Development of a predictive model to predict postoperative bone metastasis in pathological I–II non-small cell lung cancer
Highlight box
Key findings
• Pulmonary nodule with solid component, higher pT and pN stage, and specific histologic subtypes were associated with a high risk of bone metastasis in resected early-stage non-small cell lung cancer (NSCLC).
What is known and what is new?
• Bone metastasis is common metastatic site in NSCLC after surgery, but risk factors in resected early-stage NSCLC are not well investigated.
• This study focused specifically on identifying risk factors for bone metastasis in early-stage NSCLC following surgery and established a predictive model for quantifying the risk.
What is the implication, and what should change now?
• The predictive model, based on four easily obtainable clinical parameters, can identify high-risk patients, and facilitate personalized surveillance plans.
Introduction
Surgery is the established standard treatment for early-stage non-small cell lung cancer (NSCLC). However, previous study reported that up to 16.6% early-stage NSCLC patients after radical surgery experienced distant metastasis, which largely leads to poor survival (1). The advancement of low-dose computed tomography (CT) for lung cancer screening has notably increased the detection of early-stage NSCLC (2). The potential for postoperative metastasis may become more pronounced within the expanding early-stage NSCLC population. Thus, it is critically important in clinical practice to identify risk factors associated with distant metastasis in early-stage NSCLC, enabling the implementation of personalized surveillance strategies for high-risk patients.
In NSCLC, bone is one of the most frequent distant metastatic sites (3,4). Despite the advancements in treatment strategies contributing to improved prognosis, approximately 30–40% of NSCLC patients experience bone metastasis during disease progression (3,5). The development of bone metastasis indicates poor prognosis. Approximately 80% patients with bone metastasis will suffer from reduced quality of life (6) and severe complications, like spinal cord compression, pathological fractures, hypocalcemia, and other skeletal-related events (7). Previous works have made efforts to determine risk factors associated with bone metastasis in NSCLC, including demographic characteristics (younger age) (8), biomarkers (lower blood calcium, over-expression of bone sialoprotein, and higher bone alkaline phosphatase) (9-11), tumor characteristics (poor tumor differentiation, higher tumor stage, and adenocarcinoma) (8,9). Nevertheless, a considerable proportion of these studies included patients with advanced lung cancer, potentially limiting the applicability of these risk factors to the rising population of early-stage NSCLC.
In this context, this study aimed to identify risk factors for bone metastasis in patients with resected early-stage NSCLC and establish a predictive model for quantifying the risk of bone metastasis. We present this article in accordance with the TRIPOD reporting checklist (available at https://tlcr.amegroups.com/article/view/10.21037/tlcr-23-866/rc) (12).
Methods
Study population
We conducted a retrospective analysis involving individuals who underwent surgical procedures for NSCLC at the Department of Thoracic Surgery, West China Hospital between January 2015 and January 2021. The eligibility criteria were as follows: (I) pathological confirmation of NSCLC; (II) pathological stage I–II NSCLC according to the 8th edition of the American Joint Committee on Cancer Staging Manual; and (III) received lobectomy. Exclusions criteria included: (I) age less than 18 years old; (II) not R0 resection; (III) previous history of cancer; (IV) patients who received neoadjuvant chemotherapy or radiotherapy; (V) patients with incomplete medical records or survival information; and (VI) atypical adenomatous hyperplasia, adenocarcinoma in situ, and minimally invasive adenocarcinoma. The study was conducted in accordance with the Declaration of Helsinki (as revised in 2013). The study was approved by the Ethics Committee of West China Hospital of Sichuan University (No. 2022-581). For the retrospective nature, personal informed consent was exempted.
Data collection and variables definition
The demographic variables [age, sex, body mass index (BMI)], history of smoking, treatment information (resection extent, adjuvant radiotherapy, and chemotherapy), and tumor characteristics (solid component of nodules, tumor location, pathological stage, and histologic subtypes) were obtained from our database.
Continuous variables were classified into categorical variables based on recognized cutoffs. Age was divided into <60 and ≥60 years. BMI was categorized as underweight (<18.5 kg/m2), normal weight (18.5–24.9 kg/m2), and overweight (≥25 kg/m2). Based on the solid component observed on the preoperative high-resolution CT, patients without solid component were divided into solid-absent group, while the others were included in the solid-present group, irrespective of the proportion of the solid component. Pathological N stage was divided into N0, N1 single lymph node station involvement (N1a), and N1 multiple lymph node station involvement (N1b) (13). In term of the International Association for the Study of Lung Cancer (IASLC)/American Thoracic Society (ATS)/European Respiratory Society (ERS) classification system (14), lung adenocarcinoma was divided into well-differentiated (lepidic predominant), moderately differentiated (acinar and papillary predominant), and poorly differentiated (solid and micropapillary predominant) groups. The time to bone metastasis was defined as the duration from the date of surgery to the detection of bone metastasis.
Sample size
We utilized the “pmsampsize” function to compute sample size, ensuring adequate statistical power (15). These parameters included a Cox-Snell R2 of 0.05, ten candidate predictor parameters, an overall event rate of 0.0256, a prediction timepoint of 1 year, and an anticipated average follow-up time of 1 year. The final required sample size was 1,750, with 45 outcome events.
Construction of the nomogram
Univariable and multivariable Cox proportional hazards regression analyses were conducted to determine the independent risk factors associated with bone metastasis. Variables displaying a P value <0.05 in univariable Cox regression analyses, along with those deemed clinically significant, were included in the multivariable Cox regression analyses. Additionally, a nomogram was constructed to enhance the prediction of bone metastasis risk, utilizing independent risk factors derived from the multivariable Cox regression analyses, employing the “rms” package within the R software.
Assigning a score to each patient, we categorized them into low- and high-risk groups. The optimal cut-off value was determined using the “surv_cutpoint” function within the “survminer” R package, which provides a cut-off value that correspond to the most significant relation with outcome. Subsequently, the differences in bone metastasis-free survival between low- and high-risk groups were illustrated through Kaplan-Meier curves and assessed using the log-rank test.
Validation of the model
The performance of the predictive model was evaluated through discrimination and calibration.
The Harrell concordance index (C-index) evaluated the discrimination, while time-dependent receiver operating characteristic (ROC) curves and their corresponding areas under the curve (AUCs) were computed for 1-, 3-, and 5-year follow-ups to further assess discrimination. Calibration curves were plotted to evaluate the calibration, illustrating the comparison between observed and predicted bone metastasis risks. Internal validation was conducted using 1,000 bootstrap resamples to estimate the optimism-corrected C-index.
All the statistical analyses were conducted using R version 4.3.2. A two-tailed P value below 0.05 was regarded as statistically significant.
Results
Baseline characteristics
Baseline clinical and demographic characteristics are summarized in Table 1. Of 2,106 eligible patients included, 54 (2.56%) patients developed bone metastasis (Figure 1). Among them, 48.81% were male and 51.80% were more than 60 years old. The proportion of patients with right-side lung cancer were 61.54%. There were 1,800 (85.47%) patients and 306 (14.53%) patients diagnosed with pathological I and II NSCLC, respectively. The proportion of lung adenocarcinoma was 87.94%, of which 50.85% were lepidic predominant type. A total of 388 (18.42%) patients received adjuvant chemotherapy, and eight (0.38%) patients received adjuvant radiotherapy. Following a median follow-up of 29 months (interquartile interval, 18–41 months), 1.28% (27/2,106), 2.52% (53/2,106), and 2.56% (54/2,106) of the patients developed bone metastasis within 1, 3, and 5 years, respectively.
Table 1
Characteristics | Total (n=2,106) | No bone metastases (n=2,052) | Bone metastases (n=54) | P value |
---|---|---|---|---|
Age at diagnosis, years | 0.68 | |||
<60 years | 1,015 (48.20) | 987 (48.10) | 28 (51.85) | |
≥60 years | 1,091 (51.80) | 1,065 (51.90) | 26 (48.15) | |
BMI at diagnosis, kg/m2 | 0.26 | |||
<18.5 kg/m2 | 101 (4.80) | 97 (4.73) | 4 (7.41) | |
18.5–24.9 kg/m2 | 1,430 (67.90) | 1,390 (67.74) | 40 (74.07) | |
≥25 kg/m2 | 575 (27.30) | 565 (27.53) | 10 (18.52) | |
Sex | 0.005 | |||
Male | 1,028 (48.81) | 991 (48.29) | 37 (68.52) | |
Female | 1,078 (51.19) | 1,061 (51.71) | 17 (31.48) | |
Smoking status | 0.01 | |||
Never | 1,367 (64.91) | 1,341 (65.35) | 26 (48.15) | |
Current/ever | 739 (35.09) | 711 (34.65) | 28 (51.85) | |
Solid component | <0.001 | |||
Absent | 505 (23.98) | 504 (24.56) | 1 (1.85) | |
Present | 1,601 (76.02) | 1,548 (75.44) | 53 (98.15) | |
Tumor location | 0.52 | |||
Left | 810 (38.46) | 792 (38.60) | 18 (33.33) | |
Right | 1,296 (61.54) | 1,260 (61.40) | 36 (66.67) | |
Adjuvant radiotherapy | 0.51 | |||
No | 2,098 (99.62) | 2,045 (99.66) | 53 (98.15) | |
Yes | 8 (0.38) | 7 (0.34) | 1 (1.85) | |
Adjuvant chemotherapy | <0.001 | |||
No | 1,718 (81.58) | 1,687 (82.21) | 31 (57.41) | |
Yes | 388 (18.42) | 365 (17.79) | 23 (42.59) | |
Pathogenic stage | <0.001 | |||
IA | 830 (39.41) | 823 (40.11) | 7 (12.96) | |
IB | 970 (46.06) | 951 (46.35) | 19 (35.19) | |
IIA | 82 (3.89) | 77 (3.75) | 5 (9.26) | |
IIB | 224 (10.64) | 201 (9.80) | 23 (42.59) | |
pT stage | 0.001 | |||
T1 | 863 (40.98) | 854 (41.62) | 9 (16.67) | |
T2 | 1,192 (56.60) | 1,149 (55.99) | 43 (79.63) | |
T3 | 51 (2.42) | 49 (2.39) | 2 (3.70) | |
pN stage | <0.001 | |||
N0 | 1,933 (91.79) | 1,900 (92.59) | 33 (61.11) | |
N1a | 132 (6.27) | 119 (5.80) | 13 (24.07) | |
N1b | 41 (1.95) | 33 (1.61) | 8 (14.81) | |
Histologic type | <0.001 | |||
Squamous cell carcinoma | 254 (12.06) | 247 (12.04) | 7 (12.96) | |
Lepidic | 1,071 (50.85) | 1,058 (51.56) | 13 (24.07) | |
Acinar/papillary | 744 (35.33) | 712 (34.70) | 32 (59.26) | |
Solid/micropapillary | 37 (1.76) | 35 (1.71) | 2 (3.70) |
Data are presented as n (%). NSCLC, non-small cell lung cancer; BMI, body mass index.
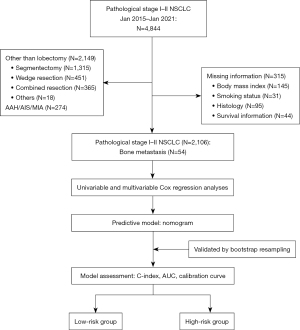
Risk factors for bone metastasis and individualized nomogram
In the analyses of risk factors associated with bone metastasis in early-stage NSCLC, tumor nodules with solid component, higher pT stage, higher pN stage, and histologic subtypes especially solid/micropapillary predominant types were highlighted as independent risk factors through the univariable and multivariable Cox regression analyses (Table 2). These independent risk factors were included in the final predictive model.
Table 2
Variables | Univariable | Multivariable | |||||
---|---|---|---|---|---|---|---|
HR | 95% CI | P value | HR | 95% CI | P value | ||
Age at diagnosis | |||||||
<60 years | Ref | Ref | |||||
≥60 years | 0.87 | 0.51–1.49 | 0.62 | 0.80 | 0.46–1.37 | 0.41 | |
BMI at diagnosis | |||||||
<18.5 kg/m2 | Ref | ||||||
18.5–24.9 kg/m2 | 0.70 | 0.25–1.95 | 0.49 | ||||
≥25 kg/m2 | 0.43 | 0.13–1.37 | 0.15 | ||||
Sex | |||||||
Male | Ref | Ref | |||||
Female | 0.43 | 0.24–0.77 | 0.004 | 0.55 | 0.25–1.23 | 0.15 | |
Smoking status | |||||||
Never | Ref | Ref | |||||
Current/former | 1.93 | 1.13–3.28 | 0.02 | 1.13 | 0.53–2.41 | 0.75 | |
Solid component | |||||||
Absent | Ref | Ref | |||||
Present | 16.52 | 2.28–119.46 | 0.005 | 8.44 | 1.13–63.29 | 0.04 | |
Tumor location | |||||||
Left | Ref | ||||||
Right | 1.26 | 0.71–2.21 | 0.43 | ||||
Adjuvant chemotherapy | |||||||
No | Ref | Ref | |||||
Yes | 3.05 | 1.77–5.23 | <0.001 | 0.92 | 0.47–1.83 | 0.82 | |
pT stage | |||||||
T1 | Ref | Ref | |||||
T2 | 3.30 | 1.61–6.77 | 0.001 | 2.12 | 1.01–4.43 | 0.047 | |
T3 | 3.85 | 0.83–17.84 | 0.08 | 4.55 | 0.91–22.87 | 0.07 | |
pN stage | |||||||
N0 | Ref | Ref | |||||
N1a | 5.79 | 3.05–11.00 | <0.001 | 4.15 | 1.95–8.77 | <0.001 | |
N1b | 10.9 | 5.03–23.60 | <0.001 | 6.81 | 2.70–17.18 | <0.001 | |
Histologic type | |||||||
Squamous cell carcinoma | Ref | Ref | |||||
Lepidic | 0.48 | 0.19–1.20 | 0.12 | 1.89 | 0.68–5.28 | 0.22 | |
Acinar/papillary | 1.55 | 0.68–3.51 | 0.29 | 2.84 | 1.18–6.83 | 0.02 | |
Solid/micropapillary | 2.18 | 0.45–10.49 | 0.33 | 2.84 | 0.58–13.79 | 0.20 |
NSCLC, non-small cell lung cancer; HR, hazard ratio; CI, confidence interval; BMI, body mass index; Ref, reference.
Based on selected risk factors, an individualized nomogram was established for predicting bone metastasis in early-stage NSCLC patients (Figure 2). To facilitate the clinical practice of the model, a dynamic nomogram was available online (https://wudongsheng.shinyapps.io/BoneMet/). The score of each patient was estimated based on the established nomogram. Based on the optimal cut-off value of 170 points, all patients were classified into either the low-risk [bone metastasis ratio: 30/1,880 (1.60%); scores: 0–170] or the high-risk [bone metastasis ratio: 24/280 (8.57%); scores: 171–280] groups. Significant difference of bone metastasis-free survival rates was observed between the low-risk and high-risk groups, as demonstrated by the Kaplan-Meier curves and assessed through the log-rank test (P<0.001) (Figure 3).
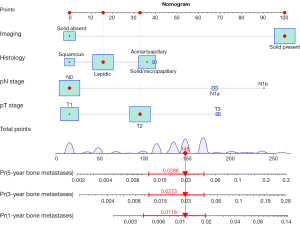
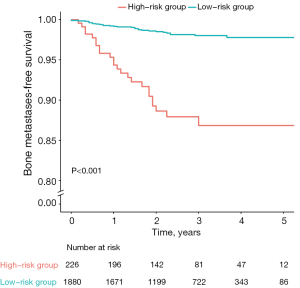
Validation of the nomogram
We evaluated the performance of the nomogram through the discrimination and calibration. The nomogram achieved a C-index of 0.793 (95% CI: 0.736–0.850), highlighting the model’s strong discriminatory capability. Across 1-, 3-, and 5-year follow-ups, the AUCs were 0.807 (95% CI: 0.733–0.881), 0.769 (95% CI: 0.704–0.833), and 0.761 (95% CI: 0.679–0.844) respectively (refer to Figure 4A-4C). Using 1000 bootstraps resampling, the C-indexes for the 1-, 3-, and 5-year follow-ups were 0.820, 0.793, and 0.777, correspondingly. Notably, the calibration curves exhibited robust consistency between predicted and observed risks of bone metastasis (Figure 4D-4F).
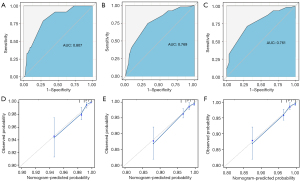
Discussion
In this study, we performed a retrospective study to determine independent risk factors associated with bone metastasis in early-stage NSCLC who received radical surgery. Meanwhile, a quantitative nomogram was established to predict the likelihood of bone metastasis. The predictive model showed good discrimination and calibration.
The presence of bone metastasis in NSCLC patients is often associated with poor prognosis. According to the previous literature, the incidence of bone metastasis in NSCLC varied from 30% to 40% (3,5). This study, specifically focused on pathologic stage I–II NSCLC patients, revealed a bone metastasis incidence of 2.56%. Despite the relatively low incidence, the occurrence of bone metastasis was linked to a shorter survival time and poor quality of life, especially when skeletal related events occur (16). In a retrospective analysis involving 374 patients (70.1% with stage I + II disease and 29.9% with stage III disease), those with bone metastasis exhibited a median survival time of 17 months (17). Comparatively, patients without bone metastasis demonstrated a higher 3-year overall survival rate (71.23% vs. 46.81%; P<0.001) (17).
In our study, pathological T and N stage were considered as independent risk factors associated with bone metastasis in resected stage I–II NSCLC. Similarly, Zhang et al. (10), involving 180 NSCLC patients receiving surgery, revealed that higher pathological T and N stages correlated with an elevated risk of bone metastasis. In a large sample size of Surveillance, Epidemiology, and End Results (SEER) database study, Wang et al. (8) also found that elevated N stage was significantly correlated with an increased occurrence of bone metastasis. However, in the research conducted by Wang et al. (17), while the pathological stage was identified as a risk factor associated with bone metastasis, individual pathological T and N stages did not independently contribute to the risk.
To explore the correlation between the subclassification of pathological N1 stage and the development of bone metastasis, we divided pathological N1 stage into two subcategories: single lymph node station involvement (N1a) and multiple lymph node station involvement (N1b). Our findings indicated that patients with multiple lymph node station involvement displayed a higher probability of developing bone metastasis in contrast to those with single lymph node station involvement, which might indicate lymph station number as a potential N descriptor. This result aligned with previous studies, which have reported that multiple station involvement is associated with a poorer survival compared to single station involvement (18,19).
In this study, we noted that adenocarcinoma presented a higher risk of bone metastasis compared to squamous cell carcinoma, which is consistent with recent findings (8,17). Despite that many studies aimed at identifying the underlying reasons for this increased risk in patients with lung adenocarcinoma, the exact mechanism remains elusive. Previous research has suggested a potential link between elevated levels of vascular endothelial growth factor (VEGF) in adenocarcinoma and tumor metastasis due to the pivotal role of VEGF in angiogenesis within tumor tissues (20,21). Additionally, certain histologic subtype such as solid and micropapillary predominant types in adenocarcinoma were linked to an elevated risk of postoperative recurrence (22-24). Furthermore, Caso et al. (25) reported that solid and micropapillary predominant tumors exhibited higher tumor mutational burden, copy number amplifications, fraction of genome altered, number of oncogenic pathways altered, and rate of whole genome doubling compared to other invasive adenocarcinoma subtypes. Considering these observations, we sought to determine the association between detailed histologic subtypes and the risk of bone metastasis. Following the IASLC/ATS/ERS classification (14), we divided adenocarcinoma into detailed subtypes and found that solid/micropapillary types exhibited a high risk of bone metastasis, which is consistent with well-known association between these histologic subtypes and unfavorable prognosis (14,26).
Prior research has suggested that a high consolidation-to-tumor ratio of tumor nodules is indicative of aggressive tumor behavior and poor prognosis (27,28). However, the relationship between the solid component of a tumor nodule and the risk of bone metastasis in early-stage NSCLC has not been explored. In our study, a significant association between the presence of solid component and the occurrence of bone metastasis was observed. This finding suggested that the presence of solid component on preoperative CT scans was a predictor for the risk of bone metastasis in early-stage NSCLC.
In recent years, immune checkpoint inhibitors have been approved for use in the neoadjuvant and adjuvant settings based on event-free survival and disease-free survival benefit. For example, the results of PEARLS (29) and IMpower 010 (30) trial indicated that platinum-based chemotherapy followed by adjuvant atezolizumab or pembrolizumab could significantly extend disease-free survival among resected stage II–IIIA NSCLC patients. According to the result of CheckMate-816 (31), the efficacy of neoadjuvant platinum-based chemotherapy with nivolumab was impressive in preventing distant metastasis compared to chemotherapy group among resectable early-stage NSCLC (median event-free survival durations: 31.6 versus 20.8 months; hazard ratio =0.63; 97.38% CI: 0.43–0.91; P=0.005). The metastatic diagram of early-stage resectable NSCLC would experience a great shift as the popularization of immunochemotherapy in clinic. Although our study only identified high-risk patients for bone metastasis after surgery restricted to traditional treatment regimens, the result of which can serve as a reference for future decision on clinical treatment strategies: high-risk patients predicted by this model may be prioritized for treatment with neoadjuvant/adjuvant chemoimmunotherapy to maximumly lower the possibility of postoperative bone metastasis.
Timely detection of bone metastasis holds the potential to facilitate less aggressive therapeutic interventions (32) and reduce the occurrence of skeletal-related complications (33). While skeletal system imaging and some bone formation markers (e.g., alkaline phosphatase, procollagen I carboxyl-terminal propeptide, etc.) stands as an effective means of early detection for bone metastasis (5,34), these examinations are performed in instances where symptoms indicative of bone metastasis present or when re-staging is required, especially in early-stage NSCLC. Moreover, clinical implementation of routine postoperative bone imaging in early-stage NSCLC is restricted by concerns regarding radiation exposure and financial implications. To address this issue, we developed a predictive model based on preoperative imaging features, pathological stage, and histologic subtypes. This model aids clinicians in identifying high-risk patients who may benefit from high-sensitivity tests for bone metastases, such as alkaline phosphatase tests and whole-body scans, during their personalized follow-up.
Inevitably, several limitations existed in our study. Firstly, retrospective design might introduce selection biases and data from a single center might restrict the generalizability of the predictive model. Secondly, due to the limited sample size of the sublobectomy and pneumonectomy groups, our focus was on patients who underwent lobectomy. Further research is needed to examine the comprehensive analyses beyond lobectomy. Thirdly, the classification of lung adenocarcinoma into detailed subtypes was restricted by the limited sample size in each subtype, thereby constraining the predictive power of the predictive model. Additionally, the limited sample size may introduce a risk of overfitting. Thus, prospective studies with large sample size are needed to validate our study.
Conclusions
In this study, a nomogram was developed and validated to predict the probability of bone metastasis in patients with resected early-stage NSCLC. Leveraging four easy-to-acquire variables, our nomogram can help identify high-risk patients and facilitate the individualized surveillance plans.
Acknowledgments
Funding: This work was supported by
Footnote
Reporting Checklist: The authors have completed the TRIPOD reporting checklist. Available at https://tlcr.amegroups.com/article/view/10.21037/tlcr-23-866/rc
Data Sharing Statement: Available at https://tlcr.amegroups.com/article/view/10.21037/tlcr-23-866/dss
Peer Review File: Available at https://tlcr.amegroups.com/article/view/10.21037/tlcr-23-866/prf
Conflicts of Interest: All authors have completed the ICMJE uniform disclosure form (available at https://tlcr.amegroups.com/article/view/10.21037/tlcr-23-866/coif). The authors have no conflicts of interest to declare.
Ethical Statement: The authors are accountable for all aspects of the work in ensuring that questions related to the accuracy or integrity of any part of the work are appropriately investigated and resolved. The study was conducted in accordance with the Declaration of Helsinki (as revised in 2013). The study was approved by the Ethics Committee of West China Hospital of Sichuan University (No. 2022-581) and individual consent for this retrospective analysis was waived.
Open Access Statement: This is an Open Access article distributed in accordance with the Creative Commons Attribution-NonCommercial-NoDerivs 4.0 International License (CC BY-NC-ND 4.0), which permits the non-commercial replication and distribution of the article with the strict proviso that no changes or edits are made and the original work is properly cited (including links to both the formal publication through the relevant DOI and the license). See: https://creativecommons.org/licenses/by-nc-nd/4.0/.
References
- Kelsey CR, Marks LB, Hollis D, et al. Local recurrence after surgery for early stage lung cancer: an 11-year experience with 975 patients. Cancer 2009;115:5218-27. [Crossref] [PubMed]
- Passiglia F, Cinquini M, Bertolaccini L, et al. Benefits and Harms of Lung Cancer Screening by Chest Computed Tomography: A Systematic Review and Meta-Analysis. J Clin Oncol 2021;39:2574-85. [Crossref] [PubMed]
- Kuchuk M, Kuchuk I, Sabri E, et al. The incidence and clinical impact of bone metastases in non-small cell lung cancer. Lung Cancer 2015;89:197-202. [Crossref] [PubMed]
- Qin A, Zhao S, Miah A, et al. Bone Metastases, Skeletal-Related Events, and Survival in Patients With Metastatic Non-Small Cell Lung Cancer Treated With Immune Checkpoint Inhibitors. J Natl Compr Canc Netw 2021;19:915-21. [Crossref] [PubMed]
- Clézardin P, Coleman R, Puppo M, et al. Bone metastasis: mechanisms, therapies, and biomarkers. Physiol Rev 2021;101:797-855. [Crossref] [PubMed]
- Migliorini F, Maffulli N, Trivellas A, et al. Bone metastases: a comprehensive review of the literature. Mol Biol Rep 2020;47:6337-45. [Crossref] [PubMed]
- Turpin A, Duterque-Coquillaud M, Vieillard MH. Bone Metastasis: Current State of Play. Transl Oncol 2020;13:308-20. [Crossref] [PubMed]
- Wang B, Chen L, Huang C, et al. The homogeneous and heterogeneous risk factors for occurrence and prognosis in lung cancer patients with bone metastasis. J Bone Oncol 2019;17:100251. [Crossref] [PubMed]
- Katakami N, Kunikane H, Takeda K, et al. Prospective study on the incidence of bone metastasis (BM) and skeletal-related events (SREs) in patients (pts) with stage IIIB and IV lung cancer-CSP-HOR 13. J Thorac Oncol 2014;9:231-8. [Crossref] [PubMed]
- Zhang L, Hou X, Lu S, et al. Predictive significance of bone sialoprotein and osteopontin for bone metastases in resected Chinese non-small-cell lung cancer patients: a large cohort retrospective study. Lung Cancer 2010;67:114-9. [Crossref] [PubMed]
- Niu Y, Lin Y, Pang H, et al. Risk factors for bone metastasis in patients with primary lung cancer: A systematic review. Medicine (Baltimore) 2019;98:e14084. [Crossref] [PubMed]
- Collins GS, Reitsma JB, Altman DG, et al. Transparent reporting of a multivariable prediction model for individual prognosis or diagnosis (TRIPOD): the TRIPOD statement. BMJ 2015;350:g7594. [Crossref] [PubMed]
- Asamura H, Chansky K, Crowley J, et al. The International Association for the Study of Lung Cancer Lung Cancer Staging Project: Proposals for the Revision of the N Descriptors in the Forthcoming 8th Edition of the TNM Classification for Lung Cancer. J Thorac Oncol 2015;10:1675-84.
- Travis WD, Brambilla E, Noguchi M, et al. International association for the study of lung cancer/american thoracic society/european respiratory society international multidisciplinary classification of lung adenocarcinoma. J Thorac Oncol 2011;6:244-85. [Crossref] [PubMed]
- Riley RD, Ensor J, Snell KIE, et al. Calculating the sample size required for developing a clinical prediction model. BMJ 2020;368:m441. [Crossref] [PubMed]
- Coleman RE, Croucher PI, Padhani AR, et al. Bone metastases. Nat Rev Dis Primers 2020;6:83. [Crossref] [PubMed]
- Wang H, Zhang Y, Zhu H, et al. Risk factors for bone metastasis in completely resected non-small-cell lung cancer. Future Oncol 2017;13:695-704. [Crossref] [PubMed]
- Lee GD, Kim DK, Moon DH, et al. A comparison of the proposed classifications for the revision of N descriptors for non-small-cell lung cancer. Eur J Cardiothorac Surg 2016;49:580-8. [Crossref] [PubMed]
- Aksoy Y, Çıtak N, Obuz Ç, et al. Does anatomical location-based metastatic lymph node density affect prognosis in lung cancer patients? Interact Cardiovasc Thorac Surg 2021;33:541-9. [Crossref] [PubMed]
- Boudria A, Abou Faycal C, Jia T, et al. VEGF(165)b, a splice variant of VEGF-A, promotes lung tumor progression and escape from anti-angiogenic therapies through a β1 integrin/VEGFR autocrine loop. Oncogene 2019;38:1050-66. [Crossref] [PubMed]
- Guo J, Higashi K, Ueda Y, et al. VEGF-A and its isoform VEGF121 mRNA expression measured by quantitative real-time RT-PCR: correlation with F-18 FDG uptake and aggressiveness of lung adenocarcinoma: preliminary study. Ann Nucl Med 2011;25:29-36. [Crossref] [PubMed]
- Ujiie H, Kadota K, Chaft JE, et al. Solid Predominant Histologic Subtype in Resected Stage I Lung Adenocarcinoma Is an Independent Predictor of Early, Extrathoracic, Multisite Recurrence and of Poor Postrecurrence Survival. J Clin Oncol 2015;33:2877-84. [Crossref] [PubMed]
- Hung JJ, Yeh YC, Jeng WJ, et al. Predictive value of the international association for the study of lung cancer/American Thoracic Society/European Respiratory Society classification of lung adenocarcinoma in tumor recurrence and patient survival. J Clin Oncol 2014;32:2357-64. [Crossref] [PubMed]
- Hung JJ, Jeng WJ, Chou TY, et al. Prognostic value of the new International Association for the Study of Lung Cancer/American Thoracic Society/European Respiratory Society lung adenocarcinoma classification on death and recurrence in completely resected stage I lung adenocarcinoma. Ann Surg 2013;258:1079-86. [Crossref] [PubMed]
- Caso R, Sanchez-Vega F, Tan KS, et al. The Underlying Tumor Genomics of Predominant Histologic Subtypes in Lung Adenocarcinoma. J Thorac Oncol 2020;15:1844-56. [Crossref] [PubMed]
- Moreira AL, Ocampo PSS, Xia Y, et al. A Grading System for Invasive Pulmonary Adenocarcinoma: A Proposal From the International Association for the Study of Lung Cancer Pathology Committee. J Thorac Oncol 2020;15:1599-610. [Crossref] [PubMed]
- MacMahon H, Naidich DP, Goo JM, et al. Guidelines for Management of Incidental Pulmonary Nodules Detected on CT Images: From the Fleischner Society 2017. Radiology 2017;284:228-43. [Crossref] [PubMed]
- Ibuki Y, Tsutani Y, Miyata Y, et al. Preoperative predictors of distant recurrence in patients with clinical stage IA lung adenocarcinoma undergoing complete resection. Jpn J Clin Oncol 2017;47:157-63. [PubMed]
- Paz-Ares L, O'Brien MER, Mauer M, et al. VP3-2022: Pembrolizumab (pembro) versus placebo for early-stage non-small cell lung cancer (NSCLC) following complete resection and adjuvant chemotherapy (chemo) when indicated: Randomized, triple-blind, phase III EORTC-1416-LCG/ETOP 8-15 e PEARLS/KEYNOTE-091 study. Ann Oncol 2022;33:451-3. [Crossref]
- Felip E, Altorki N, Zhou C, et al. Adjuvant atezolizumab after adjuvant chemotherapy in resected stage IB-IIIA non-small-cell lung cancer (IMpower010): a randomised, multicentre, open-label, phase 3 trial. Lancet 2021;398:1344-57. [Crossref] [PubMed]
- Forde PM, Spicer J, Lu S, et al. Neoadjuvant Nivolumab plus Chemotherapy in Resectable Lung Cancer. N Engl J Med 2022;386:1973-85. [Crossref] [PubMed]
- Curigliano G, Mayer EL, Burstein HJ, et al. Cardiac toxicity from systemic cancer therapy: a comprehensive review. Prog Cardiovasc Dis 2010;53:94-104. [Crossref] [PubMed]
- LeVasseur N, Clemons M, Hutton B, et al. Bone-targeted therapy use in patients with bone metastases from lung cancer: A systematic review of randomized controlled trials. Cancer Treat Rev 2016;50:183-93. [Crossref] [PubMed]
- Guo X, Zhang C, Guo Q, et al. The homogeneous and heterogeneous risk factors for the morbidity and prognosis of bone metastasis in patients with prostate cancer. Cancer Manag Res 2018;10:1639-46. [Crossref] [PubMed]