A cross-trait study of lung cancer and its related respiratory diseases based on large-scale exome sequencing population
Highlight box
Key findings
• We identified rare coding variants and genes associated with respiratory diseases, revealing genetic pleiotropy with lung cancer. It quantified mediation effects of these variants on lung cancer development via other respiratory diseases. Pathway and protein interaction analyses elucidated functional significance, highlighting potential therapeutic targets.
What is known and what is new?
• Genetic variants were associated with respiratory diseases and genetic pleiotropy was observed in complex diseases like lung cancer.
• Rare coding variants associated with respiratory diseases were identified, broadening understanding beyond common variants. Mediation effects of these variants on lung cancer via other respiratory diseases were quantified, revealing complex disease relationships.
What is the implication, and what should change now?
• This research underscores the complex genetic underpinnings of respiratory diseases and lung cancer, highlighting the need for a comprehensive understanding of genetic factors in disease development. Healthcare practices should integrate genetic screening for respiratory diseases, considering both common and rare variants.
Introduction
Lung cancer is one of the most common and fatal cancer. A few respiratory diseases have been described as possible risk factors for lung cancer (1,2), such as chronic obstructive pulmonary disease (COPD) and emphysema. Existing studies have confirmed that the close relationship between COPD and lung cancer is not just about shared smoking exposure, but is likely to reflect in part, a shared genetic susceptibility to chronic smoking-induced inflammation (3). By parity of reasoning, these respiratory diseases might share a common mechanism with the development of lung cancer. Studying them can identify shared genetic factors and provide an important foundation for lung cancer prevention and early warning.
In the past decade, genome-wide association study (GWAS) has thoroughly changed the perception of complex diseases and provided us with a number of significant and compelling risk variants (4,5). However, GWAS tends to concentrate on common variants, which usually have weak effect sizes and are difficult to map to causal genes (6). From the view of natural evolution, common variants appear early and have withstood natural selection pressure. Nevertheless, low-frequency variants have emerged late and have not been eliminated during human evolution and are more likely to be functional (7). Thus, rare variants may play an essential role in the development of disease.
In recent years, next-generation sequencing technologies have been iteratively upgraded, and large cohort studies have been scaled up. The UK Biobank (UKB) provides us with an unprecedented chance to explore the effect of both common and rare variants in human diseases (8-10). Compared with previous sequencing studies with limited sample size, large-scale exome sequencing population enables sufficient statistical power that could be used to identify rare coding variants associated with diseases (11,12).
In this study, we analyzed lung cancer and five respiratory diseases (asthma, COPD, emphysema, fibrosis, and pneumonia) with significant genetic correlations with lung cancer. We performed a comprehensive association study using exome sequencing data from 427,934 UKB participants of European ancestry at both variant-level and gene-level. Subsequent cross-trait meta-analysis, functional analyses, and causal mediation inference comprehensively depicted the genetic relationship between these five respiratory diseases and lung cancer.
Methods
Study population and phenotypes
The UKB is a large population-based prospective cohort study from the UK with deep phenotypic and genetic data on approximately 500,000 individuals aged 40–69 years at enrollment (13). The work described herein was approved by the UKB under application No. 57471. All the phenotype data were accessed in March 2022. The study was conducted in accordance with the Declaration of Helsinki (as revised in 2013). Health-related outcomes were ascertained via individual record linkage to national cancer and mortality registries and hospital in-patient encounters. Cancer diagnoses were coded by the International Classification of Diseases version 10 (ICD-10) codes. Individuals with at least one recorded incident diagnosis of a borderline, in situ, or primary malignant cancer were defined as cases collected from data fields 41270 (diagnoses: ICD-10), 41202 (diagnoses: main ICD10), 40006 (type of cancer: ICD-10), and 40001 (primary cause of death: ICD-10). ICD-10 codes for other diseases included in the study is shown in Table S1.
The UKB provides detailed diseases follow-up information linked to whole-exome sequencing (WES) for approximately 450,000 participants (data field: 23148). We included 427,934 white European participants in this research, and detailed inclusion information is presented in Table S2. Ten respiratory diseases were analyzed (Table 1), including lung cancer (n=5,003), asthma (n=38,627), COPD (n=17,561), emphysema (n=4,002), idiopathic pulmonary fibrosis (n=2,340), pneumonia (n=21,424), bronchiectasis (n=4,660), acute bronchitis (n=14,364), chronic bronchitis (n=1,382), and tuberculosis (n=654).
Table 1
Characteristics | N (%) |
---|---|
Sex | |
Female | 232,409 (54.31) |
Male | 195,525 (45.69) |
Age (years) | |
38–49 | 95,773 (22.38) |
50–59 | 141,946 (33.17) |
60–73 | 190,215 (44.45) |
Smoke | |
Ever | 259,671 (60.68) |
Never | 166,895 (39.00) |
Diseases | |
Lung cancer | 5,003 (1.17) |
Asthma | 38,627 (9.03) |
COPD | 17,561 (4.10) |
Emphysema | 4,002 (0.94) |
Fibrosis | 2,340 (0.55) |
Pneumonia | 21,424 (5.01) |
Bronchiectasis | 4,660 (1.09) |
Acute bronchitis | 14,364 (3.36) |
Chronic bronchitis | 1,382 (0.32) |
Tuberculosis | 654 (0.15) |
UKB, UK Biobank; COPD, chronic obstructive pulmonary disease.
Genetic correlation estimation
To find the respiratory diseases with significant genetic relationships with lung cancer, we used the linkage disequilibrium score regression (LDSC) (14) to assess the genetic correlation between each disease pair using the imputed genetic variants from the UKB (data field: 22828) (15). We conducted a genetic correlation analysis on ten respiratory diseases using the imputed genotype data from the Haplotype Reference Consortium (HRC) and UK10K haplotype resource (data field: 22828), and utilized the resulting summary data to estimate genetic correlations, which was not biased by sample overlap (15). Diseases significantly genetically correlated with lung cancer (nominal P<0.05) were included in further analyses.
Quality control for the genetic variants
WES data for UKB participants were generated using the IDT xGen v1 capture kit on the NovaSeq6000 platform. The UKB 450k release was performed with a Functional Equivalence specification that retained the original quality scores (OQFE protocol) in the CRAM files (16). The OQFE protocol mapped to a full GRCh38 reference version including all alternative contigs in an alt-aware manner. The OQFE CRAMs were then called for small variants with DeepVariant 0.0.10 to generate per-sample genome variant call formats (gVCFs), which were aggregated and joint-genotyped with GLnexus 1.2.6 to create a single multi-sample VCF [project VCF (pVCF)] for all UKB 450k samples. Genotype depth (DP) filters [single nucleotide variant (SNV) DP ≥7, indel DP ≥10] were applied prior to variant site filters requiring at least one variant genotype passing an allele balance (AB) filter (heterozygous SNV AB >0.15, heterozygous indel <0.20). The detailed parameters were described in Category 170 of the UKB showcase. In addition, all the variants with call rate <90% and minor allele count (MAC) ≤1 were filtered out.
Single-variant association tests
Single-variant association tests were performed on 427,934 European participants. All the variants with MAC ≥10 were incorporated in the following association tests. We used SAIGE v1.1.4 (11) to conduct association tests based on logistic mixed models adjusting for age, gender, smoking status, and top five ancestry principal components to assess the association between the respiratory diseases and genetic variants (17,18). A genetic relationship matrix (GRM) was created to fit the model to eliminate the effect of kinship. We also included five principal components in mixed model to adjust for both population structures and non-genetic confounders (19). We included all the variants that passed quality control in the WES dataset, including loss-of-function (LOF), missense, synonymous, and a small proportion of non-coding variants. Variants passed the genome-wide significance threshold (P≤5×10−8) were defined as significant and independent variants were pruned out using the PLINK v1.9 clump function (-clump-r2 0.50, -clump-kb 500). We calculated the adjusted genomic inflation factor λadj. Because the genomic inflation factor increases with sample size, we rescaled the genomic inflation factor λobs to adjusted the genomic inflation factor λadj reflecting a standardized sample size of 1,000 cases and 1,000 controls based on the following formula:
If genomic inflation arose, SAIGE integrated linear mixed models to control for population structure and familial relationships, effectively mitigating genomic inflation.
Gene-based association tests for rare variants and ultra-rare variants
Afterward, we performed gene-level association tests using the SKAT-O method (20), which was implemented by SAIGE-GENE+ (21). Variants with minor allele frequency (MAF) ≤1% were considered rare, while variants with MAC ≤10 were considered ultra-rare. To improve power to detect the association signals, we performed tests for rare variants with different MAF cutoffs (MAF ≤1%, MAF ≤0.1%, and MAF ≤0.01%). According to the latest research that synonymous mutations may be strongly non-neutral (22), we considered all the functional annotations. Therefore, multiple variant sets with different MAF cutoffs and functional annotations (LOF, missense, and synonymous) were analyzed. We reported the association results with the lowest P value for one gene to collectively capture a wide range of genetic architectures (23). We used a P value threshold of P≤1×10−5 to report genes associated with these diseases.
Cross-trait meta-analysis for the respiratory diseases
We conducted cross-trait meta-analysis via the R package association analysis based on subsets (ASSET) (24). Briefly, ASSET explored all possible subsets of all six diseases (five lung cancer-related diseases and lung cancer) for the presence of association signals, resulting in the best combination of diseases to maximize the test statistic. According to the result of single-variant association tests, the variants with P value ≤1×10−4 in any one of the six diseases association tests were included. Because the method explores all possible subsets of studies and evaluates fixed-effect meta-analysis-type test-statistics for each subset, to avoid excessive computational effort, we used a relatively lenient P value to comprehensively consider all suggestive association variants across the six respiratory diseases.
By ASSET, we achieved the P values of significance for the overall evidence of association of a variant across these diseases as well as the “best subset” that contributed to the overall association signal (24). Finally, all the independent variants with P<5×10−8 were reported.
Intermediate pathways analysis
We were interested in whether there was a mediating effect of variants causing other respiratory diseases and consequently leading to lung cancer. Based on the cross-trait meta-analysis, we searched for variants shared between lung cancer and five other respiratory diseases. Then, we constructed polygenic scores (PGSs) for the shared variants found for each respiratory disease (25). PGS is not applied here for the purpose of disease risk prediction, but for the purpose of using the idea of PGS to comprehensively measure the impact of all shared variants and calculate the mediation effect using a unified indicator. The beta coefficients of each variant was used as the weight in PGSs: (26). We calculated the area under the receiver operator characteristic curves (AUCs) of all PGSs used for mediation analyses using Bootstrap. Finally, we carried out mediation analyses by these polygenic risk scores and identified mediating effects for these five respiratory diseases. All the mediation analyses were performed by R package “mediation”.
Genetic functional analysis
To explore further biological explanations and assess the biological functions of the pleiotropic genes, we conducted pathway enrichment analysis via Metascape (27). During this analysis, the gene list we detected were compared to thousands of gene sets defined by their involvement in specific biological processes, protein localization, pathway member, or other features (27). Pathway and process enrichment analysis had been carried out with the following ontology sources: Kyoto Encyclopedia of Genes and Genomes (KEGG), Gene Ontology (GO) biological processes, reactome gene sets, canonical pathways, and WikiPathways.
Metascape reported the terms with a P value <0.01, a minimum count of 3, and an enrichment factor >1.5 (the enrichment factor was the ratio between the observed counts and the counts expected by chance). Then the terms were grouped into clusters based on their membership similarities. Kappa scores were used as the similarity metric when clustering on the enriched terms (28), and sub-trees with similarity >0.3 were considered a cluster.
To further understand the protein-protein interactions, we used the Search Tool for the Retrieval of Interacting Genes-Proteins (STRING) database, which considered both physical interactions as well as functional associations (29). The protein-protein interaction network was clustered into different colors using k-means clustering.
We used the R software (version 4.2.0) for statistical analysis and graphing. All P values were two-sided.
Results
Genetic correlation of the respiratory diseases
Figure 1 depicts the study design and workflow. The cross-trait genetic correlation calculated by LDSC showed intricate relationships among respiratory diseases. Lung cancer was significantly genetically correlated with five respiratory diseases, including emphysema (rg=0.61, P=0.0001), pneumonia (rg=0.64, P=0.0018), asthma (rg=0.24, P=0.0056), COPD (rg=0.69, P=9×10−7), and idiopathic pulmonary fibrosis (rg=0.60, P=0.0285) (Figure S1). Therefore, we focused on the shared genetic basis for them and lung cancer in the subsequent exome sequencing analyses.
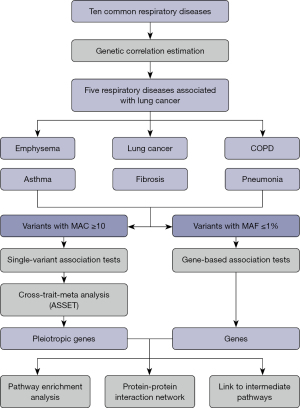
Single-variant association tests
In the single-variant association analysis, 102 independent loci mapped to 53 genes passed the genome-wide significance level (P<5×10−8). Of them, six were associated with lung cancer, six were associated with COPD, six were associated with emphysema, three were associated with idiopathic pulmonary fibrosis, three were associated with pneumonia, and 88 were associated with asthma (Figure 2). The adjusted genomic inflation factor λadj did not suggest population stratification (Figure S2). Noteworthy, only one genomic region 15q25.1 had shared signals with lung cancer. The sentinel variant 15:78590583:G>A (missense, HGVSp: p.Asp398Asn) in CHRNA5 were significant in lung cancer {odds ratio (OR) [95% confidence interval (CI)]: 1.22 (1.18, 1.26), P=8.41×10−20}, emphysema [OR (95% CI): 1.22 (1.16, 1.28), P=1.50×10−14], and COPD [OR (95% CI): 1.13 (1.10, 1.15), P=7.79×10−25]. CHRNA5 was related to the mechanism of nicotine addiction (30) in smoking that could lead to respiratory diseases (31,32).
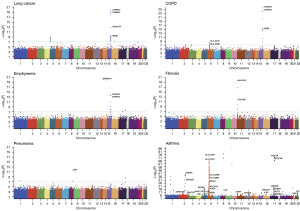
In addition, the missense variant 11:1167980:C>T [MAF =4.0%, OR (95% CI): 2.21 (1.87, 2.61), P=1.98×10−20] in MUC5AC was significant in fibrosis. The synonymous variant 6:32584335:A>G [MAF =9.3%, OR (95% CI): 1.18 (1.15, 1.21), P=2.20×10−37] in HLA-DRB1 was associated with asthma. Moreover, we identified 15 additional rare variants with MAF ≤1% in asthma, emphysema, and pneumonia. For example, the missense variant 9:5073770:G>T in JAK2 [MAF =0.03%, OR (95% CI): 6.23 (3.69, 10.51), P=7.14×10−12] was associated with pneumonia. These rare variants with large effects may play an essential role in the onset of respiratory diseases (33). All the association results for independent single variants with P<5×10−8 are shown in Table S3.
Gene-based association tests
We analyzed 18,184 protein-coding genes in the gene-based association tests and identified 14 significant genes (P≤1×10−5) (Figure 3, Table S4). Among them, HSD3B7 (P=7.7×10−7) and TARM1 (P=9.2×10−6) were associated with lung cancer; LACRT (P=6.8×10−6) was associated with COPD; MOSPD3 (P=2.7×10−6) was associated with emphysema; TERT (P=3.8×10−7) and LMNA (P=1.1×10−6) were associated with fibrosis; SRSF2 (P=7.4×10−15) and JAK2 (P=2.5×10−6) were associated with pneumonia; TNXB (P=2.4×10−7), C6orf10 (P=9.4×10−7), NOTCH4 (P=8.2×10−6), HLA-DQA2 (P=8.3×10−6), TTK (P=8.9×10−6), and SPINK7 (P=9.4×10−6) were associated with asthma. Moreover, 87 genes showed suggestive significance (P≤1×10−4) (Table S4).
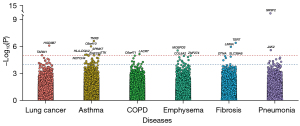
Shared genetic variants for the six respiratory diseases
A total of 781 independent variants that reached P<10−4 in any disease were included in the cross-trait meta-analysis. Strong evidence supported the shared genetic foundation underlying these six diseases that 145 independent variants had P ≤5×10−8 (Figure 4A, table available at https://cdn.amegroups.cn/static/public/tlcr-24-4-1.xlsx). The number of variants that overlapped between disease pairs is demonstrated in Figure 4B. Best subset of these diseases that contributed to the overall association signal is shown in Figure 4C. It was illustrated that lung cancer and its related respiratory diseases were genetically linked.
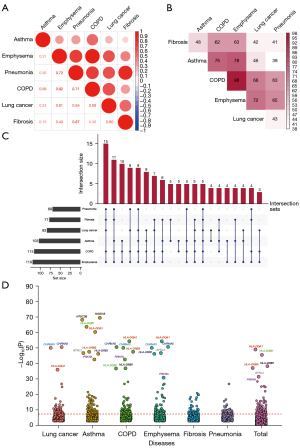
In summary, the independent pleiotropic genes associated with any two or more of these six diseases are shown in Figure 4D. Intuitively, human leukocyte antigen (HLA) family made a remarkable contribution to genetic interactions among these diseases. The pleiotropic genes that overlapped with lung cancer with the largest OR among all the significant genes in cross-trait meta-analysis are displayed in Table 2. In the previous analysis, few genes shared by respiratory diseases and lung cancer were found, but a large number of shared genes associated with lung cancer were found in this step. For example, missense variant 10:132909243:C>T in CFAP46 (MAF =1.2×10−5), missense variant 1:38017675:C>T in UTP11 (MAF =1.8×10−5), synonymous variant 19:42079623:C>T in ZNF574 (MAF =8.2×10−4), and LOF variant 1:16208697:G>A in ARHGEF19 (MAF =1.2×10−4) were all associated with lung cancer. Moreover, these genes were also strongly associated with other respiratory diseases.
Table 2
Marker ID | MAF | Gene | Annotation | P | OR (95% CI) | Subset |
---|---|---|---|---|---|---|
10:132909243:C>T | 1.2×10−5 | CFAP46 | Missense | 3.80×10−8 | 245.01 (39.31, 1,527.15) | LC, COPD, EM, PN, AS |
1:38017675:C>T | 1.8×10−5 | UTP11 | Missense | 1.03×10−8 | 240.05 (36.84, 1,564.14) | LC, COPD, EM, PN, AS |
14:94055016:T>C | 1.3×10−5 | DDX24 | Missense | 4.64×10−9 | 174.38 (33.01, 921.28) | LC, COPD, EM, PN, AS |
19:32383003:G>A | 2.6×10−5 | ZNF507 | Missense | 8.74×10−10 | 155.66 (32.04, 756.23) | LC, COPD, EM, FI, PN |
1:53264370:G>A | 2.1×10−5 | LRP8 | Missense | 1.71×10−10 | 64.05 (15.98, 256.72) | LC, COPD, EM, FI, PN, AS |
19:42079623:C>T | 8.2×10−4 | ZNF574 | Synonymous | 1.96×10−8 | 11.47 (4.73, 27.80) | LC, COPD, FI, PN |
1:16208697:G>A | 1.2×10−4 | ARHGEF19 | LOF | 4.05×10−9 | 11.42 (5.44, 24.00) | LC, COPD, EM, FI, PN |
1:43313932:G>A | 1.6×10−4 | TIE1 | Synonymous | 3.87×10−8 | 5.00 (2.74, 9.11) | LC, EM, PN, AS |
1:171783869:C>T | 5.9×10−4 | METTL13 | Missense | 1.18×10−9 | 2.26 (1.70, 3.02) | LC, EM, PN, AS |
OR, odds ratio; MAF, minor allele frequency; CI, confidence interval; LC, lung cancer; COPD, chronic obstructive pulmonary disease; EM, emphysema; PN, pneumonia; AS, asthma; FI, fibrosis; LOF, loss-of-function.
Intermediate causal pathways
Based on the identified pleiotropic variants, we screened for shared genetic variants for the respiratory diseases and lung cancer, and the shared variants and their weights are provided in table available at https://cdn.amegroups.cn/static/public/tlcr-24-4-2.xlsx. Then, the PGS was constructed for these five respiratory diseases. The AUCs (95% CI) of PGS_asthma (PGS_AS), PGS_COPD, PGS_emphysema (PGS_EM), PGS_fibrosis (PGS_FI), and PGS_pneumonia (PGS_PN) are shown in Table S5. Because only shared variants with lung cancer were included in the PGS models, the AUCs performed moderately, but they were all statistically significant.
Applying causal mediation analysis to these polygenic risk scores, we identified the mediating effect of variants causing other respiratory diseases and consequently leading to lung cancer. The direct effect (DE), indirect effect (IE), proportion of mediation, and corresponding significant P value are all shown in Figure 5. The mediating effect of COPD was 20%, the mediating effect of emphysema was 23%, and the mediating effect of pneumonia was 20%. This further suggested the existence of some shared variants by causing the development of these three respiratory diseases, which in turn allowed patients to eventually develop lung cancer. However, the mediating effect of asthma and fibrosis was relatively low.
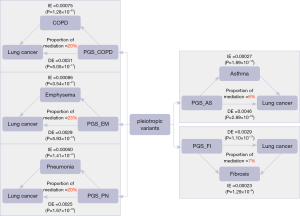
Pathway enrichment analysis and protein-protein interaction network for the pleiotropic genes
We performed gene set enrichment analyses for the 157 unique pleiotropic genes based cross-trait meta-analysis and gene-based association tests using Metascape. We discovered 146 significant pathways [false discovery rate (FDR)-q <0.05] (table available at https://cdn.amegroups.cn/static/public/tlcr-24-4-3.xlsx). The top significant pathways were immune-related, such as phosphorylation of CD3 and TCR zeta chains (P=1.35×10−13), which was associated with T cell receptors (34,35). For the KEGG terms, Th17 cell differentiation (P=1.62×10−6) and T helper (Th)1 and Th2 cell differentiation (P=2.88×10−8) were significant. The biological process of these pleiotropic genes was prominently enriched in positive regulation of immune response (P=5.01×10−8) and regulation of immune effector process (P=3.98×10−7) (Figure S3A).
Furthermore, additional functional pathways were discovered, including regulation of leukocyte proliferation (P=8.13×10−6), response to the bacterium (P=3.09×10−5), regulation of cell-cell adhesion mediated by cadherin (P=1.70×10−4). Meanwhile, the KEGG and Wiki enrichment analysis identified these genes enriched in some respiratory-related pathways, such as asthma (P=2.12×10−10), staphylococcus aureus infection (pulmonary infection, P=7.26×10−7), tuberculosis (P=4.90×10−6), pathogenesis of SARS-CoV-2 mediated by nsp9-nsp10 complex (P=1.71×10−4) and lung fibrosis (P=3.30×10−4).
Meanwhile, we used the STRING database to explore all known and predicted protein-protein interactions among these 157 protein-coding genes (Figure S3B). We identified four large clusters: first was related to the HLA and immune function; second was related to cell adhesion and leukocyte proliferation (including asthma); third was related to pulmonary diseases (lung cancer and COPD) and telomerase; the last was related to inflammatory response. Overall, these results further supported the effect of the identified pleiotropic genes on the respiratory system.
Discussion
In the present work, we comprehensively evaluated the shared genetics of human exome on lung cancer-related respiratory diseases in approximately 420,000 UKB participants of European ancestry, which could improve the statistical power and compensate for the neglect of rare variants in GWASs. To our knowledge, this is the first exome-wide association study for respiratory diseases including almost the whole UKB population. We systematically examined nine common respiratory diseases and identified five that were significantly associated with lung cancer. Based on the single-variant and gene-based association tests, we carried out cross-trait meta-analysis and pathway enrichment analysis, which provided crucial insights into genetic background underlying these diseases and revealed their shared genetic factors with lung cancer. It is worthy to note that there is a small overlap of cases among between these six diseases in which while overlapping subjects can inflate the test statistics of association signals (36,37). Therefore, we adopted ASSET to perform cross-trait meta-analysis to reduce the bias by overlapping subjects (38). Moreover, based on the signal genes and pleiotropic genes, we analyzed protein-protein interaction and identified key modules. Pathway enrichment analysis confirmed that these genes were associated with immune system function and cancer development.
Our first major discovery was that exome-wide signals were associated with the lung cancer-related respiratory diseases. In addition to some known genes (e.g., CHRNA3, CHRNA5) (39,40), we identified novel genes that have not been reported. HSD3B7, which had previously found to be been linked to immune and bile acid function (41,42), was found to be associated with lung cancer. The 3-beta-HSD enzymatic system plays a crucial role in the biosynthesis of all classes of hormonal steroids and HSD VII is active against four 7-alpha-hydroxylated sterols. SRSF2 and JAK2 are highly associated with the occurrence of pneumonia, SRSF2 is necessary for the splicing of pre-messenger RNA (pre-mRNA), and is required for formation of the earliest ATP-dependent splicing complex and interacts with spliceosomal components bound to both the 5’- and 3’-splice sites during spliceosome assembly. While SRSF2 was found to contribute to myelodysplasia in previous study (43), JAK2 regulates non-receptor tyrosine kinase involved in various processes such as cell growth, development, differentiation or histone modifications, and mediates essential signaling events in both innate and adaptive immunity.
Our second major contribution was the exploration of the pleiotropic variants shared among lung cancer-related diseases. In single-variant association tests, we did not identify lots of shared variants between respiratory diseases and lung cancer. Nevertheless, we identified 83 shared variants between lung cancer and other five respiratory diseases through cross-trait meta-analysis. Among these shared variants, there were several with incredibly large OR values. This was attributed to the inverse relationship between MAF and OR, where smaller MAFs yield larger ORs. If these variants meet significance thresholds, it suggests their potential significant roles in the occurrence and progression of respiratory diseases, warranting focused investigation. We observed strong functional evidence for the identified genes from the KEGG pathway, GO pathway, Wiki pathway, and protein-protein interaction network. These genes significantly enriched in immune, inflammation, and cell adhesion pathway, which were closely associated with the development of diseases. From protein-protein interaction network, we found these pleiotropic genes could be grouped into four clusters with distinct biological function. The most well-known is HLA family locating on chromosome 6, which is considered as the most polymorphic regions of the human genome (44). Various mutations of HLA are deeply related with immune evasion events and progression of diseases including multiple cancers (45,46). Other clusters included cell adhesion and leukocyte proliferation, pulmonary diseases and telomerase and inflammatory response. These biological processes are closely related to the development of respiratory diseases and even lung cancer, further confirming the important role of these pleiotropic genes in lung cancer and its related respiratory diseases.
Our third major contribution was the exploration of the relation of genetic variants and intermediate causal pathways. We assume that some pleiotropic variants might contribute to the development of lung cancer by causing other respiratory diseases first. Many respiratory diseases have been commonly considered to be risk factors for lung cancer (3,47,48). It is reasonable to assume that there may be some variants that first cause certain respiratory diseases that lead to the development of lung cancer. We calculated the mediating effects of COPD, emphysema, pneumonia, asthma, and fibrosis. The mediating effect was significant for all five lung cancer-related diseases, and the proportions of the mediating effect for COPD, emphysema, and pneumonia all exceeded 20%, which further proved the relationship between these shared pleiotropic variants and the occurrence of lung cancer. Understanding the impact of these common respiratory diseases on lung cancer at the level of shared pleiotropic variants can help us to better assess the risk of lung cancer and to provide effective early warning and prevention of lung cancer.
Our work has several prominent features. First, we comprehensively evaluated the exome-wide genetic variants in six respiratory diseases among 420,000 participants and discovered many coding variants that are difficult to find in traditional GWAS studies. We analyzed the genetic pleiotropy centered on lung cancer and identified the potential shared genes through cross-trait meta-analysis. Second, we identified the proportion of mediation effects of these pleiotropic variants by causing other respiratory diseases to develop, which in turn cause lung cancer. Third, we explored the relationship between identified genes and diseases by exhaustive enrichment pathway analysis and protein-protein interaction analysis.
It is essential to acknowledge the limitations of our study. First, this study was conducted with the UKB population only. The results need further replication in external independent cohorts with large-scale sequencing profiles. Second, we focused on individuals of European ancestry only, and the number of incident lung cancer cases in the UKB is low (n≈4,000) and provides insufficient power to assess the effects of rare variants. It is necessary to include individuals from non-European ancestries in genetic analyses, which is crucial for healthcare equity and genetic discovery (49). Third, the candidate genes were reported based on statistical evidence and further basic medical experimental studies are still needed to confirm.
Conclusions
Our study provides novel insights into human exomes and rare variants through comprehensive analyses of genetic susceptibility to lung cancer-related diseases and subsequent exploration of shared pleiotropic genes and potential causal pathways.
Acknowledgments
Funding: This study was supported by
Footnote
Peer Review File: Available at https://tlcr.amegroups.com/article/view/10.21037/tlcr-24-4/prf
Conflicts of Interest: All authors have completed the ICMJE uniform disclosure form (available at https://tlcr.amegroups.com/article/view/10.21037/tlcr-24-4/coif). The authors have no conflicts of interest to declare.
Ethical Statement: The authors are accountable for all aspects of the work in ensuring that questions related to the accuracy or integrity of any part of the work are appropriately investigated and resolved. The study was conducted in accordance with the Declaration of Helsinki (as revised in 2013).
Open Access Statement: This is an Open Access article distributed in accordance with the Creative Commons Attribution-NonCommercial-NoDerivs 4.0 International License (CC BY-NC-ND 4.0), which permits the non-commercial replication and distribution of the article with the strict proviso that no changes or edits are made and the original work is properly cited (including links to both the formal publication through the relevant DOI and the license). See: https://creativecommons.org/licenses/by-nc-nd/4.0/.
References
- Mouronte-Roibás C, Leiro-Fernández V, Fernández-Villar A, et al. COPD, emphysema and the onset of lung cancer. A systematic review. Cancer Lett 2016;382:240-4. [Crossref] [PubMed]
- Zhu Z, Guo Y, Shi H, et al. Shared genetic and experimental links between obesity-related traits and asthma subtypes in UK Biobank. J Allergy Clin Immunol 2020;145:537-49. [Crossref] [PubMed]
- Young RP, Hopkins RJ, Christmas T, et al. COPD prevalence is increased in lung cancer, independent of age, sex and smoking history. Eur Respir J 2009;34:380-6. [Crossref] [PubMed]
- Visscher PM, Brown MA, McCarthy MI, et al. Five years of GWAS discovery. Am J Hum Genet 2012;90:7-24. [Crossref] [PubMed]
- Visscher PM, Wray NR, Zhang Q, et al. 10 Years of GWAS Discovery: Biology, Function, and Translation. Am J Hum Genet 2017;101:5-22. [Crossref] [PubMed]
- Claussnitzer M, Cho JH, Collins R, et al. A brief history of human disease genetics. Nature 2020;577:179-89. [Crossref] [PubMed]
- Raychaudhuri S. Mapping rare and common causal alleles for complex human diseases. Cell 2011;147:57-69. [Crossref] [PubMed]
- Cirulli ET, White S, Read RW, et al. Genome-wide rare variant analysis for thousands of phenotypes in over 70,000 exomes from two cohorts. Nat Commun 2020;11:542.
- Van Hout CV, Tachmazidou I, Backman JD, et al. Exome sequencing and characterization of 49,960 individuals in the UK Biobank. Nature 2020;586:749-56. [Crossref] [PubMed]
- Wang Q, Dhindsa RS, Carss K, et al. Rare variant contribution to human disease in 281,104 UK Biobank exomes. Nature 2021;597:527-32. [Crossref] [PubMed]
- Zhou W, Nielsen JB, Fritsche LG, et al. Efficiently controlling for case-control imbalance and sample relatedness in large-scale genetic association studies. Nat Genet 2018;50:1335-41. [Crossref] [PubMed]
- Jiang L, Zheng Z, Fang H, et al. A generalized linear mixed model association tool for biobank-scale data. Nat Genet 2021;53:1616-21. [Crossref] [PubMed]
- Bycroft C, Freeman C, Petkova D, et al. The UK Biobank resource with deep phenotyping and genomic data. Nature 2018;562:203-9. [Crossref] [PubMed]
- Bulik-Sullivan BK, Loh PR, Finucane HK, et al. LD Score regression distinguishes confounding from polygenicity in genome-wide association studies. Nat Genet 2015;47:291-5. [Crossref] [PubMed]
- Bulik-Sullivan B, Finucane HK, Anttila V, et al. An atlas of genetic correlations across human diseases and traits. Nat Genet 2015;47:1236-41. [Crossref] [PubMed]
- Szustakowski JD, Balasubramanian S, Kvikstad E, et al. Advancing human genetics research and drug discovery through exome sequencing of the UK Biobank. Nat Genet 2021;53:942-8. [Crossref] [PubMed]
- Bouaziz M, Ambroise C, Guedj M. Accounting for population stratification in practice: a comparison of the main strategies dedicated to genome-wide association studies. PLoS One 2011;6:e28845. [Crossref] [PubMed]
- Kang M, Ang TFA, Devine SA, et al. A genome-wide search for pleiotropy in more than 100,000 harmonized longitudinal cognitive domain scores. Mol Neurodegener 2023;18:40. [Crossref] [PubMed]
- Zhang Y, Pan W. Principal component regression and linear mixed model in association analysis of structured samples: competitors or complements? Genet Epidemiol 2015;39:149-55. [Crossref] [PubMed]
- Lee S, Wu MC, Lin X. Optimal tests for rare variant effects in sequencing association studies. Biostatistics 2012;13:762-75. [Crossref] [PubMed]
- Zhou W, Bi W, Zhao Z, et al. SAIGE-GENE+ improves the efficiency and accuracy of set-based rare variant association tests. Nat Genet 2022;54:1466-9. [Crossref] [PubMed]
- Shen X, Song S, Li C, et al. Synonymous mutations in representative yeast genes are mostly strongly non-neutral. Nature 2022;606:725-31. [Crossref] [PubMed]
- Li X, Li Z, Zhou H, et al. Dynamic incorporation of multiple in silico functional annotations empowers rare variant association analysis of large whole-genome sequencing studies at scale. Nat Genet 2020;52:969-83. [Crossref] [PubMed]
- Bhattacharjee S, Rajaraman P, Jacobs KB, et al. A subset-based approach improves power and interpretation for the combined analysis of genetic association studies of heterogeneous traits. Am J Hum Genet 2012;90:821-35.
- Hung RJ, Warkentin MT, Brhane Y, et al. Assessing Lung Cancer Absolute Risk Trajectory Based on a Polygenic Risk Model. Cancer Res 2021;81:1607-15. [Crossref] [PubMed]
- Khera AV, Chaffin M, Aragam KG, et al. Genome-wide polygenic scores for common diseases identify individuals with risk equivalent to monogenic mutations. Nat Genet 2018;50:1219-24. [Crossref] [PubMed]
- Zhou Y, Zhou B, Pache L, et al. Metascape provides a biologist-oriented resource for the analysis of systems-level datasets. Nat Commun 2019;10:1523. [Crossref] [PubMed]
- Cohen J. A coefficient of agreement for nominal scales. Educ Psychol Meas 1960;20:37-46.
- Szklarczyk D, Gable AL, Nastou KC, et al. The STRING database in 2021: customizable protein-protein networks, and functional characterization of user-uploaded gene/measurement sets. Nucleic Acids Res 2021;49:D605-12. [Crossref] [PubMed]
- Picciotto MR, Kenny PJ. Mechanisms of Nicotine Addiction. Cold Spring Harb Perspect Med 2021;11:a039610. [Crossref] [PubMed]
- Ware JJ, van den Bree M, Munafò MR. From men to mice: CHRNA5/CHRNA3, smoking behavior and disease. Nicotine Tob Res 2012;14:1291-9. [Crossref] [PubMed]
- Zhou JS, Li ZY, Xu XC, et al. Cigarette smoke-initiated autoimmunity facilitates sensitisation to elastin-induced COPD-like pathologies in mice. Eur Respir J 2020;56:2000404. [Crossref] [PubMed]
- Povysil G, Petrovski S, Hostyk J, et al. Rare-variant collapsing analyses for complex traits: guidelines and applications. Nat Rev Genet 2019;20:747-59. [Crossref] [PubMed]
- Wu W, Zhou Q, Masubuchi T, et al. Multiple Signaling Roles of CD3ε and Its Application in CAR-T Cell Therapy. Cell 2020;182:855-871.e23. [Crossref] [PubMed]
- Whisler RL, Karanfilov CI, Newhouse YG, et al. Phosphorylation and coupling of zeta-chains to activated T-cell receptor (TCR)/CD3 complexes from peripheral blood T-cells of elderly humans. Mech Ageing Dev 1998;105:115-35. [Crossref] [PubMed]
- Han B, Duong D, Sul JH, et al. A general framework for meta-analyzing dependent studies with overlapping subjects in association mapping. Hum Mol Genet 2016;25:1857-66. [Crossref] [PubMed]
- LeBlanc M, Zuber V, Thompson WK, et al. A correction for sample overlap in genome-wide association studies in a polygenic pleiotropy-informed framework. BMC Genomics 2018;19:494.
- Rashkin SR, Graff RE, Kachuri L, et al. Pan-cancer study detects genetic risk variants and shared genetic basis in two large cohorts. Nat Commun 2020;11:4423. [Crossref] [PubMed]
- Shibao CA, Joos K, Phillips JA 3rd, et al. Familial Autonomic Ganglionopathy Caused by Rare CHRNA3 Genetic Variants. Neurology 2021;97:e145-55. [Crossref] [PubMed]
- Lassi G, Taylor AE, Timpson NJ, et al. The CHRNA5-A3-B4 Gene Cluster and Smoking: From Discovery to Therapeutics. Trends Neurosci 2016;39:851-61. [Crossref] [PubMed]
- Shea HC, Head DD, Setchell KD, et al. Analysis of HSD3B7 knockout mice reveals that a 3alpha-hydroxyl stereochemistry is required for bile acid function. Proc Natl Acad Sci U S A 2007;104:11526-33. [Crossref] [PubMed]
- Yi T, Wang X, Kelly LM, et al. Oxysterol gradient generation by lymphoid stromal cells guides activated B cell movement during humoral responses. Immunity 2012;37:535-48. [Crossref] [PubMed]
- Kim E, Ilagan JO, Liang Y, et al. SRSF2 Mutations Contribute to Myelodysplasia by Mutant-Specific Effects on Exon Recognition. Cancer Cell 2015;27:617-30. [Crossref] [PubMed]
- Cabrera T, López-Nevot MA, Gaforio JJ, et al. Analysis of HLA expression in human tumor tissues. Cancer Immunol Immunother 2003;52:1-9.
- Lawrence MS, Stojanov P, Mermel CH, et al. Discovery and saturation analysis of cancer genes across 21 tumour types. Nature 2014;505:495-501. [Crossref] [PubMed]
- Shukla SA, Rooney MS, Rajasagi M, et al. Comprehensive analysis of cancer-associated somatic mutations in class I HLA genes. Nat Biotechnol 2015;33:1152-8. [Crossref] [PubMed]
- García Sanz MT, González Barcala FJ, Alvarez Dobaño JM, et al. Asthma and risk of lung cancer. Clin Transl Oncol 2011;13:728-30. [Crossref] [PubMed]
- Jiang L, Sun YQ, Langhammer A, et al. Asthma and asthma symptom control in relation to incidence of lung cancer in the HUNT study. Sci Rep 2021;11:4539. [Crossref] [PubMed]
- Ben-Eghan C, Sun R, Hleap JS, et al. Don't ignore genetic data from minority populations. Nature 2020;585:184-6. [Crossref] [PubMed]