Development of a haematological indices-based nomogram for prognostic prediction and immunotherapy response assessment in primary pulmonary lymphoepithelioma-like carcinoma patients
Introduction
Primary pulmonary lymphoepithelioma-like carcinoma (PPLELC) is a rare and distinct pathological subtype of lung malignancies characterized by significant lymphocyte infiltration (1,2), and in the World Health Organization (WHO) 2021 Classification of Lung Tumors, it has been re-categorized from unclassified carcinomas to the subgroup of squamous cell carcinomas (3). The incidence of PPLELC is approximately 0.8% among non-small cell lung cancer (NSCLC) cases in Southeast Asia, but it remains exceedingly rare in most other parts of the world (4). PPLELC predominantly affects younger and nonsmoking females and is strongly associated with Epstein-Barr virus (EBV) infection (5,6). Comprehensive clinical data on PPLELC are limited due to the small retrospective cohorts from which outcomes have been reported, and standardized treatment regimens remain lacking. In clinical practice, the treatment approach for PPLELC is often based on experiences gained from managing lung squamous cell carcinomas (7). Patients diagnosed at early stages are amenable to surgical resection, which can lead to long-term remissions. In contrast, advanced and recurrent cases are typically managed with chemotherapy, radiotherapy, or a combination of these modalities (8). Additionally, it is noteworthy that despite initial positive responses to platinum-based chemotherapy in most cases, relapse occurs within 6 to 12 months (7). Given the unmet clinical need in the management of PPLELC, numerous novel therapies are currently under investigation.
The advent of immune checkpoint inhibitors (ICIs) has significantly improved the survival rates of patients across various malignancies and has become a mainstay in the management of advanced lung cancer (9,10). Previous studies have revealed an upregulation of programmed cell death-ligand 1 (PD-L1) expression in the tumor tissue of patients with PPLELC (1). Consequently, immunotherapy emerges as a potent treatment strategy for this disease. Notably, Zhou et al. (11) have demonstrated the significant therapeutic efficacy of PD-1/PD-L1 inhibitors in PPLELC patients. Additionally, findings from Pang et al. (12) have further demonstrated that patients receiving first-line chemoimmunotherapy experience a longer progression-free survival (PFS) compared to those undergoing traditional chemotherapy alone. Nevertheless, it is crucial to recognize that not all PPLELC patients respond positively to immunotherapy, and some may develop resistance after experiencing initial benefits, primarily due to the tumor’s inherent heterogeneity. Consequently, there exists an urgent need to develop robust and non-invasive prognostic tools. These tools have the potential to refine patient selection criteria and provide valuable guidance to oncologists in the realm of treatment decision-making.
In this study, we introduce a novel prognostic approach known as the haematological indices-based signature (HIBS) derived from a comprehensive analysis of routine hematological features using the least absolute shrinkage and selection operator (LASSO) Cox regression with the primary objective to enhance the accuracy of prognostic predictions in patients with PPLELC. We further present a clinically oriented nomogram that incorporates HIBS with relevant clinical parameters and serves as a predictive tool for estimating the PFS of PPLELC patients undergoing ICI therapy. To our knowledge, this study represents the first and the largest set to develop a practical tool for predicting the clinical outcomes of PPLELC patients with ICI. We present this article in accordance with the TRIPOD reporting checklist (available at https://tlcr.amegroups.com/article/view/10.21037/tlcr-23-813/rc).
Methods
Patients and study design
This is a retrospective study conducted on consecutive patients with histologically confirmed PPLELC who received at least one cycle of immunotherapy, irrespective of treatment line, at the Sun Yat-sen University Cancer Center (SYSUCC; Guangzhou, China) between May 2017 and August 2022. Patients were excluded if they had lung metastasis originating from nasopharyngeal cancer or lymphoepithelioma-like carcinoma of other primary sites, prior or synchronous malignant tumors, or incomplete clinical follow-up data. A total of 117 patients were randomly divided into a training cohort (n=82) and a validation cohort (n=35) in a 7:3 ratio. Written informed consent was obtained from all patients, and the study was approved by the Institute Research Ethics Committee of Sun Yat-Sen University Cancer Center (No. SL-B2023-507-01). The study was conducted in accordance with the Declaration of Helsinki (as revised in 2013).
Data collection
Demographic, clinical, laboratory and treatment data were extracted from the digital medical database of SYSUCC, which included baseline clinical characteristics such as age, gender, smoking history, Eastern Cooperative Oncology Group (ECOG) performance status, tumor location, tumor staging according to the eighth edition of the TNM classification, presence of liver, bone, or brain metastases, therapeutic regimens, and follow-up data. Baseline hematological tests, which encompassed serum biochemical parameters and routine blood count parameters, were conducted before the initiation of immunotherapy. A detailed list of these baseline hematological tests is provided in Appendix 1.
Treatment and outcome
All eligible patients received ICI therapy as their primary treatment, either as monotherapy or in combination with chemotherapy. The immunotherapy drugs administered included camrelizumab, pembrolizumab, penpulimab, sintilimab, tislelizumab, toripalimab, nivolumab, atezolizumab, and durvalumab. Chemotherapy regimens comprised platinum-based doublet chemotherapy or paclitaxel. Patients underwent contrast-enhanced computed tomography (CT) scans at intervals of 6–8 weeks, both during and after the completion of systemic treatment, as part of the standard treatment protocol. Tumor response was assessed using the Response Evaluation Criteria in Solid Tumors version 1.1 (RECIST 1.1) criteria, with responses categorized as complete response (CR), partial response (PR), stable disease (SD), or progressive disease (PD). The primary study endpoint was PFS, defined as the duration from the initiation of anti-PD-1/PD-L1 treatment to the occurrence of objective tumor progression, death from any cause, or the last follow-up date (June 30, 2023) in cases where no progression had occurred and the patient remained alive. We also calculated PFS rates at 1 and 2 years. The “best overall response” referred to the most favorable response recorded from the initiation of ICI therapy until disease progression/recurrence.
Statistical analysis
Statistical analyses were performed using the R (version 4.2.1, Vienna, Austria) and SPSS software (version 16.0; IBM, Chicago, IL, USA). Eligible patients were categorized into a training or validation cohort randomly using the “caret” R package. LASSO Cox regression was performed with the “glmnet” R package, utilizing an L1 regularization penalty to shrink the coefficients of each feature to zero and employing a 10-fold cross-validation. HIBS was constructed by linearly combining the non-zero features and their respective LASSO regression coefficients among the 41 hematological biomarkers using data from the training cohort. An optimal cutoff value for HIBS score was determined by identifying the median value of HIBS, which was then used to classify patients into high and low HIBS groups in the training cohort. This same cutoff value was subsequently applied to the validation cohort for similar patient stratification. To assess the prognostic value of HIBS and clinical characteristics, forward-backward stepwise multivariable Cox regression was performed. A nomogram was developed for predicting the 1- and 2-year PFS in PPLELC patients, utilizing variables with a significance level of P<0.05. The “rms” R package was used to construct the nomogram, whose performance based on accuracy and calibration was evaluated in both the training and validation cohorts. Internal validation was conducted using the bootstrap method with 500 repetitions and the calibration curve. Total points for each patient were calculated based on the established nomogram, and patients were categorized into high and low-risk groups using the X-tile program. Survival curves were generated using the Kaplan-Meier method, and time-dependent receiver operating characteristic (ROC) curves at 24 months were computed using the “survival ROC” package in R to assess the accuracy of the nomogram score in predicting tumor progression at 2 years after immunotherapy. All statistical tests were two-sided, and significance was defined as P<0.05.
Results
Patient characteristics
Patient enrollment and the study design schematic are presented in Figure 1. A total of 117 patients diagnosed with PPLELC who underwent immunotherapy were included and randomly allocated into two groups: a training cohort (n=82) and a validation cohort (n=35). Their demographic and clinical characteristics are summarized in Table 1. The study population comprised 51 males and 66 females, with a median age of 56 years [interquartile range (IQR): 47–63 years]. Most patients (64.1%, 75/117) were diagnosed with stage IV disease. Of the 117 patients, 87 (74.4%) received ICI as their initial treatment, and 98 (83.8%) received combination therapy with ICIs. The median follow-up duration for the entire study population was 14 months (IQR: 7–20 months), and treatment failure was observed in 58 (49.6%) patients. Notably, there were no significant differences in clinical characteristics between the training and validation cohorts.
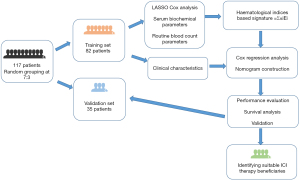
Table 1
Variable | Total (n=117) | Training cohort (n=82) | Validation cohort (n=35) |
---|---|---|---|
Age, median [IQR], years | 56 [47–63] | 55 [46–62] | 55 [49–63] |
Sex | |||
Male | 51 | 41 | 10 |
Female | 66 | 41 | 25 |
Smoking | |||
Never | 91 | 63 | 28 |
Prior or current | 26 | 19 | 7 |
ECOG | |||
0 | 73 | 52 | 21 |
1 | 44 | 30 | 14 |
Tumour location | |||
Left upper lobe | 21 | 14 | 7 |
Left lower lobe | 29 | 20 | 9 |
Right upper lobe | 15 | 10 | 5 |
Right middle lobe | 24 | 16 | 8 |
Right lower lobe | 28 | 22 | 6 |
TNM stage | |||
II | 9 | 6 | 3 |
III | 33 | 24 | 9 |
IV | 75 | 52 | 23 |
Liver metastasis | |||
Yes | 20 | 12 | 8 |
No | 97 | 70 | 27 |
Bone metastasis | |||
Yes | 26 | 21 | 5 |
No | 91 | 61 | 30 |
Brain metastasis | |||
Yes | 3 | 2 | 1 |
No | 114 | 80 | 34 |
Therapy | |||
ICI monotherapy | 19 | 12 | 7 |
ICI combination therapy | 98 | 70 | 28 |
Treatment line | |||
First-line | 87 | 65 | 22 |
Second-line | 25 | 14 | 11 |
Subsequent line | 5 | 3 | 2 |
Objective tumor progression | |||
Yes | 58 | 42 | 16 |
No | 59 | 40 | 19 |
IQR, interquartile range; ECOG, Eastern Cooperative Oncology Group; TNM, tumor node metastasis; ICI, immune checkpoint inhibitor.
Construction of the HIBS
The Cox regression model with LASSO was used to select relevant features from the initial set of 41 hematological biomarkers. Parameter selection for constructing HIBS was achieved through 10-fold cross-validation (Figure 2A,2B), and the final HIBS, which comprises 14 hematological features, is detailed in Appendix 2. An optimal cutoff value, determined by the median risk score, was set at 0.64 for the training cohort. Consequently, both the training and validation cohorts were divided into high-HIBS and low-HIBS groups based on this threshold. Figure 2C displays the distributions of HIBS scores, PFS, tumor progression status and the expression patterns of selected hematological features. Notably, patients with advanced disease exhibited significantly higher HIBS scores compared to those with non-advanced disease (P<0.001, Figure 2D).
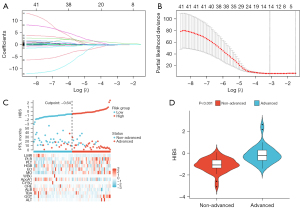
Development and validation of a HIBS-based nomogram
In the univariate Cox regression analysis conducted in the training cohort (Table 2), several factors were found to be significantly associated with PFS, including HIBS (P<0.001), gender (P=0.012), TNM stage (P=0.019), presence of liver metastasis (P=0.016), bone metastasis (P=0.006), type of therapy (P=0.019), and treatment line (P=0.028). Subsequently, a backward stepwise multivariate Cox regression analysis was performed and revealed HIBS, TNM stage, presence of bone metastasis and type of therapy as independent predictors of PFS. A nomogram was then constructed based on these independent risk factors to predict the 1- and 2-year PFS of patients with PPLELC (Figure 3A), in which each variable is assigned points along the vertical axis, with a higher total score indicating a shorter PFS. High-HIBS corresponds to 100 points, the presence of bone metastasis corresponds to 45 points, ICI monotherapy corresponds to 57 points, TNM stage III corresponds to 10 points, and TNM stage IV corresponds to 72 points. The total points are calculated by summing these corresponding points. The discrimination ability of the HIBS-based nomogram was assessed using the Concordance Index (C-index), which demonstrated good performance with C-index values of 0.766 and 0.765 in the training and validation cohorts, respectively. For internal validation, calibration plots displayed strong agreement between the nomogram-predicted PFS and the actual probability of PFS at both 1- and 2-year intervals (Figure 3B,3C).
Table 2
Characteristics | Univariate analysis | Multivariate analysis | |||
---|---|---|---|---|---|
HR (95% CI) | P | HR (95% CI) | P | ||
Age (years) | |||||
≤60 vs. >60 | 0.688 (0.329–1.439) | 0.321 | |||
Sex | |||||
Male vs. female | 0.443 (0.235–0.837) | 0.012 | 0.588 (0.301–1.148) | 0.12 | |
Smoking | |||||
Never vs. prior or current | 1.772 (0.905–3.470) | 0.095 | |||
ECOG | |||||
0 vs. 1 | 1.216 (0.659–2.244) | 0.532 | |||
Tumour location | |||||
Left upper lobe | Reference | 0.073 | |||
Left lower lobe | 0.623 (0.207–1.868) | 0.398 | |||
Right upper lobe | 1.788 (0.597–5.358) | 0.299 | |||
Right middle lobe | 0.902 (0.299–2.902) | 0.902 | |||
Right lower lobe | 0.143 (0.788–5.197) | 0.143 | |||
TNM stage | |||||
II vs. III vs. IV | 2.147 (1.134–4.064) | 0.019 | 2.146 (1.099–4.193) | 0.025 | |
Liver metastasis | |||||
Yes vs. no | 2.428 (1.182–4.987) | 0.016 | 1.466 (0.629–3.417) | 0.376 | |
Bone metastasis | |||||
Yes vs. no | 2.416 (1.292–4.516) | 0.006 | 2.048 (1.033–4.060) | 0.04 | |
Brain metastasis | |||||
Yes vs. no | 0.047 (0.000–71.069) | 0.412 | |||
Therapy | |||||
ICI monotherapy vs. ICI combination therapy | 0.410 (0.195–0.865) | 0.019 | 0.386 (0.177–0.838) | 0.016 | |
Treatment line | |||||
First-line | Reference | 0.028 | Reference | 0.311 | |
Second-line | 1.465 (0.694–3.094) | 0.317 | 0.784 (0.291–2.112) | 0.63 | |
Subsequent line | 4.907 (1.478–16.299) | 0.009 | 2.441 (0.611–9.748) | 0.206 | |
HIBS | |||||
Low vs. high | 4.460 (2.221–8.955) | <0.001 | 4.740 (2.309–9.733) | <0.001 |
HIBS, haematological indices-based signature; HR, hazard ratio; CI, confidence interval; ECOG, Eastern Cooperative Oncology Group; TNM, tumor node metastasis; ICI, immune checkpoint inhibitor.
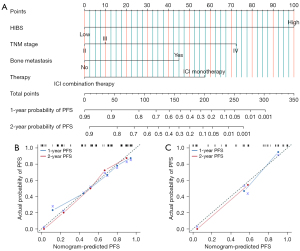
Risk stratification of PFS and predicts immunotherapy response
Based on the total points obtained from the nomogram and the corresponding PFS estimates, both the training and validation cohorts were stratified into two risk groups: a low-risk group (≤167 points) and a high-risk group (>167 points). The optimum prognostic cutoff values, which were determined based on the highest standardized log-rank statistic, were used for this classification. The nomogram exhibited satisfactory ability to stratify PPLELC patients who underwent immunotherapy. In the training cohort, the median PFS (interquartile range) for the low-risk group was 18.0 (11.0–24.0) months, whereas for the high-risk group, it was 7.0 (5.0–13.0) months (P<0.0001, Figure 4A). Furthermore, the time-dependent ROC curve analysis for the nomogram’s predictive performance at 2- and 1-year PFS in the training cohort resulted in an AUROC of 0.837 and 0.771, respectively (Figure 4B). Lastly, we assessed the relationship between the nomogram and the best overall response (BOR) to immunotherapy in PPLELC patients, which showed that in the training cohort, patients in the low-risk group displayed significantly higher BOR rates compared to the high-risk group (52.7% vs. 22.2%, Figure 4C). Similarly, in the validation cohort, the low-risk group had a significantly longer median PFS of 16.5 (12.8–20.5) months compared to the high-risk group, which had a median PFS of 11.0 (4.0–17.0) months (P=0.0002, Figure 4D). Moreover, the AUROC values for 2- and 1-year PFS were 0.855 and 0.828, respectively (Figure 4E). And the low-risk group had a significantly higher BOR rate (59.1% vs. 23.1%) than the high-risk group (Figure 4F) in the validation cohort. These findings highlight the clinical utility of the proposed HIBS-based nomogram for effectively predicting immunotherapy response in PPLELC patients.
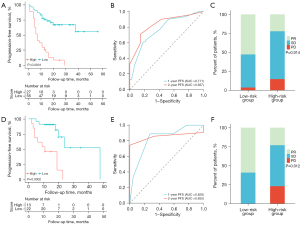
Discussion
Accurate prognosis prediction and assessing the benefits of ICI therapy are crucial for managing PPLELC patients. In this study, we developed an HIBS-based nomogram to predict patient prognosis during ICI therapy. The nomogram effectively stratified patients into high- and low-risk groups and could also predict their immunotherapy efficacy and PFS. Patients in the Low-risk group had a BOR rate of 52.7%, and the median PFS was extended to 18.0 months. In contrast, the High-risk group had a BOR rate of 22.2% and a median PFS of 7.0 months. These results suggest that patients in the Low-risk group could be more likely to benefit from immunotherapy. Overall, our study provides valuable insights for future research in precision medicine for PPLELC, aiding in tailored patient management.
Prior investigations have highlighted that classical driver gene mutations, such as epidermal growth factor receptor (EGFR) mutation and anaplastic lymphoma kinase (ALK) rearrangement, are rarely identified in PPLELC patient samples (13,14). Despite the low tumor mutation burden, approximately 70% of PPLELC patients exhibit positive expression of PD-L1 in their tumor cells, a proportion higher than observed in other histological subtypes of lung cancer (1,15). A retrospective study reported a median PFS of 6.9 months in the chemotherapy group, 11.0 months in the immunotherapy group, and 11.8 months in the chemoimmunotherapy group when used as front-line treatments for advanced PPLELC (16). Recently, two studies have also indicated that patients treated with chemoimmunotherapy had significantly greater survival benefits compared to those receiving chemotherapy alone (8,11). Our study’s results align with and extend these previous findings, demonstrating that ICI combination therapy is an independent predictor for PFS and offers superior clinical benefits compared to ICI monotherapy in the context of PPLELC. These results provide a strong rationale for considering first-line combination chemotherapy and immunotherapy as a treatment approach for PPLELC.
In recent years, there has been growing interest in utilizing routine laboratory tests, including both routine blood and biochemical assessments, as clinical tools to predict clinical responses and outcomes to immunotherapies (17-20). For instance, Pang et al. observed that in PPLELC patients receiving first-line treatment with immunotherapy plus chemotherapy, those in the high monocyte-to-lymphocyte ratio (MLR) group had significantly shorter PFS compared to the MLR-low group (12). However, these factors have often been examined in relatively small patient cohorts and were not included in multivariate analyses. In this study, we employed a practical machine learning approach to manage high-dimensional data and select relevant hematological biomarkers for prognostic assessment in PPLELC patients undergoing immunotherapy, based on which the HIBS was constructed, comprising 14 factors that reflect aspects of tumor metabolism, systemic inflammation and nutritional status. These factors are known to correlate with tumor development, treatment response and survival outcomes (21-25). We observed that patients in the disease progression group exhibited significantly higher HIBS scores than those in the no-progression group. Importantly, even after multivariate adjustment for key prognostic clinical factors, HIBS remained an effective predictor of both prognosis and immunotherapy benefits for PPLELC patients.
The most common metastatic sites for primary lung cancers include the liver, bone and brain (26,27). Compared with other sites of metastases, our results revealed that the presence of bone metastases was associated with shorter PFS in PPLELC patients treated with immunotherapy. A recent retrospective study reported that bone metastasis could attenuate the efficacy of ICI therapy and is associated with “cold” immune characteristics. Patients without bone metastasis exhibited higher levels of CD3+ lymphocytes, CD8+ Tc cells, and CD68+ tumor-associated macrophages compared to those with bone metastasis (28). Moreover, bone metastatic cells release cytokines such as interleukin-6, tumor necrosis factor-alpha, vascular endothelial growth factor, and monocyte colony-stimulating factor, which promote osteoclast differentiation (29). Osteoclasts, in turn, secrete various pro-tumor growth factors, facilitating the seeding and proliferation of bone metastatic cells, ultimately resulting in a poorer prognosis (30). Several studies have suggested that combining ICI with chemotherapy, antiangiogenic agents or bisphosphonates may yield improved therapeutic outcomes for patients with bone metastases (28,31,32), which deserves both clinical attention and further investigations.
This study has some limitations that should be noted. Firstly, the inclusion of participants from a single center may introduce selection biases. Secondly, due to the limited availability of tumor molecular biomarker data for PPLELC patients, we could not include these biomarkers in the nomogram. Lastly, the follow-up duration was relatively short, and longer-term follow-up is needed to assess outcomes more comprehensively. Despite these limitations, the HIBS-based nomogram presented in this study provides valuable insights into the prognosis and therapeutic effects of immunotherapy in PPLELC patients. We expect that this nomogram could be used as a reference for further prospective research and multi-center clinical investigations involving diverse patient populations to enhance and validate its clinical utility for PPLELC patients.
Conclusions
This study introduces a clinically oriented nomogram showing promising ability in accurately predicting the prognosis of PPLELC patients receiving checkpoint inhibitor immunotherapy and identifying those likely to benefit from this treatment. Our findings offer clinicians a valuable referential tool for informed decision-making in the clinical management of PPLELC patients.
Acknowledgments
Funding: This work was supported by
Footnote
Reporting Checklist: The authors have completed the TRIPOD reporting checklist. Available at https://tlcr.amegroups.com/article/view/10.21037/tlcr-23-813/rc
Data Sharing Statement: The authenticity of this article has been validated by uploading the key raw data onto the Research Data Deposit public platform (www.researchdata.org.cn), with the approval RDD number as RDDA2024839326. Available at https://tlcr.amegroups.com/article/view/10.21037/tlcr-23-813/dss
Peer Review File: Available at https://tlcr.amegroups.com/article/view/10.21037/tlcr-23-813/prf
Conflicts of Interest: All authors have completed the ICMJE uniform disclosure form (available at https://tlcr.amegroups.com/article/view/10.21037/tlcr-23-813/coif). The authors have no conflicts of interest to declare.
Ethical Statement: The authors are accountable for all aspects of the work in ensuring that questions related to the accuracy or integrity of any part of the work are appropriately investigated and resolved. The study was conducted in accordance with the Declaration of Helsinki (as revised in 2013). The Institute Research Ethics Committee of Sun Yat-Sen University Cancer Center approved the study (No. SL-B2023-507-01). The authenticity of this article has been validated by uploading the key raw data onto the Research Data Deposit public platform (www.researchdata.org.cn), with the approval RDD number as RDDA2024839326. Written informed consent was obtained from all patients.
Open Access Statement: This is an Open Access article distributed in accordance with the Creative Commons Attribution-NonCommercial-NoDerivs 4.0 International License (CC BY-NC-ND 4.0), which permits the non-commercial replication and distribution of the article with the strict proviso that no changes or edits are made and the original work is properly cited (including links to both the formal publication through the relevant DOI and the license). See: https://creativecommons.org/licenses/by-nc-nd/4.0/.
References
- Fan Y, Li C, Qin J, et al. Primary pulmonary lymphoepithelioma-like carcinoma. Med Oncol 2020;37:20. [Crossref] [PubMed]
- Liang Y, Wang L, Zhu Y, et al. Primary pulmonary lymphoepithelioma-like carcinoma: fifty-two patients with long-term follow-up. Cancer 2012;118:4748-58. [Crossref] [PubMed]
- Nicholson AG, Tsao MS, Beasley MB, et al. The 2021 WHO Classification of Lung Tumors: Impact of Advances Since 2015. J Thorac Oncol 2022;17:362-87. [Crossref] [PubMed]
- Ho JC, Wong MP, Lam WK. Lymphoepithelioma-like carcinoma of the lung. Respirology 2006;11:539-45. [Crossref] [PubMed]
- He J, Shen J, Pan H, et al. Pulmonary lymphoepithelioma-like carcinoma: a Surveillance, Epidemiology, and End Results database analysis. J Thorac Dis 2015;7:2330-8. [Crossref] [PubMed]
- Li L, Ma BBY, Chan ATC, et al. Epstein-Barr Virus-Induced Epigenetic Pathogenesis of Viral-Associated Lymphoepithelioma-Like Carcinomas and Natural Killer/T-Cell Lymphomas. Pathogens 2018;7:63. [Crossref] [PubMed]
- Lin Z, Fu S, Zhou Y, et al. First-line platinum-based chemotherapy and survival outcomes in locally advanced or metastatic pulmonary lymphoepithelioma-like carcinoma. Lung Cancer 2019;137:100-7. [Crossref] [PubMed]
- Zhang X, Zhou Y, Chen H, et al. PD-1 inhibition plus platinum-based chemotherapy (PBC) or PBC alone in the first-line treatment of locally advanced or metastatic pulmonary lymphoepithelioma-like carcinoma. Front Immunol 2022;13:1015444. [Crossref] [PubMed]
- He J, Hu Q. Progress in the clinical application of immune checkpoint inhibitors in small cell lung cancer. Front Immunol 2023;14:1126582. [Crossref] [PubMed]
- Gan J, Huang Y, Fang W, et al. Research progress in immune checkpoint inhibitors for lung cancer in China. Ther Adv Med Oncol. 2021;13:17588359211029826. [Crossref] [PubMed]
- Zhou N, Tang H, Yu S, et al. Anti-PD-1 antibodies, a novel treatment option for advanced chemoresistant pulmonary lymphoepithelioma carcinoma. Front Immunol 2022;13:1001414. [Crossref] [PubMed]
- Pang LL, Liao J, Huang YH, et al. Exploration of immunotherapy in advanced pulmonary lymphoepithelioma-like carcinoma. Int J Cancer 2023;152:2338-50. [Crossref] [PubMed]
- Yin K, Feng HB, Li LL, et al. Low frequency of mutation of epidermal growth factor receptor (EGFR) and arrangement of anaplastic lymphoma kinase (ALK) in primary pulmonary lymphoepithelioma-like carcinoma. Thorac Cancer 2020;11:346-52. [Crossref] [PubMed]
- Chang YL, Wu CT, Shih JY, et al. Unique p53 and epidermal growth factor receptor gene mutation status in 46 pulmonary lymphoepithelioma-like carcinomas. Cancer Sci 2011;102:282-7. [Crossref] [PubMed]
- Chen B, Zhang Y, Dai S, et al. Molecular characteristics of primary pulmonary lymphoepithelioma-like carcinoma based on integrated genomic analyses. Signal Transduct Target Ther 2021;6:6. [Crossref] [PubMed]
- Xiao Y, He J, Luo S, et al. Comparison of Immunotherapy, Chemotherapy, and Chemoimmunotherapy in Advanced Pulmonary Lymphoepithelioma-Like Carcinoma: A Retrospective Study. Front Oncol 2022;12:820302. [Crossref] [PubMed]
- Huang Y, Zhao JJ, Soon YY, et al. Factors Predictive of Primary Resistance to Immune Checkpoint Inhibitors in Patients with Advanced Non-Small Cell Lung Cancer. Cancers (Basel) 2023;15:2733. [Crossref] [PubMed]
- Sung M, Jang WS, Kim HR, et al. Prognostic value of baseline and early treatment response of neutrophil-lymphocyte ratio, C-reactive protein, and lactate dehydrogenase in non-small cell lung cancer patients undergoing immunotherapy. Transl Lung Cancer Res 2023;12:1506-16. [Crossref] [PubMed]
- Klümper N, Saal J, Berner F, et al. C reactive protein flare predicts response to checkpoint inhibitor treatment in non-small cell lung cancer. J Immunother Cancer 2022;10:e004024. [Crossref] [PubMed]
- Peng L, Wang Y, Liu F, et al. Peripheral blood markers predictive of outcome and immune-related adverse events in advanced non-small cell lung cancer treated with PD-1 inhibitors. Cancer Immunol Immunother 2020;69:1813-22. [Crossref] [PubMed]
- Hanahan D, Weinberg RA. Hallmarks of cancer: the next generation. Cell 2011;144:646-74. [Crossref] [PubMed]
- Prelaj A, Rebuzzi SE, Pizzutilo P, et al. EPSILoN: A Prognostic Score Using Clinical and Blood Biomarkers in Advanced Non-Small-cell Lung Cancer Treated With Immunotherapy. Clin Lung Cancer 2020;21:365-377.e5. [Crossref] [PubMed]
- Xie E, Yeo YH, Scheiner B, et al. Immune Checkpoint Inhibitors for Child-Pugh Class B Advanced Hepatocellular Carcinoma: A Systematic Review and Meta-Analysis. JAMA Oncol 2023;9:1423-31. [Crossref] [PubMed]
- Gao S, Xie H, Wei L, et al. Serum creatinine/cystatin C ratio as a prognostic indicator for patients with colorectal cancer. Front Oncol 2023;13:1155520. [Crossref] [PubMed]
- Sakai A, Iijima H, Ebisumoto K, et al. Prognostic Value of Inflammatory and Nutritional Biomarkers of Immune Checkpoint Inhibitor Treatment for Recurrent or Metastatic Squamous Cell Carcinoma of the Head and Neck. Cancers (Basel) 2023;15:2021. [Crossref] [PubMed]
- Wan L, Pantel K, Kang Y. Tumor metastasis: moving new biological insights into the clinic. Nat Med 2013;19:1450-64. [Crossref] [PubMed]
- Zheng XQ, Huang JF, Lin JL, et al. Incidence, prognostic factors, and a nomogram of lung cancer with bone metastasis at initial diagnosis: a population-based study. Transl Lung Cancer Res 2019;8:367-79. [Crossref] [PubMed]
- Zhu YJ, Chang XS, Zhou R, et al. Bone metastasis attenuates efficacy of immune checkpoint inhibitors and displays "cold" immune characteristics in Non-small cell lung cancer. Lung Cancer 2022;166:189-96. [Crossref] [PubMed]
- Xiang L, Gilkes DM. The Contribution of the Immune System in Bone Metastasis Pathogenesis. Int J Mol Sci 2019;20:999. [Crossref] [PubMed]
- Guise TA. The vicious cycle of bone metastases. J Musculoskelet Neuronal Interact 2002;2:570-2.
- Kimachi K, Kajiya H, Nakayama S, et al. Zoledronic acid inhibits RANK expression and migration of osteoclast precursors during osteoclastogenesis. Naunyn Schmiedebergs Arch Pharmacol 2011;383:297-308. [Crossref] [PubMed]
- Manegold C, Dingemans AC, Gray JE, et al. The Potential of Combined Immunotherapy and Antiangiogenesis for the Synergistic Treatment of Advanced NSCLC. J Thorac Oncol 2017;12:194-207. [Crossref] [PubMed]