, USA), NEUROD1 (clone EPR17084, dilution 1:50, Abcam, Cambridge, UK), POU2F3 (clone 6D1, dilution 1:100, Santa Cruz, Texas, USA) and YAP1 (clone 63.7, dilution 1:2000, Santa Cruz) using Anti-mouse/rabbit Immunohistochemistry (IHC) Detection Kit (Cat No. PK10006, Proteintech, Chicago, USA) according to the manufacture protocol. Two independent pathologists reviewed the stained slides in a blinded fashion. The expression of each marker was assessed by histoscore (H-score, range, 0–300), which was calculated by multiplying the proportion of positive cells (0–100%) by the intensity of positive staining (no staining =0, weak staining =1, moderate staining =2, and strong staining =3). And the molecular subtype for a tumor was assigned based on the highest H-score among subtyping markers (15).
Multiplex immunofluorescence (mIF) staining
mIF analysis was performed on TMAs. TMA sections were stained using antibodies against CD4 (clone EP204, dilution 1:100, Cell Signaling Technologies, Boston, USA), CD8 (clone D8A8Y, dilution 1:200, Cell Signaling Technologies), CD68 (clone D4B9C, dilution 1:400, Cell Signaling Technologies), programmed cell death 1 (PD-1) (clone NAT105, dilution 1:100, Abcam), PD-L1 (clone E1L3N, dilution 1:100, Cell Signaling Technologies), CTLA-4 (clone CAL49, dilution 1:200, Abcam), and cytokeratins (clone C-11, dilution 1:250, Abcam). All antibodies were linked with one of the fluorophores from the Opal 7 IHC kit, (Cat No. NEL797001KT; Akoya Biosciences, MA, USA). Sections were scanned by Aperio Versa 8 tissue imaging system (Leica, Hesse, Germany). Imaging analysis was performed with a quantitative image analysis software (Halo, Indica Labs, New Mexico, USA). X-tile was used to determine the cut-off points for survival analysis.
RNA sequencing
The RNA sequencing process was performed for 67 patients with available tumor tissues as previously reported (16). Briefly, a total amount of 3 µg RNA was extracted from tumor tissues per sample using NucleoZOL (Macherey-Nagel, Dueren, Germany) and NucleoSpin RNA Set for NucleoZOL (Macherey-Nagel). The sequencing libraries were generated using the NEBNext Ultra Directional RNA Library Prep Kit for Illumina (NEB, Ipswich, USA) according to manufacturer’s instructions and then sequenced on the Illumina HiSeq X Ten platform.
XGBoost and LISS
The XGBoost machine learning algorithm was adapted to construct LISS by various tumor immune biomarkers obtained by mIF. This machine learning method can discover non-linear relationships using the first and second derivatives, as well as controlling the overfitting and overcomplexity of one predictive model by employing regularization item, and scoring the importance of each measured variable (17,18).
The SCLC samples were randomly divided into training set and calibration set (7:3). After repeating 1,000 times for model construction, top three important features were selected for the LISS establishment. The obtained model formula was as follows: LISS = (0.1437 × CD8) − (0.1353 × PD-L1/CD8) + (0.1323 × CD4). We further performed time-dependent receiver operating characteristic (ROC) curve analysis and survival analysis to validate the model performance.
Statistical analysis
All data were processed using SPSS 21.0 (SPSS, Inc., Chicago, IL, USA) and R Statistical Language (version 4.1.2). Correlations between two categorical variables were analyzed using Chi-squared test or Fisher’s exact test. Student’s t-test or Mann-Whitney test were used to compare continuous variables. The PFS and OS were investigated by log-rank test to compare differences between groups. Cox proportional hazard regressions were used to predict recurrence and death status. Variables with a P value less than 0.05 in univariate analysis were entered into multivariate survival analysis. All tests were two-tailed. Statistical significance was set at P<0.05.
Results
Prognostic value of systemic immune markers
We investigated the prognostic role of the host anti-tumor immunity, i.e., STIE in a cohort of 191 patients with surgically resected stage I–III SCLC (Figure 1B), with a median follow-up time of 59.8 months. The cut-off points for several peripheral blood immune cells as well as NLR and PNI were shown in Table S1, and patients were divided into high- and low-risk groups accordingly.
As shown in Figure 1B, higher neutrophil count and NLR indicated a significantly worse prognosis (P<0.001 and P=0.001, respectively), while compared with low-PNI score group, patients in the high-PNI score group had a better OS (P=0.002, Figure 1B) as well as DFS (P=0.027, Figure S1).
Considering potential correlations between the three variables, they were included into multivariate Cox model separately. As summarized in Table 1, PNI remained a powerful and independent factor after adjustment for clinicopathological factors [hazard ratio (HR): 0.473, 95% confidence interval (CI): 0.241–0.929, P=0.030], whereas neutrophil count and NLR did not. Further stratification analysis by Tumor Node Metastasis (TNM) stage and receipt of adjuvant chemotherapy (ACT) confirmed the prognostic value of PNI, although not always statistically significant (Figure 1C).
Table 1
Variables | Univariable | Multivariate (model 1) | Multivariate (model 2) | Multivariate (model 3) | |||||||
---|---|---|---|---|---|---|---|---|---|---|---|
HR (95% CI) | P | HR (95% CI) | P | HR (95% CI) | P | HR (95% CI) | P | ||||
Age (year) (≥60 vs. <60) |
1.165 (0.713, 1.905) |
0.542 | |||||||||
Gender (female vs. male) |
0.256 (0.093, 0.706) |
0.008 | 0.130 (0.031, 0.541) |
0.005 | 0.148 (0.033, 0.655) |
0.012 | 0.148 (0.033, 0.655) |
0.012 | |||
Smoking history (ever vs. never) |
2.840 (1.351, 5.967) |
0.006 | 1.427 (0.512, 3.976) |
0.496 | 0.891 (0.295, 2.693) |
0.838 | 0.885 (0.293, 2.675) |
0.829 | |||
Operative procedure (sublobar vs. lobectomy) |
0.595 (0.187, 1.895) |
0.38 | |||||||||
p-TNM stage (II–III vs. I) | 2.378 (1.387, 4.078) |
0.002 | 2.705 (1.461, 5.010) |
0.002 | 4.277 (1.684, 10.87) |
0.002 | 4.277 (1.684, 10.87) |
0.002 | |||
LVI (presence vs. absence) | 1.921 (1.129, 3.267) |
0.016 | 1.272 (0.659, 2.455) |
0.474 | 1.212 (0.439, 3.350) |
0.711 | 1.453 (0.560, 3.770) |
0.443 | |||
VPI (presence vs. absence) | 2.463 (1.369, 4.431) |
0.003 | 2.476 (1.265, 4.846) |
0.008 | 3.780 (1.155, 12.37) |
0.028 | 3.780 (1.155, 12.37) |
0.028 | |||
Adjuvant chemotherapy (presence vs. absence) |
0.324 (0.197, 0.532) |
<0.001 | 0.254 (0.129, 0.500) |
<0.001 | 0.183 (0.076, 0.442) |
<0.001 | 0.183 (0.076, 0.442) |
<0.001 | |||
PNI status (high vs. low) | 0.391 (0.209, 0.728) |
0.003 | 0.473 (0.241, 0.929) |
0.03 | |||||||
NLR status (high vs. low) | 2.369 (1.324, 4.238) |
0.004 | 1.772 (0.743, 4.224) |
0.197 | |||||||
Neutrophil (high vs. low) | 2.628 (1.406, 4.910) |
0.002 | 1.335 (0.472, 3.778) |
0.586 |
OS, overall survival; HR, hazard ratio; CI, confidence interval; TNM, Tumor Node Metastasis; LVI, lymphovascular invasion; VPI, visceral pleural invasion; PNI, prognostic nutritional index; NLR, neutrophil-lymphocyte ratio.
Collectively, compared with other peripheral blood immune cells and index, the PNI score might be a better indicator representing systemic immune competence, as well as a strong independent prognostic factor in SCLC, which was vital for subsequent analyses.
Correlation between PNI, clinicopathological factors and molecular subtypes
The clinicopathological features of all surgically resected SCLC patients stratified by PNI score are summarized in Table 2. This cohort included 163 men (85.3%) and 28 women (14.7%), with a median age of 63 years [interquartile range (IQR), 57–68 years]. Out of 191 cases, 134 (70.2%) presented with low PNIs and 57 (29.8%) presented with high PNIs. There were no significant differences in age, sex, tobacco use, operative procedure, tumor stages, VPI and LVI status, ACT, or hyponatremia status and LDH levels between PNI-high and PNI-low groups, which also suggested the innate prognostic value of PNI but not distribution bias and cachexia conditions.
Table 2
Variables | Total patients (N=191) | PNI | P | |
---|---|---|---|---|
Low (≤55.5), N=134 | High (>55.5), N=57 | |||
Age (year) | 0.271 | |||
Median | 63 | 64 | 61 | |
IQR | 57–68 | 58–68 | 55–68 | |
Gender | 0.874 | |||
Male | 163 (85.3) | 114 (85.1) | 49 (86.0) | |
Female | 28 (14.7) | 20 (14.9) | 8 (14.0) | |
Smoking history | 0.594 | |||
Never | 45 (23.6) | 33 (24.6) | 12 (21.1) | |
Ever | 146 (76.4) | 101 (75.4) | 45 (78.9) | |
Operative procedure | 0.484 | |||
Lobectomy | 175 (91.6) | 124 (92.5) | 51 (89.5) | |
Sublobar resection | 16 (8.4) | 10 (7.5) | 6 (10.5) | |
p-TNM stage | 0.631 | |||
I | 78 (40.8) | 52 (38.8) | 26 (45.6) | |
II | 44 (23.0) | 31 (23.1) | 13 (22.8) | |
III | 69 (36.1) | 51 (38.1) | 18 (31.6) | |
Tumor stage | 0.493 | |||
pT1 | 108 (56.5) | 74 (55.2) | 34 (59.6) | |
pT2 | 63 (33.0) | 45 (33.6) | 18 (31.6) | |
pT3 | 15 (7.9) | 10 (7.5) | 5 (8.8) | |
pT4 | 5 (2.6) | 5 (3.7) | 0 | |
Nodal status | 0.420 | |||
pN0 | 90 (47.1) | 59 (44.0) | 31 (54.4) | |
pN1 | 36 (18.8) | 27 (20.1) | 9 (15.8) | |
pN2 | 65 (34.0) | 48 (35.8) | 17 (29.8) | |
*VPI | 0.528 | |||
Present | 31 (17.2) | 23 (18.4) | 8 (14.5) | |
Absent | 149 (82.8) | 102 (81.6) | 47 (85.5) | |
*LVI | 0.467 | |||
Present | 78 (44.8) | 56 (46.7) | 22 (40.7) | |
Absent | 96 (55.2) | 64 (53.3) | 32 (59.3) | |
ACT | 0.094 | |||
Present | 142 (74.3) | 95 (70.9) | 47 (82.5) | |
Absent | 49 (25.7) | 39 (29.1) | 10 (17.5) | |
Hyponatremia | 0.203 | |||
Present (<135 mmol/L) | 8 (4.2) | 4 (3.0) | 4 (7.0) | |
Absent (≥135 mmol/L) | 183 (95.8) | 130 (97.0) | 53 (93.0) | |
LDH level | 0.443 | |||
>230 U/L | 7 (3.7) | 4 (3.0) | 3 (5.3) | |
≤230 U/L | 184 (96.3) | 130 (97.0) | 54 (94.7) |
*, only cases with available data were reported. PNI, prognostic nutritional index; IQR, interquartile range; TNM, Tumor Node Metastasis; VPI, visceral pleural invasion; LVI, lymphovascular invasion; ACT, adjuvant chemotherapy; LDH, lactate dehydrogenase.
Recently, a novel molecular subtype model of SCLC defined by four transcription factors (ASCL1, NEUROD1, POU2F3 and YAP1, designated as SCLC-A, N, P, Y, respectively) has been proposed and these subtypes are reported to have distinct therapeutic vulnerabilities (19). Therefore, we next evaluated whether PNI correlated with different molecule subtype of SCLC. Using IHC in available TMAs and slides, 145 cases were successfully classified as one of these subtypes (Figure 2A).
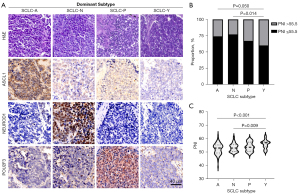
As illustrated in Figure 2B,2C, the proportion of high-PNI score cases in SCLC-Y subtype was significantly higher than other subtypes (P=0.043). The mean value of PNI score in SCLC-Y was also significantly higher than that in other subtypes when considered as a continuous variable (P=0.003).
In summary, our results revealed a certain degree of intrinsic association of systemic immune and nutritional status with tumor molecular subtypes in SCLC. This demonstrates that tumor heterogeneity can be reflected in systemic immunity, and noninvasive PNI assessment can hint at the molecular subtyping of SCLC to a certain extent.
PNI, local immune contexture and immune risk system establishment
To evaluate the association between systemic immune environment and local TIME, we performed mIF and comprehensively analyzed the tumor-infiltrating lymphocytes (CD4+ T-cell, CD8+ T-cell, CD68+ macrophage) and immune checkpoint expression (PD-1, PD-L1, CTLA-4) on TMA slides (Figure 3A).
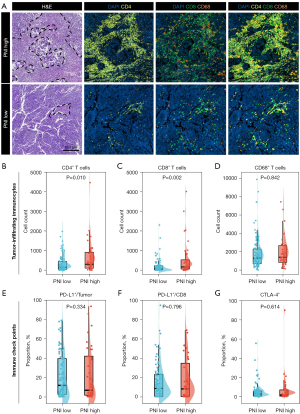
Quantification using mIF demonstrated that CD4+ T-cell (Figure 3B) and CD8+ T-cell counts (Figure 3C) were relatively higher in high-PNI group when compared to low-PNI group (P=0.010 and P=0.002, respectively). However, the CD68+ cell count and expression levels of immune checkpoints whether on tumor cells or lymphocytes had no association with PNI score (Figure 3D-3G). PD-1 expression was not detected in most cases, which was therefore not included in subsequent analyses.
Further survival analyses of these local immune markers indicated different degrees of prognostic value (Figure 4A). For example, higher CD8+ T-cell count correlated significantly with better prognosis (P<0.001) while patients with higher percentage of PD-L1+ cell in CD8+ T-cell had a significantly worse prognosis (P=0.016). And several local immune markers were associated with patient pathological TNM stages (Figure S2A). Spearman’s correlation analysis showed that there were positive correlations among these indicators (Figure S2B).
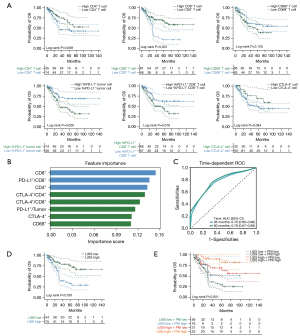
When the variables were correlated, the traditional Cox model was not applicable. Therefore, we adapted XGBoost machine learning algorithm for importance assessment and feature selection. This method can discover non-linear relationships by working with data of the first and second derivatives, as well as controlling the overfitting and overcomplexity of one predictive model. The importance feature map demonstrated CD8+ T-cell count as the first rank, followed by PD-L1 expression on CD8+ T-cell and CD4+ T-cell count (Figure 4B). To construct LISS for patients with SCLC, we combined these top three biomarkers and the obtained model formula was as follows: LISS = (0.1437 × CD8) − (0.1353 × PD-L1/CD8) + (0.1323 × CD4). We performed time-dependent ROC curve analysis in the calibration set to validate the model performance and it showed well-predictive ability [area under the curve (AUC) at 3-year: 0.76; 5-year: 0.78, Figure 4C]. We further used X-tile to determine the LISS cut-off point (0.13) (Table S1), and divided patients into a high-LISS group and a low-LISS group. As shown in the survival diagram by Kaplan-Meier analysis, patients with higher LISS score had significantly better OS (P<0.001, Figure 4D) and DFS (P<0.001, Figure S2C).
To establish a comprehensive immune risk system integrating systemic tumor immune macroenvironment and local TIME, we further reclassified SCLC patients based on PNI score and LISS. The survival curve showed that the stratification based on the PNI and LISS can better distinguish patients with poor OS (P<0.001, Figure 4E) and DFS (P<0.001, Figure S2D). Another multivariate Cox regression model was built (Table S2) and this immune risk system was independently and significantly correlated with OS in SCLC [HR: 1.429, 95% confidence interval (CI): 1.028–1.986, P=0.034]. To further confirm the prognostic role of this immune risk system in SCLC, we compared the predictive performance between integrated immune risk system, TNM stages and single indicators. Among different indicators, this integrated immune risk system obtained the best AUC value of 0.75, which performed better than either TNM stages or single immune markers (Figure S2E).
High PNI score predicts PFS benefit from PD-L1 blockade
Local TIME has been well known for its key role on the responses to ICB therapies. However, the predictive value of systemic tumor immunity for immunotherapy was merely illustrated previously. It was recently reported that clinical benefit from immunotherapy is associated with tumor capacity of antigen presentation in SCLC. Therefore, we first assessed the correlations between antigen presentation machinery (APM) related gene (HLA-A, HLA-B, HLA-C, B2M, TAP1, TAP2) expressions and PNI and LISS. No correlation was observed between PNI score and APM related gene expressions (Figure S3A). However, high expression of APM related genes were associated with LISS-high groups (Figure S3B). We next attempt to validate the predictive power of the PNI score in an independent cohort of 91 patients with ES-SCLC received anti-PD-L1 immunotherapy (atezolizumab or durvalumab). Individual PFS of 91 patients with SCLC coupled with their clinical benefit, gender, age, smoking status, and immunotherapy was summarized in Figure 5A. There were no significant differences in age, sex, tobacco use or immunotherapy strategies between PNI-high and PNI-low groups (Table S3).
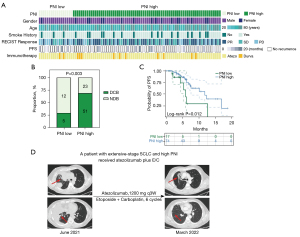
PNI-high tumors showed significantly more patients who experienced DCB, defined as best overall response of complete response (CR), partial response (PR), or SD (≥6 months) compared with PNI low tumors (P=0.003, Figure 5B). In addition, we also observed that the median PFS in PNI-high patients (11.8 months) was significantly higher than that in PNI-low patients (5.9 months) (P=0.012, Figure 5C). Last, we identified a patient with high-PNI SCLC. This patient was diagnosed with ES-SCLC and malignant pleural effusion in June 2021. The patient then began treatment with 1,200 mg of atezolizumab every 3 weeks combined with six cycles of carboplatin and etoposide and showed a durable PR for nine months, with no evidence of progression or active disease at the latest follow-up in March 2022 (Figure 5D).
In total, these data suggest that PNI-high tumors may portend survival benefit in patients with ICBs. The PNI score was not only a prognostic factor for early resected SCLCs but also a predictor for immunotherapy benefit in advanced tumors, which may better assist surgeons in identifying potential beneficiaries of ICB therapy. However, the lack of local tumor immune features of this immunotherapy cohort, mainly due to unavailable tumor tissues, hindered the construction of integrated immune system for efficacy prediction, which should be further studied.
Discussion
Despite the use of multimodal treatments, including surgery, postoperative chemotherapy and radiotherapy, and prophylactic cranial irradiation (PCI), the overall prognosis of SCLC patients is still unsatisfactory. In recent years, ICBs have significantly altered survival outcomes in many tumor types (20,21). The Food and Drug Administration (FDA) has approved PD-L1 inhibitors for use in more than 10 cancer types (22) including advanced SCLC. However, the number of SCLC patients who respond to the PD-L1 inhibitors is few and the survival benefit gained by responders is minimal (23-26). One explanation could be low or absent expression of checkpoint molecules and rare TILs infiltration in SCLC (6,27-29), and the application of ICBs in SCLC patients is still unfavorable. To date, there are no guidelines surrounding the administration of ICBs in SCLC and no predictive biomarkers have been established. In this study, therefore, we aimed to provide the comprehensive immune contexture by considering both systemic and local tumor immunity, and investigated their predictive value for prognosis and treatment efficacy.
We demonstrated that the PNI score was an outstanding, independent prognostic factor in patients with resectable SCLC, which was superior to other systemic immune cells and markers such as neutrophil and NLR. As an indicator for systemic immune competence and nutritional status, the PNI was initially designed to evaluate surgical complications and postoperative mortality in patients with gastrointestinal cancers (30,31). Since then, several studies have revealed that PNI is significantly associated with prognosis in various types of cancers including NSCLC (9,11,12,32). Nevertheless, the prognostic value of PNI in SCLC has not been reported, possibly hampered by small sample size. Our study first assessed PNI status in a much larger cohort of surgically resected patients with SCLC, and revealed that PNI-low was associated with an unfavorable clinical outcome. Moreover, considering that postoperative ACT could significantly impact the survival of limited-resected SCLC patients, the result that PNI remained as an independent prognostic factor regardless of ACT significantly increased its extensiveness of clinical applicability. Given that the PNI can be measured easily from clinical tests (10,33), it might be suitable as a routine prognostic marker for SCLC patients.
There are extensive interactions between systemic immune status (i.e., STIE) and TIME through capillary vessels and lymphatic drainage system in the tumor (34). Thus, we speculated that the PNI might influence patients prognosis via local tumor immunity. Okadome et al. (10) confirmed the relationship between high-PNI score and abundant CD8+ TILs in patients with esophageal cancer. Kitahara et al. (35) also showed that the absolute number of TILs positive for CD3, CD4 and CD8 in the high-PNI lung squamous cell carcinoma patients was significantly increased compared with the low-PNI group. These are similar to our results. Furthermore, we additionally revealed the complex interrelationship between STIE, TIME and tumor heterogeneity. We found that the median PNI score in SCLC-I subtype, a recently proposed infiltrated-inflamed “hot” subtype (19), was higher than that in other subtypes. Given that the SCLC-I subtype was observed deriving greater benefit from ICB therapy, our observations are likely to have clinical implications. In fact, the predictive power of PNI in our PD-L1 inhibitors-treated SCLC cohort was confirmed. High-PNI patients achieved longer PFS and better overall response than low-PNI group, which may assist surgeons in identifying potential beneficiaries of ICB therapy.
Nevertheless, a single indicator is far from accurate prognostic assessment and appropriate regimens selection. To establish a simple and practical tool incorporating both systemic and local tumor immunity features, we first constructed a XGBoost-based local immune risk model which consisted of top three local immune markers, and further integrated it with the PNI to provide a personalized system for patients with SCLC to predict outcomes. It turned out that in comparison with single biomarkers as well as TNM stages, this integrated immune risk system exhibited the best prediction performance with the maximal AUC value (Figure S2), and might provide the possibility for patients with the same TNM stage to receive different treatments to improve their long-term prognosis.
Our study has several limitations. First, no validation cohort was included in this study. The two cohorts were distinct in tumor stages and treatments, and these findings must be treated with caution and validated in future multi-center studies with larger sample size. Second, although most patients draw blood tests within 7 days before the surgery or immunotherapy, the longest interval was up to 15 days and the patient’s nutritional status could change significantly over days. Third, this was a retrospective, single-institution study. Thus, selection bias and time-trend bias are inevitable.
Conclusions
These findings show a strong relationship between systemic immune index and TIL intensity, patient survival and immunotherapy efficacy, suggesting its potential use as biomarker and shedding light on potential mechanistic relationships between the systemic response and local TME, pointing the way to further studies.
Acknowledgments
We thank the Department of Pathology, Fudan University Shanghai Cancer Center for providing the SCLC tissue samples and related anonymous clinical data.
Funding: This work was supported by
Footnote
Reporting Checklist: The authors have completed the TRIPOD reporting checklist. Available at https://tlcr.amegroups.com/article/view/10.21037/tlcr-23-696/rc
Data Sharing Statement: Available at https://tlcr.amegroups.com/article/view/10.21037/tlcr-23-696/dss
Peer Review File: Available at https://tlcr.amegroups.com/article/view/10.21037/tlcr-23-696/prf
Conflicts of Interest: All authors have completed the ICMJE uniform disclosure form (available at https://tlcr.amegroups.com/article/view/10.21037/tlcr-23-696/coif). C.D. reports funding from Shanghai Anticancer Association EYAS PROJECT (No. SACA-CY21B07). J.W. reports funding from the Shanghai Health Commission Collaborative Innovation Project (No. 2020CXJQ02). H.C. reports funding from the National Natural Science Foundation of China (No. 81930073) and Shanghai Technology Innovation Action Project (No. 20JC1417200). Y.Z. reports funding from Shanghai Rising-Star Program (No. 21QC1400600). The other authors have no conflicts of interest to declare.
Ethical Statement: The authors are accountable for all aspects of the work in ensuring that questions related to the accuracy or integrity of any part of the work are appropriately investigated and resolved. This study was approved by the Institutional Review Board of FUSCC (IRB No. 2008223-9). Informed consents were waived because it was a retrospective study. The study was conducted in accordance with the Declaration of Helsinki (as revised in 2013).
Open Access Statement: This is an Open Access article distributed in accordance with the Creative Commons Attribution-NonCommercial-NoDerivs 4.0 International License (CC BY-NC-ND 4.0), which permits the non-commercial replication and distribution of the article with the strict proviso that no changes or edits are made and the original work is properly cited (including links to both the formal publication through the relevant DOI and the license). See: https://creativecommons.org/licenses/by-nc-nd/4.0/.
References
- van Meerbeeck JP, Fennell DA, De Ruysscher DK. Small-cell lung cancer. Lancet 2011;378:1741-55. [Crossref] [PubMed]
- Byers LA, Rudin CM. Small cell lung cancer: where do we go from here? Cancer 2015;121:664-72. [Crossref] [PubMed]
- Chung HC, Piha-Paul SA, Lopez-Martin J, et al. Pembrolizumab After Two or More Lines of Previous Therapy in Patients With Recurrent or Metastatic SCLC: Results From the KEYNOTE-028 and KEYNOTE-158 Studies. J Thorac Oncol 2020;15:618-27.
- Paz-Ares L, Dvorkin M, Chen Y, et al. Durvalumab plus platinum-etoposide versus platinum-etoposide in first-line treatment of extensive-stage small-cell lung cancer (CASPIAN): a randomised, controlled, open-label, phase 3 trial. Lancet 2019;394:1929-39. [Crossref] [PubMed]
- Horn L, Mansfield AS, Szczęsna A, et al. First-Line Atezolizumab plus Chemotherapy in Extensive-Stage Small-Cell Lung Cancer. N Engl J Med 2018;379:2220-9. [Crossref] [PubMed]
- Antonia SJ, López-Martin JA, Bendell J, et al. Nivolumab alone and nivolumab plus ipilimumab in recurrent small-cell lung cancer (CheckMate 032): a multicentre, open-label, phase 1/2 trial. Lancet Oncol 2016;17:883-95. [Crossref] [PubMed]
- Binnewies M, Roberts EW, Kersten K, et al. Understanding the tumor immune microenvironment (TIME) for effective therapy. Nat Med 2018;24:541-50. [Crossref] [PubMed]
- Hiam-Galvez KJ, Allen BM, Spitzer MH. Systemic immunity in cancer. Nat Rev Cancer 2021;21:345-59. [Crossref] [PubMed]
- Takahashi M, Sowa T, Tokumasu H, et al. Comparison of three nutritional scoring systems for outcomes after complete resection of non-small cell lung cancer. J Thorac Cardiovasc Surg 2021;162:1257-1268.e3. [Crossref] [PubMed]
- Okadome K, Baba Y, Yagi T, et al. Prognostic Nutritional Index, Tumor-infiltrating Lymphocytes, and Prognosis in Patients with Esophageal Cancer. Ann Surg 2020;271:693-700. [Crossref] [PubMed]
- Kanda M, Fujii T, Kodera Y, et al. Nutritional predictors of postoperative outcome in pancreatic cancer. Br J Surg 2011;98:268-74. [Crossref] [PubMed]
- Pinato DJ, North BV, Sharma R. A novel, externally validated inflammation-based prognostic algorithm in hepatocellular carcinoma: the prognostic nutritional index (PNI). Br J Cancer 2012;106:1439-45. [Crossref] [PubMed]
- Choi Y, Kim JW, Nam KH, et al. Systemic inflammation is associated with the density of immune cells in the tumor microenvironment of gastric cancer. Gastric Cancer 2017;20:602-11. [Crossref] [PubMed]
- Eisenhauer EA, Therasse P, Bogaerts J, et al. New response evaluation criteria in solid tumours: revised RECIST guideline (version 1.1). Eur J Cancer 2009;45:228-47. [Crossref] [PubMed]
- Qu S, Fetsch P, Thomas A, et al. Molecular Subtypes of Primary SCLC Tumors and Their Associations With Neuroendocrine and Therapeutic Markers. J Thorac Oncol 2022;17:141-53. [Crossref] [PubMed]
- Chen H, Carrot-Zhang J, Zhao Y, et al. Genomic and immune profiling of pre-invasive lung adenocarcinoma. Nat Commun 2019;10:5472. [Crossref] [PubMed]
- Livne M, Boldsen JK, Mikkelsen IK, et al. Boosted Tree Model Reforms Multimodal Magnetic Resonance Imaging Infarct Prediction in Acute Stroke. Stroke 2018;49:912-8. [Crossref] [PubMed]
- Liang H, Jiang K, Yan TA, et al. XGBoost: An Optimal Machine Learning Model with Just Structural Features to Discover MOF Adsorbents of Xe/Kr. ACS Omega 2021;6:9066-76. [Crossref] [PubMed]
- Gay CM, Stewart CA, Park EM, et al. Patterns of transcription factor programs and immune pathway activation define four major subtypes of SCLC with distinct therapeutic vulnerabilities. Cancer Cell 2021;39:346-360.e7. [Crossref] [PubMed]
- Marin-Acevedo JA, Kimbrough EO, Lou Y. Next generation of immune checkpoint inhibitors and beyond. J Hematol Oncol 2021;14:45. [Crossref] [PubMed]
- Kennedy LB, Salama AKS. A review of cancer immunotherapy toxicity. CA Cancer J Clin 2020;70:86-104. [Crossref] [PubMed]
- Takamori S, Takada K, Azuma K, et al. Prognostic Impact of PD-L2 Expression and Association with PD-L1 in Patients with Small-cell Lung Cancer. Anticancer Res 2018;38:5903-7. [Crossref] [PubMed]
- Reck M, Luft A, Szczesna A, et al. Phase III Randomized Trial of Ipilimumab Plus Etoposide and Platinum Versus Placebo Plus Etoposide and Platinum in Extensive-Stage Small-Cell Lung Cancer. J Clin Oncol 2016;34:3740-8. [Crossref] [PubMed]
- Rudin CM, Awad MM, Navarro A, et al. Pembrolizumab or Placebo Plus Etoposide and Platinum as First-Line Therapy for Extensive-Stage Small-Cell Lung Cancer: Randomized, Double-Blind, Phase III KEYNOTE-604 Study. J Clin Oncol 2020;38:2369-79. [Crossref] [PubMed]
- Ott PA, Elez E, Hiret S, et al. Pembrolizumab in Patients With Extensive-Stage Small-Cell Lung Cancer: Results From the Phase Ib KEYNOTE-028 Study. J Clin Oncol 2017;35:3823-9. [Crossref] [PubMed]
- Gadgeel SM, Pennell NA, Fidler MJ, et al. Phase II Study of Maintenance Pembrolizumab in Patients with Extensive-Stage Small Cell Lung Cancer (SCLC). J Thorac Oncol 2018;13:1393-9. [Crossref] [PubMed]
- Zhao X, Kallakury B, Chahine JJ, et al. Surgical Resection of SCLC: Prognostic Factors and the Tumor Microenvironment. J Thorac Oncol 2019;14:914-23. [Crossref] [PubMed]
- Bonanno L, Pavan A, Dieci MV, et al. The role of immune microenvironment in small-cell lung cancer: Distribution of PD-L1 expression and prognostic role of FOXP3-positive tumour infiltrating lymphocytes. Eur J Cancer 2018;101:191-200. [Crossref] [PubMed]
- Carvajal-Hausdorf D, Altan M, Velcheti V, et al. Expression and clinical significance of PD-L1, B7-H3, B7-H4 and TILs in human small cell lung Cancer (SCLC). J Immunother Cancer 2019;7:65. [Crossref] [PubMed]
- Smale BF, Mullen JL, Buzby GP, et al. The efficacy of nutritional assessment and support in cancer surgery. Cancer 1981;47:2375-81. [Crossref] [PubMed]
- Onodera T, Goseki N, Kosaki G. Prognostic nutritional index in gastrointestinal surgery of malnourished cancer patients. Nihon Geka Gakkai Zasshi 1984;85:1001-5.
- Jeon HG, Choi DK, Sung HH, et al. Preoperative Prognostic Nutritional Index is a Significant Predictor of Survival in Renal Cell Carcinoma Patients Undergoing Nephrectomy. Ann Surg Oncol 2016;23:321-7. [Crossref] [PubMed]
- Hirahara N, Tajima Y, Fujii Y, et al. Preoperative Prognostic Nutritional Index Predicts Long-term Outcome in Gastric Cancer: A Propensity Score-matched Analysis. Anticancer Res 2018;38:4735-46. [Crossref] [PubMed]
- Xu L, Zou C, Zhang S, et al. Reshaping the systemic tumor immune environment (STIE) and tumor immune microenvironment (TIME) to enhance immunotherapy efficacy in solid tumors. J Hematol Oncol 2022;15:87. [Crossref] [PubMed]
- Kitahara H, Shoji F, Akamine T, et al. Preoperative prognostic nutritional index level is associated with tumour-infiltrating lymphocyte status in patients with surgically resected lung squamous cell carcinoma. Eur J Cardiothorac Surg 2021;60:393-401. [Crossref] [PubMed]