Development and validation of a deep learning-based model to predict response and survival of T790M mutant non-small cell lung cancer patients in early clinical phase trials using electronic medical record and pharmacokinetic data
Highlight box
Key findings
• In a study of 326 T790M mutant non-small cell lung cancer (NSCLC) patients taking epidermal growth factor receptor tyrosine kinase inhibitor (EGFR-TKI), a deep-learning model, CoxMoE, effectively predicted response and progression-free survival (PFS) in early-clinical trials. There was a 40% improvement in PFS in low-risk patients, offering a strategy for effective patient selection for EGFR-TKI therapy.
What is known and what is new?
• Not all patients with EGFR mutations respond to this therapy, and even responsive patients may develop resistance.
• Our findings offer a viable strategy for patient selection in early-phase clinical trials and help identify those who are more likely to benefit from third-generation EGFR-TKI therapy.
What is the implication, and what should change now?
• CoxMoE can complement current EGFR-genotype detection by non-invasively predicting third-generation EGFR-TKI efficacy in T790M-mutated NSCLC patients. The predictors include routine laboratory tests and pharmacokinetic parameters. This strategy changes the landscape of patient selection in early-phase clinical trials and helps identify those who can benefit the most from third-gen EGFR-TKI therapy.
Introduction
The advent of epidermal growth factor receptor tyrosine kinase inhibitor (EGFR-TKI) has revolutionized the treatment of non-small cell lung cancer (NSCLC) to a great extent (1). EGFR genotype detection is the most common method to identify patients sensitive to EGFR-TKI in clinical practice and clinical trials of novel EGFR-TKIs. Previous studies have demonstrated that third-generation EGFR-TKI can effectively overcome the acquired TKI resistance led by secondary T790M mutation, and there are a total of 24 third-generation EGFR-TKIs being developed globally, with approximately half of the drugs in the early stages of clinical trials, such as YZJ-0318 and TQB3456. Whereas around 30% of patients with T790M mutation may fail to respond to third-generation EGFR-TKI (2,3), based on our experience, it might be even higher in clinical trials of EGFR-TKI. Meanwhile, EGFR-sensitive patients inevitably develop drug resistance, suggesting EGFR testing alone is insufficient (4,5). Due to tumor heterogeneity and difficulties in obtaining tissue from advanced-stage patients, non-invasive biomarkers that could stratify NSCLC patients with a specific EGFR mutation are needed to aid in targeted therapy administration.
Presently, EGFR genotype detection of tumor tissues is considered as the gold standard for EGFR-TKIs treatment in NSCLC. While not all EGFR-mutant NSCLC patients respond to EGFR-TKI therapy, complete responses (CRs) are rare. Moreover, EGFR-sensitive patients inevitably develop drug resistance, suggesting EGFR testing alone is not enough. Efforts have been made to develop new approaches for predicting efficacy and prognosis stratification. Currently, the primary tool monitoring EGFR-TKI future risk is computed tomography (CT), which exhibits tumor features in CT imaging non-invasively. AI combined with CT has shown potential for predicting EGFR-TKI responses and optimizing treatment decisions. For example, previous studies have proposed a fully automated artificial intelligence system (FAIS) that mines lung information from CT images focusing on EGFR mutation status prediction to identify patients sensitive to EGFR-TKI (6-9). These results illustrate that constructing a machine-learning model based on clinical data has great potential in predicting the efficacy of EGFR-TKI.
More recently, there has been a groundswell of interest in using artificial intelligence and laboratory values obtained from electronic medical record (EMR) data to develop risk models for disease diagnosis and prognosis prediction. For instance, a previous study developed a modified version of the well-validated 2012 Prostate, Lung, Colorectal and Ovarian Cancer Screening Trial risk model (mPLCOm2012) using Extreme Gradient Boosting (XGBoost) algorithm based mainly on routine laboratory test data. MPLCOm2012 was designed to diagnose NSCLC, and the performance of mPLCOm2012 was evaluated in 6,505 NSCLC patients and 189,597 control subjects with an area under the curve (AUC) of 0.79 and a sensitivity of 27.9% at a specificity of 95% (10). Furthermore, a gradient-boosted decision tree (GBDT) model incorporating patient demographic features (age, sex, race) with 27 routine laboratory tests to predict an individual’s severe acute respiratory syndrome coronavirus 2 (SARS-CoV-2) infection status with AUCs of 0.838–0.854 (11). Moreover, EMR has been commonly and economically used as inclusion and exclusion criteria in clinical trials.
In contrast, the majority of clinical laboratory tests utilize established reference values for defining thresholds, which may not always be suitable for a particular study for being either too strict or too permissive. EMR itself usually cannot fully identify patients’ responses to the drug, while sophisticated analytics methods could assist in making full use of the EMR data to identify high-risk patients’ subsets, probably with poor prognoses. For example, Trial Pathfinder, has been developed to associate EMR with survival hazard ratios (12). In addition, machine learning (ML) algorithms combined with EMR and genotype data are a potentially helpful tool for providing clinicians with early toxicity prediction in phase I clinical trials (13). Thus, leveraging AI algorithms to analyze EMR data in early clinical trials might hold great potential for diagnosing disease and predicting efficacy and toxicity, aiding in patient selection and improving the success rates of clinical trials.
Since mixture-of-expert (MoE) contains a neural network, similar feature input will yield a similar output. Therefore, more similar samples were assigned to the same expert model to realize the data’s automatic grouping and clustering. To some extent, this property aligns with our perception of the real world. For example, men and women have different prognostic patterns in certain diseases. We proposed CoxMoE based on a MoE multimodal deep generative model to mitigate such clinical challenges by incorporating EMR and pharmacokinetic data. Investigating T790M-mutated NSCLC patients from clinical trials of two third-generation EGFR-TKIs, namely abivertinib and BPI-7711, we focused on predicting the therapeutic response and progression-free survival (PFS)-based on the baseline EMR data ahead of treatment. This non-invasive prognostic system performs better than traditional ML methods and could complement EGFR genotype information in clinical practice. We present this article in accordance with the TRIPOD reporting checklist (available at https://tlcr.amegroups.com/article/view/10.21037/tlcr-23-737/rc).
Methods
Study design and participants
The workflow of this study is graphically summarized in Figure 1. Initially, we assembled 177 patients in abivertinib phase I clinical trial into a training cohort (n=177) and patients from phase II clinical trial of Abivertinib were used as validation cohort 1 (n=106). Forty-three patients were randomly selected from the BPI-7711 phase I clinical trial as validation cohort 2. The preprocessed single feature data of training cohort were fed into CoxNet, and the top 15 features were selected based on Concordance index (C-index). Then, these 15 features were shrunk into 4 features based on C-index calculated by CoxSVM. Subsequently, we trained CoxMoE in the training cohort and computed the probability of patients being responder (R)/non-responder (NR) and the risk score of survival in two validation cohorts. We retrospectively included 326 advanced NSCLC patients with EGFR T790M mutation comprising 283 patients from third-generation EGFR-TKI Abivertinib clinical trials (Clinical Trials Registration ID: NCT02274337, NCT02330367) (14,15) in 16 hospitals (Table S1) from January 1, 2015, to March 15, 2019, and 43 patients from third-generation EGFR-TKI BPI-7711 clinical trials (Clinical Trials Registration ID: NCT03386955) (16,17) at 12 hospitals (Table S2) from September 11, 2017, to October 17, 2019. The primary efficacy endpoint was objective response rate (ORR), defined as the percentage of patients with CR or partial response (PR) according to Response Evaluation Criteria in Solid Tumors (RECIST) 1.1. We classified patients with CR or PR as R and patients with stable disease (SD) or disease progression (PD) as NR. The secondary endpoints included PFS and overall survival (OS) determined using RECIST 1.1 assessed by investigators. The dataset of focus included patients with an acquired T790M mutation after first-generation EGFR-TKI (including gefitinib, erlotinib, and icotinib) treatment or primary T790M mutation-positive patients. T790M status was conducted by a central laboratory from a tissue biopsy specimen or plasma samples using an amplification refractory mutation system (ARMS) (14,15) or the cobas EGFR mutation test (17).
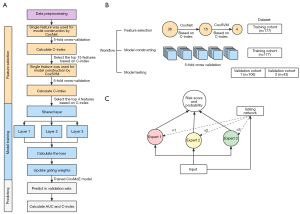
Candidate variables
We collected the baseline information of cases from the following categories: (I) demographic data (age, sex, body mass index, smoking status); (II) liver function [alanine aminotransferase (ALT), aspartate aminotransferase (AST), ALT/AST, alkaline phosphatase (ALP), lactate dehydrogenase (LDH), total protein (TP), albumin (ALB), total bilirubin (TBIL), apolipoprotein A, apolipoprotein B, total bile acid (TBA), γ-GT, urea nitrogen (UrBun), creatinine (Crea), uric acid (UA), glucose (Glu), potassiun (K), sodium (NA), chloride (CL), calcium (Ca), magnesium (Mg), creatinine clearance (Ccr), D-dimer, creatine kinase (CK)]; (III) coagulation function [prothrombin time (PT), activated partial thromboplastin time (APTT)]; (IV) blood routine test [haemoglobin (Hbc), erythrocyte (RBC), leucocyte (WBC), neutrophils (NeuA), lymphocyte (LymA), monocyte (MoA), platelet]; (V) urine test (uPH); (VI) pharmacokinetic indicators (Cssmax, Cssmin); (VII) best objective response (BoR) (CR, PR, SD, PD). Patients with CR or PR efficacy were categorized as R, whereas patients with SD or PD were defined as NR. (VIII) PFS time and outcome (Event). Among the features we collected, therapeutic response and PFS were employed as predictors of survival analysis, and the rest of the features were included in the modeling features.
Data preprocessing
We first discarded invalid samples with missing labels and removed features with missing values exceeding 30%. Missing “Event” was regarded as “censored” meaning no PD was observed during the follow-up period. For features with severely unbalanced multi-class categories, we simplified them into binary categories. Then, we discarded the binary features with the severely unbalanced distribution. The definition of each feature is shown in Table S3. We used the k-nearest neighbor (KNN) algorithm to impute the missing values on the numerical features and performed minimum and maximum normalizations calculated by the formula below. We converted classification features into one-hot encoding to prepare for algorithm execution.
Feature importance analysis
Reducing the number of features is crucial for enhancing the feasibility and interpretability of the model. Firstly, we employed the CoxNet and CoxSVM models to generate the single-feature C-index, thereby ascertaining the contribution of individual features toward the prediction task. Using the feature-feature correlation approach, we prioritized features with higher contributions during modeling and analyzed the interrelationships between different indicators. For feature pairs displaying high correlation, we selected the one with a higher task contribution instead of incorporating them simultaneously into the CoxMoE model. Subsequently, upon obtaining the model, we utilized the Shapley value to evaluate the contribution of each feature.
Model algorithms
Here, we developed a new algorithm, CoxMoE, based on the MoE system as shown in Figure 1C. We used the softmax function to represent the weight of the gated output as the weight of the result fusion. Suppose CoxMoE has a gated network and expert networks, which can be expressed as:
Furthermore, we integrated the therapeutic response and PFS risk score prediction tasks into deep-learning models to make multi-task-enabled models, which traditional machine-learning methods cannot fulfill. The negative log-likelihood (NLL) and cross-entropy function were used for loss calculation. While we adopted NLL from DeepSurv, we designed cross-entropy for therapeutic response prediction. We designed the objective function as follows:
where was NLL loss and was cross-entropy loss, was a constant that moderates the weight of cross-entropy loss ( in this study).
Model validation and evaluation
For model validation and evaluation, patients from the phase I abivertinib trial (n=177) were used for model training and were randomly divided into train and internal validation datasets at a ratio of 8:2. Phase II abivertinib trial patients (n=106) were assembled as the validation cohort 1 and 43 patients from phase I BPI-7711 trial were validation cohort 2. For therapeutic response prediction evaluation, we utilized accuracy and receiver operating characteristic (ROC)-AUC. We used the C-index for PFS prediction to estimate the probability that the predicted result is consistent with the actual observed result. The calculation strategy of the C-index is to randomly form pairs of all the research objects in the data.
To illustrate the advantage of the deep-learning model, we employed three survival analysis algorithms for comparison, containing two traditional machine-learning algorithms (CoxNet and CoxSVM) based on Cox and a deep-learning algorithm (DeepSurv) developed by our team. CoxNet is a model based on ElasticNet, an improved version of CoxPH, a linear regression model that uses L1 and L2 priors as regularization matrices (18,19), while CoxSVM is a nonlinear Cox model (20). DeepSurv is a deep-learning-based model utilizing a multilayer perceptron (MLP) to fit features and NLL function for loss calculation (21).
Statistical analysis
CoxNet and CoxSVM were implemented by the Python package sksurv. Data normalization was calculated by Python package scikit-learn, and the Shapley value was calculated by the Python package SHAP. Decision curve analysis (DCA) and clinical impact curves (CICs) analysis were conducted by R package rmda and the cutoff value for risk stratification was calculated by R package survminer. We applied Spearman correlation analysis to explore the association between four features and genetic mutations. Kyoto Encyclopedia of Genes and Genomes (KEGG) signaling pathway enrichment analyses were conducted using DAVID using the genetic mutations that were significantly associated (P≤0.05) with each feature. Then, unsupervised hierarchical clustering (Pearson correlation, average-linkage method) was performed on the correlation coefficients of Spearman correlation analysis. All analyses were performed by Python version 3.10.0 and R version 4.0.2.
Results
Data sources and characteristics
Three hundred twenty-six advanced NSCLC patients with EGFR mutations receiving third-generation EGFR-TKI therapy were included. One hundred seventy-seven patients from the phase I abivertinib trial were used for model training [age, 56±10 years; female 95 (53.6%)], which was randomly divided into a training and an internal validation dataset in an 8:2 ratio. Phase II abivertinib trial patients [n=106; age, 58±9 years; female 69 (65.0%)] were assembled as validation cohort 1. It was observed that the optimal sample size was 43 (Figure S1) to achieve 80% power with α=0.0005. Thus, we randomly selected 43 patients from BPI-7711 trial as validation cohort 2 [age, 59±10 years; female 31 (68.8%)]. The patients of training cohort were enrolled among seven hospitals, with an average of 25.29±29.70 samples in each hospital, of which hospital 1 had the largest number of samples (n=97), while hospital 8 had the least with only five samples (Table S1). The validation cohort 1 enrolled 106 samples distributed in 11 hospitals with a relatively uniform distribution of sample numbers similar to validation cohort 2 (3.92±3.37) (Table S2), where the average number of samples was 6.63, and the standard deviation was 5.60 (Table S1).
The median PFS of R patients was 8.89 (2.97–33.00) months for the training cohort, 7.48 (2.68–16.54) months for validation cohort 1, and 15.18 (4.14–27.40) months for validation cohort 2. The median PFS of NR patients was 3.00 (0.75–35.00) months for training cohort, 3.00 (1.04–13.50) months for validation cohort 1 and 4.16 (1.38–11.10) months for validation cohort 2. Compared with training and validation cohort 1, validation cohort 2 had a higher proportion of female patients; other characteristics were comparable among the three datasets (Table 1).
Table 1
Variables | Training cohort | Validation cohort 1 | Validation cohort 2 | |||||
---|---|---|---|---|---|---|---|---|
R (n=51) | NR (n=126) | R (n=58) | NR (n=48) | R (n=35) | NR (n=9) | |||
Gender, n (%) | ||||||||
Female | 28 (54.9) | 67 (53.2) | 42 (72.4) | 27 (56.2) | 26 (74.3) | 5 (55.6) | ||
Male | 23 (45.1) | 59 (46.8) | 16 (27.6) | 21(43.8) | 9 (25.7) | 4 (44.4) | ||
Age, n (%) | ||||||||
≤60 years | 39 (76.5) | 76 (60.3) | 35 (60.3) | 31 (64.6) | 19 (54.3) | 5 (55.6) | ||
>60 years | 12 (23.5) | 50 (39.7) | 23 (39.7) | 17 (35.4) | 16 (45.7) | 4 (44.4) | ||
EGFR variant, n (%) | ||||||||
EGFR 19Del | 20 (39.2) | 52 (41.2) | 48 (82.7) | 27 (56.2) | 20 (57.1) | 6 (66.7) | ||
EGFR 21L858R | 15 (29.4) | 39 (30.9) | 10 (17.2) | 21 (43.7) | 13 (37.1) | 3 (33.3) | ||
Other | 16 (31.4) | 35 (27.9) | – | – | 2 (5.8) | – | ||
T790M, n (%) | ||||||||
Positive | 51 (100.0) | 126 (100.0) | 58 (100.0) | 48 (100.0) | 23 (65.7) | 9 (100.0) | ||
Negative | 0 (0.0) | 0 (0.0) | 0 (0.0) | 0 (0.0) | 12 (34.3) | 0 (0.0) | ||
Smoke (yes), n | 15 | 38 | 12 | 17 | NA | NA | ||
mPFS, years (median) | 8.89 | 3.00 | 7.48 | 3.00 | 15.18 | 4.16 |
R, responder; NR, non-responder; mPFS, median progression-free survival.
Feature selection
After data preprocessing of removing features containing more than 30% missing values and feature merging, a total of 36 features remained (Table S3). In general, the process of feature selection contributes to the performance of the model by getting rid of noisy and redundant features. Here, by Pearson correlation analysis, three pairs of features [WBC vs. NeuA, Cssminvs. Cssmax, LDH vs. α-hydroxybutyrate dehydrogenase (α-HBDH)] showed a close correlation with correlation coefficients of more than 0.8 (Table S4), which will be fully considered for further feature selection. Firstly, we calculated the C-index of each simple feature in the training cohort by CoxNet analysis and then ranked the features by C-index (top 15 features: CL, APTT, NA, Ccr, CK, ALT/AST, K, ALP, LDH, uPH, LymA, Cssmin, Hbc, MoA, age) (Figure S2). Then, we applied a nonlinear method CoxSVM, to further validate the performance of the 15 parameters. The optimal-feature group with the highest C-index score was found, including four features, i.e., APTT, MoA, Ccr, and Cssmin. APTT is the most commonly used clinical indicator to reflect the coagulation activity of the endogenous coagulation system. In addition, APTT is used to detect endogenous coagulation factor defects and related inhibitors and activate protein C resistance. Cssmin means steady-state plasma concentrations at the trough, which always correlate with drug efficacy and adverse effects. It is vital to monitor drug concentration for new clinical trials or certain drugs such as antiarrhythmic.
CoxMoE performance in predicting therapeutic response and PFS
Deep-learning models are capable of predicting continuous and discrete variables simultaneously, which cannot be achieved by CoxNet and CoxSVM. As shown in Figure 2 and Table 2, CoxMoE had good performance in predicting therapeutic response with an averaged AUC of 0.832 [95% confidence interval (CI): 0.767–0.897] in the training cohort and achieved AUCs of 0.728 (95% CI: 0.591–0.864) and 0.732 (95% CI: 0.532–0.932) in validation cohort 1 and 2, respectively. For PFS prediction, CoxMoE achieved an averaged C-index of 0.65 in training cohort and reached 0.64 in validation cohort 1 and validation cohort 2 (Table 2). Furthermore, CoxMoE performed better than typical machine-learning models (CoxNet and CoxSVM) for survival analysis and another deep-learning model, DeepSurv (Tables S5,S6). We evaluated the performance of two machine-learning models (CoxNet and CoxSVM) and two deep-learning models (CoxMoE and DeepSurv). CoxMoE achieved the highest C-index in the training cohort with an averaged C-index score of 0.6761 for cross-validation (Table S5). CoxNet performed worst with an averaged C-index of 0.6443 (Table S5). As shown in Table S6, CoxMoE performed better than DeepSurv in predicting PFS (C-index for CoxMoE and DeepSurv reached 0.6732 and 0.6527, respectively) and efficacy (accuracy: 0.7714 and 0.7564, respectively; AUC: 0.8181 and 0.7814, respectively) for cross-validation. Based on the risk score calculated for the training cohort, we divided the Abivertinib trial cohort into high- and low-risk groups and the two groups exhibited significant distinct PFS (HR, 0.56; 95% CI, 0.40–0.78; P=0.0013) (Figure 3A). Using the same cutoff value, we stratified the BPI-7711 clinical trial cohort into high-risk [median, 11.0 (range, 1.4–25.1) months] and low-risk [median, 16.5 (range, 1.4–27.4) months] groups and significantly distinct PFS (HR, 0; 95% CI, 0–0; P=0.01) was also obtained between the two groups (Figure 3B). When we applied CoxMoE to select low-risk patients, the median PFS increased by 28% (HR, 0.66; 95% CI, 0.48–0.91; P=0.02) compared to the original whole cohort in Abivertinib treated cohort (Figure 3C). For BPI-7711, the median PFS increased by 50% (HR, 0; 95% CI, 0–0; P=0.02) compared to the whole cohort (Figure 3D).
Table 2
Method | CoxMoE |
---|---|
Training cohort CV (averaged) | |
Risk score (prediction, C-index) | 0.65 |
Treatment response (prediction, AUC) | 0.83 |
Validation cohort 1 | |
Risk score (prediction, C-index) | 0.64 |
Treatment response (prediction, AUC) | 0.73 |
Validation cohort 2 | |
Risk score (prediction, C-index) | 0.64 |
Treatment response (prediction, AUC) | 0.73 |
CV, cross-validation; C-index, Concordance index; AUC, area under the curve.
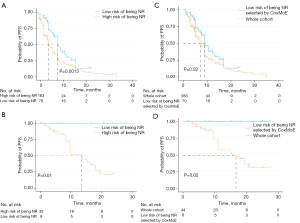
DCA
The DCA indicated that the prediction of therapeutic response could achieve better clinical benefits than PFS prediction across the risk probabilities of 12–60% (Figure 4A), revealing the necessity for early efficacy prediction. Furthermore, the CIC analysis indicated that the patients at high risk for NR were consistent with those who did not respond to treatment when the risk threshold was 0.6 (Figure 4B) and 0.4 for PFS prediction (Figure 4C).
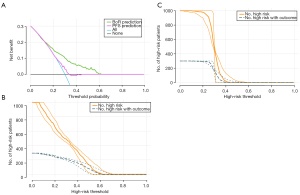
Interpretation of CoxMoE by whole-exome sequencing (WES) and Shapley values
In the 18 patients in the validation cohort 2 who underwent WES detection (detailed WES procedures can be found in Appendix 1), we found that four deep-learning features were associated with distinct altered pathways contributing to tumor aggressiveness and metabolism. Cssmin was positively correlated with gene mutations enriched in pathways such as DNA replication and pyrimidine metabolism (Figure 5), which may explain why Cssmin could be attributed to CoxMoE. Elevated APTT correlated with gene mutations in EGFR-TKI resistance and tumor survival, such as mTOR pathway (Figure 5). Especially, APTT correlated with PTEN, JAK1, and AKT mutations (Figure S3).
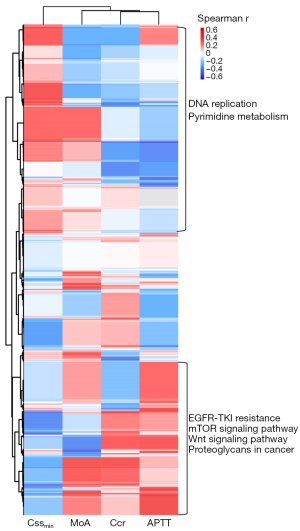
The Shapley values were calculated via SHapley Additive exPlanations (SHAP) to explain the effect of features attributed to CoxMoE. As shown in Figure 6A,6B, the optimal feature of the two prediction tasks differs. For therapeutic response prediction, APTT contributed the most to the model, followed by Cssmin, Ccr, and MoA (Figure 6A). The beeswarm plot showed that lower APTT would likely fail in treatment (Figure 6B). Furthermore, only APTT showed a significant relationship with therapeutic response, and APTT was significantly elevated in NR patients (Figure 6C).
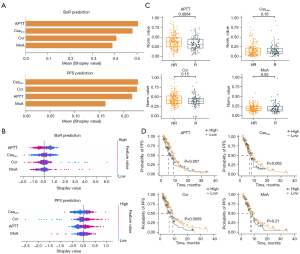
For PFS risk score prediction, Cssmin contributed the most absolute impact on prediction among the four features, followed by Ccr (Figure 6A). The beeswarm plot (contribution distribution) showed that lower Cssmin value had lower Shapley values, indicating longer PFS (Figure 6B). Ccr demonstrated a similar trend (Figure 6B). When patients were divided into high and low groups according to the mean value of Cssmin and Ccr, patients with low Cssmin had longer PFS with marginally significant P value (Figure 6D) and the low Ccr group also had better survival significantly (Figure 6D).
Discussion
This study developed and validated a deep-learning model CoxMoE, that uses pre-therapy EMR data to predict both therapeutic response and PFS in patients with T790M-positive NSCLC treated with third-generation EGFR-TKI. As a result, 61% of low-risk patients predicted by CoxMoE to have a low likelihood of failing to respond to third-generation EGFR-TKI showed a significant 40–50% increase in PFS compared to high-risk patients and a 28–50% increase compared to the whole cohort. It was indicated that CoxMoE demonstrated its potential for guiding patient selection in late-phase clinical trials and complementing current EGFR genotype detection in identifying patients with poor prognoses.
Previous studies have indicated that only 70% of patients with EGFR-positive mutation will respond to EGFR-TKI drugs (2,3). Many patients with such mutation can even experience PD within 9–15 months after receiving treatment (22). There is a huge need for stratifying EGFR-mutant patients according to their prognosis to targeted therapy, which cannot be reflected simply by EGFR genotypes. Consequently, previous studies have explored other methods, such as a non-invasive method to stratify patients with an EGFR mutation. Many studies focused on ML or artificial intelligence combined with CT imaging (6,9,23), making routine laboratory testing a significant waste. Past studies applying EMR for model construction have been reported in breast cancer recurrence (24), 30-day mortality in terminally ill cancer patients (25), and risk prediction in other diseases (26-28).
In contrast with previous artificial intelligence-based models, CoxMoE simultaneously predicts efficacy and personalized prognosis. A previous study demonstrated that EGFR genotype and prognostic information cannot be obtained only from tumor tissues. Macro-level changes were also correlated with therapeutic efficacy and prognosis (7). The good performance of CoxMoE further proved this point. Unlike previous studies that extract tumor information from pre-therapy CT images as the input, this study is the first to explore EMR data by artificial intelligence for efficacy and prognosis prediction of EGFR-TKI. To ensure the robustness of CoxMoE, we built and validated CoxMoE in two prospective multicenter cohorts collected from 16 hospitals and 12 hospitals, respectively.
In this study, CoxMoE model performed better than DeepSurv because the design of CoxMoE was more conducive to capturing different intrinsic subgroup patterns of enrolled patients. Machine-learning methods generally performed worse on every score than deep-learning methods. While deep-learning methods are much more prone to be overfitted on given data than machine-learning methods, there are also plenty of ways to prevent it (e.g., add a dropout layer or add a regularization loss item). More importantly, deep-learning methods can quickly implement different tasks in a single model, but machine-learning methods cannot. After the new task was added, the predictive performance decrement of models for the original task was almost negligible, mainly due to the correlation between the two tasks. Also, our proposed model, CoxMoE, has shown its advantages in this multi-task modeling experiment.
This study found that APTT, Ccr, monocyte, and Cssmin selected for model construction were linked to drug resistance and aggressive tumor pathways. Consistently, retrospective studies have demonstrated the utility of platelet count (29), blood coagulation tests (30), and monocytes (31) in predicting the prognosis of EGFR-TKI treatment for lung cancer. Cancer cells can activate the coagulation system, and coagulation activation and tumor progression are closely related (32). We found that NRs tended to have prolonged APTT, which is in accordance with previous findings (30). The mechanism underneath may be complicated, so further research is needed for mechanism explanations. Cssmin is the indicator of the steady-state plasma drug concentration that is closely related to treatment response. Ccr could reflect the kidney function essential in eliminating (excreting) drugs. If kidney function is impaired, this will slow down the clearance of drugs and thus may influence the drug concentration in the body. Ccr is also necessary for deciding on the usage of drugs in the clinic (33).
Patient selection is a time-consuming process and a key factor in developing novel drugs during clinical trials. Almost one-third of all phase III trials fail due to patient enrollment obstacles, and the recruitment step takes on one-third of the entire trial duration. Ideally, patient-specific molecular profiling is used to determine the biomarkers for drug targets and identify appropriate patient subsets. EMR data is relatively easy to obtain and applicable for practice. Previous studies have investigated combining AI and EMR data for clinical trial outcome prediction and disease monitoring (34-36). The main challenge is the overfitting of the AI model due to the variety of EMR data. To fix this problem, we collected data from a multicenter and enrolled two independent cohorts for validation, exploring the extrapolation capability of the model to other scenarios of the same drug and similar drugs. On the basis of CoxMoE, patients predicted to be high risk made up 28% of all patients, similar to the 30% of patients who failed to respond, as reported by recent studies on EGFR-TKI therapy. DCA analysis indicated that therapeutic response prediction might obtain more clinical benefits than PFS prediction, indicating the necessity for early intervention when a patient is predicted to be at a high risk of being NR. CIC analysis demonstrated that the predicted tumor progression was consistent with actual progression when the probability was higher than 0.4. This result agreed with almost half of the patient’s progress 9–11 months after osimertinib treatment (37). The CoxMoE model effectively supplements EGFR genotype detection, which could aid in selecting appropriate patients for EGFR-TKI treatment. Patients confirmed to have an EGFR mutation by gene sequencing and predicted to be R to EGFR-targeted therapy by CoxMoE showed good prognosis. However, those with a confirmed EGFR mutation by gene sequencing but predicted to be NR showed a poor prognosis. Importantly, the CoxMoE model provides personalized PFS predictions for patients undergoing EGFR-TKIs, offering a means to stratify EGFR-mutant genotypes based on individual therapeutic responses. Consequently, the CoxMoE system represents a considerable expansion to gene sequencing.
However, this study has several limitations. Firstly, the EMR data might not directly link to tumorigenesis and development, rendering the interpretation of the four features and CoxMoE. Features related to genetic mutations associated with PD, such as those demonstrated in earlier studies (38), could be incorporated into further studies. Secondly, the cohort is mostly composed of T790M mutant patients, and further studies should explore the potential application of the CoxMoE model in T790M negative NSCLC patients with EGFR mutations, particularly in the context of third-generation EGFR-TKIs such as furmonertinib, which is more effective than gefitinib in patients with exon 19 deletions or exon 21 L858R mutations (39). Besides, we recognize that the clinical trials included in this study were phase I and II, and as such, it might be required to verify further the CoxMoE model in phase III clinical trials, which we will conduct in the future.
Conclusions
In conclusion, CoxMoE provides a non-invasive way of using routine laboratory tests and pharmacokinetic parameters for predicting therapeutic response and PFS in T790M-positive NSCLC patients treated with third-generation EGFR-TKI in early-phase clinical trials, which will be complementary to current EGFR genotype detection.
Acknowledgments
We would like to thank all of the patients, their families and the study investigators.
Funding: The study was supported by
Footnote
Reporting Checklist: The authors have completed the TRIPOD reporting checklist. Available at https://tlcr.amegroups.com/article/view/10.21037/tlcr-23-737/rc
Peer Review File: Available at https://tlcr.amegroups.com/article/view/10.21037/tlcr-23-737/prf
Conflicts of Interest: All authors have completed the ICMJE uniform disclosure form (available at https://tlcr.amegroups.com/article/view/10.21037/tlcr-23-737/coif). X.L. is a current employee of Huawei Technologies Co., Ltd. C.X. and N.Q. are former employees of Huawei Technologies Co., Ltd. S.W. and W.S. are current employees of Hangzhou ACEA Pharmaceutical Research Co., Ltd. The other authors have no conflicts of interest to declare.
Ethical Statement: The authors are accountable for all aspects of the work in ensuring that questions related to the accuracy or integrity of any part of the work are appropriately investigated and resolved.
Open Access Statement: This is an Open Access article distributed in accordance with the Creative Commons Attribution-NonCommercial-NoDerivs 4.0 International License (CC BY-NC-ND 4.0), which permits the non-commercial replication and distribution of the article with the strict proviso that no changes or edits are made and the original work is properly cited (including links to both the formal publication through the relevant DOI and the license). See: https://creativecommons.org/licenses/by-nc-nd/4.0/.
References
- Akamatsu H, Toi Y, Hayashi H, et al. Efficacy of Osimertinib Plus Bevacizumab vs Osimertinib in Patients With EGFR T790M-Mutated Non-Small Cell Lung Cancer Previously Treated With Epidermal Growth Factor Receptor-Tyrosine Kinase Inhibitor: West Japan Oncology Group 8715L Phase 2 Randomized Clinical Trial. JAMA Oncol 2021;7:386-94. [Crossref] [PubMed]
- Barnet MB, O'Toole S, Horvath LG, et al. EGFR-Co-Mutated Advanced NSCLC and Response to EGFR Tyrosine Kinase Inhibitors. J Thorac Oncol 2017;12:585-90. [Crossref] [PubMed]
- Soria JC, Wu YL, Nakagawa K, et al. Gefitinib plus chemotherapy versus placebo plus chemotherapy in EGFR-mutation-positive non-small-cell lung cancer after progression on first-line gefitinib (IMPRESS): a phase 3 randomised trial. Lancet Oncol 2015;16:990-8. [Crossref] [PubMed]
- Soria JC, Ohe Y, Vansteenkiste J, et al. Osimertinib in Untreated EGFR-Mutated Advanced Non-Small-Cell Lung Cancer. N Engl J Med 2018;378:113-25. [Crossref] [PubMed]
- Ramalingam SS, Vansteenkiste J, Planchard D, et al. Overall Survival with Osimertinib in Untreated, EGFR-Mutated Advanced NSCLC. N Engl J Med 2020;382:41-50. [Crossref] [PubMed]
- Deng K, Wang L, Liu Y, et al. A deep learning-based system for survival benefit prediction of tyrosine kinase inhibitors and immune checkpoint inhibitors in stage IV non-small cell lung cancer patients: A multicenter, prognostic study. EClinicalMedicine 2022;51:101541. [Crossref] [PubMed]
- Wang S, Yu H, Gan Y, et al. Mining whole-lung information by artificial intelligence for predicting EGFR genotype and targeted therapy response in lung cancer: a multicohort study. Lancet Digit Health 2022;4:e309-19. [Crossref] [PubMed]
- Mu W, Jiang L, Zhang J, et al. Non-invasive decision support for NSCLC treatment using PET/CT radiomics. Nat Commun 2020;11:5228. [Crossref] [PubMed]
- Song J, Wang L, Ng NN, et al. Development and Validation of a Machine Learning Model to Explore Tyrosine Kinase Inhibitor Response in Patients With Stage IV EGFR Variant-Positive Non-Small Cell Lung Cancer. JAMA Netw Open 2020;3:e2030442. [Crossref] [PubMed]
- Gould MK, Huang BZ, Tammemagi MC, et al. Machine Learning for Early Lung Cancer Identification Using Routine Clinical and Laboratory Data. Am J Respir Crit Care Med 2021;204:445-53. [Crossref] [PubMed]
- Yang HS, Hou Y, Vasovic LV, et al. Routine Laboratory Blood Tests Predict SARS-CoV-2 Infection Using Machine Learning. Clin Chem 2020;66:1396-404. [Crossref] [PubMed]
- Liu R, Rizzo S, Whipple S, et al. Evaluating eligibility criteria of oncology trials using real-world data and AI. Nature 2021;592:629-33. [Crossref] [PubMed]
- Bedon L, Cecchin E, Fabbiani E, et al. Machine Learning Application in a Phase I Clinical Trial Allows for the Identification of Clinical-Biomolecular Markers Significantly Associated With Toxicity. Clin Pharmacol Ther 2022;111:686-96. [Crossref] [PubMed]
- Zhou Q, Wu L, Hu P, et al. A Novel Third-generation EGFR Tyrosine Kinase Inhibitor Abivertinib for EGFR T790M-mutant Non-Small Cell Lung Cancer: a Multicenter Phase I/II Study. Clin Cancer Res 2022;28:1127-35. [Crossref] [PubMed]
- Ma Y, Zheng X, Zhao H, et al. First-in-Human Phase I Study of AC0010, a Mutant-Selective EGFR Inhibitor in Non-Small Cell Lung Cancer: Safety, Efficacy, and Potential Mechanism of Resistance. J Thorac Oncol 2018;13:968-77. [Crossref] [PubMed]
- Shi Y, Zhou J, Zhao Y, et al. Results of the phase IIa study to evaluate the efficacy and safety of rezivertinib (BPI-7711) for the first-line treatment of locally advanced or metastatic/recurrent NSCLC patients with EGFR mutation from a phase I/IIa study. BMC Med 2023;21:11. [Crossref] [PubMed]
- Shi Y, Zhao Y, Yang S, et al. Safety, Efficacy, and Pharmacokinetics of Rezivertinib (BPI-7711) in Patients With Advanced NSCLC With EGFR T790M Mutation: A Phase 1 Dose-Escalation and Dose-Expansion Study. J Thorac Oncol 2022;17:708-17. [Crossref] [PubMed]
- Bellal Z, Nour B, Mastorakis S. CoxNet: A Computation Reuse Architecture at the Edge. IEEE Trans Green Commun Netw 2021;5:765-77. [Crossref] [PubMed]
- Simon N, Friedman J, Hastie T, et al. Regularization Paths for Cox's Proportional Hazards Model via Coordinate Descent. J Stat Softw 2011;39:1-13. [Crossref] [PubMed]
- Pölsterl S, Navab N, Katouzian A. Fast Training of Support Vector Machines for Survival Analysis. In: Appice A, Rodrigues P, Santos Costa V, et al. editors. Machine Learning and Knowledge Discovery in Databases. Cham: Springer, 2015.
- Katzman JL, Shaham U, Cloninger A, et al. DeepSurv: personalized treatment recommender system using a Cox proportional hazards deep neural network. BMC Med Res Methodol 2018;18:24. [Crossref] [PubMed]
- Recondo G, Facchinetti F, Olaussen KA, et al. Making the first move in EGFR-driven or ALK-driven NSCLC: first-generation or next-generation TKI? Nat Rev Clin Oncol 2018;15:694-708. [Crossref] [PubMed]
- Song J, Shi J, Dong D, et al. A New Approach to Predict Progression-free Survival in Stage IV EGFR-mutant NSCLC Patients with EGFR-TKI Therapy. Clin Cancer Res 2018;24:3583-92. [Crossref] [PubMed]
- Zhu Z, Li L, Ye Z, et al. Prognostic value of routine laboratory variables in prediction of breast cancer recurrence. Sci Rep 2017;7:8135. [Crossref] [PubMed]
- Kawai N, Yuasa N. Laboratory prognostic score for predicting 30-day mortality in terminally ill cancer patients. Nagoya J Med Sci 2018;80:571-82. [PubMed]
- Yang C, Zhu X, Liu J, et al. Development and Validation of Prognostic Models to Estimate the Risk of Overt Hepatic Encephalopathy After TIPS Creation: A Multicenter Study. Clin Transl Gastroenterol 2022;13:e00461. [Crossref] [PubMed]
- Haimovich AD, Ravindra NG, Stoytchev S, et al. Development and Validation of the Quick COVID-19 Severity Index: A Prognostic Tool for Early Clinical Decompensation. Ann Emerg Med 2020;76:442-53. [Crossref] [PubMed]
- Farrell PR, Hornung L, Farmer P, et al. Who's at Risk? A Prognostic Model for Severity Prediction in Pediatric Acute Pancreatitis. J Pediatr Gastroenterol Nutr 2020;71:536-42. [Crossref] [PubMed]
- Xu L, Xu F, Kong H, et al. Effects of reduced platelet count on the prognosis for patients with non-small cell lung cancer treated with EGFR-TKI: a retrospective study. BMC Cancer 2020;20:1152. [Crossref] [PubMed]
- Tas F, Kilic L, Serilmez M, et al. Clinical and prognostic significance of coagulation assays in lung cancer. Respir Med 2013;107:451-7. [Crossref] [PubMed]
- Watanabe K, Yasumoto A, Amano Y, et al. Mean platelet volume and lymphocyte-to-monocyte ratio are associated with shorter progression-free survival in EGFR-mutant lung adenocarcinoma treated by EGFR tyrosine kinase inhibitor. PLoS One 2018;13:e0203625. [Crossref] [PubMed]
- Falanga A, Marchetti M, Vignoli A. Coagulation and cancer: biological and clinical aspects. J Thromb Haemost 2013;11:223-33. [Crossref] [PubMed]
- Shah J, Fogel J, Balsam L. Importance of creatinine clearance for drug dosing in nursing home residents. Ren Fail 2014;36:46-9. [Crossref] [PubMed]
- Sun Z, Ghosh S, Li Y, et al. A probabilistic disease progression modeling approach and its application to integrated Huntington's disease observational data. JAMIA Open 2019;2:123-30. [Crossref] [PubMed]
- Raghu VK, Walia AS, Zinzuwadia AN, et al. Validation of a Deep Learning-Based Model to Predict Lung Cancer Risk Using Chest Radiographs and Electronic Medical Record Data. JAMA Netw Open 2022;5:e2248793. [Crossref] [PubMed]
- Lee RY, Kross EK, Torrence J, et al. Assessment of Natural Language Processing of Electronic Health Records to Measure Goals-of-Care Discussions as a Clinical Trial Outcome. JAMA Netw Open 2023;6:e231204. [Crossref] [PubMed]
- Mok TS, Wu Y-L, Ahn M-J, et al. Osimertinib or Platinum-Pemetrexed in EGFR T790M-Positive Lung Cancer. N Engl J Med 2017;376:629-40. [Crossref] [PubMed]
- Shi Y, Hu X, Zhang S, et al. Efficacy, safety, and genetic analysis of furmonertinib (AST2818) in patients with EGFR T790M mutated non-small-cell lung cancer: a phase 2b, multicentre, single-arm, open-label study. Lancet Respir Med 2021;9:829-39. [Crossref] [PubMed]
- Shi Y, Chen G, Wang X, et al. Furmonertinib (AST2818) versus gefitinib as first-line therapy for Chinese patients with locally advanced or metastatic EGFR mutation-positive non-small-cell lung cancer (FURLONG): a multicentre, double-blind, randomised phase 3 study. Lancet Respir Med 2022;10:1019-28. [Crossref] [PubMed]