Contribution of crosstalk of mesothelial and tumoral epithelial cells in pleural metastasis of lung cancer
Highlight box
Key findings
• Single-cell profile of pleural mesothelial cells was illustrated. Cancer-associated mesothelial cells (CAMCs) enhance the invasiveness of lung cancer cells. Epidermal growth factor receptor (EGFR) antagonist cetuximab might prevent the progression of lung cancer.
What is known and what is new?
• The pleural metastasis is common in lung cancer development, which can lead to the development of malignant pleural effusion (MPE). The mesothelial cells in pleura may play an important role in metastasis through interacting with malignant epithelial cells, which has not been systematically investigated at single-cell resolution.
• Single-cell profile of pleural mesothelial cells was demonstrated, including one predominately identified in MPE, which was referred to as CAMCs. Mesothelial cells can increase the migration ability of lung cancer cells in vitro. EGFR was identified as the key affected gene in cancer cells, which exhibited interaction with mesothelial cells and was associated with poor prognosis. Using EGFR antagonist cetuximab prevented the increased invasiveness of lung cancer cells. Moreover, epithelial mitogen (EPGN)-EGFR interaction was supported through spatial distribution analysis, revealing the significant proximity between EPGN+ mesothelial cells and EGFR+ epithelial cells.
What is the implication, and what should change now?
• Our findings highlighted the important role of mesothelial cells and their interactions with cancer cells in pleural metastasis of lung cancer, which may facilitate cancer progression and offer significant contributions towards the advancement of targeted therapies of lung cancer.
Introduction
Metastasis in pleural space frequently occurred in advanced stage of multiple cancer types and can lead to the development of malignant pleural effusion (MPE) in up to 60% of patients with pleural metastasis (1,2). MPE is most common in lung cancer, which is one of the most prevalent cancer types and the leading cause of cancer-related deaths worldwide (3,4). In addition, patients with non-small cell lung cancer (NSCLC) are more prevalent in adenocarcinoma than squamous cell carcinoma in terms of MPE development (5,6), and exhibit poor prognosis than patients with “dry” pleural metastasis (7,8).
The pleura is primarily composed of mesothelial cells. These cells form a single layer of flat cells that cover the surfaces of serous cavities, such as the peritoneal and pleural cavity. Notably, mesothelial cells exhibit a specialized property as expressing both cytokeratin, a marker that is characteristic of epithelial cells, and vimentin, a marker that is typically observed in mesenchymal cells. Traditionally, mesothelial cells were believed to act as physical barriers or regulators of lubricant substances. Recent research has uncovered additional functions of mesothelial cells, including immune regulation, synthesis and secretion of cytokines and extracellular matrix, as well as their involvement in fibrosis and serous cavity adhesion (9-12).
Nevertheless, further investigation is required to determine the function of mesothelial cells in the tumor microenvironment (TME). In recent years, numerous studies have highlighted the role of peritoneal mesothelial cells in promoting peritoneal dissemination and metastasis of various tumors in abdomen, such as gastric and ovarian cancers (13-18). Additionally, mesothelial cells might also lead to resistance to anti-tumor drugs, such as platinum (19). However, research on the tumor-promoting effects of pleural mesothelial cells in the context of lung cancer is limited, and the underlying regulatory mechanisms require further exploration.
Mesothelial cells are a relatively small constitute of lung cancer samples. The studies on transcriptomic characteristics of mesothelial cells in TME were often hindered by traditional bulk analyses because they average signals from various cells. Single-cell RNA-sequencing (scRNA-seq) quantifies the transcriptomics of individual cells, displaying advantages in characterizing subsets or rare cellular components, as well as cellular interactions in TME at pan-cancer level (20-24). Taking advantage of integrated scRNA-seq datasets, a novel subset of mesothelial cells, cancer-associated mesothelial cells (CAMCs) were identified and comprehensively analyzed by us. The interaction between CAMCs and epithelial cells in TME was further evaluated. Putative ligand-receptors involved in the interaction were utilized to construct a prediction model of prognosis. To validate our findings, the co-culture of mesothelial cells and epithelial cells in vitro, as well as RNA-seq of both cell types were conducted. In addition, spatial distribution of cells in patients’ samples derived from lung adenocarcinoma (LUAD) invading pleural were analyzed (Figure 1A). It is anticipated that our findings will offer significant contributions towards the advancement of targeted therapies of lung cancer. We present this article in accordance with the MDAR reporting checklist (available at https://tlcr.amegroups.com/article/view/10.21037/tlcr-24-118/rc).
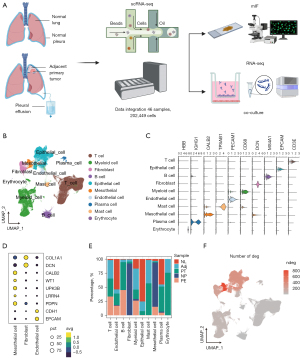
Methods
Single-cell RNA-seq and data integration
This study was performed in accordance with the Declaration of Helsinki (as revised in 2013) and was approved by the West China Hospital Ethics Committee [No. 2023(586)]. The participants have been given informed consent before taking part. The single-cell suspensions were prepared from the pleural effusion (PE) of 3 lung cancer patients under treatment at West China Hospital (Chengdu, China). In accordance with the manufacturer’s instructions in the Chromium Single Cell 3' Reagents Kits v2 User Guide, the Chromium Single Cell 3' Library & Gel Bead Kit v2 (PN-120237), Chromium Single Cell 3' Chip Kit v2 (PN-120236), and Chromium i7 Multiplex Kit (PN-120262) were used. 1× phosphate-buffered saline (PBS) + 0.04% BSA were utilized to wash single-cell suspension twice. Cell number and concentration were confirmed with TC20 Automated Cell Counter. Cells were then subjected immediately onto a 10× Genomics Chromium Controller machine for gel beads-in-emulsion (GEM) generation. 10× Genomics Chromium Single Cell 3' reagent kit (V2 chemistry) was used to prepare barcoded complementary DNAs (cDNAs), which was subsequently recovered, purified, and amplified to generate sufficient quantities for library preparation. We then took advantage of Agilent Bioanalyzer 2100 to assess library quality and concentration. Libraries were run on the NovaSeq platform of Illumina for PE150 sequencing. Next, we retrieved publicly available scRNA-seq datasets. FASTQ files from 10× Genomics were aligned and quantified along the GRCh38 human reference genome through Cell Ranger. We followed the scRNA-seq data analysis pipeline as we described previously (23,25,26), Briefly, we took advantage of the Read10X function from the Seurat package (Version 4.0.4) and read.table function to read the output of the cellranger and count matrix, respectively. Doubletfinder was utilized to remove the potential doublets. The merge function was used to integrate our data and publicly available datasets. Quality control was then applied to the aggregated data. Cells with less than 200 detected genes and more than 30% mitochondrial genes were removed. We also excluded cells with more than 6,000 detected genes to avoid possible doublets. After filtering, 202,449 cells were involved in further investigation. SCTransform was applied for normalization. Principal component analysis (PCA) was employed to incorporate highly variable features, reducing the dataset’s dimensionality. The first 30 PCs were identified for analysis. We then took advantage of the RunFastMNN function in SeuratWrappers package (Version 0.3.0) to remove batch effects. FindNeighbors and FindCluster functions were used for clustering analysis, based on the edge weights between any two cells. A shared nearest-neighbor graph was produced by the Louvain algorithm. The uniform manifold approximation and projection (UMAP) method was used to visualize the identified clusters. A similar procedure was applied for subclustering analysis, including normalization, variable expressed feature, and dimension reduction batch correction. Differentially expressed features of the clusters were identified with the FindAllMarkers function, which utilized the default nonparametric Wilcoxon rank sum test with Bonferroni correction. The raw sequencing data from this study have been deposited in the Genome Sequence Archive in BIG Data Center, Beijing Institute of Genomics, Chinese Academy of Sciences (https://ngdc.cncb.ac.cn/gsub/, access number: PRJCA021707). Other scRNA-seq data were retrieved from Gene Expression Omnibus (https://www.ncbi.nlm.nih.gov/geo/). The scRNA-seq data were available under accession number [GSE227136, GSE131907 (27), GSE134355 (28), PRJNA668853 (29), E-MTAB-8530 (30)]. The RNA-seq of A549 and MET-5A after co-culturing are deposited in Gene Expression Omnibus (accession No. GSE242951). The integrated data are also available from the corresponding author once needed.
Statistical analysis
Statistical analyses were performed using the R (version 4.1.3). Comparisons of quantitative variable were performed using the Mann-Whitney U test or student test. All statistical tests were two-sided, and significance was defined as P<0.05.
Cellphonedb analysis
Cellphonedb was applied to analyze cell interactions between cellular components. Input files for the statistical analysis function were the raw count matrix extracted from the Seurat object and the annotation file of the cells. We then took advantage of the pheatmap package (Version 1.0.12) and the Circlize package (Version 0.4.14) to illustrate the frequency of interactions between two cell types. The potential interaction between ligand and receptor was visualized using the dot_plot function from the Cellphonedb. Significant ligand-receptor pairs (P<0.05) were extracted.
NicheNet analysis
NicheNet (Version 1.0.0) was applied to identify potential ligands which drove the subtypes of fibroblasts, The top 15 differentially expressed genes (DEGs) were ordered, and mesothelial cells were treated as potential ligands. Sender cells from mesothelial cells and receptor cells from fibroblasts were included to construct the expressed ligand-receptor interactions and calculate the ligand activity. We also took advantage of the active_ligand_traget_links and active_ligand_receptor_links functions to compute the potential intensity of the interactions.
Cellchat analysis
CellChat (Version 0.0.2) was ascertained by evaluating the expression of pairs of ligands and receptors between cellular components. We examined the interaction between different cellular components, and gene expression of 0.2 was set as the valid cutoff point.
Trajectory analysis
To investigate the dynamic biological processes of mesothelial cells during the tumor progression, we applied the Monocle (Version 2.22.0) algorithm. We used the NewCellDataset function to create a new object from the transcript count data of the included cells for the monocle. estimateSizeFactors and estimateDispersions functions were then employed for further analysis. Order genes were expressed in at least 10% of cells of the dataset and with a P<0.01 identified by differentialGeneTest function. We then took advantage of the ReduceDimension function to reduce the space to two dimensions and the orderCells function to order the cells based on order genes. Pseudotime-dependent genes were identified by the differentialGeneTest function, and smooth expression curves were illustrated with the plot_pseudotime_heatmap function.
Enrichment analysis
Gene Ontology (GO) enrichment analysis was performed through the clusterProfiler packages.
Survival analysis
mRNA expression counts and clinical information from The Cancer Genome Atlas (TCGA) were downloaded from https://portal.gdc.cancer.gov/. We used Survival (Version 2.42-3) and Survminer (Version 0.4.9) packages for analysis and visualization. Univariate Cox regression analyses were implemented on ligand-receptor genes between mesothelial cells and epithelial cells to identify the predictor of overall survival (OS).
Prediction model
The survival prediction data for this study were collected from the cBioPortal database (http://www.cbioportal.org/). A total of 517 cases of LUAD with mRNA expression data were downloaded. Cases with incomplete survival information or short follow-up periods were excluded, resulting in data from 501 cases being included in this study.
The primary endpoint of the study was OS. we developed a prediction model based on the clinical information and 21 interaction genes. The collected clinical information included age, gender, TNM staging, location (central/peripheral of the lung), residual disease, and smoking (status and packages*years). The included genes were selected from ligand-receptor pairs between mesothelial cells and epithelial cells that were more enhanced in cancer subtypes. Cox analysis was performed on the ligand-receptor pairs, and finally, 21 input genes were selected.
The data preprocessing for this study was performed using Python 3.7.2. As per the limitations of machine learning algorithms, nominal categorical variables were transformed into dummy variables. During the data imputation process, the random forest regressor was used.
The data were stratified-randomly split into train and validation cohorts with a ratio of 7:3 before adjusting the hyperparameters. Only the train cohort data was used to determine the hyperparameters. The best values for the hyperparameters were chosen based on the mean results of 10-fold cross-validation.
Two curves were used to evaluate and illustrate the performance of the models: the receiver operating characteristic (ROC) curve and the calibration curve. The ROC curve assesses the discrimination between alive and dead cases, and the quantitatively comparable metric is the area under the curve (AUC). The calibration curve evaluates the agreement between predicted survival and actual survival. To provide a better understanding of the prediction model, the feature importance of the random forest model, measured by Gini coefficients, was exported.
The predicted probability of 3-year OS was used as risk scores to gain a better understanding of the model. The validation cohorts were divided into four risk groups based on these scores. Kaplan-Meier curves were used to compare the survival outcomes between the risk groups and TNM stage.
Cell culture
The human NSCLC cell line (A549, CVCL_0023; NCI-H1299, CRL-5803) and human pleural mesothelial cell line (MET-5A, CVCL_3749) obtained from Procell Life Science & Technology in China were used for the wet experimental validation. Both cell lines have been authenticated using short tandem repeats (STR) or single nucleotide polymorphism (SNP) profiling within the last three years. All experiments were performed with mycoplasma-free cells. Both cell lines were cultured in RPMI 1640 medium supplemented with 10% fetal bovine serum (FBS) and 1% penicillin/streptomycin. The cells were maintained in a cell incubator at 37 ℃ with a 5% CO2 atmosphere. To establish co-culture conditions, Transwell chambers with 0.4 µm pores (Corning, USA) were employed. These chambers allow cells to communicate through the exchange of secreted cytokines while physically separating them.
Proliferation assay
The proliferation assay utilizing the cell counting kit-8 (CCK-8) method was conducted to evaluate the proliferation rate of A549 cells. After co-culture or culture alone for 24 or 48 hours as described before, LUAD cells were seeded at a density of 5,000 cells/well in a 96-well plate and allowed to adhere in unchanged culture media. CCK-8 working solution was added to each well and incubated for 1 hour. Absorbance readings were measured at 450 nm using a microplate reader after the incubation period.
Invasion assay
To assess the invasive potential of the NSCLC cells (A549 and NCI-H1299), we performed an in vitro invasion assay using Transwell inserts with an 8-µm porous polycarbonate membrane (Corning, USA). Prior to the assay, mesothelial cells (MET-5A) were seeded into 24-well plate. When 70% confluence of the mesothelial cells, the Transwell inserts were coated with a thin layer of Matrigel (Corning, USA) diluted in serum-free medium at a concentration of 1:8 and allowed to solidify for 30 min at 37 ℃. Following this, a total of 3×104 A549 or NCI-H1299 cells in 200 µL serum-free medium, or in 200 µL serum-free medium with 0.5 mg/mL cetuximab (Erbitux), were seeded in the upper chamber of the Transwell inserts, while the lower chamber was filled with 800 µL medium containing 10% FBS.
The assembled Transwell plate were then incubated at 37 ℃ in a humidified atmosphere with 5% CO2 for 24 hours. Subsequently, non-invading cells were gently removed from the upper surface of the membrane using a cotton swab. The invaded cells on the lower surface of the membrane were fixed with 4% paraformaldehyde and stained with 0.1% crystal violet. The number of invaded cells was quantified by capturing images in randomly selected fields of view using an Olympus DP80 under magnification 100×. Then, ImageJ software was utilized to automatically calculate the ratio of stained cell areas to the total field areas in each view. The experiments were repeated 3 times to verify our findings.
Multiplexed immunofluorescence (mIF) analysis
Tissue samples of LUAD invading pleural were obtained from West China Hospital. These samples were processed into paraffin blocks and cut into FFPE sections with 5 µm thick. We took advantage of an Opal 7-color kit (Akoya Bioscience, Marlborough, USA; NEL801001KT) to conduct multiplex immunohistochemistry (IHC) staining. The markers PANCK (4545S, Cell Signaling Technology, Danvers, USA; 1:200, Opal 620), Calretinin (A9807, ABclonal, Wuhan, China; 1:100, Opal 690), epithelial mitogen (EPGN) (XY-KT5767R, Shanghai Xuanke Biotechnology, China; 1:200, Opal 520), epidermal growth factor receptor (EGFR) (ab52894, Abcam, Boston, USA; 1:200, Opal 570) were elevated by IHC. The sample sections were dewaxed with xylene for 20 minutes and rehydrated with ethanol. We then performed microwave treatment for antigen retrieval with buffer (pH 9.0). Sections were cooled for 30 minutes to room temperature. An antibody diluent/block (72424205; Akyoya Bioscience) was utilized at room temperature for 10 min to block endogenous peroxidase activity. Slides were incubated with a primary antibody at room temperature for 1 hour, followed by secondary regents at 37 ℃ for 20 min a tyramide signal amplification reagent at room temperature for 10 min (Opal 520, Opal 570, Opal 620, Opal 690, Akoya Bioscience, 1:100). After all markers were stained, MWT antigen retrieval was performed. DAPI (Akoya Bioscience, 1:5) was used at room temperature for 5 min for nuclear staining. Slides were mounted by anti-Fade fluorescence mounting medium (ab104135, Abcam) and stored at 4 ℃ until image acquisition. Slides were scanned by a confocal microscope (TCS SP8, Leica, Wetzlar, German) with Vectra Polaris (PerkinElmer, Waltham, USA) system. The percentage of positively stained cells among all nucleated cells were determined. Multispectral image unmixing was achieved by QuPath software (Version 3.0) (31). Next, we identified 4',6-diamidino-2-phenylindole (DAPI)-positive cells by the “cell detection” command. Each single channel threshold was selected. As a result, all detected cells were divided into different subgroups for further analysis. The defective samples or areas with staining artifacts were reanalyzed or excluded.
RNA-seq and analysis
After co-culture, the RNA of LUAD and pleural mesothelial cells of control group and co-culture group was respectively extracted with RNAsimple Total RNA Kit (Tiangen, China). After library construction, Qubit 3.0 fluorometer dsDNA HS Assay (Thermo Fisher Scientific, USA) was utilized to quantify concentration of the resulting sequencing libraries, while the size distribution was analyzed by Agilent BioAnalyzer (Agilent, USA). Sequencing was carried out on the Illumina Novaseq 6000 platform based on Illumina-provided protocols for 2×150 paired-end sequencing at Sequanta Technologies Co., Ltd. (Shanghai, China).
Beginning with the raw reads from RNA-seq, we firstly retained the qualified reads which passed the quality control step by Trim Galore (v0.6.7). Low base quality reads were excluded for further anaysis. By taking advantage of HISAT2 (v2.2.1), clean reads of each sample were then aligned to the GRCh38 human reference genome retrieved from GENECODE. Subsequently, gene and transcript expression were estimated with featureCounts (v2.0.1) (32). Differential expression analysis were performed using the “DESeq2” package (33). RNAs with false discovery rate (FDR) values <0.05 and |log2foldchange| >0.25 were considered DEGs between control and co-culture groups.
Results
Landscape of TME in lung cancer using integrated scRNA-seq data
To identify the TME of lung cancer, we performed scRNA-seq derived from PE of 3 LUAD patients. We also acquired the publicly available scRNA-seq data of lung cancer and integrated our data with them (28-30,34). Subsequently, a scRNA-seq dataset was constructed, which derived from 15 primary lung tumors (PT), 11 lung tissues adjacent to tumor (Adj), 18 PE, 1 normal lung tissue (NL), and 1 normal pleural (NP) samples collected from 33 individuals. All datasets were generated using the 10× Genomics platform. After quality control and batch correction, a total of 202,449 cells were included for further investigation (Figure 1B). Unsupervised clustering of the cells was performed, resulting in the identification of 10 major cellular components using marker genes. These components included T cells (CD3E), epithelial cells (EPCAM), B cells (MS4A1), fibroblasts (DCN), myeloid cells (CD68), endothelial cells (PECAM1), mast cells (TPSAB1), mesothelial cells (CALB2), plasma cells (IGHG1), and erythrocytes (HBB) (Figure 1C). Mesothelial cells exhibited distinct marker expression, including podoplanin (PDPN), leucine rich repeat neuronal 4 (LRRN4), uroplakin 3B (UPK3B), Wilms’ Tumor Gene 1 (WT1), and calbindin 2 (CALB2), in comparison to epithelial cells and fibroblasts, thus confirming our annotation of the cellular components (Figure 1D). Upon normalizing the proportions of sample sites within cellular components, mesothelial cells were predominantly derived from PE and NP samples. Epithelial cells were mainly obtained from NL, Adj, PT, and PE samples (Figure 1E). To investigate cellular alterations during the transition from normal conditions to TME, we calculated the DEGs for each cellular component between cancer (PT and PE samples) and normal (NL, Adj, and NP samples) subtypes. Mesothelial cells exhibited the highest number of DEGs (Figure 1F), indicating their potentially essential role in the TME.
The re-cluster of mesothelial cells from different disease status
Subsequently, we isolated mesothelial cells in TME and integrated them with mesothelial cells derived from lung tissues of non-malignant diseases (NMDs), pulmonary fibrosis, for further analysis (GSE227136). A total of 6,807 mesothelial cells were re-clustered and visualized. Distinct distributions of mesothelial cells from different disease status were discovered. Intelectin 1 positive (ITLN1+) mesothelial cells, cell migration inducing hyaluronidase 1 positive (CEMIP+) mesothelial cells, and C-C motif chemokine ligand 2 positive (CCL2+) mesothelial cells were predominantly composed of mesothelial cells from normal, cancer, and NMD conditions, respectively (Figure 2A). The top features of each cluster are displayed in Figure 2B. Intelectin 1 (ITLN1) was highly expressed in ITLN1+ mesothelial cells. Previous studies have demonstrated that ITLN1 suppresses cancer cell invasion and proliferation in omental mesothelial cells during ovarian cancer progression (35). Conversely, genes associated with tumor progression and metastasis, such as cell migration inducing hyaluronidase 1 (CEMIP) (36), nuclear paraspeckle assembly transcript 1 (NEAT1) (37,38), and serum amyloid A1 (SAA1) (39,40) were upregulated in CEMIP+ mesothelial cells. Meanwhile, the expression of extracellular matrix related genes, epidermal growth factor like domain multiple 6 (EGFL6) and insulin like growth factor binding protein 7 (IGFBP7) were also increased in CEMIP+ mesothelial cells. Cytokine and chemokine related genes, CCL2 and C-X-C motif chemokine ligand 1 (CXCL1) were enriched in CCL2+ mesothelial cells. Tyrosine 3-monooxygenase/tryptophan 5-monooxygenase activation protein eta positive (YWHAH+) mesothelial cells highly expressed YWHAH, cysteine rich protein 1 (CRIP1), ezrin (EZR) and plectin (PLEC). Meanwhile, the most highly expressed genes in STMN1+ mesothelial cells were stathmin 1 (STMN1), galectin 1 (LGALS1), high mobility group box 1 (HMGB1), and high mobility group nucleosomal binding domain 2 (HMGN2). Coherently, GO enrichment analysis of highly expressed genes in CEMIP+ mesothelial cells revealed pathways related to extracellular matrix (Figure 2C). We also observed enrichment of cytokine activity and chemokine activity in CCL2+ mesothelial cells (Figure 2D).
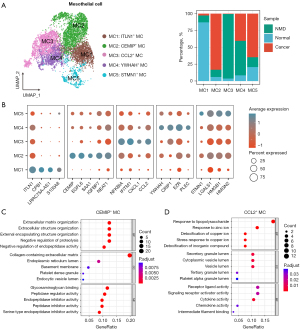
The identification of CAMCs
To illustrate the dynamic transformation of mesothelial cells originated from normal subtypes, we conducted trajectory analysis. Two distinct trajectory paths were identified. The transition path from normal to cancer mesothelial cells was separated from NMDs. Therefore, we identified CEMIP+ mesothelial cells as CAMCs, which were predominantly distributed in State 1 (Figure 3A). Next, the branch dependent gene expression programs of mesothelial cells’ development were calculated. GO analysis revealed that pathways related to Wnt signaling pathway, cell-matrix adhesion, and cell migration were enriched during the transition from normal mesothelial cells to CAMCs. Meanwhile, response to oxidative stress and lipopolysaccharide, as well as apoptotic signaling pathway were enriched during the development of NMD-associated mesothelial cells (Figure 3B). Consistently, the expression levels of CEMIP, integrin subunit alpha 3 (ITGA3), EPGN and Wnt family member 2 (WNT2) were increased in state 1, while caveolin 1 (CAV1), CXCL1, CCL2, and macrophage migration inhibitory factor (MIF) expressions were highly elevated in state 3 (Figure 3C). Notably, the expressions of CEMIP, EPGN and WNT2 were increased during the transition from normal mesothelial cells to CAMCs (Figure 3D). We further investigated the role of mesothelial cells in the TME by examining their interactions with other cells. Both CellphoneDB (Figure 3E) and CellChat (Figure 3F) analyses revealed that the interactions between mesothelial cells and other cells were high in PE, highlighting the potential pivotal role of CAMCs in the TME. Fibroblasts and epithelial cells exhibited relatively strong interactions with mesothelial cells (Figure 3E). Figure S1A shows the essential ligand-receptor pairs between CAMCs and fibroblasts identified by CellphoneDB. NicheNet analysis identified the top ligands of mesothelial cells that potentially led to DEGs (targets) between fibroblasts of cancer and normal subtypes through ligand-receptor interactions, including vascular cell adhesion molecule 1 (VCAM1), interleukin 6 (IL6), SAA1, connective tissue growth factor (CTGF), CXCL6 and CXCL2 (Figure S1B), which have been implicated in enhancing tumor progression. When taking cellular number into account, mesothelial cells most strongly interacted with epithelial cells in TME (Figure 3F). Calreticulin (CALR), MIF, LGALS3, ADP ribosylation factor 1 (ARF1), and glutathione S-transferase pi 1 (GSTP1) of epithelial cells potentially resulted in DEGs between mesothelial cells of cancer and normal subtypes through ligand-receptor interactions, transforming normal mesothelial cells to CAMCs (Figure 2A).
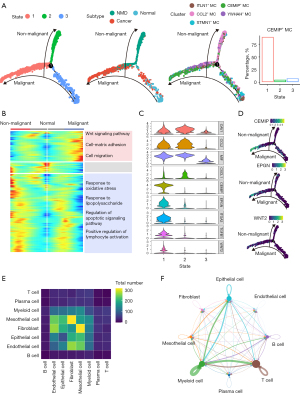
The interaction between mesothelial cells and epithelial cells in TME
Epithelial cells were subsequently dissociated and re-clustered to analyze their interactions with mesothelial cells. 5 clusters were identified. Surfactant associated protein C positive (SFTPC+) epithelial cells was mainly obtained from NL and Adj samples. The majority of ZNFX1 antisense RNA 1 positive (ZFAS1+) epithelial cells was composed of PT samples, while mucin 21 positive (MUC21+) epithelial cells and chaperonin containing TCP1 subunit 5 (CCT5+) epithelial cells predominantly consisted of epithelial cells derived from PE (Figure 4A). The top features of each cluster are displayed in Figure 4B. We specified the DEG analysis between MUC21+ epithelial cells and other epithelial cells. It is revealed that the expressions of carcinoembryonic antigen cell adhesion molecule 5 (CEACAM5), lipocalin 2 (LCN2), CD24, FAM3 metabolism regulating signaling molecule C (FAM3C), MUC21, and SRY-box transcription factor 4 (SOX4) were increased in MUC21+ epithelial cells (table available at https://cdn.amegroups.cn/static/public/tlcr-24-118-1.xlsx, Figure 4C), indicating the dedifferentiation features of this subcluster of epithelial cells. GO analysis also indicated the enrichment of cell adhesions, epithelial cell migration and response to hypoxia pathways in MUC21+ epithelial cells (Figure 4C). Meanwhile, the DEGs between CCT5+ epithelial cells and other epithelial cells was analyzed (Figure S3A). GO analysis confirmed the enrichment of adenosine triphosphate (ATP) metabolic process, RNA localization, and positive regulation of binding in CCT5+ epithelial cells (Figure S3B). The inferred interaction counts demonstrated that MUC21+ epithelial cells exhibited the strongest interactions with CEMIP+ mesothelial cells between mesothelial cells and epithelial cells (Figure 4D). To illustrate the dynamic transition of epithelial cells, we conducted trajectory analysis. Two distinct trajectory paths, Fate1 and Fate2, were identified. State 5 primarily consisted of epithelial cells retrieved from NL and Adj samples, while State 1 were predominantly composed of PE samples, mostly derived from MUC21+ epithelial cells (Figure 4E). To identify the branch dependent genes, we noticed that the expression levels of EGFR, hypoxia inducible factor 1 subunit alpha (HIF1A), MUC21 and NAD(P)HX epimerase (NAXE) were increased in state 1, while aldolase, fructose-bisphosphate A (ALDOA), capricious (CAPS), and glutathione peroxidase 1 (GPX1) expressions were highly elevated in state 4. Intriguingly, the expression level of EGFR was increased along the transition from NL/Adj to PT, and eventually to PE epithelial cells in MUC21+ epithelial cells (Figure 4F). The ligand-receptor pairs between CEMIP+ mesothelial cells and MUC21+ epithelial cells were inferred by CellphoneDB (Figure S4). These interaction genes were subjected to univariate Cox analysis using LUAD patient data acquired from TCGA datasets. Twenty-one genes including EGFR were identified as prognostic factors for LUAD patients (Figure 4G).
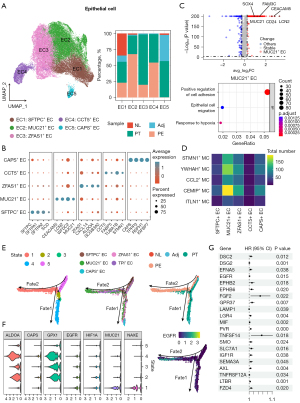
A prediction model constructed based on the interaction genes between CAMCs and epithelial cells
The enrichment score of 21 interaction genes was significantly higher in late-stage samples (stage III and IV) compared to early-stage samples (stage I and II) (P=0.004) (Figure 5A). Moreover, both early-stage and late-stage patients with higher enrichment score had significantly lower survival probabilities (P<0.001, P=0.012) (Figure 5B,5C). Considering the crucial role of the strong interactions between mesothelial cells and epithelial cells in TME, we developed a prediction model based on the 21 interaction genes and clinical information. Patients were divided it into training and validation cohorts in a ratio of 7:3. Using the Random Forest algorithm, we constructed a prediction model. The AUC for 3-year OS achieved 0.75±0.10 in the validation cohort (Figure 5D). In addition, our model outperformed the use of clinical information alone (Figure S5A). The feature importance, as measured by Gini coefficients, is presented in Figure S5B. EGFR was ranked as a vital feature in our model. Furthermore, the risk groups predicted by our model better distinguished patients with different prognoses (Figure 5E) compared to TNM stages (P=0.001), highlighting the potential clinical value of our model.
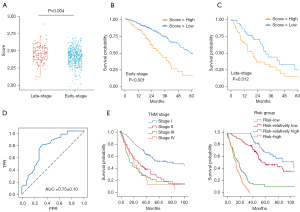
EPGN-EGFR appears as a vital ligand-receptor between CAMCs and epithelial cells in TME
To validate the interactions between mesothelial cells and epithelial cells, human pleural mesothelial cells MET-5A were co-cultured with the LUAD cell line A549. RNA-seq was performed to compare the alterations in gene expression in both mesothelial cells and epithelial cells after co-culture (Figure 6A). Consistent with the increased DEGs in CEMIP+ mesothelial cells (table available at https://cdn.amegroups.cn/static/public/tlcr-24-118-2.xlsx, Figure 2B), the expressions of NEAT1, CEMIP, and transforming growth factor beta 1 (TGFB1) were increased in mesothelial cells after co-culture (Figure S6A, table available at https://cdn.amegroups.cn/static/public/tlcr-24-118-3.xlsx). GO enrichment analysis of the upregulated DEGs in mesothelial cells after co-culture revealed pathways related to response to hypoxia and extracellular matrix (Figure 6B), which were also coherently enriched in CEMIP+ mesothelial cells (Figure 2D). The most enriched pathways in epithelial cells after co-culture also identified pathways of response to hypoxia (Figure 6C, Figure S6B, table available at https://cdn.amegroups.cn/static/public/tlcr-24-118-4.xlsx), indicating a hypoxia environment in TME. By intersecting the potential ligands identified by CellphoneDB, genes related to prognosis, and the increased DEGs after co-culture with mesothelial cells, EGFR was identified in epithelial cells (Figure 6D). The expression of EGFR in epithelial cells was significantly enhanced after co-culturing with mesothelial cells (Figure 6E). Moreover, among LUAD patients without EGFR mutation, high EGFR expression level was significantly associated with lower survival probabilities (P=0.008) (Figure 6F), while no significant association was observed in patients with EGFR mutations (Figure S6C). Even though the proliferation of the malignant epithelial cells were not significant increased after co-culturing with mesothelial cells (Figure S6D), we noticed that the invasiveness of malignant epithelial cells was significantly enhanced. EGFR antagonist cetuximab prevented malignant epithelial cells’ increased invasiveness induced by mesothelial cells (Figure 6G, Figure S6E). The expression of EPGN has been discovered to highly enriched in CAMCs (Figure 3C). Therefore, EPGN-EGFR were presumably identified as a vital ligand-receptor between CAMCs and malignant epithelial cells. To validate our hypothesis, we performed mIF on samples of LUAD invading the pleura to assess the spatial distribution of EGFR+ epithelial cells and EPGN+ mesothelial cells. In accordance with the mIF of 5 samples, EPGN+ mesothelial cells were consistently more proximal to EGFR+ epithelial cells, compared with EPGN- mesothelial cells (P=0.05). Additionally, the spatial distributions of EPGN+ mesothelial cells were also closer to EGFR+ epithelial cells (Figure 6H). However, further mechanistic investigations are necessary in the future to confirm these findings.
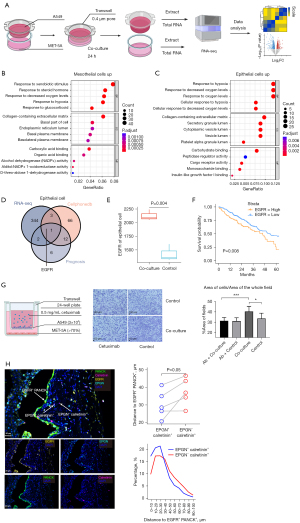
Discussion
The pleura and peritoneum are common sites of tumor metastasis, both of which are predominantly composed of mesothelial cells. Recent studies have demonstrated the critical role of mesothelial cells in the TME by directly interacting with disseminated tumor cells. Most researches have primarily focused on ovarian or gastric tumors and peritoneal mesothelial cells (17,41-43). Nevertheless, studies specifically exploring the pleura and its interaction with lung cancer cells are limited. To the best of our knowledge, this study is the first to focus on the interactions between lung cancer cells and pleural mesothelial cells, which has substantial clinical translational value.
Mesothelial cells are a relatively small constitute of TME, while playing a significant role during serous cavity dissemination (43). Taking advantage of integrated scRNA-seq data, rare cellular components in lung cancer’s microenvironment, such as mesothelial cells, could be systematically characterized. During cluster annotation, CALB2 was employed as a classification marker for mesothelial cells, as it has demonstrated good sensitivity (92%) and specificity (98%) in previous studies (44). Additionally, several other markers, including PDPN, LRRN4, UPK3B, and WT1, were used for validation (41). It is noteworthy that mesothelial cells exhibited the most DEGs when comparing cellular components between cancer and normal subtypes. It indicated that significant modifications were occurred in the mesothelium when exposed to lung cancer, also emphasizing the potentially essential role of mesothelial cells in the TME.
Various studies have reported that peritoneal mesothelial cells may undergo transformation in the presence of tumors. Tanaka et al. demonstrated that gastric cancer-derived extracellular vesicles can influence the function of mesothelial cells, promoting cancer invasion (45). Wang et al. also found that ovarian cancer can alter mesothelial cells, facilitating peritoneal metastasis (14). Some studies have referred to these transformed mesothelial cells as CAMCs, which have been implicated in promoting tumor growth through the secretion of growth factors (17,46), and converting immune cells’ function in the TME (47). However, a comprehensive depiction of pleural mesothelial cells at single-cell resolution, especially in lung cancer microenvironment, is still lacking. In the present study, we re-clustered and annotated pleural mesothelial cells derived from different disease status. Based on the trajectory analysis, we observed distinct transition paths of mesothelial cells from normal conditions to malignant diseases and NMDs. We identified CEMIP+ mesothelial cells as CAMCs. CEMIP has been demonstrated to influence tumor proliferation dedifferentiation, and metastasis, associated with poor survival (48). Genes associated with tumor progression and metastasis, such as NEAT1 (37,38) and SAA1 (39,40) were also upregulated in the CAMCs. Furthermore, the Wnt signaling pathway, cell-matrix adhesion, and cell migration pathways were enriched along the transition from normal mesothelial cells to the CAMCs, demonstrating the transformation of pleural mesothelial cells caused by the TME. Reciprocally, the expression of secretory factors like EPGN, TGFB1, and WNT2 was increased in the CAMCs. it is reasonable to speculate that they have the potential to promote tumor progression. Consistently, the intercellular communication analyses reveal a robust interaction between mesothelial cells and epithelial cells in the TME.
After re-clustering of epithelial cells, we observed the strongest interaction between the CAMCs and MUC21+ epithelial cells. MUC21+ epithelial cells highly expressed genes associated with tumor progression, such as MUC21 (49,50), HIF1A (51), MMP7 (52), CEACAM5, and LCN2 (53,54), promoting angiogenesis, EMT, as well as cancer invasion. Coherently, the invasiveness of malignant epithelial cells was enhanced after co-culturing with mesothelial cells. Given these findings, the abundant crosstalk between the CAMCs and the MUC21+ epithelial cells in lung cancer microenvironment, characterized by ligand-receptor pairs, becomes intriguing for investigation. A prognostic model was constructed based on 22 inferred ligand-receptor pairs between the CAMCs and MUC21+ epithelial cells. The model exhibited favorable performance with an AUC of 0.74±0.06 for predicting 3-year OS. For context, Liang et al. reported a survival prediction nomogram for NSCLCs with a C-index of 0.71 (55), and Huang et al. also developed one with the C-index of 0.72±0.01 (56). This indirectly verified the prognostic significance of the interaction genes between the CAMCs and malignant epithelial cells in lung cancer.
By intersecting scRNA-seq data and RNA-seq data obtained before and after co-culture, EPGN-EGFR was identified by us as an essential ligand-receptor between the CAMCs and malignant epithelial cells. EPGN, also known as epithelial mitogen or epigen, belongs to the epidermal growth factor (EGF) superfamily. Existing studies suggest that epigen is overexpressed in various cancer types, such as bladder and breast cancer (57). In addition, epigen has shown promising potential as a predictor of recurrence and survival in breast cancer (58). However, there have been no investigations specifically focusing on mesothelium-derived epigen, which might also be essential in cancer progression. EGFR serves as the direct receptor for epigen (59). In various cancers, EGFR overexpression or activation has been linked to enhanced cancer cell invasiveness (60), poorer survival outcomes (61), and the promotion of EMT in cancers like head and neck squamous cell carcinoma (HNSCC) (62), pancreatic cancer (63), and NSCLC (64,65). Currently, most studies have focused on the EGFR mutations in lung cancer because patients with EGFR-mutant typically respond well to EGFR tyrosine kinase inhibitors (TKIs) (66,67). Our study has revealed that pleural mesothelial cells potentially enhanced the invasiveness in LUAD cells, with EPGN/EGFR identified as a key ligand-receptor pair involved in this process, indicating the potential biomarker value for EGFR expression in lung cancer, which would also be detected through noninvasive approach (68). We also confirmed the close proximity of EPGN+ mesothelial cells and EGFR+ cancer cells in human LUAD samples with pleural invasion. Thus, inhibiting the EPGN/EGFR molecule pairs might potentially mitigate the impact of the mesothelium on cancer progression. Coherently, we noticed that EGFR antagonist cetuximab could prevent the lung cancer cells’ enhanced invasiveness induced by mesothelial cells in vitro. Cetuximab has shown its significance in managing advanced colorectal and head and neck cancers (69,70). However, cetuximab is not widely applied in NSCLC due to its marginal clinical benefit (71-73). Our study proposed that cetuximab might play an essential role in preventing pleural metastasis in NSCLC. Patients with pleural metastasis might be more likely to benefit from cetuximab.
Conclusions
This study represents the first investigation of the interaction between LUAD and pleural mesothelium, providing potential targets for further research. However, it is important to acknowledge several limitations. First, the availability of scRNA-seq data from LUAD samples with PE is limited, which may introduce bias and reduce generalizability. Future studies should aim to include diverse samples from different races, countries, and age groups to increase the robustness of the findings. Second, there may be potential differences between the in vitro co-culture model and the real clinical scenario. Further research involving animal models and clinical trials in the future will be necessary to confirm our findings and to establish the clinical relevance of the identified targets.
Acknowledgments
Funding: This study was supported by
Footnote
Reporting Checklist: The authors have completed the MDAR reporting checklist. Available at https://tlcr.amegroups.com/article/view/10.21037/tlcr-24-118/rc
Data Sharing Statement: Available at https://tlcr.amegroups.com/article/view/10.21037/tlcr-24-118/dss
Peer Review File: Available at https://tlcr.amegroups.com/article/view/10.21037/tlcr-24-118/prf
Conflicts of Interest: All authors have completed the ICMJE uniform disclosure form (available at https://tlcr.amegroups.com/article/view/10.21037/tlcr-24-118/coif). The authors have no conflicts of interest to declare.
Ethical Statement: The authors are accountable for all aspects of the work in ensuring that questions related to the accuracy or integrity of any part of the work are appropriately investigated and resolved. This study was performed in accordance with the Declaration of Helsinki (as revised in 2013) and was approved by the West China Hospital Ethics Committee [No. 2023(586)]. The participants have been given informed consent before taking part.
Open Access Statement: This is an Open Access article distributed in accordance with the Creative Commons Attribution-NonCommercial-NoDerivs 4.0 International License (CC BY-NC-ND 4.0), which permits the non-commercial replication and distribution of the article with the strict proviso that no changes or edits are made and the original work is properly cited (including links to both the formal publication through the relevant DOI and the license). See: https://creativecommons.org/licenses/by-nc-nd/4.0/.
References
- Tsou PH, Chiang PH, Lin ZT, et al. Rapid purification of lung cancer cells in pleural effusion through spiral microfluidic channels for diagnosis improvement. Lab Chip 2020;20:4007-15. [Crossref] [PubMed]
- Stathopoulos GT, Kalomenidis I. Malignant pleural effusion: tumor-host interactions unleashed. Am J Respir Crit Care Med 2012;186:487-92. [Crossref] [PubMed]
- Sung H, Ferlay J, Siegel RL, et al. Global Cancer Statistics 2020: GLOBOCAN Estimates of Incidence and Mortality Worldwide for 36 Cancers in 185 Countries. CA Cancer J Clin 2021;71:209-49. [Crossref] [PubMed]
- Xia C, Dong X, Li H, et al. Cancer statistics in China and United States, 2022: profiles, trends, and determinants. Chin Med J (Engl) 2022;135:584-90. [Crossref] [PubMed]
- Luo L, Deng S, Tang W, et al. Recruitment of IL-1β-producing intermediate monocytes enhanced by C5a contributes to the development of malignant pleural effusion. Thorac Cancer 2022;13:811-23. [Crossref] [PubMed]
- Dorry M, Davidson K, Dash R, et al. Pleural effusions associated with squamous cell lung carcinoma have a low diagnostic yield and a poor prognosis. Transl Lung Cancer Res 2021;10:2500-8. [Crossref] [PubMed]
- Morgensztern D, Waqar S, Subramanian J, et al. Prognostic impact of malignant pleural effusion at presentation in patients with metastatic non-small-cell lung cancer. J Thorac Oncol 2012;7:1485-9. [Crossref] [PubMed]
- Skok K, Hladnik G, Grm A, et al. Malignant Pleural Effusion and Its Current Management: A Review. Medicina (Kaunas) 2019;55:490. [Crossref] [PubMed]
- Yung S, Li FK, Chan TM. Peritoneal mesothelial cell culture and biology. Perit Dial Int 2006;26:162-73.
- Chen Z, Yang X, Bi G, et al. Ligand-receptor interaction atlas within and between tumor cells and T cells in lung adenocarcinoma. Int J Biol Sci 2020;16:2205-19. [Crossref] [PubMed]
- Mutsaers SE. Mesothelial cells: their structure, function and role in serosal repair. Respirology 2002;7:171-91. [Crossref] [PubMed]
- Xiao G, Wang X, Wang J, et al. CXCL16/CXCR6 chemokine signaling mediates breast cancer progression by pERK1/2-dependent mechanisms. Oncotarget 2015;6:14165-78. [Crossref] [PubMed]
- Worzfeld T, Pogge von Strandmann E, Huber M, et al. The Unique Molecular and Cellular Microenvironment of Ovarian Cancer. Front Oncol 2017;7:24. [Crossref] [PubMed]
- Wang L, Xu Z, Hu C, et al. Peritoneal metastatic gastric carcinoma cells exhibit more malignant behavior when co-cultured with HMrSV5 cells. Aging (Albany NY) 2020;12:3238-48. [Crossref] [PubMed]
- Zhang Y, Liu T, Hu X, et al. CellCall: integrating paired ligand-receptor and transcription factor activities for cell-cell communication. Nucleic Acids Res 2021;49:8520-34. [Crossref] [PubMed]
- Li Q, Li B, Li Q, et al. Exosomal miR-21-5p derived from gastric cancer promotes peritoneal metastasis via mesothelial-to-mesenchymal transition. Cell Death Dis 2018;9:854. [Crossref] [PubMed]
- Qian J, LeSavage BL, Hubka KM, et al. Cancer-associated mesothelial cells promote ovarian cancer chemoresistance through paracrine osteopontin signaling. J Clin Invest 2021;131:e146186. [Crossref] [PubMed]
- Wu H, Xiang Z, Huang G, et al. BGN/FAP/STAT3 positive feedback loop mediated mutual interaction between tumor cells and mesothelial cells contributes to peritoneal metastasis of gastric cancer. Int J Biol Sci 2023;19:465-83. [Crossref] [PubMed]
- Yoshihara M, Kajiyama H, Yokoi A, et al. Ovarian cancer-associated mesothelial cells induce acquired platinum-resistance in peritoneal metastasis via the FN1/Akt signaling pathway. Int J Cancer 2020;146:2268-80. [Crossref] [PubMed]
- Li PH, Kong XY, He YZ, et al. Recent developments in application of single-cell RNA sequencing in the tumour immune microenvironment and cancer therapy. Mil Med Res 2022;9:52. [Crossref] [PubMed]
- Zheng L, Qin S, Si W, et al. Pan-cancer single-cell landscape of tumor-infiltrating T cells. Science 2021;374:abe6474. [Crossref] [PubMed]
- Cheng S, Li Z, Gao R, et al. A pan-cancer single-cell transcriptional atlas of tumor infiltrating myeloid cells. Cell 2021;184:792-809.e23. [Crossref] [PubMed]
- Luo H, Xia X, Huang LB, et al. Pan-cancer single-cell analysis reveals the heterogeneity and plasticity of cancer-associated fibroblasts in the tumor microenvironment. Nat Commun 2022;13:6619. [Crossref] [PubMed]
- Yin D, Zhou T, Xia X, Han C, Liu Z, Li Q, et al. Novel insights into HBV-hepatocellular carcinoma at single-cell sequencing. MedComm – Oncology 2023;2:e60.
- Luo H, Xia X, Kim GD, et al. Characterizing dedifferentiation of thyroid cancer by integrated analysis. Sci Adv 2021;7:eabf3657. [Crossref] [PubMed]
- Cao M, Deng Y, Deng Y, et al. Characterization of immature ovarian teratomas through single-cell transcriptome. Front Immunol 2023;14:1131814. [Crossref] [PubMed]
- Kim N, Kim HK, Lee K, et al. Single-cell RNA sequencing demonstrates the molecular and cellular reprogramming of metastatic lung adenocarcinoma. Nat Commun 2020;11:2285. [Crossref] [PubMed]
- Han X, Zhou Z, Fei L, et al. Construction of a human cell landscape at single-cell level. Nature 2020;581:303-9. [Crossref] [PubMed]
- Jeong HO, Lee H, Kim H, et al. Cellular plasticity and immune microenvironment of malignant pleural effusion are associated with EGFR-TKI resistance in non-small-cell lung carcinoma. iScience 2022;25:105358. [Crossref] [PubMed]
- Dong Y, Wang Z, Shi Q. Liquid Biopsy Based Single-Cell Transcriptome Profiling Characterizes Heterogeneity of Disseminated Tumor Cells from Lung Adenocarcinoma. Proteomics 2020;20:e1900224. [Crossref] [PubMed]
- Bankhead P, Loughrey MB, Fernández JA, et al. QuPath: Open source software for digital pathology image analysis. Sci Rep 2017;7:16878. [Crossref] [PubMed]
- Liao Y, Smyth GK, Shi W. featureCounts: an efficient general purpose program for assigning sequence reads to genomic features. Bioinformatics 2014;30:923-30. [Crossref] [PubMed]
- Love MI, Huber W, Anders S. Moderated estimation of fold change and dispersion for RNA-seq data with DESeq2. Genome Biol 2014;15:550. [Crossref] [PubMed]
- Ahn YH, Kim JS. Long Non-Coding RNAs as Regulators of Interactions between Cancer-Associated Fibroblasts and Cancer Cells in the Tumor Microenvironment. Int J Mol Sci 2020;21:7484. [Crossref] [PubMed]
- Au-Yeung CL, Yeung TL, Achreja A, et al. ITLN1 modulates invasive potential and metabolic reprogramming of ovarian cancer cells in omental microenvironment. Nat Commun 2020;11:3546. [Crossref] [PubMed]
- Hua Q, Zhang B, Xu G, et al. CEMIP, a novel adaptor protein of OGT, promotes colorectal cancer metastasis through glutamine metabolic reprogramming via reciprocal regulation of β-catenin. Oncogene 2021;40:6443-55. [Crossref] [PubMed]
- Tang H, Liang Y, Anders RA, et al. PD-L1 on host cells is essential for PD-L1 blockade-mediated tumor regression. J Clin Invest 2018;128:580-8. [Crossref] [PubMed]
- Jiang P, Chen A, Wu X, et al. NEAT1 acts as an inducer of cancer stem cell-like phenotypes in NSCLC by inhibiting EGCG-upregulated CTR1. J Cell Physiol 2018;233:4852-63. [Crossref] [PubMed]
- Milan E, Lazzari C, Anand S, et al. SAA1 is over-expressed in plasma of non small cell lung cancer patients with poor outcome after treatment with epidermal growth factor receptor tyrosine-kinase inhibitors. J Proteomics 2012;76:91-101. [Crossref] [PubMed]
- Sung HJ, Ahn JM, Yoon YH, et al. Identification and validation of SAA as a potential lung cancer biomarker and its involvement in metastatic pathogenesis of lung cancer. J Proteome Res 2011;10:1383-95. [Crossref] [PubMed]
- Eum HH, Kwon M, Ryu D, et al. Tumor-promoting macrophages prevail in malignant ascites of advanced gastric cancer. Exp Mol Med 2020;52:1976-88. [Crossref] [PubMed]
- Loessner D, Rockstroh A, Shokoohmand A, et al. A 3D tumor microenvironment regulates cell proliferation, peritoneal growth and expression patterns. Biomaterials 2019;190-191:63-75. [Crossref] [PubMed]
- Zheng A, Wei Y, Zhao Y, et al. The role of cancer-associated mesothelial cells in the progression and therapy of ovarian cancer. Front Immunol 2022;13:1013506. [Crossref] [PubMed]
- Cury PM, Butcher DN, Fisher C, et al. Value of the mesothelium-associated antibodies thrombomodulin, cytokeratin 5/6, calretinin, and CD44H in distinguishing epithelioid pleural mesothelioma from adenocarcinoma metastatic to the pleura. Mod Pathol 2000;13:107-12. [Crossref] [PubMed]
- Tanaka M, Kuriyama S, Itoh G, et al. Mesothelial Cells Create a Novel Tissue Niche That Facilitates Gastric Cancer Invasion. Cancer Res 2017;77:684-95. [Crossref] [PubMed]
- Chauvin M, Meinsohn MC, Dasari S, et al. Cancer-associated mesothelial cells are regulated by the anti-Müllerian hormone axis. Cell Rep 2023;42:112730. [Crossref] [PubMed]
- Huang H, Wang Z, Zhang Y, et al. Mesothelial cell-derived antigen-presenting cancer-associated fibroblasts induce expansion of regulatory T cells in pancreatic cancer. Cancer Cell 2022;40:656-673.e7. [Crossref] [PubMed]
- Chen Y, Zhou H, Jiang WJ, et al. The role of CEMIP in tumors: An update based on cellular and molecular insights. Biomed Pharmacother 2022;146:112504. [Crossref] [PubMed]
- Itoh Y, Kamata-Sakurai M, Denda-Nagai K, et al. Identification and expression of human epiglycanin/MUC21: a novel transmembrane mucin. Glycobiology 2008;18:74-83. [Crossref] [PubMed]
- Yoshimoto T, Matsubara D, Soda M, et al. Mucin 21 is a key molecule involved in the incohesive growth pattern in lung adenocarcinoma. Cancer Sci 2019;110:3006-11. [Crossref] [PubMed]
- Pugh CW, Ratcliffe PJ. Regulation of angiogenesis by hypoxia: role of the HIF system. Nat Med 2003;9:677-84. [Crossref] [PubMed]
- Shiomi T, Okada Y. MT1-MMP and MMP-7 in invasion and metastasis of human cancers. Cancer Metastasis Rev 2003;22:145-52. [Crossref] [PubMed]
- Beauchemin N, Arabzadeh A. Carcinoembryonic antigen-related cell adhesion molecules (CEACAMs) in cancer progression and metastasis. Cancer Metastasis Rev 2013;32:643-71. [Crossref] [PubMed]
- Rodvold JJ, Mahadevan NR, Zanetti M. Lipocalin 2 in cancer: when good immunity goes bad. Cancer Lett 2012;316:132-8. [Crossref] [PubMed]
- Liang W, Zhang L, Jiang G, et al. Development and validation of a nomogram for predicting survival in patients with resected non-small-cell lung cancer. J Clin Oncol 2015;33:861-9. [Crossref] [PubMed]
- Huang Y, Liu Z, He L, et al. Radiomics Signature: A Potential Biomarker for the Prediction of Disease-Free Survival in Early-Stage (I or II) Non-Small Cell Lung Cancer. Radiology 2016;281:947-57. [Crossref] [PubMed]
- Schneider MR, Yarden Y. Structure and function of epigen, the last EGFR ligand. Semin Cell Dev Biol 2014;28:57-61. [Crossref] [PubMed]
- McIntyre E, Blackburn E, Brown PJ, et al. The complete family of epidermal growth factor receptors and their ligands are co-ordinately expressed in breast cancer. Breast Cancer Res Treat 2010;122:105-10. [Crossref] [PubMed]
- Kochupurakkal BS, Harari D, Di-Segni A, et al. Epigen, the last ligand of ErbB receptors, reveals intricate relationships between affinity and mitogenicity. J Biol Chem 2005;280:8503-12. [Crossref] [PubMed]
- Chen J, Zeng F, Forrester SJ, et al. Expression and Function of the Epidermal Growth Factor Receptor in Physiology and Disease. Physiol Rev 2016;96:1025-69. [Crossref] [PubMed]
- Shi Y, Du L, Lin L, et al. Tumour-associated mesenchymal stem/stromal cells: emerging therapeutic targets. Nat Rev Drug Discov 2017;16:35-52. [Crossref] [PubMed]
- Schinke H, Shi E, Lin Z, et al. A transcriptomic map of EGFR-induced epithelial-to-mesenchymal transition identifies prognostic and therapeutic targets for head and neck cancer. Mol Cancer 2022;21:178. [Crossref] [PubMed]
- Sheng W, Chen C, Dong M, et al. Calreticulin promotes EGF-induced EMT in pancreatic cancer cells via Integrin/EGFR-ERK/MAPK signaling pathway. Cell Death Dis 2017;8:e3147. [Crossref] [PubMed]
- Jeong YJ, Park YY, Park KK, et al. Bee Venom Suppresses EGF-Induced Epithelial-Mesenchymal Transition and Tumor Invasion in Lung Cancer Cells. Am J Chin Med 2019;47:1869-83. [Crossref] [PubMed]
- Buonato JM, Lazzara MJ. ERK1/2 blockade prevents epithelial-mesenchymal transition in lung cancer cells and promotes their sensitivity to EGFR inhibition. Cancer Res 2014;74:309-19. [Crossref] [PubMed]
- Mok TS, Wu YL, Thongprasert S, et al. Gefitinib or carboplatin-paclitaxel in pulmonary adenocarcinoma. N Engl J Med 2009;361:947-57. [Crossref] [PubMed]
- Soria JC, Ohe Y, Vansteenkiste J, et al. Osimertinib in Untreated EGFR-Mutated Advanced Non-Small-Cell Lung Cancer. N Engl J Med 2018;378:113-25. [Crossref] [PubMed]
- Bao H, Min L, Bu F, et al. Recent advances of liquid biopsy: Interdisciplinary strategies toward clinical decision-making. Interdisciplinary Medicine 2023;1:e20230021.
- Biller LH, Schrag D. Diagnosis and Treatment of Metastatic Colorectal Cancer: A Review. JAMA 2021;325:669-85. [Crossref] [PubMed]
- Johnson DE, Burtness B, Leemans CR, et al. Head and neck squamous cell carcinoma. Nat Rev Dis Primers 2020;6:92. [Crossref] [PubMed]
- Mazzarella L, Guida A, Curigliano G. Cetuximab for treating non-small cell lung cancer. Expert Opin Biol Ther 2018;18:483-93. [Crossref] [PubMed]
- Bradley JD, Hu C, Komaki RR, et al. Long-Term Results of NRG Oncology RTOG 0617: Standard- Versus High-Dose Chemoradiotherapy With or Without Cetuximab for Unresectable Stage III Non-Small-Cell Lung Cancer. J Clin Oncol 2020;38:706-14. [Crossref] [PubMed]
- Pirker R, Pereira JR, von Pawel J, et al. EGFR expression as a predictor of survival for first-line chemotherapy plus cetuximab in patients with advanced non-small-cell lung cancer: analysis of data from the phase 3 FLEX study. Lancet Oncol 2012;13:33-42. [Crossref] [PubMed]