Accurate categorization and rapid pathological diagnosis correction with Micro-Raman technique in human lung adenocarcinoma infiltration level
Highlight box
Key findings
• This study amalgamates Micro-Raman technique with artificial intelligence algorithms, unveiling molecular-level nuances in diverse levels of infiltration within lung adenocarcinomas. This synergistic integration not only attains high-precision discrimination of tissue types but also rectifies inaccuracies in rapid pathology diagnostic outcomes.
What is known and what is new?
• In conventional lung cancer surgeries, differentiating between invasive adenocarcinoma and microinvasive adenocarcinoma involves rapid pathological diagnosis through tissue biopsies. However, the process is prone to errors, and varying diagnoses significantly influence the surgeon’s formulation of precise surgical plans.
• The integration of Micro-Raman and artificial intelligence classification algorithm enables direct, highly accurate lesion diagnosis without the need for biopsy sampling. The diagnostic accuracy closely approaches the gold standard in pathology.
What is the implication, and what should change now?
• A broader patient cohort is imperative for a comprehensive exploration of the utility of artificial intelligence-based Micro-Raman technique in lung cancer surgery. The integration of a standardized Raman diagnostic module into the surgical process is essential, offering surgeons an objective and reliable foundation for formulating surgical treatment plans.
Introduction
Lung cancer, a prevailing malignancy worldwide, contributes to 18% of total mortality in neoplastic conditions (1,2). Lung adenocarcinoma (LADC) is the predominant histologic subtype of primary lung cancer, representing over 40% of cases, and a major contributor to mortality, especially in advanced stages (3-5). Treating LADC poses significant challenges due to tumor cell recurrence and lymphatic metastasis (6,7). The degree of infiltration in LADC dictates the surgical approach, with patients diagnosed with invasive adenocarcinoma (IAC) often requiring lobectomy and systematic lymph node dissection. Conversely, for microinvasive adenocarcinomas (MIA), sublobar resection and lymph node sampling (8,9).
Quantitative immunohistochemical analysis of tumor markers in formalin-fixed paraffin-embedded (FFPE) assays can assist in detecting tumor infiltration (10), and positron emission tomography/computed tomography (PET/CT) scans are effective in identifying metastatic lesions in LADCs based on molecular metabolism (11,12). However, current diagnostic and medical imaging methods encounter challenges in accurately assessing the intraoperative extent of LADC infiltration. The only reliable option, intraoperative rapid pathology, is often prone to inherent subjectivity based on the specimen site and pathologist, resulting in the oversight of lesions and significant inter- and intra-observer disparities. A study has reported 15.6% discrepancy between intraoperative rapid pathology and postoperative gold standard results (13), underscoring the profound impact of these discrepancies on the management of LADC patients, given the distinct clinical significance between minimally invasive and invasive cases.
Research has shown that lymph node dissection compromises the systemic anti-tumor immune response, increasing the risk of complications such as lymphedema in patients (14). Although broader lymph node clearance can enhance recurrence-free survival rates, extensive clearance often results in various complications and does not significantly improve overall survival rates and prognosis for these patients (15,16). Therefore, accurately determining the infiltration depth of LADC intraoperatively is crucial for designing appropriate surgical strategies. This approach not only effectively prevents tumor recurrence and metastasis but also reduces complications from excessive lymph node removal, such as infection, lymphedema, and weakened immune response to tumors. Currently, there is a lack of an effective intraoperative tool with high specificity for determining the infiltration depth of LADC, highlighting the need to supplement or replace intraoperative rapid pathology diagnostic results.
The Micro-Raman technique is an optical technique based on laser-molecule interaction, offering high molecular specificity and allowing comprehensive molecular characterization of pathological tissue sections (17-19). Given the dynamic changes in the composition and structural configuration of biological molecules in tissues and cells during carcinogenesis, this technique aids in assessing the infiltration depth of LADC through parameters such as the characteristics and intensity of Raman spectral peaks. McGregor et al. (20) presented a multivariate analysis and band selection method using endoscopic Raman spectroscopy that demonstrated the ability to detect highly heterogeneous hyperplasia (HGD) and malignant lung lesions with high sensitivity (90%) and good specificity (65%). Qi et al. (21) reported that the diagnosis of LADC and squamous cell carcinoma tissues by deep learning combined with Raman spectroscopy had high sensitivity and specificity. Subsequently, other research groups have reported the ability of combining surface-enhanced Raman scattering (SERS) to differentiate between lung cancer and cell lines of different diseases (22-24). Although Raman spectroscopy has demonstrated its potential in lung cancer identification, to the best of our knowledge, this technique has not been used to diagnose the depth of infiltration of LADC or to correct intraoperative rapid pathologic diagnosis.
In this investigation, we integrated Micro-Raman techniques into the comprehensive workflow of LADC tumor surgery. Figure 1 provides an overview of our approach pipeline, which encompasses the entire LADC clinical surgical process and highlights the role of Micro-Raman techniques. Using samples from 59 patients after clinical postoperative, we employed the Micro-Raman technique on pathological tissue sections following the established protocols of the Department of Pathology. This procedure is devised to gather Raman spectral data for diagnostic purposes through the utilization of Micro-Raman techniques. Subsequently, artificial intelligence algorithmic models will be developed based on distinct categories of spectra. These models will undergo training and testing to discern variations between LADC and normal tissue. Additionally, our endeavor includes the differentiation of various degrees of LADC infiltration, with the aim of surpassing the diagnostic accuracy attained by intraoperative rapid pathology methods. We present this article in accordance with the TRIPOD reporting checklist (available at https://tlcr.amegroups.com/article/view/10.21037/tlcr-24-168/rc).
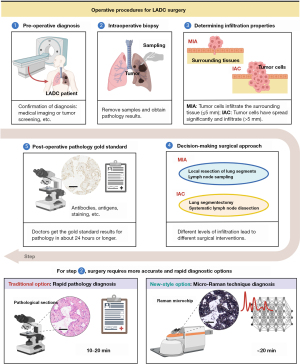
Methods
Study design
In this investigation, we collected patients with LADC to explore the potential of Micro-Raman technology, coupled with artificial intelligence classification models, in aiding diagnostic strategies for intraoperative identification of the extent of LADC infiltrative properties. Microscopic Raman technology entails obtaining fingerprinted spectra from lesion site to discern lesion trends within the organism by identifying variations between spectra. Furthermore, classification algorithms were employed to predict tissue type and the degree of infiltrative nature of LADC. The specific clinical inquiry addressed herein was whether Raman spectroscopy could be employed to rectify diagnostic errors during intraoperative pathological assessments, thereby aiding surgeons in formulating a judicious surgical plan. The acquired Raman spectra served as inputs to classification model [support vector machine (SVM), convolutional neural network (CNN)], and subsequent classification was performed.
Study population
We employed a stepwise sample accumulation approach, recruiting and enrolling patients who met the inclusion criteria throughout the study period. The study cohort included 59 patients diagnosed with LADC who received surgical interventions at Nanjing Drum Tower Hospital, The Affiliated Hospital of Nanjing University Medical School, from March 2023 to October 2023. Information about all patients is in Table S1. All these individuals underwent both rapid intraoperative pathological diagnosis and postoperative gold-standard pathological diagnosis, with pathologists providing the diagnostic outcomes.
A more nuanced tumor classification was conducted in accordance with the 2015 World Health Organization (WHO) criteria for lung tumor classification. Herein, tissues unequivocally identified as MIA and IAC consistently fell under the broader classification of LADC within the lung cancer classification framework. The study was conducted in accordance with the Declaration of Helsinki (as revised in 2013). Our study received approval from the Medical Ethics Committee of Nanjing Drum Tower Hospital, The Affiliated Hospital of Nanjing University Medical School (Approval Number: 2020-134-01). Informed consent was provided by all the patients. The clinical data were anonymized before the computational analysis.
Tissue section preparation
Tissue specimens obtained after surgery were carefully fixed to preserve their intrinsic morphological characteristics, then processed into FFPE sections and serially sliced. The first slice, which had a pathology thickness of 4 µm, was delicately affixed to a slide and subjected to hematoxylin and eosin (H&E) staining, adhering to meticulously standardized protocols within the pathology department. The second slice, specifically designated for Raman spectroscopy in the realm of pathology, had a thickness of 6 µm. Following the deparaffinization process, it underwent natural desiccation and was carefully placed on a sleek aluminum slide, denoted as Raman microchip. Concurrently, the third slice underwent immunohistochemical staining, primarily leveraging the thyroid transcription factor-1 (TTF-1) tumor marker, possessing a thickness of 4 µm. More operational details and a schematic diagram of serial slicing can be found in the Appendix 1 and Figure S1. TTF-1, a thyroid-specific enhancer-binding protein and nuclear transcription factor, plays a crucial role in identifying primary tumor metastasis and cancer differentiation, exhibiting positive expression in 60–80% of LADC cases (25). This strategic approach aims to augment the interpretative depth and diagnostic acumen for pathologists. The integration of serial slices from the same specimen ensures that spectroscopic diagnostic outcomes are scrutinized in parallel with histological evaluations, meticulously aligned with the pertinent pathology coordinates.
Raman measurements and data preprocessing
The Micro-Raman system (InVia; Renishaw, Wotton-under-Edge, UK) with a 785 nm excitation laser is based on an optical microscope used to focus the excitation light and collect it in a backscattering configuration, a monochromator, notch filters system, and a charge coupled detector. The system was calibrated against the 520.5 cm−1 line of an internal silicon wafer before testing. The spectra were registered in the 765–1,721 cm−1 range, particularly sensitive the bioinformatics-intensive area. The measurements were conducted at room temperature on a metal slide using the 50× short working distance objective [numerical aperture (NA) =0.75, working distance (WD) =0.35 mm], with the sample mounted on a translation stage of a Leica microscope (Leica, Wetzlar, Germany). Subsequently, we selected three 200×200 µm2 rectangular regions within the cell-rich tissue of interest, with 100–200 collection sites designated for each rectangular region. A double-blind method was employed during collection, where the operator selecting the regions was not aware of the pathological diagnosis of the samples, ensuring objectivity and fairness in the analysis. Each spectrum was generated by integrating at 50% laser power (~150 mW) for duration of 3 seconds, and the final data were averaged over 3 accumulations in order to maximize the signal to noise ratio (SNR). Additional operational details can be found in the Appendix 1.
Before conducting statistical analyses, WIRE 5.4 software (Renishaw, UK) was employed to eliminate anomalous spectra and cosmic rays. The preprocessing of all data commenced with background subtraction from the substrate, followed by noise reduction employing principal component analysis, retaining at least 2 principal components. Subsequently, baseline corrections and Savitzky-Golay smoothing were applied, with smooth window and polynomial order set at 5 and 3, respectively. Finally, a maxima normalization process was implemented to confine the Y-axis of the data within the range of 0 to 1 (18). A double-blind approach was used in data preprocessing, with personnel unaware of sample details and diagnoses to reduce bias.
Classification algorithms
The SVM serves as a supervised learning algorithm catering to classification and regression tasks. Its functionality lies in discerning the optimal hyperplane within a high-dimensional space, effectively segregating distinct classes of data points (26). The model adopts a linear kernel function, configuring the regularization parameter (C) to 1 and the kernel coefficient (gamma) to 0.01. Throughout the classification process, the SVM model prioritizes balancing category weights to ensure sensitivity across all categories. The dataset is partitioned into 5 folds, and each iteration entails training on a subset of the data and validating on the remainder. For the validation set comprising 20% randomly retained patients, a logistic regression function is employed by the model to predict category probabilities. The serialized SVM model is archived for subsequent testing on the remaining patient dataset.
The CNN architecture features three 1-dimensional (1D) convolutional layers tailored for binary classification tasks with labels 0 and 1. Inputting as 1D data with dimensions (888, 1), Batch Normalization and MaxPooling1D layers follow each convolutional layer, culminating in a fully connected layer and an output layer. The initial convolutional layer is equipped with 32 filters and a kernel size of 3. Subsequent convolutional layers incorporate 64 filters, maintaining the kernel size of 3, whereas the third convolutional layer integrates 128 filters with the same kernel size of 3. Nonlinear properties are introduced through the application of the rectified linear unit (ReLU) activation function. The model’s output represents the prediction probability, spanning from 0 to 1. In the training phase, an Adam optimizer and a binary cross-entropy loss function are employed. Besides, we adopt balanced weight strategy due to the imbalanced datasets. The model undergoes training and evaluation utilizing a 5-fold cross-validation method, with evaluation metrics such as the confusion matrix and receiver operating characteristic (ROC) curves employed for a comprehensive performance assessment. ModelCheckpoint callbacks are implemented to retain the model weights with the highest validation accuracy in each iteration.
Notably, each patient is treated as a distinct dataset in both models, distinguishing this study from others that amalgamate all patient data before categorization. This approach upholds the model’s capacity to generalize across populations or individuals, as differences between individuals are smaller than those between organizations, preventing the same patient’s data from appearing in both the training and test sets.
Statistical analysis
Statistical evaluation involved the use of analysis of variance (ANOVA) complemented by Fisher’s post hoc least significant difference (LSD) test to discern spectral variations among distinct tissues, with statistical significance defined as a P value ≤0.05. For meaningful clinical interpretation, the anomalous spectral data were screened and removed using WIRE 5.4 software and subsequently the SVM model was employed to extract relevant Raman spectral eigenvalues. Tissue diagnosis and classification were accomplished through the implementation of SVM and CNN models in this investigation. Given the acquisition of multiple Raman spectra from each lesion, a per-lesion-based analysis was conducted utilizing majority voting. Confusion matrices were generated to delineate the interplay between diagnostic sensitivity, specificity, accuracy, and F1-score (see Appendix 1 and Table S2). Additionally, the area under the curve (AUC) for ROC analysis was calculated to assess the robustness of Micro-Raman in vitro, specifically in diagnosing the extent of LADC infiltration.
Statistical computations were performed using Microsoft Excel (Microsoft Corp., Redmond, WA, USA) within the Microsoft system, whereas the classification models for lung cancer detection based on Raman spectra were implemented in Python 3.9 environment using the Scikit-learn and TensorFlow libraries. This comprehensive approach allowed for meticulous statistical analyses and the development of sophisticated machine learning models, ensuring a rigorous investigation into the intricacies of lung cancer diagnostics through Raman spectroscopy.
Results
Patient characteristics
Among the cohort of 59 eligible patients included in our study, adjacent non-cancerous samples from 12 individuals were meticulously examined, yielding 12 isolated specimens of normal lung tissue. These specimens, alongside the 41 patients diagnosed with LADC, were incorporated into both the cross-validation of the tissue type dichotomy model and the subsequent test set. Notably, this inclusive approach aimed to holistically assess the robustness of the model across a diverse patient population.
To further validate the efficacy of the Raman technique in error correction for rapid pathological diagnosis, the LADC patients underwent meticulous classification into 5 cases of MIA and 35 cases of IAC (randomly selected from 36 patients), conforming to the gold standard pathological diagnosis. Subsequently, this classification was rigorously cross-validated. The test set for evaluating the degree of infiltration comprised 6 carefully selected patients, including 3 cases initially diagnosed intraoperatively as MIA by rapid pathological diagnosis. These cases were later validated as IAC based on the meticulous diagnosis of the pathological gold standard adenocarcinoma. Additionally, 3 cases were unequivocally identified as IAC in both rapid pathology and the gold standard pathology diagnosis. All classification models were executed for 2 distinct tissue types. Firstly, normal tissue versus LADC, with the primary objective of swiftly identifying LADC intraoperatively, and subsequently, the degree of infiltration of the LADC intraoperatively. This sequential approach was designed to ensure that the outcomes of this diagnostic step significantly influenced subsequent surgical protocols. Table 1 provides comprehensive details on the clinical information of the patients and specifics of the Raman acquisition model.
Table 1
Sample type | Patients | Age (years), range | Specimens | Raman points | Rapid pathology | Gold standard |
---|---|---|---|---|---|---|
Normal tissue & LADC | ||||||
Normal tissue | 12 | 51–75 | 12 | 2,699 | Normal | Normal |
LADC | 41 | 26–74 | 61 | 16,210 | LADC | LADC |
MIA & IAC (train) | ||||||
MIA | 5 | 26–55 | 7 | 2,654 | MIA | MIA |
IAC | 36 | 41–74 | 54 | 13,556 | IAC | IAC |
MIA & IAC (test) | ||||||
MIA | 3 | 55–62 | 3 | 1,681 | MIA | IAC |
IAC | 3 | 54–74 | 3 | 1,693 | IAC | IAC |
LADC, lung adenocarcinoma; MIA, microinvasive adenocarcinomas; IAC, invasive adenocarcinoma.
Raman spectra analysis
Digitally captured using a digital pathology slide scanner (Konfoong Bioinformation Tech Co., Ltd., KF-PRO-005, China), slides initially stained with immunohistochemistry (IHC) and H&E underwent thorough examination by the pathologist. The presence of LADC cells, MIA regions, IAC sites, and normal tissue on the pathology slides was meticulously identified and confirmed. Raman spectroscopy was then conducted on pathological tissues obtained from 59 participants. Given the inherent heterogeneity and intricacy of cancerous tissues, it became imperative to ascertain that the adjacent Raman microchip within the target acquisition area conformed to morphologically characterized pathology criteria, serving as a crucial reference (Figure 2A). The Raman spectra from all tissue sections underwent a rigorous and standardized preprocessing procedure. T-distributed stochastic neighbor embedding (T-SNE) plots vividly illustrated the clustering patterns among the preprocessed data of various tissues as shown in Figure 2B,2C. Notably, the data from LADC with distinct infiltration degrees exhibited a more pronounced separation compared to normal and cancerous tissues. This observation implies a higher feasibility in distinguishing between normal and cancerous tissues. Subsequently, the mean (M) and standard deviation (SD) of the Raman spectra across different tissues were computed and visualized (Figure 2D,2E). The broader ± SD shading captured individual variations and deviations from tissue homeostasis attributed to lesions.
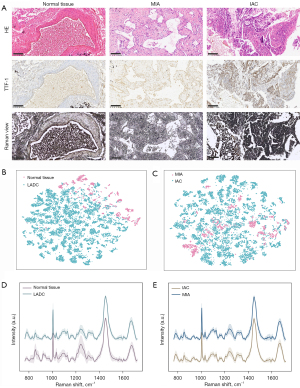
In the feature selection phase of the SVM model, we filtered the features by feature relevance information and retained the top ten features that have significant impact on the model prediction. Following this, the normalized Raman spectra of the 2 classified tissues underwent subtraction to generate a Raman difference map (Figure 3A,3B). This meticulous approach facilitated the detailed delineation of spectral variations attributed to alterations in biological composition, leveraging disparities in the feature peaks of each tissue. Referring to Table S3, detailing the vibrational modes of major Raman peaks and their corresponding representative bioinformation, it is evident that the intensities of the 10 characteristic Raman peaks were notably higher in normal tissues compared to LADC tissues. Conversely, in most cases, the intensities of individual peaks in MIA were significantly higher than those in IAC (P<0.001). The majority of these peaks are associated with aromatic amino acids, proteins, and lipids. To elaborate, peaks near 852, 937, 1,032, and 1,209 cm−1 align with proline, tryptophan, tyrosine, or phenylalanine, respectively. At 1,004 cm−1, the Raman spectral line represents the C-C aromatic ring stretching of carotenoids. Additional features at 1,308 and 1,451 cm−1 are predominantly associated with lipids or fatty acids, and the band at 1,341 cm−1 can be attributed to nucleic acids. The peak at 1,238 cm−1 corresponds to the C-N and N-H bends of amide III, whereas the band at 1,671 cm−1 corresponds to the C=O stretching and N-H bending of the amide I band. The distinctions in Raman spectra between normal tissues and LADC with varying degrees of infiltration underscored the alterations in the biochemical composition of lung tissues during carcinogenesis, forming a foundational basis for distinguishing cancerous tissues from normal tissues.
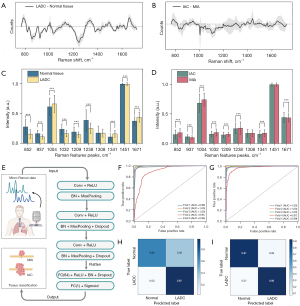
In the subsequent refined peak comparison analysis depicted in Figure 3C, the primary disparity in Raman signal intensity between the normal and LADC groups was observed in protein-related Raman peaks (852, 937, 1,032, 1,209, and 1,238 cm−1), exhibiting a significant elevation in the normal group. In contrast, most lipid- and nucleic acid-related peaks, such as 1,341, 1,308, 1,451, and 1,671 cm−1, displayed higher intensities in the LADC group. Furthermore, precise determination of LADC infiltrability holds implications for selecting surgical strategies and prognostic assessments. As depicted in Figure 3D, the principal spectral distinctions between MIA and IAC were identified in phenylalanine at 1,004, 1,032 cm−1, tryptophan within protein at 1,209 and 1,238 cm−1, and nucleic acids at 1,341 cm−1, with higher intensities observed in the MIA group. The peak comparison plot underscores that the overall spectral differences between LADC and the normal group are more prominent than the disparities between LADCs with varying degrees of infiltration.
We subsequently devised 2 classification models: an SVM model grounded in machine learning and another CNN model rooted in deep learning (workflow in Figure 3E). These models were specifically designed to accurately differentiate between tissue types. The stability of each model was assessed by computing diagnostic metrics. A notable observation from Table 1 is the substantial variance in data volume across different groups in the dichotomous classification model. Consequently, it is imperative not only to carefully control the weighting of specific categories during model construction but also to uphold the generalization capability of the model.
Evaluation and validation of classification models
Given the straightforward differentiation between normal tissue and LADC in histopathology, our initial step involved testing the development of a classification model to validate the system’s capabilities. In the classification model for normal tissue and LADC, normal tissue from 10 patients and tumor tissue from 35 patients constituted the 5-fold cross-validation dataset, whereas the remaining dataset from 8 patients served as the test set. The ROC curves displayed in Figure 3F,3G were employed for quantitative validation of classifier performance. Among the 5 models trained in the 5-fold cross-validation, the AUC values for the SVM model ranged between 0.91 and 1.0, whereas those for the CNN model ranged between 0.97 and 1.0. The test set confusion matrix displayed in Figure 3H,3I, when combined with these results, illustrates the SVM model’s limited predictive ability, suggesting that individual differences and tumor heterogeneity pose challenges to the classification of tumor tissues and the discernment of infiltration degree. Conversely, the CNN model demonstrates superior computational performance and higher accuracy in identifying tissues of different pathology types, particularly in handling unbalanced data volumes.
It was interpreted that both models effectively differentiate normal tissue from LADC, as evidenced by cross-validation sensitivity, specificity, accuracy, F1-score, and AUC values of 99.3%±0.7%, 69.5%±22.6%, 94.7%±4.4%, 96.9%±2.6%, and 97.6%±3.5%, respectively, for the SVM model (Table 2). When the model underwent testing on an independent cohort of 8 patients, its classification performance remained consistent, yielding sensitivity, specificity, accuracy, F1-score, and AUC values of 96.6%, 63.5%, 93.2%, 96.2%, and 96.2%, respectively. However, the suboptimal specificity of the SVM model suggests challenges in accurately identifying negative category samples, potentially resulting in false positives by misclassifying some negative category samples as positive. In contrast, CNNs excel in extracting features from data through layers and adapting to the complex structure of the data during the learning process. Consequently, CNNs exhibit greater sensitivity in distinguishing subtle differences between categories. The results demonstrate that CNNs, tested via cross-validation on the same dataset, achieved sensitivity, specificity, accuracy, F1-score, and AUC values of 93.3%, 91.2%, 93.1%, 96.0%, and 96.1%, respectively. This underscores the suitability of CNNs for handling complex and high-dimensional data, enabling effective learning and generalization across the dataset’s significant variability. In certain scenarios, CNNs prove more advantageous compared to traditional models such as SVM.
Table 2
Arithmetic | Classified tissues | Sensitivity, % | Specificity, % | Accuracy, % | F1-score, % | AUC, % |
---|---|---|---|---|---|---|
Normal tissue & LADC | ||||||
SVM | Training | 99.3±0.7 | 69.5±22.6 | 94.7±4.4 | 96.9±2.6 | 97.6±3.5 |
Testing | 96.6 | 63.5 | 93.2 | 96.2 | 96.2 | |
CNN | Training | 98.0±1.8 | 92.1±6.4 | 97.1±1.7 | 98.3±1.0 | 99.2±0.9 |
Testing | 93.3 | 91.2 | 93.1 | 96 | 96.1 | |
MIA & IAC | ||||||
SVM | Training | 99.5±0.4 | 45±28.8 | 89.2±6.5 | 93.7±4.0 | 94.1±9.8 |
CNN | Training | 98.5±1.9 | 95.8±4.5 | 98.2±2.0 | 98.9±1.1 | 99.4±0.9 |
LADC, lung adenocarcinoma; MIA, microinvasive adenocarcinomas; IAC, invasive adenocarcinoma; SD, standard deviation; AUC, area under the curve; SVM, support vector machine; CNN, convolutional neural network.
Building upon these findings, we proceeded to construct a classification model designed to differentiate between MIA and IAC tissues. In this study, we acquired a total of 2,654 Raman spectra from 7 Raman microchips obtained from 5 MIA patients. For classification purposes, these spectra were compared against 13,556 Raman spectra from 54 Raman microchips gathered from 36 IAC patients. The classification model underwent cross-validation using the entire available dataset. We trained SVM and CNN models with 5-fold cross-validation on a dataset including 5 MIA patients and 35 randomly selected IAC patients. The SVM models exhibited sensitivity, specificity, accuracy, F1-score, and AUC of 99.5%±0.4%, 45%±28.8%, 89.2%±6.5%, 93.7%±4.0%, and 94.1%±9.8%, respectively. Meanwhile, the corresponding metrics for CNN models were 98.5%±1.9%, 95.8%±4.5%, 98.2%±2.0%, 98.9%±1.1%, and 99.4%±0.9%, respectively. As shown in Figure 4A,4B, the AUC values of the SVM models ranged between 0.74 and 1, whereas that of the CNN models ranged between 0.98 and 1. In the assessment of LADC infiltration, the conventional SVM model displayed lower specificity attributed to variations in data quantity among different categories. In contrast, the CNN model showcased superior diagnostic capabilities.
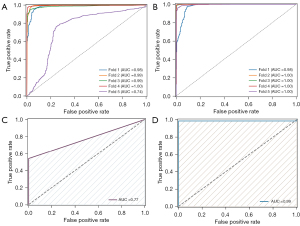
Correction for rapid intraoperative pathologic diagnosis
Trained SVM and CNN models were tested on a dataset containing six patients in total. Among them, three patients were initially diagnosed with MIA at rapid intraoperative diagnosis but later diagnosed with IAC by postoperative pathologic gold standard, while the other three patients were diagnosed with IAC by either rapid pathology or gold standard. The AUC results are 77% and 99% for SVM and CNN separately (Figure 4C,4D). In Table 3, we provide a comprehensive illustration of the significant role played by Raman technique in facilitating the pathologic diagnosis process across distinct patient cases. Specifically, the CNN model evaluates the volume of data erroneously diagnosed as MIA within the entire Raman dataset for a patient initially misdiagnosed through rapid intraoperative pathology. The CNN-assisted Raman technique effectively rectified the diagnoses for the three IAC patients misclassified as MIA by rapid pathology, achieving correction rates of 99.85%, 100%, and 96.86%, respectively, when compared against the pathology gold standard. Remarkably, for the three patients with IAC who were not misdiagnosed by rapid pathology, the Raman technique demonstrated a flawless 100% correct diagnosis rate.
Table 3
Patients | Rapid pathology diagnosis |
Raman dataset | Number of MIA diagnoses |
Number of IAC diagnoses |
Pathological gold standard |
---|---|---|---|---|---|
1 | MIA | 677 | 1 | 676 | IAC |
2 | MIA | 398 | 0 | 398 | IAC |
3 | MIA | 606 | 19 | 587 | IAC |
4 | IAC | 777 | 0 | 777 | IAC |
5 | IAC | 544 | 0 | 544 | IAC |
6 | IAC | 372 | 0 | 372 | IAC |
CNN, convolutional neural network; IAC, invasive adenocarcinoma; MIA, microinvasive adenocarcinomas.
Discussion
LADC is the most important histologic subtype of lung cancer, and early diagnosis and clinical intervention are particularly important for patient prognosis (27). Given the distinct surgical prognostic measures triggered by diagnostic results of MIA and IAC, accurate intraoperative diagnosis provides an objective foundation for surgeons in determining the next surgical plan during LADC procedures, holding significant clinical and practical implications. This study underscores the clinical significance of intelligent and digital intraoperative pathology diagnosis while shedding light on the potential advancement offered by deep learning-assisted Micro-Raman technique as a versatile tool for intraoperative diagnosis of LADC infiltrative pathology. The accelerated detection speed and heightened diagnostic accuracy of the Raman diagnostic method employed in this investigation enable the swift identification of biomolecular vibrational signals with fingerprint specificity, obviating the need for dependence on tumor markers.
Leveraging imaging data and insights from prior studies, clinicians can generally discern the benign or malignant nature of a nodule. However, achieving a consensus on the reliable identification of the extent of LADC infiltration remains an ongoing challenge. In their investigation, He et al. (28) utilized preoperative predictive CT images to delve into the lymph node region for a more in-depth assessment of infiltration extent. Their findings revealed a differential diagnostic AUC value of 0.677 for MIA in the lymph node area, whereas the AUC value for the differential diagnosis of IAC reached 0.819. Zhou et al. (29) assessed the expression of programmed cell death ligand 1 (PD-L1) from pre-IAC to IAC stage, and the study findings revealed a positive correlation between PD-L1 expression and the aggressiveness, distinct pathological subtypes, or unfavorable biological behaviors of early-stage LADC. Given the well-established correlation between various levels of LADC infiltration through multiple techniques, their findings lend support to the significance of Micro-Raman diagnosis facilitated by deep learning classification models.
To ensure maximum clinical validity, the stability of the classification model was rigorously trained and tested on an individual patient population following a comprehensive diagnostic procedure for lesions in accordance with the Department of Pathology’s morphological and IHC classification. Validation of the utility of Raman datasets from patients with misdiagnoses in rapid pathology was conducted, affirming the potential of Raman spectroscopy as a biomarker for identifying the extent of LADC infiltration. Analysis of spectral distinctions among different tissues distinctly reveals diverse Raman patterns in pathological tissues with varying degrees of LADC infiltration, illuminating the intricate biochemical and metabolic changes during the transition from normal tissues to LADC. In recent years, deep learning has emerged as a pivotal tool in medical research. For instance, Shah et al. (30) utilized a Deep Ensemble 2D CNN model on lung cancer CT images to achieve a diagnostic accuracy of 95%. Meanwhile, Park et al. (31) developed a deep learning model for automated segmentation of lung cancer on [18F]FDG PET/CT, achieving a Dice similarity coefficient of 0.78 in the test set. In this work, Raman spectroscopy combined with deep learning CNN models can continuously capture and recognize spectral patterns of different pathological samples, correcting the errors of existing clinical intraoperative pathology diagnostic results.
In addition to showcasing its ability to identify the diagnostic features of LADC infiltration, this study emphasizes the seamless compatibility of the Micro-Raman technique with standard intraoperative diagnostic procedures in clinical settings. Unlike rapid freezing, which may result in section fragmentation and freezing artifacts, rendering significant portions of the tumor unsuitable for routine H&E evaluation and potentially underestimating disease severity (32), the Micro-Raman technique requires no sample processing. This allows for the direct diagnosis of suspicious areas, relying on the previously established pathology Raman database to achieve the initial diagnosis through classification models. Additionally, the cost of conventional Micro-Raman systems is comparable to that of pathology slide scanners. The dimensions of the commonly employed Raman metal-aluminum sheet are identical to those of pathology slides, making it a cost-effective and well-suited option for clinical use scenarios. The intraoperative collection of Raman spectra differs from laboratory settings, potentially leading to a reduced number of data points and a shorter diagnostic time. It is essential to highlight, from a technical standpoint, the significance of characterizing the “Raman-consistent” region around the tumor, as its localization may impact diagnostic accuracy when assessing the extent of the lesion (26).
Overall, Micro-Raman technique holds significant promise as a valuable complement to histopathologic research. However, its widespread clinical implementation necessitates the collection of a substantial number of clinical samples and the conduct of multi-geographic, multi-institutional studies. This study highlights the integration of Raman diagnostic methods with pathology assessments, noting limitations. Future research could enhance diagnostic accuracy by expanding Raman collecting coverage and refining algorithms. Additionally, exploring alternative Raman diagnostic modalities, such as SERS or the utilization of hand-held Raman probes for direct lesion diagnosis, can enhance the efficiency of acquiring large specimens or replace the SERS base to amplify spectral intensity (33-37). The integration of Micro-Raman technique into clinical diagnosis is a systematic and meticulous process, requiring the establishment of extensive Raman databases encompassing various tumor types and lesion extents (38). Furthermore, it involves developing a standardized acquisition process suitable for clinical settings and providing specialized training for physicians in rapid intraoperative Raman diagnosis to ensure the provision of reliable diagnostic services.
Conclusions
We present a comprehensive protocol for utilizing microscopic Raman techniques in conjunction with deep learning models for the diagnosis of LADCs, specifically targeting various degrees of infiltration. The study outcomes not only facilitate the classification of tissues with distinct levels of infiltration but also allow for the correction of tissues that have been inaccurately diagnosed during intraoperative rapid pathology. These findings establish a robust foundation for the clinical implementation of microscopic Raman technology as an intraoperative tool for diagnosing LADCs with diverse degrees of infiltrative characteristics.
Acknowledgments
Funding: This research received generous support from
Footnote
Reporting Checklist: The authors have completed the TRIPOD reporting checklists. Available at https://tlcr.amegroups.com/article/view/10.21037/tlcr-24-168/rc
Data Sharing Statement: Available at https://tlcr.amegroups.com/article/view/10.21037/tlcr-24-168/dss
Peer Review File: Available at https://tlcr.amegroups.com/article/view/10.21037/tlcr-24-168/prf
Conflicts of Interest: All authors have completed the ICMJE uniform disclosure form (available at https://tlcr.amegroups.com/article/view/10.21037/tlcr-24-168/coif). H.C. is from Nanjing Nuoyuan Medical Devices Co., Ltd., Nanjing, China. The other authors have no conflicts of interest to declare.
Ethical Statement: The authors are accountable for all aspects of the work in ensuring that questions related to the accuracy or integrity of any part of the work are appropriately investigated and resolved. The study was conducted in accordance with the Declaration of Helsinki (as revised in 2013). The study was approved by the Medical Ethics Committee of Nanjing Drum Tower Hospital, The Affiliated Hospital of Nanjing University Medical School (Approval Number: 2020-134-01), and informed consent was provided by all the patients.
Open Access Statement: This is an Open Access article distributed in accordance with the Creative Commons Attribution-NonCommercial-NoDerivs 4.0 International License (CC BY-NC-ND 4.0), which permits the non-commercial replication and distribution of the article with the strict proviso that no changes or edits are made and the original work is properly cited (including links to both the formal publication through the relevant DOI and the license). See: https://creativecommons.org/licenses/by-nc-nd/4.0/.
References
- DeSantis CE, Miller KD, Goding Sauer A, et al. Cancer statistics for African Americans, 2019. CA Cancer J Clin 2019;69:211-33. [Crossref] [PubMed]
- Siegel RL, Miller KD, Fuchs HE, et al. Cancer Statistics, 2021. CA Cancer J Clin 2021;71:7-33. [Crossref] [PubMed]
- de Biase D, Genestreti G, Visani M, et al. The percentage of Epidermal Growth Factor Receptor (EGFR)-mutated neoplastic cells correlates to response to tyrosine kinase inhibitors in lung adenocarcinoma. PLoS One 2017;12:e0177822. [Crossref] [PubMed]
- Moeller M, Schaedlich F, Schuette W. Retrospective Data Analysis of Patients With Metastatic Lung Adenocarcinoma With or Without KRAS-Mutation or TTF1-Expression. Cancer Control 2022;29:10732748221126949. [Crossref] [PubMed]
- Wei D, Sun D, Sirera R, et al. Overexpression of MRPL19 in predicting poor prognosis and promoting the development of lung adenocarcinoma. Transl Lung Cancer Res 2023;12:1517-38. [Crossref] [PubMed]
- Yeh YC, Kadota K, Nitadori J, et al. International Association for the Study of Lung Cancer/American Thoracic Society/European Respiratory Society classification predicts occult lymph node metastasis in clinically mediastinal node-negative lung adenocarcinoma. Eur J Cardiothorac Surg 2016;49:e9-e15. [Crossref] [PubMed]
- Kirita K, Ishii G, Matsuwaki R, et al. Identification of biological properties of intralymphatic tumor related to the development of lymph node metastasis in lung adenocarcinoma. PLoS One 2013;8:e83537. [Crossref] [PubMed]
- Chang C, Sun X, Zhao W, et al. Minor components of micropapillary and solid subtypes in lung invasive adenocarcinoma (≤ 3 cm): PET/CT findings and correlations with lymph node metastasis. Radiol Med 2020;125:257-64. [Crossref] [PubMed]
- Ettinger DS, Wood DE, Aisner DL, et al. Non-Small Cell Lung Cancer, Version 3.2022, NCCN Clinical Practice Guidelines in Oncology. J Natl Compr Canc Netw 2022;20:497-530. [Crossref] [PubMed]
- Singh U, Cui Y, Dimaano N, et al. Analytical validation of quantitative immunohistochemical assays of tumor infiltrating lymphocyte biomarkers. Biotech Histochem 2018;93:411-23. [Crossref] [PubMed]
- Chang C, Ruan M, Lei B, et al. Development of a PET/CT molecular radiomics-clinical model to predict thoracic lymph node metastasis of invasive lung adenocarcinoma ≤ 3 cm in diameter. EJNMMI Res 2022;12:23. [Crossref] [PubMed]
- Wilk AM, Kozłowska E, Borys D, et al. Radiomic signature accurately predicts the risk of metastatic dissemination in late-stage non-small cell lung cancer. Transl Lung Cancer Res 2023;12:1372-83. [Crossref] [PubMed]
- Liu S, Wang R, Zhang Y, et al. Precise Diagnosis of Intraoperative Frozen Section Is an Effective Method to Guide Resection Strategy for Peripheral Small-Sized Lung Adenocarcinoma. J Clin Oncol 2016;34:307-13. [Crossref] [PubMed]
- Deng H, Zhou J, Chen H, et al. Impact of lymphadenectomy extent on immunotherapy efficacy in postresectional recurred non-small cell lung cancer: a multi-institutional retrospective cohort study. Int J Surg 2024;110:238-252. [Crossref] [PubMed]
- Gajra A, Newman N, Gamble GP, et al. Effect of number of lymph nodes sampled on outcome in patients with stage I non-small-cell lung cancer. J Clin Oncol 2003;21:1029-34. [Crossref] [PubMed]
- Watanabe S. Lymph node dissection for lung cancer: past, present, and future. Gen Thorac Cardiovasc Surg 2014;62:407-14. [Crossref] [PubMed]
- Høgset H, Horgan CC, Armstrong JPK, et al. In vivo biomolecular imaging of zebrafish embryos using confocal Raman spectroscopy. Nat Commun 2020;11:6172. [Crossref] [PubMed]
- Guo S, Popp J, Bocklitz T. Chemometric analysis in Raman spectroscopy from experimental design to machine learning-based modeling. Nat Protoc 2021;16:5426-59. [Crossref] [PubMed]
- Hu S, Li H, Chen C, et al. Raman spectroscopy combined with machine learning algorithms to detect adulterated Suichang native honey. Sci Rep 2022;12:3456. [Crossref] [PubMed]
- McGregor HC, Short MA, McWilliams A, et al. Real-time endoscopic Raman spectroscopy for in vivo early lung cancer detection. J Biophotonics 2017;10:98-110. [Crossref] [PubMed]
- Qi Y, Yang L, Liu B, et al. Highly accurate diagnosis of lung adenocarcinoma and squamous cell carcinoma tissues by deep learning. Spectrochim Acta A Mol Biomol Spectrosc 2022;265:120400. [Crossref] [PubMed]
- Park J, Hwang M, Choi B, et al. Exosome Classification by Pattern Analysis of Surface-Enhanced Raman Spectroscopy Data for Lung Cancer Diagnosis. Anal Chem 2017;89:6695-701. [Crossref] [PubMed]
- Qian K, Wang Y, Hua L, et al. New method of lung cancer detection by saliva test using surface-enhanced Raman spectroscopy. Thorac Cancer 2018;9:1556-61. [Crossref] [PubMed]
- Chon H, Lee S, Son SW, et al. Highly sensitive immunoassay of lung cancer marker carcinoembryonic antigen using surface-enhanced Raman scattering of hollow gold nanospheres. Anal Chem 2009;81:3029-34. [Crossref] [PubMed]
- Frost N, Zhamurashvili T, von Laffert M, et al. Pemetrexed-Based Chemotherapy Is Inferior to Pemetrexed-Free Regimens in Thyroid Transcription Factor 1 (TTF-1)-Negative, EGFR/ALK-Negative Lung Adenocarcinoma: A Propensity Score Matched Pairs Analysis. Clin Lung Cancer 2020;21:e607-21. [Crossref] [PubMed]
- Grosset AA, Dallaire F, Nguyen T, et al. Identification of intraductal carcinoma of the prostate on tissue specimens using Raman micro-spectroscopy: A diagnostic accuracy case-control study with multicohort validation. PLoS Med 2020;17:e1003281. [Crossref] [PubMed]
- Siegel RL, Miller KD, Jemal A. Cancer Statistics, 2017. CA Cancer J Clin 2017;67:7-30. [Crossref] [PubMed]
- He W, Guo G, Du X, et al. CT imaging indications correlate with the degree of lung adenocarcinoma infiltration. Front Oncol 2023;13:1108758. [Crossref] [PubMed]
- Zhou J, Lin H, Ni Z, et al. Expression of PD-L1 through evolution phase from pre-invasive to invasive lung adenocarcinoma. BMC Pulm Med 2023;23:18. [Crossref] [PubMed]
- Shah AA, Malik HAM, Muhammad A, et al. Deep learning ensemble 2D CNN approach towards the detection of lung cancer. Sci Rep 2023;13:2987. [Crossref] [PubMed]
- Park J, Kang SK, Hwang D, et al. Automatic Lung Cancer Segmentation in [18F]FDG PET/CT Using a Two-Stage Deep Learning Approach. Nucl Med Mol Imaging 2023;57:86-93. [Crossref] [PubMed]
- Mahadevappa A, Nisha TG, Manjunath GV. Intra-operative Diagnosis of Breast Lesions by Imprint Cytology and Frozen Section with Histopathological Correlation. J Clin Diagn Res 2017;11:EC01-6. [Crossref] [PubMed]
- Rajput S, Pink D, Findlay S, et al. Application of Surface-Enhanced Raman Spectroscopy to Guide Therapy for Advanced Prostate Cancer Patients. ACS Sens 2022;7:827-38. [Crossref] [PubMed]
- Sun C, Zhang L, Zhang R, et al. Facilely synthesized polydopamine encapsulated surface-enhanced Raman scattering (SERS) probes for multiplex tumor associated cell surface antigen detection using SERS imaging. RSC Advances 2015;5:72369-72. [Crossref]
- Dai X, Fu W, Chi H, et al. Optical tweezers-controlled hotspot for sensitive and reproducible surface-enhanced Raman spectroscopy characterization of native protein structures. Nat Commun 2021;12:1292. [Crossref] [PubMed]
- Lin D, Qiu S, Huang W, et al. Autofluorescence and white light imaging-guided endoscopic Raman and diffuse reflectance spectroscopy for in vivo nasopharyngeal cancer detection. J Biophotonics 2018;11:e201700251. [Crossref] [PubMed]
- Bergholt MS, Lin K, Wang J, et al. Simultaneous fingerprint and high-wavenumber fiber-optic Raman spectroscopy enhances real-time in vivo diagnosis of adenomatous polyps during colonoscopy. J Biophotonics 2016;9:333-42. [Crossref] [PubMed]
- Traynor D, Behl I, O’Dea D, et al. Raman spectral cytopathology for cancer diagnostic applications. Nat Protoc 2021;16:3716-35. [Crossref] [PubMed]