Molecular and immune characterization of Chinese early-stage non-squamous non-small cell lung cancer: a multi-omics cohort study
Highlight box
Key findings
• This study comprehensively profiled the genomic, transcriptomic, and T-cell receptor repertoire spectrums of Chinese early-stage non-squamous non-small cell lung cancer (Ns-NSCLC), which may affect prognosis and influence the crosstalk between tumor and the immune system, unveiling novel cancer biological meanings.
What is known and what is new?
• Several molecular and immune characteristics of non-small cell lung cancer (NSCLC) have been identified previously, while their associations with prognoses were unclear.
• We innovatively characterized the multi-omics features of Chinese early-stage Ns-NSCLC and interrogated the relationships between various alterations, either alone or in combination, with prognosis. A robust multi-omics prognostic model was also established, demonstrating the best performer in integrating clinical and molecular features.
What is the implication, and what should change now?
• The reported biological underpinnings and candidate biomarkers of Chinese Ns-NSCLC may contribute to therapy and prognosis development.
• Prospective and cross-cohort validation examining the associations or causalities between the findings and clinical outcomes are requisite for better clinical practice.
Introduction
Lung cancer (LC) is among the most diagnosed cancers and the predominant cause of cancer-related death globally (1). Despite a dramatic improvement in LC prognoses with the advent of targeted therapies, especially in lung adenocarcinoma (LUAD), more advances are restricted by the poor comprehension of its pathogenesis (2). Non-small cell lung cancer (NSCLC) is the major subtype of LC, and a remarkable increase in detecting early-stage non-squamous NSCLC (Ns-NSCLC) was observed with the growing application of low-dose computed tomography screening (3,4). While radical resection is the primary treatment option for early-stage Ns-NSCLC, around 30% of the patients die of relapse within 5 years (5). Therefore, it is essential to comprehensively characterize the early-stage Ns-NSCLC by multi-dimension approaches, including genomic, transcriptomic, proteomic, and immunological profiling, which may help risk stratification and personalized strategy development.
Diverse gene mutations, fusions, copy number variations (CNVs), and epigenetic variations have been depicted with the development of the next-generation sequencing (NGS) technique, accentuating the intratumoral and intertumoral heterogeneity of NSCLC (6,7). For instance, KRAS and EGFR mutations are commonly detected in smoker and non-smoker LUAD, respectively (8). Meanwhile, mutations in TP53 and PIK3CA are most frequently identified in lung squamous cell cancer (LUSC) (9). Moreover, Kadara and colleagues reported that mutation in SETD2 correlated with poor prognosis, and EGFR and PIK3CA mutations predicted unfavorable responses to adjuvant chemotherapy of early-stage LUAD in the American population (10). Choi et al. further found that MLL2 mutation correlated with unfavorable recurrence-free survival of American early-stage LUSC patients (11). Although these variations have been implicated in the classical cancer signal pathways and have deepened our knowledge of the molecular pathogenesis of NSCLC, their associations with prognoses and personalized therapeutic strategies in early-stage NSCLC, especially in the Chinese population, are still unclear.
At the transcriptomic level, a previous study has classified three predominant LUAD subtypes, including the terminal respiratory unit, proximal proliferative and proximal inflammatory subcluster, indicative of the potential for precise treatment (12). Other groups have also established gene expression-based models, like immune-related gene signature, to predict prognosis and treatment response (13,14), underscoring the important role of the immune microenvironment in LC pathobiology.
The essential role of the immune components in the tumor microenvironment (TME) is also accentuated by recent successes describing durable responses to immune checkpoint inhibitors (ICIs) of NSCLC patients (15). The biological underpinnings of successful immunotherapy are precisely recognizing neoantigen peptides by clonally proliferative T-cell receptors (TCRs) and subsequent activation of tumoricidal effects by the host immune system (16). TCR repertoire represents the strength and breadth of T-cell response, undertaking a vital role in antitumor immunity across various cancers (17). Recent studies have demonstrated the predictive and prognostic roles of peripheral TCR repertoire in ICIs therapies of advanced NSCLC (18,19). Nonetheless, the TCR repertoire landscape concerning different genetic alterations and its prognostic significance of early-stage Ns-NSCLC remains to be uncovered.
In the present study, we comprehensively profiled the genomic, transcriptomic, and TCR repertoire spectrums of Chinese early-stage Ns-NSCLC, accentuating the unique biological heterogeneity and biomolecular network. Rich annotation of this cohort also enabled us to interrogate the relationships between various alterations, either alone or in combination, with prognosis. Eventually, a robust multi-omics prognostic model was established, demonstrating the best performer integrating clinical and molecular features. We present this article in accordance with the TRIPOD reporting checklist (available at https://tlcr.amegroups.com/article/view/10.21037/tlcr-23-800/rc).
Methods
Study population and sample collection
Radically resected Ns-NSCLC, matched blood samples, and normal lung tissues (n=76 pairs) were collected at the First Affiliated Hospital of Guangzhou Medical University (GZMU1H) between 2012 and 2015. Eligible patients were stage IA–III pathologically confirmed Ns-NSCLC and no personal history of cancer (20). Patients who (I) underwent neoadjuvant therapy; (II) did not have R0 excision; and (III) had multiple primary LCs were excluded. Informed consent of included patients was obtained, and this research was approved by the GZMU1H Ethics Committee (approval No. KLS-17-03). The study was conducted in accordance with the Declaration of Helsinki (as revised in 2013) (21).
Multi-omics detection of the samples was completed at Yucebio Technology Co., Ltd. (Shenzhen, China). Details of the kits and software used are available in Tables S1,S2.
Processing whole-exome sequencing (WES) data
Library preparation and sequencing
We extracted genomic DNAs from formalin-fixed paraffin-embedded (FFPE) slides and the matched blood controls (BCs) by QIAamp DNA FFPE Tissue Kit and DNeasy Blood and Tissue Kit (Qiagen, USA), respectively, which were subsequently quantified by Qubit 3.0 via the dsDNA HS Assay Kit (ThermoFisher Scientific, USA). Library preparations were conducted by KAPA Hyper Prep Kit (KAPA Biosystems, USA). Target enrichment was performed by the xGen Exome Research Panel and Hybridization and Wash Reagents Kit (Integrated DNA Technologies, USA). Sequencing was carried out on the Illumina HiSeq4000 platform (Illumina, USA), and the average sequencing depths were 140× and 64× for tumors and normal BCs, respectively.
Data processing of exome libraries
We filtered out the N rate beyond 10% and low-quality reads by SOAPnuke. The Burrows-Wheeler Alignment tool was utilized to align the clean reads to the human reference genome based on the UCSC hg19. Conversing, sorting, and indexing alignment data was performed via SAMtools. SAMBLASTER was used to mark the duplicates for decreasing biases.
Copy number variants and intratumoral heterogeneity (ITH) analyses
The VarScan software was employed to identify somatic mutations, including small insertions and deletions (indels) and single nucleotide variants. Aiming at retrieving false-negative mutations and removing false-positive mutations, strict selection was conducted by our in-house variant detection tool. Subsequently, each mutation was annotated by the SnpEff software. CNVs were determined by exome-wide profile comparisons between tumors and matched BCs via CNVkit. The cancer cell fraction of mutations was calculated by PyClone, with the tumor purity computed by All-FIT. The proportions of subclone mutations to all mutations were defined as ITH (22).
Human leukocyte antigen (HLA) typing and loss of heterozygosity (LOH) analysis
HLA typing of the paired tumor and BCs was estimated from WES data via POLYSOLVER and Bwakit software. Then LOH in the HLA approach was employed, and LOH was determined if (I) a copy number <0.5 and (II) imbalanced allelic identified by P<0.01 by the paired Student’s t-test between the two distributions (23).
Genomic biomarker analyses
Tumor mutational burden (TMB) was defined as the number of non-synonymous somatic mutations per mega-base (Mb) of the genome interrogated. Through in-house software centering on mutant amino acids, all indels and non-synonymous mutations were translated into 21-mer peptide sequences, which were subsequently utilized to create a 9- to 11-mer peptide by the sliding window method for predicting major histocompatibility complex (MHC) class I binding affinity. The NetMHCpan software was employed for predicting the binding intensity of mutant peptides to patient-specific HLA alleles. The estimated binding strength of a peptide with any HLA allele was selected if half-maximal inhibitory concentration (IC50) <500 nM. Consequently, neoantigen was calculated as multifarious selective peptides generated from the same mutation. Tumor neoantigen burden (TNB) was determined by the number of such peptides per Mb of the genome interrogated. The whole chromosomal instability (wCIN) score was utilized to assess the copy number burden by the allele-specific copy number analysis of tumors (ASCAT) approach (24). Gene mutation pathway analyses were conducted as previously described (25).
RNA sequencing workflow
We isolated the total RNA of tumor samples from the tumor tissues via RNeasy Plus Universal Kit (Qiagen, USA). Utilizing the QubitTM RNA HS Assay Kit, the concentrations of the extracted RNA were then quantified. The integrity and purity of the extracted RNA were tested by the RNA Cartridge kit of the Qseq100 Bio-Fragment Analyzer (Bioptic, China) and the Take3 kits (BioTek, USA), respectively. We then generated the RNA-seq libraries by the VAHTS mRNA-seq V3 Library Prep Kit (Vazyme, China). Eventually, the libraries were sequenced on the NextSeq 550AR (Illumina, USA) with 150-bp paired-end reads.
Gene expression analysis
Low-quality adapters and reads from raw RNA sequencing data were filtered by trim galore. The read counts and transcripts per million values were analyzed via Kallisto based on the Gencode database (26). Identification of differentially expressed genes (DEGs) was conducted based on the read counts matrix, of which DEGs with P value <0.05 and |log2foldchange| >1 were considered significant. Gene set enrichment and variation analysis based on Gene Ontology and Kyoto Encyclopedia of Genes and Genomes datasets were utilized to interrogate the pathway activity differences between groups (27). Enriched pathways with Bonferroni-corrected Padj<0.05 were considered significant (28).
TCR sequencing
Isolating from peripheral blood mononuclear cells (PBMCs) by RNeasy Plus Mini Kit, the concentration of total RNA was detected by the Take3 kit. Via iRepertoire Short Read iR-Profile Reagent System HTBI-vc (iRepertoire, USA), total RNA was synthesized into the cDNA library. Sequencing was conducted on the NextSeq 550AR system with 150-bp paired-end reads. We then trimmed Fastq reads concerning their low-quality 3' ends bases. Integration of processed pair-end reads was executed based on overlapped alignment with the adjusted Needleman-Wunsch method. Identification of the CDR3 sequences of V-D-J gene fragments with reference sequences from the IMGT database (29) was conducted on the MiXCR (30). Evaluation of the immune repertoire sequencing was carried out on the VDJtools (31). Additionally, we executed frequency-based adjustments on samples. The chaoE, shannonWiener, Simpson, and d50 indexes were utilized to assess the TCR clone diversity.
Estimation of cellular abundance by bulk RNA‑seq data
CIBERSORT, an approach assessing the contents of 22 kinds of immune cell populations, including natural killer (NK) cell, B cell, T cell, dendritic cell (DC), monocyte, macrophage, mast cell, neutrophil, eosinophil, and their subclusters, was used to uncover the immune infiltrating spectrums by bulk RNA-seq data (32). MCP-counter, a versatile computational approach which robustly quantifies the absolute abundance of two stromal and eight immune cell populations from bulk transcriptomic data, was also exploited (33).
Construction and validation of the multi-omics prognostic model
The multi-omics prognostic model was constructed and validated according to the TRIPOD checklist (34). The machine-learning (ML) framework was built on Python (version 3.9.13) using the following libraries: scikit-learn (version 1.2.2), pandas (version 1.5.3), scipy (version 1.9.1), and imbalanced-learn (version 0.10.1).
We performed combinations of different omics biomarkers: (I) Clinical features + DNA; (II) Clinical features + RNA; (III) Clinical features + TCR; (IV) Clinical features + DNA + RNA; (V) Clinical features + DNA + RNA + TCR; (VI) DNA + RNA; (VII) DNA + RNA + TCR, resulting in seven different datasets. Seven ML approaches, including decision tree classifier (DTC), extra tree classifier (ETC), gradient boosting classifier (GBC), Gaussian process classifier (GPC), K-nearest neighbors (KNN), random forest (RF), and support vector machine (SVM) were adopted to screen out the robust prognosticators and develop the multi-omics prognostic model.
Each dataset (n=76) was divided into a training cohort (n=60, 80%) and an internal testing cohort (n=16, 20%) via stratified sampling (35). For the data input to the KNN model, we used Z-score normalization to scale the original data and transform it into a standard normal distribution. This process eliminated dimensionality differences between features, balancing the impact of various features on the model. For the data input to the GPC model, we applied the Synthetic Minority Over-sampling Technique (SMOTE) preprocessing method. SMOTE approach generates synthetic samples by computing the differences between minority class samples and their nearest neighbors, achieving a balance of sample categories in the dataset. The remaining models were trained via the original and unprocessed data.
Grid search and five-fold cross-validation were employed to perform hyperparameter optimization and model performance evaluation on five algorithms except for KNN and GPC. Specifically, the grid search method exhaustively searched all possible combinations within a given parameter space. Table S3 provides detailed information on hyperparameter combinations per model. In five-fold cross-validation, we divided the training set into five equal-sized subsets. We selected four subsets as the training set and the remaining one subset as the validation set. By performing this process five times, with a different subset as the validation set per trial, we obtained five sets of training results. We compared the performance of each parameter combination on training metrics to determine the best parameter combination, optimizing model performance and preventing overfitting. Accuracy (ACC) and area under the curve (AUC) are the major metrics to measure the performance of the models. Other evaluation indices like precision, recall, and F1-score were also employed.
After training on all datasets, we selected the more accurate and stabler tree-based algorithms: RF and ETC. Gini importance analysis was subsequently used to assess the importance of specific features within each dataset. The Gini index measured the purity or impurity of samples within a node. In binary classification issues, the calculation formula for the Gini index is given by , where and represents the proportions of samples belonging to two different classes within the node. Gini importance measures the importance of a feature by calculating the reduction in the average Gini index for each feature over all nodes of the decision tree. Given that RF and ETC are both ensemble methods of decision trees, we aggregated the Gini importance of each corresponding feature across all trees, resulting in the final Gini index.
External dataset utilized for analyses
The genomic profiles, mRNA expression, and clinical characteristics data of LUAD samples from The Cancer Genome Atlas (TCGA)-LUAD cohort were downloaded from the UCSC Xena (https://xena.ucsc.edu/) database (acquisition date, 2023/1/11).
Statistical analysis
The Mann-Whitney U test, Wilcoxon t-test, and Kruskal-Wallis test were employed to compare the differences of continuous and categorical data. Spearman rank correlation test was used to evaluate the correlation between two variables, and the |correlation coefficient| >0.5 was regarded as significant. Survival differences between two groups were evaluated by the Kaplan-Meier approach and the log-rank test. Univariate and multivariate Cox regression analyses correcting for age, sex, and clinical tumor staging (cTNM) were utilized to assess the prognostic effect of signature. The X-tile software was employed to determine the optimal cutoff point of continuous variables (36). The receiver operating characteristic (ROC) curves and the corresponding predictive ACC were analyzed via the AUC values. DeLong’s test was utilized to compare the predictivity ACC, evaluated by AUCs, between different methods. Statistical analyses and plot generation were performed in GraphPad Prism (version 8.0), SPSS (version 25.0), and R (version 4.0.4). P value <0.05 of statistical analyses was regarded as significant. Data were presented by mean ± standard error or by box and whisker plots.
Results
Baseline characteristics of the included patients
A total of 76 patients, with the majority of LUAD samples (n=68, 89%), meeting the inclusion criteria were recruited (Table 1). The median age was 60 (ranging from 36 to 86) years, and 34.2% of the patients were ever-smokers. Twenty-six patients relapsed during the follow-up period, and the recurrent rates of IA, IB, II, and III samples were 11.54%, 15.38%, 23.08%, and 50%, respectively.
Table 1
Characteristics | Disease-free | Relapse | P value | |||
---|---|---|---|---|---|---|
Number | Percentage (%) | Number | Percentage (%) | |||
Gender | 0.23 | |||||
Female | 27 | 54 | 10 | 38.46 | ||
Male | 23 | 46 | 16 | 61.54 | ||
Smoking status | 0.45 | |||||
Never | 33 | 66 | 17 | 65.38 | ||
Former | 10 | 20 | 3 | 11.54 | ||
Current | 7 | 14 | 6 | 23.08 | ||
Stage | 0.003 | |||||
IA | 16 | 32 | 3 | 11.54 | ||
IB | 15 | 30 | 4 | 15.38 | ||
II | 12 | 24 | 6 | 23.08 | ||
III | 7 | 14 | 13 | 50 | ||
EGFR | 0.09 | |||||
Mutant | 24 | 48 | 18 | 69.23 | ||
Wild-type | 26 | 52 | 8 | 30.77 |
Quality control of the multi-omics data
After appropriate sample quality control, a total of 76 paired tumor-control WES data, 71 paired tumor-paratumor RNA-sequencing data, and 139 cases of TCR-sequencing data of tumor-paratumor-blood samples were eligible for downstream analyses (Figure 1A).
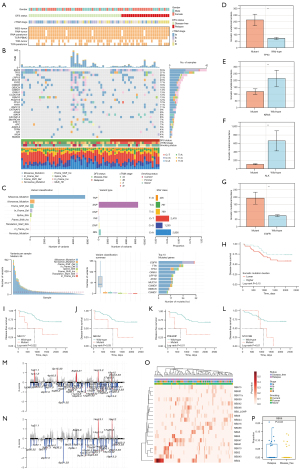
Molecular profiling of early-stage Ns-NSCLC
The mutation landscape of early-stage Ns-NSCLC was depicted, showing that EGFR was the most frequently mutant gene (55%), followed by TP53 (37%) and TTN (26%) (Figure 1B), accordant with the recent report (37). A mean of 128 mutations was observed, and ever-smokers harbored significantly more somatic mutations than never-smokers (mean: 238 vs. 70, P<0.001), consistent with previous literature (38). The most common base substitutions were C > A and C > T transversions, and they were more frequently detected in ever than never smokers (P<0.01) (Figure 1C).
We also compared the mutation landscape between the present and the TCGA-LUAD cohorts (available at https://xena.ucsc.edu/) (Figure S1A). Among the top ten mutant genes in our cohort, the mutation frequencies of EGFR (55% vs. 13%, P<0.001), TP53 (37% vs. 50%, P=0.03), TTN (26% vs. 48%, P=0.02), LRP1B (21% vs. 34%, P=0.02), MUC16 (20% vs. 42%, P<0.001), RYR2 (17% vs. 37%, P<0.001), and CSMD3 (16% vs. 40%, P<0.001), were significantly different from Chinese and Caucasian patients (Figure S1B-S1H), underscoring LC genome diversity between ethnicities (38).
Ns-NSCLC with mutant TP53 (Figure 1D), KRAS (Figure 1E), and ALK (Figure 1F) displayed higher somatic mutational burden than wild-type ones (P<0.01). On the contrary, the mutation in EGFR (Figure 1G) predicted lower somatic mutational burden than wild-type ones (P<0.01). Moreover, early-stage Ns-NSCLC harboring higher somatic mutational burden exhibited a trend of better DFS than fewer ones (Figure 1H). Higher mutation frequencies of ASXL1, ANK3, FGD5, FLT4, MYO18B, MYO3A, and SELENOV were observed in patients with recurrence than without.
Mutations in MUC17 [hazard ratio (HR) 3.252, 95% confidence interval (CI): 1.118–9.463, P=0.02] (Figure 1I), ABCA2 (HR 3.363, 95% CI: 1.007–11.232, P=0.03) (Figure 1J), PDE4DIP (HR 3.647, 95% CI: 1.250–10.637, P=0.01) (Figure 1K), and MYO18B (HR 3.502, 95% CI: 1.201–10.211, P=0.01) (Figure 1L) were found to predict significantly shorter disease-free survival (DFS) in the univariate Cox setting. Nonetheless, mutations in these four genes did not correlate with prognosis in the TCGA-LUAD cohort from Caucasian populations, suggesting genomic differences (Figure S2A-S2D). Moreover, the transcriptomic spectrums were significantly different between mutant and wild-type populations. The DEGs of these four genes were enriched into up-regulation of the cell cycle process and down-regulation of epithelial cell development, representing malignant transformation of cells (Figure S2E-S2L). Intriguingly, the mutation in MUC17 also correlated with higher neutrophil-mediated immunity of Ns-NSCLC.
As for somatic copy number alteration (sCNA), more frequent deletion (Del) than amplification (Amp) genes were observed generally. Significant Dels in 8q24.3, 7q22.1 (containing COL1A2 gene), and 15q25.2 and Amps in the 4p16.3 (encoding FGFR3 gene), 5p15.33, and 8q24.21 (encoding MYC gene) were found in patients without relapse (Figure 1M). Significant Amps in 8q24.3, 14q13.1, and 14q11.2, and Del in 3p21.1 were highlighted in early-stage recurrent Ns-NSCLC (Figure 1N). The frequency of smoking-related single-base substitution 4 (SBS4) was the highest among all SBS signatures (Figure 1O) (39). Moreover, the frequency of mismatch-repair-deficient-related SBS6 signature was higher in patients with relapse than without (Figure 1P) (40).
Landscape of genomic biomarkers and their prognostic effects
The median values of TMB, TNB, and wCIN-score were 2.065 mut/Mb, 0.850 na/Mb, and 0.010, respectively (Figure 2A). HLA-LOH was discovered in 28.9% (22 of 76) of Ns-NSCLC patients and was more frequently identified in ever-smokers than never-smokers (P=0.01), consistent with previous findings (23). The incidence of lost alleles was highest in HLA-A (14.5%) and lowest in HLA-C (2.6%).
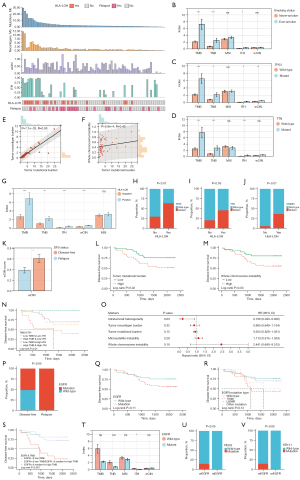
TMB and TNB were significantly higher in ever-smokers than never-smokers (P<0.001), indicative of a higher mutational burden (Figure 2B). Moreover, TP53 mutation was associated with significantly higher TMB, TNB, ITH, and wCIN-score (P<0.05) (Figure 2C). Likewise, TTN mutation correlated with higher TMB and TNB (P<0.001), indicative of higher immunogenicity (Figure 2D) and has been reported to be a potential predictor of ICIs efficiency (41). Additionally, strong correlations of TMB-TNB (R=0.93, P<0.001) (Figure 2E) and TMB-wCIN (R=0.40, P<0.001) (Figure 2F) were observed.
Significantly higher TMB, TNB, ITH, and wCIN-score (P<0.001) were discovered in patients with HLA-LOH than without (Figure 2G). Moreover, the mutation frequencies of TP53 (36.3% vs. 29.6%, P<0.01) (Figure 2H), TTN (45.4% vs. 20.3%, P<0.05) (Figure 2I), and CSMD1 (36.3% vs. 5.5%, P<0.01) (Figure 2J) were significantly higher in HLA-LOH positive than the negative group.
A higher frequency of wCIN was observed in stage II and III patients with relapse than without (Figure 2K). No significant associations were found between TMB, TNB, ITH, or HLA-LOH and relapse status and disease stages. Higher TMB (log-rank test, P=0.02) (Figure 2L) and wCIN-score (log-rank test, P=0.03) (Figure 2M) predicted unfavorable DFS. Patients with low-TMB & high-ITH have significantly better prognoses than those with high-TMB & low-ITH (log-rank test, P=0.01) (Figure 2N). Multivariate Cox regression analysis adjusting for age, sex, and cTNM stages indicated that ITH was an independent prognostic factor (HR 0.196, 95% CI: 0.043–0.900, P=0.03), possibly because most included patients being early-stage (Figure 2O).
EGFR mutation status-related analyses
Recurrence probability was significantly higher in stage I patients with EGFR mutation than without (P<0.05) (Figure 2P). A trend of unfavorable prognosis of patients with EGFR mutation was observed, whereas without statistical significance (Figure 2Q). In descending order, the DFS days concerning EGFR mutation status were wild-type, L858R, other mutation types, and 19Del (Figure 2R). Moreover, patients with EGFR mutation and median-to-high-TMB correlated with significantly shorter DFS than those with low-TMB and without EGFR mutation (log-rank test, P<0.001) (Figure 2S). Higher TMB, TNB, and ITH were found in wild-type EGFR versus mutant EGFR individuals (Figure 2T). Additionally, the mutation frequencies of PEG3 (Figure 2U) and STK11 (Figure 2V) were significantly higher in wild-type than in mutant EGFR patients.
Transcriptional landscape of early-stage Ns-NSCLC
Significantly higher expression levels of KDM5D, NLGN4Y, and PRAME were found in ever-smokers than never-smokers (Figure 3A), which have been reported to correlate with tobacco use (42-44). Enhanced DNA repair, replication and methylation pathway activities and attenuated cell adhesion and epithelium development pathway activities were further identified in ever-smokers (Figure 3B), indicative of the carcinogenic effects of tobacco (45). Altogether, one hundred and forty-three DEGs were identified in patients with recurrence than without (Figure 3C), which were associated with upregulated mitotic pathway activity and downregulated immune-related pathways activity (Figure 3D).
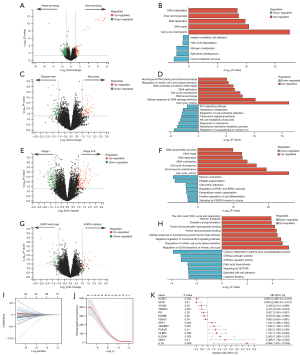
A total of 140 up-regulated genes and 251 down-regulated genes were discovered in stage II–III compared with stage I samples (Figure 3E). The DEGs were enriched into up-regulation of cell growth processes like cell cycle and DNA replication pathways, whereas downregulated cell adhesion and differentiation pathways were observed (Figure 3F), indicating the augmented invasive capacity of tumor cells (46). A total of 106 DEGs were found in EGFR mutation than wild-type patients (Figure 3G), which were also correlated with augmented mitotic cell cycle pathway activity and muted cell adhesion activity (Figure 3H), suggesting higher invasion and metastasis capacity.
We subsequently sought to evaluate the prognostic effects of these genes. Firstly, 2,483 immune-related genes were extracted referring to the ImmPort database (47), among which 1,443 genes were differentially expressed between tumor nest (TN) and paratumor tissue in our cohort (Table S4). Univariate Cox regression analysis further identified 65 genes with prognostic effects, which were used as candidate genes (Table S5). Subsequently, the least absolute shrinkage and selection operator (LASSO) Cox regression model was employed to select the robust prognosticators among the candidate factors (Figure 3I,3J). Eventually, a total of 14 genes, including KLRD1, NCR1, ICAM2, TRIM22, PI3, INHBB, IGHG3, SPP1, CRABP2, PLAUR, ULBP2, IL31RA, GDF5, and IL1A were screened out (Figure 3K). Up-regulation of KLRD1, NCR1, ICAM2, TRIM22, PI3, INHBB, and IGHG3 was associated with better DFS, whereas up-regulation of SPP1, CRABP2, PLAUR, ULBP2, IL31RA, GDF5, and IL1A predicted the unfavorable DFS. Up-regulation of CRABP2 (HR 1.163, 95% CI: 1.010–1.339, P=0.03), ULBP2 (HR 1.552, 95% CI: 1.049–2.296, P=0.02), IL31RA (HR 1.809, 95% CI: 1.096–2.985, P=0.02), and IL1A (HR 5.438, 95% CI: 1.626–18.184, P=0.006) also independently correlated with dismal DFS in the multivariate Cox setting.
Immune infiltration landscape of early-stage Ns-NSCLC
In the tumor tissues, the CIBERSORT approach showed that infiltration of activated memory CD4+ T cells increased with TMB (Figure 4A) while infiltrating naïve B cells increased with TNB (Figure 4B). Lower memory B cell, neutrophil and higher plasma cell infiltrates were found in patients with HLA-LOH than without (Figure 4C). Infiltration of CD8+ T cells, activated memory CD4+ T cells, and naïve B cells was significantly lower in patients with EGFR mutation than without (Figure 4D), indicating impaired antitumor immunity. Lower infiltrating levels of CD8+ T cell and higher Treg infiltrates were observed in patients with relapse than without (Figure 4E). Enriched plasma cells, CD8+ T cells, and resting mast cells in TN were observed in patients without relapse. Compared with stage II–III, TME in stage I was featured by higher infiltrating immune effector cells like plasma cells, CD8+ T cells, resting CD4+ memory T cells, and lower infiltrating Tregs (Figure 4F), indicative of the “immune-hot” TME. Similar findings were validated by the MCP-counter approach (Figure S3A-S3F).

In the paratumor tissues, significantly lower CD8+ T cell and activated NK cell infiltrates were found in patients with relapse than without. Moreover, EGFR mutation correlated with higher Tregs and lower CD8+ T cells infiltration, indicating underactive TME. Interestingly, the infiltrating levels of M0 macrophages and resting DC increased with TMB and TNB levels, suggesting higher immunogenicity (Figure S3G-S3L).
The immune infiltration landscapes in the tumor and paratumor tissues were subsequently compared. Higher plasma cell, Treg, and M2 macrophage infiltrates were discovered in the tumor tissue. In contrast, higher infiltrating levels of CD8+ T cells, activated NK cells, and activated CD4+ memory T cells were found in the paratumor tissue (Figure S3M). Overall, immune-enriched TME was presented in early-stage Ns-NSCLC, and paratumor tissue seemed to hold higher immunoactivity than in tumor tissue.
Eventually, an unsupervised K-means clustering method via the Canberra metric was utilized to characterize the immune landscape of early-stage Ns-NSCLC (38). The TME could be generally classified into two major groups: tumor-inflamed and stroma-inflamed (Figure 4G). The stroma-inflamed group was characterized by high infiltrates of immune effector cells in paratumor tissue, including activated NK cells, activated CD4+ memory T cells, and CD8+ T cells, indicative of active immune response. Inversely, the tumor-inflamed group contained enriched M2 macrophages, Tregs and follicular helper T cells in tumor tissue, suggesting an immunosuppressive milieu.
TCR repertoire diversity spectrum
The mean values of observedDiversity, chaoE, d50Index, and normalizedShannonWienerIndex were utilized to compare the TCR repertoire diversity. The TCR repertoire was highest in PBMC, followed by tumor and paratumor tumor tissues (Figure 5A,5B). As for the TCR repertoire of PBMCs, decreased d50Index was observed in stage II–III compared with stage I samples (Figure 5C). Mutation in TP53 indicated higher normalizedShannonWienerIndex, suggesting enhanced TCR diversity (Figure 5D). Inversely, mutations in EGFR and ALK correlated with muted TCR diversity (Figure 5E,5F). Insignificant differences in TCR diversity were found between different mutation types of EGFR.
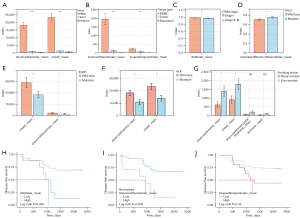
As for the TCR repertoire of tumor tissue, augmented TCR diversity was demonstrated in ever-smokers than non-smokers (Figure 5G). Concerning the TCR repertoire of paratumor tissue, higher d50Index predicted significantly longer DFS (log-rank test, P=0.005) (Figure 5H), whereas higher normalizedShannonWienerIndex correlated with unfavorable prognosis (log-rank test, P=0.004) (Figure 5I). Higher inverseSimpsonIndex predicted significantly longer DFS (log-rank test, P=0.03) than lower ones (Figure 5J). Nevertheless, none of the above metrics used to estimate TCR repertoire diversity significantly correlated with DFS in the multivariate Cox analysis.
Prognostic effects of the individual omics/biomarker
The cTNM stage (AUC 0.727, 95% CI: 0.606–0.847) independently showed predictive capacity of DFS (Figure 6A). Among the genomic features, wCIN (AUC 0.630, 95% CI: 0.499–0.762) showed the highest predictive AUC, followed by TMB (AUC 0.588, 95% CI: 0.456–0.719) and microsatellite instability (MSI) (AUC 0.558, 95% CI: 0.420–0.695) (Figure 6B-6H). Among the transcriptomic characteristics, KLRD1 (AUC 0.730, 95% CI: 0.616–0.844) showed the highest effectiveness, followed by NCR1 (AUC 0.701, 95% CI: 0.573–0.830) and PI3 (AUC 0.701, 95% CI: 0.577–0.824). None of the AUC values of TCR repertoire biomarkers exceeded 0.6, with the highest of d50Index (AUC 0.590, 95% CI: 0.446–0.733). Consequently, individual omics/biomarker held limited performance to distinguish patients more prone to relapse (Figure S4).
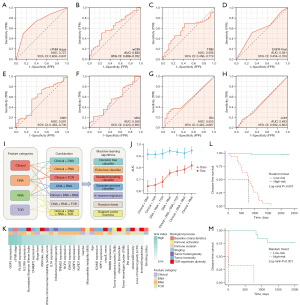
Development of the multi-omics prognostic signature
Due to the unsatisfied performance of the individual omics/biomarker, we further developed the multi-omics prognostic signature (Figure 6I). We discovered that the integration of genomic, transcriptomic, clinical, and TCR repertoire features achieved stable and accurate efficacy across the seven methods in the training group (AUC =0.973, 1.000, 0.986, 0.904, 0.869, 1.000, and 0.735 of DTC, ETC, GBC, GPC, KNN, RF, and SVM, respectively) (Figure 6J). Feature importance analyses further demonstrated that GDF5 expression, cTNM stage, PLAUR expression, IL31RA expression, and normalizedShannonWienerIndex were the dominant features (Figure 6K), and RNA features held the largest contribution generally in the RF model. Given the generalization of NGS in clinical practice, we further interrogated whether Clinical + RNA or Clinical + DNA characteristics could reach comparable performance. Surprisingly, both combinations showed precise and stable predictive performance (Clinical + RNA: AUC =0.978, 1.000, 0.998, 0.999, 0.815, 0.975, and 0.818 of DTC, ETC, GBC, GPC, KNN, RF, and SVM, respectively; Clinical + DNA: AUC =0.999, 0.988, 1.000, 0.997, 0.803, 0.936, and 0.829 of DTC, ETC, GBC, GPC, KNN, RF, and SVM, respectively).
We then validated these algorithms in the internal testing cohort, showing that the Clinical + RNA features combination reached the most stable and accurate performance generally (AUC =0.692, 0.933, 0.800, 0.733, 0.867, 0.833, and 0.900 of DTC, ETC, GBC, GPC, KNN, RF, and SVM, respectively). The ACC, precision, recall, F1-score, and AUC of the seven combination categories across the seven ML algorithms in the training and testing cohort are listed in Tables S6,S7.
Given that the Clinical + RNA features combination of the RF algorithm exhibited stabler and more accurate performance than other ML methods (AUC 0.975, 95% CI: 0.926–1.000 in the training cohort; AUC 0.833, 95% CI: 0.627–1.000 in the testing cohort), it was chosen to be the ultimate predictor. The RF algorithm showed better AUC than the cTNM stage across the seven combination categories (Clinical features + DNA: P=0.14; Clinical features + RNA: P<0.001; Clinical features + TCR: P=0.26; Clinical features + DNA + RNA: P=0.05; Clinical features + DNA + RNA + TCR: P<0.001; DNA + RNA: P<0.001; DNA + RNA + TCR: P<0.001). The predicted high-risk early-stage Ns-NSCLC population held significantly shorter DFS in training (Figure 6L) and testing (Figure 6M) cohort (P<0.001).
Discussion
Our study pinpointed the unique molecular and immune alterations in the Chinese early-stage Ns-NSCLC cohort, which may affect prognosis and influence the crosstalk between tumor and the immune system, unveiling novel cancer biological meanings.
Our WES analysis identified consistent findings which were previously reported on the East-Asian Ns-NSCLC population. For instance, EGFR was the most frequently mutated gene and C > A base substitution was more frequently detected in ever-smokers (48). Frequently mutated smoking-related SBS4 signature was also detected, which has been reported to be the major feature of smoker LCs. Besides, different driver genes mutations may predict inverse somatic mutational burden (43).
Besides corroborating previous findings, several novel genomic alterations in recurrent early-stage Ns-NSCLC were discovered. For instance, a higher mutation rate of FGD5 was found in recurrent patients, which has been postulated to maintain breast cancer stem cell characteristics and facilitate tumor development (49), whereas no relevant findings on Ns-NSCLC were found. A higher mutation frequency of FLT4 was also discovered, which may activate the PI3K-AKT pathway to promote the angiogenesis of LUAD (50). Besides, a higher mutation frequency of MYO18B, a tumor suppressor gene (51), was identified in recurrent samples, and it was also a negative predictor of DFS. Mutations in MUC17, ABCA2, and PDE4DIP were also accentuated to play an important role in prognosis. Functional analyses further suggested that the mutation of these genes correlated with augmented malignant transformation of cells, warranting future experimental studies to examine the underlying biological implications.
As for the sCNA spectrum, alterations in cytobands of chr4p, chr8q, chr14q, etc., demonstrated significant variation of Del or Amp. Significant Amps in 8q24.3, 14q13.1, 14q11.2, and Del in 3p21.1 were highlighted in early-stage recurrent Ns-NSCLC. Amp in 14q13.1 has been postulated to be the oncogenic alteration of pulmonary lymphoepithelioma-like carcinoma, a rare subtype of NSCLC (52). Besides, PSCA at 8q24.3 is among the most frequently detected gastric cancer-susceptibility genes (53). Del in 3p21.1 has been reported to correlate with metastasis of renal and pancreatic cancer (54) while it was first described in Ns-NSCLC. Concerning disease-free samples, cytoband Amp in the chr7q22.1 region was found, which included the COL1A2 gene, and it has been postulated to correlate with extracellular matrix-receptor interaction in esophageal cancer (55). Amp in the chr8q24.21 that included the MYC gene was also found. And MYC is a classical oncogene crucial for tumor evolution and immune evasion in TME, mainly relating to Wnt/β-catenin signal pathways (56). Overall, our findings supplemented the repertoire of candidate genomic alterations contained in Ns-NSCLC pathogenesis.
We comprehensively depicted the spectrum of genomic biomarkers of early-stage Ns-NSCLC, including TMB, TNB, ITH, HLA-LOH, and wCIN. The occurrence of HLA-LOH was 28.9%, similar to a recent report of the Chinese NSCLC cohort (23.8%) (38). HLA-LOH was associated with higher mutation frequencies of classical oncogene TP53 and immune-related genes like TTN and CSMD1. In the context of inconsistent findings concerning the role of HLA-LOH in predicting ICI response (57), combining HLA-LOH status with concurrent mutated gene signatures, especially immune-related ones, may reach better performance. Moreover, HLA-LOH predicted higher TMB, TNB, ITH, and wCIN-score, suggesting higher mutational burden and a greater probability of tumor immune escape. Mutations in TP53 and TTN correlated with higher TMB and TNB, representing higher immunogenicity. Higher incidences of HLA-LOH, higher TMB and TNB were identified in ever-smokers than never-smokers, recapitulating previous findings.
We subsequently investigated the associations between various biomarkers, either alone or in combination, with prognosis. We found that higher TMB and wCIN-score predicted unfavorable DFS. On the contrary, higher ITH was an independent predictor of better DFS in early-stage Ns-NSCLC, showing the opposite effect from a previous study that focused on advanced NSCLC (22). Interestingly, low-TMB & high-ITH correlated with significantly longer DFS than those with high-TMB & low-ITH, implying combining the characteristics of subclonal mutations and immunogenic neoantigens may better achieve risk stratification. Besides, patients who harbored EGFR mutation and median-to-high TMB held worse DFS than those with low TMB and without EGFR mutation, corroborating previous reports (58).
Transcriptomic analysis underscored higher cell cycle pathway activity and lower cell differentiation and adhesion activity in recurrent, relatively advanced-stage, smokers, and EGFR-mutated Ns-NSCLC, mirroring the accumulation of cancer cell invasion and metastasis capacity that empowered the immune escape. The expression patterns of immune-related genes were fully assessed, pinpointing several essential prognosticators of early-stage Ns-NSCLC. CRABP2, i.e., cellular retinoic acid-binding protein, has been reported to facilitate LC metastasis by integrin β1/FAK/ERK pathway of in vitro model (59). Likewise, up-regulation of CRABP2 was discovered in TN than paratumor tissue, and it was an independent prognosticator of unfavorable DFS, suggesting a potential target to improve prognosis. ULBP2, a ligand of NKG2D that usually expresses under tissue stress or injury, is an important target of immune surveillance and a trigger of antitumor immunity. We found that the expression level of ULBP2 was significantly higher in TN than in paratumor tissue, and it correlated with dismal DFS, consistent with a previous study by Yamaguchi and colleagues (60). Additionally, up-regulated IL1A, a cytokine with pleiotropic functions in tumor evolution, portended a significantly worse prognosis. However, we could not further determine the cellular sources of expression of these genes and their correlations with other known druggable targets like by single-cell RNA sequencing (61) and multiplex immunofluorescence (62).
Via the deconvolution algorithm, phenotypic heterogeneity in TME was observed, and two major subtypes, including the tumor-inflamed and stroma-inflamed groups, were generated. The stroma-inflamed group featured by high activated NK cell, CD4+ T cell, and CD8+ T cell infiltrates indicated the benefit of ICI treatment (63). On the contrary, the tumor-inflamed group exhibited enriched M2 macrophages and Tregs, indicating ICI plus therapy targeting specific cell lineage like macrophages may better work (64,65). Additionally, we also parsed the different TME landscapes concerning different genomic alteration patterns, benefiting from a multi-omics setting.
Through parsing the TCR repertoire spectrum, we found that driver gene mutations like EGFR and ALK predicted muted TCR diversity while mutation in tumor suppressor genes like TP53 correlated with augmented TCR diversity inversely. Prognostic effects of TCR diversity of PBMCs were noted, demonstrating a noninvasive method for relapse surveillance.
Studies using other different omics also succeeded in predicting the prognosis and immunotherapy response of cancers. For instance, Triozzi and colleagues developed a metabolomic signature that correlated with glycolysis to characterize the ICI responders of melanoma (66). Moreover, they also found that higher extracellular acidification rate and lactate-to-pyruvate ratio were prognostic of superior outcomes. Proteomics narrows the gap between cancer genotypes and phenotypes and has paved the way for precision oncology in recent years. Recently, Harel et al. proposed a predictive signature of ICI response of NSCLC based on plasma proteomic profiling, including CXCL8 and CXCL10 proteins (67).
Eventually, seven ML algorithms were employed to estimate the predictive accuracy of seven combination categories based on clinical, genomic, transcriptomic, and TCR repertoire data. Clinical and RNA features combination in the RF algorithm, with AUC of 97.5% and 83.3% in the training and testing cohort, respectively, significantly outperformed other methods. Consequently, the model was robust and had the potential to optimize risk stratification of early-stage Ns-NSCLC along with the increased clinical utility of target panel sequencing. More importantly, such a framework underscored the significance of data integration via ML approaches for predicting relapse and may be feasible for other cancer types.
Several limitations should be noted. First, our study is restricted by the sample size and the number of individuals with specific mutant genes. In this sense, the generated hypotheses on the effects of molecular alterations on prognosis need to be examined in other populations or cohorts. Second, the immune subtypes of early-stage Ns-NSCLC were inferred from the deconvolution algorithm of RNA-seq data and lacked validation at the protein level, like by multiplex immunofluorescence (62). Third, while diversity, clonality, and similarity are the essential features of TCR repertoire, only diversity was probed. Moreover, the proposed multi-omics prognosticator held the risk of over-fitting due to limited cohort size and a lack of validation in the external cohort.
In recent years, numerous novel omics techniques have empowered researchers to dissect the pathogenesis of cancers at unprecedented resolution and scale, demonstrating a holistic view of cancer biology. Multi-omics analyses also pose the potential to identify innovative druggable targets and biomarkers for optimizing treatment benefits. The advent of multi-layer and broader data also raises the challenges of better synthesizing them and generalizing the findings into clinical utility. In our view, first, the employment of ML/deep learning algorithms, which could capture complex high-dimensional associations of multimodality data, is imperative. Second, technical specification and standardization across different labs to establish reproducible data are indispensable. Third, prospective and cross-cohort validation examining the associations or causalities between experimental findings and clinical outcomes are requisite. Overall, great efforts are underway to dissect the heterogeneity and plasticity of tumors, and we anticipate their integration into precision oncology.
Conclusions
In brief, this study comprehensively profiled the genomic, transcriptomic, and TCR repertoire spectrums of Chinese early-stage Ns-NSCLC, shedding light on biological underpinnings and candidate biomarkers for prognosis development.
Acknowledgments
Funding: This work was supported by
Footnote
Reporting Checklist: The authors have completed the TRIPOD reporting checklist. Available at https://tlcr.amegroups.com/article/view/10.21037/tlcr-23-800/rc
Data Sharing Statement: Available at https://tlcr.amegroups.com/article/view/10.21037/tlcr-23-800/dss
Peer Review File: Available at https://tlcr.amegroups.com/article/view/10.21037/tlcr-23-800/prf
Conflicts of Interest: All authors have completed the ICMJE uniform disclosure form (available at https://tlcr.amegroups.com/article/view/10.21037/tlcr-23-800/coif). W.L. serves as an unpaid Associate Editor-in-Chief of Translational Lung Cancer Research from May 2023 to April 2024. Xiaoli Cui, D.W., Z.G., and H.L. are current employees of YuceBio Technology Co., Ltd. The other authors have no conflicts of interest to declare.
Ethical Statement: The authors are accountable for all aspects of the work in ensuring that questions related to the accuracy or integrity of any part of the work are appropriately investigated and resolved. Informed consent of included patients was obtained, and this research was approved by the First Affiliated Hospital of Guangzhou Medical University Ethics Committee (approval No. KLS-17-03). The study was conducted in accordance with the Declaration of Helsinki (as revised in 2013).
Open Access Statement: This is an Open Access article distributed in accordance with the Creative Commons Attribution-NonCommercial-NoDerivs 4.0 International License (CC BY-NC-ND 4.0), which permits the non-commercial replication and distribution of the article with the strict proviso that no changes or edits are made and the original work is properly cited (including links to both the formal publication through the relevant DOI and the license). See: https://creativecommons.org/licenses/by-nc-nd/4.0/.
References
- Sung H, Ferlay J, Siegel RL, et al. Global Cancer Statistics 2020: GLOBOCAN Estimates of Incidence and Mortality Worldwide for 36 Cancers in 185 Countries. CA Cancer J Clin 2021;71:209-49. [Crossref] [PubMed]
- Miller M, Hanna N. Advances in systemic therapy for non-small cell lung cancer. BMJ 2021;375: [Crossref] [PubMed]
- Li C, Wang H, Jiang Y, et al. Advances in lung cancer screening and early detection. Cancer Biol Med 2022;19:591-608. [Crossref] [PubMed]
- Jonas DE, Reuland DS, Reddy SM, et al. Screening for Lung Cancer With Low-Dose Computed Tomography: Updated Evidence Report and Systematic Review for the US Preventive Services Task Force. JAMA 2021;325:971-87. [Crossref] [PubMed]
- Taylor MD, Nagji AS, Bhamidipati CM, et al. Tumor recurrence after complete resection for non-small cell lung cancer. Ann Thorac Surg 2012;93:1813-20; discussion 1820-1. [Crossref] [PubMed]
- Devarakonda S, Morgensztern D, Govindan R. Genomic alterations in lung adenocarcinoma. Lancet Oncol 2015;16:e342-51. [Crossref] [PubMed]
- Cancer Genome Atlas Research Network. Comprehensive genomic characterization of squamous cell lung cancers. Nature 2012;489:519-25. [Crossref] [PubMed]
- Zhang T, Joubert P, Ansari-Pour N, et al. Genomic and evolutionary classification of lung cancer in never smokers. Nat Genet 2021;53:1348-59. [Crossref] [PubMed]
- Rooney M, Devarakonda S, Govindan R. Genomics of squamous cell lung cancer. Oncologist 2013;18:707-16. [Crossref] [PubMed]
- Kadara H, Choi M, Zhang J, et al. Whole-exome sequencing and immune profiling of early-stage lung adenocarcinoma with fully annotated clinical follow-up. Ann Oncol 2017;28:75-82. [Crossref] [PubMed]
- Choi M, Kadara H, Zhang J, et al. Mutation profiles in early-stage lung squamous cell carcinoma with clinical follow-up and correlation with markers of immune function. Ann Oncol 2017;28:83-9. [Crossref] [PubMed]
- Faruki H, Mayhew GM, Serody JS, et al. Lung Adenocarcinoma and Squamous Cell Carcinoma Gene Expression Subtypes Demonstrate Significant Differences in Tumor Immune Landscape. J Thorac Oncol 2017;12:943-53. [Crossref] [PubMed]
- Li B, Cui Y, Diehn M, et al. Development and Validation of an Individualized Immune Prognostic Signature in Early-Stage Nonsquamous Non-Small Cell Lung Cancer. JAMA Oncol 2017;3:1529-37. [Crossref] [PubMed]
- Kratz JR, He J, Van Den Eeden SK, et al. A practical molecular assay to predict survival in resected non-squamous, non-small-cell lung cancer: development and international validation studies. Lancet 2012;379:823-32. [Crossref] [PubMed]
- Lahiri A, Maji A, Potdar PD, et al. Lung cancer immunotherapy: progress, pitfalls, and promises. Mol Cancer 2023;22:40. [Crossref] [PubMed]
- Riaz N, Havel JJ, Makarov V, et al. Tumor and Microenvironment Evolution during Immunotherapy with Nivolumab. Cell 2017;171:934-949.e16. [Crossref] [PubMed]
- Aran A, Garrigós L, Curigliano G, et al. Evaluation of the TCR Repertoire as a Predictive and Prognostic Biomarker in Cancer: Diversity or Clonality? Cancers (Basel) 2022;14:1771. [Crossref] [PubMed]
- Han J, Duan J, Bai H, et al. TCR Repertoire Diversity of Peripheral PD-1(+)CD8(+) T Cells Predicts Clinical Outcomes after Immunotherapy in Patients with Non-Small Cell Lung Cancer. Cancer Immunol Res 2020;8:146-54. [Crossref] [PubMed]
- Liu YY, Yang QF, Yang JS, et al. Characteristics and prognostic significance of profiling the peripheral blood T-cell receptor repertoire in patients with advanced lung cancer. Int J Cancer 2019;145:1423-31. [Crossref] [PubMed]
- Ettinger DS, Wood DE, Aisner DL, et al. Non-Small Cell Lung Cancer, Version 3.2022, NCCN Clinical Practice Guidelines in Oncology. J Natl Compr Canc Netw 2022;20:497-530. [Crossref] [PubMed]
- World Medical Association Declaration of Helsinki. ethical principles for medical research involving human subjects. JAMA 2013;310:2191-4. [Crossref] [PubMed]
- Fang W, Jin H, Zhou H, et al. Intratumoral heterogeneity as a predictive biomarker in anti-PD-(L)1 therapies for non-small cell lung cancer. Mol Cancer 2021;20:37. [Crossref] [PubMed]
- McGranahan N, Rosenthal R, Hiley CT, et al. Allele-Specific HLA Loss and Immune Escape in Lung Cancer Evolution. Cell 2017;171:1259-1271.e11. [Crossref] [PubMed]
- Braun DA, Hou Y, Bakouny Z, et al. Interplay of somatic alterations and immune infiltration modulates response to PD-1 blockade in advanced clear cell renal cell carcinoma. Nat Med 2020;26:909-18. [Crossref] [PubMed]
- Sanchez-Vega F, Mina M, Armenia J, et al. Oncogenic Signaling Pathways in The Cancer Genome Atlas. Cell 2018;173:321-337.e10. [Crossref] [PubMed]
- Bray NL, Pimentel H, Melsted P, et al. Near-optimal probabilistic RNA-seq quantification. Nat Biotechnol 2016;34:525-7. [Crossref] [PubMed]
- Subramanian A, Tamayo P, Mootha VK, et al. Gene set enrichment analysis: a knowledge-based approach for interpreting genome-wide expression profiles. Proc Natl Acad Sci U S A 2005;102:15545-50. [Crossref] [PubMed]
- Laughney AM, Hu J, Campbell NR, et al. Regenerative lineages and immune-mediated pruning in lung cancer metastasis. Nat Med 2020;26:259-69. [Crossref] [PubMed]
- Barker DJ, Maccari G, Georgiou X, et al. The IPD-IMGT/HLA Database. Nucleic Acids Res 2023;51:D1053-60. [Crossref] [PubMed]
- Bolotin DA, Poslavsky S, Mitrophanov I, et al. MiXCR: software for comprehensive adaptive immunity profiling. Nat Methods 2015;12:380-1. [Crossref] [PubMed]
- Shugay M, Bagaev DV, Turchaninova MA, et al. VDJtools: Unifying Post-analysis of T Cell Receptor Repertoires. PLoS Comput Biol 2015;11:e1004503. [Crossref] [PubMed]
- Newman AM, Liu CL, Green MR, et al. Robust enumeration of cell subsets from tissue expression profiles. Nat Methods 2015;12:453-7. [Crossref] [PubMed]
- Becht E, Giraldo NA, Lacroix L, et al. Estimating the population abundance of tissue-infiltrating immune and stromal cell populations using gene expression. Genome Biol 2016;17:218. [Crossref] [PubMed]
- Garfinkle R, Filion KB, Bhatnagar S, et al. Prediction model and web-based risk calculator for postoperative ileus after loop ileostomy closure. Br J Surg 2019;106:1676-84. [Crossref] [PubMed]
- Riley RD, Ensor J, Snell KIE, et al. Calculating the sample size required for developing a clinical prediction model. BMJ 2020;368:m441. [Crossref] [PubMed]
- Camp RL, Dolled-Filhart M, Rimm DL. X-tile: a new bio-informatics tool for biomarker assessment and outcome-based cut-point optimization. Clin Cancer Res 2004;10:7252-9. [Crossref] [PubMed]
- Wang C, Yin R, Dai J, et al. Whole-genome sequencing reveals genomic signatures associated with the inflammatory microenvironments in Chinese NSCLC patients. Nat Commun 2018;9:2054. [Crossref] [PubMed]
- Cancer Genome Atlas Research Network. Comprehensive molecular profiling of lung adenocarcinoma. Nature 2014;511:543-50. [Crossref] [PubMed]
- Pfeifer GP. Environmental exposures and mutational patterns of cancer genomes. Genome Med 2010;2:54. [Crossref] [PubMed]
- Liu Y, Gusev A, Heng YJ, et al. Somatic mutational profiles and germline polygenic risk scores in human cancer. Genome Med 2022;14:14. [Crossref] [PubMed]
- Wang Z, Wang C, Lin S, et al. Effect of TTN Mutations on Immune Microenvironment and Efficacy of Immunotherapy in Lung Adenocarcinoma Patients. Front Oncol 2021;11:725292. [Crossref] [PubMed]
- Saunders GRB, Wang X, Chen F, et al. Genetic diversity fuels gene discovery for tobacco and alcohol use. Nature 2022;612:720-4. [Crossref] [PubMed]
- Huang Z, Sun S, Lee M, et al. Single-cell analysis of somatic mutations in human bronchial epithelial cells in relation to aging and smoking. Nat Genet 2022;54:492-8. [Crossref] [PubMed]
- Duan Y, Du Y, Gu Z, et al. Expression, Prognostic Value, and Functional Mechanism of the KDM5 Family in Pancreatic Cancer. Front Cell Dev Biol 2022;10:887385. [Crossref] [PubMed]
- Hecht SS. Cigarette smoking and lung cancer: chemical mechanisms and approaches to prevention. Lancet Oncol 2002;3:461-9. [Crossref] [PubMed]
- Martínez-Ruiz C, Black JRM, Puttick C, et al. Genomic-transcriptomic evolution in lung cancer and metastasis. Nature 2023;616:543-52. [Crossref] [PubMed]
- Shen S, Wang G, Zhang R, et al. Development and validation of an immune gene-set based Prognostic signature in ovarian cancer. EBioMedicine 2019;40:318-26. [Crossref] [PubMed]
- Chen J, Yang H, Teo ASM, et al. Genomic landscape of lung adenocarcinoma in East Asians. Nat Genet 2020;52:177-86. [Crossref] [PubMed]
- Li K, Zhang TT, Zhao CX, et al. Faciogenital Dysplasia 5 supports cancer stem cell traits in basal-like breast cancer by enhancing EGFR stability. Sci Transl Med 2021;13:eabb2914. [Crossref] [PubMed]
- Fang H, Sun Q, Zhou J, et al. m(6)A methylation reader IGF2BP2 activates endothelial cells to promote angiogenesis and metastasis of lung adenocarcinoma. Mol Cancer 2023;22:99. [Crossref] [PubMed]
- Nishioka M, Kohno T, Tani M, et al. MYO18B, a candidate tumor suppressor gene at chromosome 22q12.1, deleted, mutated, and methylated in human lung cancer. Proc Natl Acad Sci U S A 2002;99:12269-74. [Crossref] [PubMed]
- Chen B, Zhang Y, Dai S, et al. Molecular characteristics of primary pulmonary lymphoepithelioma-like carcinoma based on integrated genomic analyses. Signal Transduct Target Ther 2021;6:6. [Crossref] [PubMed]
- Study Group of Millennium Genome Project for Cancer. Genetic variation in PSCA is associated with susceptibility to diffuse-type gastric cancer. Nat Genet 2008;40:730-40. [Crossref] [PubMed]
- Yang J, Lin P, Yang M, et al. Integrated genomic and transcriptomic analysis reveals unique characteristics of hepatic metastases and pro-metastatic role of complement C1q in pancreatic ductal adenocarcinoma. Genome Biol 2021;22:4. [Crossref] [PubMed]
- Li G, Jiang W, Kang Y, et al. High expression of collagen 1A2 promotes the proliferation and metastasis of esophageal cancer cells. Ann Transl Med 2020;8:1672. [Crossref] [PubMed]
- Dhanasekaran R, Deutzmann A, Mahauad-Fernandez WD, et al. The MYC oncogene - the grand orchestrator of cancer growth and immune evasion. Nat Rev Clin Oncol 2022;19:23-36. [Crossref] [PubMed]
- Chowell D, Morris LGT, Grigg CM, et al. Patient HLA class I genotype influences cancer response to checkpoint blockade immunotherapy. Science 2018;359:582-7. [Crossref] [PubMed]
- Offin M, Rizvi H, Tenet M, et al. Tumor Mutation Burden and Efficacy of EGFR-Tyrosine Kinase Inhibitors in Patients with EGFR-Mutant Lung Cancers. Clin Cancer Res 2019;25:1063-9. [Crossref] [PubMed]
- Wu JI, Lin YP, Tseng CW, et al. Crabp2 Promotes Metastasis of Lung Cancer Cells via HuR and Integrin β1/FAK/ERK Signaling. Sci Rep 2019;9:845. [Crossref] [PubMed]
- Yamaguchi K, Chikumi H, Shimizu A, et al. Diagnostic and prognostic impact of serum-soluble UL16-binding protein 2 in lung cancer patients. Cancer Sci 2012;103:1405-13. [Crossref] [PubMed]
- Pullikuth AK, Routh ED, Zimmerman KD, et al. Bulk and Single-Cell Profiling of Breast Tumors Identifies TREM-1 as a Dominant Immune Suppressive Marker Associated With Poor Outcomes. Front Oncol 2021;11:734959. [Crossref] [PubMed]
- Peng H, Wu X, Liu S, et al. Multiplex immunofluorescence and single-cell transcriptomic profiling reveal the spatial cell interaction networks in the non-small cell lung cancer microenvironment. Clin Transl Med 2023;13:e1155. [Crossref] [PubMed]
- Peng H, Wu X, Zhong R, et al. Profiling Tumor Immune Microenvironment of Non-Small Cell Lung Cancer Using Multiplex Immunofluorescence. Front Immunol 2021;12:750046. [Crossref] [PubMed]
- Yang L, Zhang Y. Tumor-associated macrophages: from basic research to clinical application. J Hematol Oncol 2017;10:58. [Crossref] [PubMed]
- Wu XR, Peng HX, He M, et al. Macrophages-based immune-related risk score model for relapse prediction in stage I-III non-small cell lung cancer assessed by multiplex immunofluorescence. Transl Lung Cancer Res 2022;11:523-42. [Crossref] [PubMed]
- Triozzi PL, Stirling ER, Song Q, et al. Circulating Immune Bioenergetic, Metabolic, and Genetic Signatures Predict Melanoma Patients' Response to Anti-PD-1 Immune Checkpoint Blockade. Clin Cancer Res 2022;28:1192-202. [Crossref] [PubMed]
- Harel M, Lahav C, Jacob E, et al. Longitudinal plasma proteomic profiling of patients with non-small cell lung cancer undergoing immune checkpoint blockade. J Immunother Cancer 2022;10:e004582. [Crossref] [PubMed]