Clinical predictors of severe radiation pneumonitis in patients undergoing thoracic radiotherapy for lung cancer
Highlight box
Key findings
• Severe radiation pneumonitis (RP) was up to 9.4%. The standardized steroids could contribute to a decline the incidence of severe RP (14.8% vs. 34.8%). Subclinical interstitial lung disease (ILD), diffusing capacity of the lung for carbon monoxide, ipsilateral lung volume exceeding 20 Gy (V20), and standardized steroids could affect the incidence of severe RP.
What is known, and what is new?
• The rate of symptomatic RP in patients with lung cancer receiving radiotherapy is 5–37%. Different degrees of RP can lead to varying results in RP prognosis. Effectively reducing the occurrence of severe RP through providing standardized treatment is an urgent clinical need.
• We collected and analyzed the data related to RP patients who received radiotherapy. On the basis of screening methods, we identified the best clinical prognostic model via a variety of methods to improve the treatment of patients and inform clinical recommendations.
What is the implication, and what should change now?
• Patients with subclinical ILD and ipsilateral lung V20 >40% of radiotherapy could increase the risk of grade 3 or higher RP, and with a better lung diffusion function and standardized steroids treatment for RP could decrease the risk of grade 3 or higher RP.
Introduction
Background
Acute radiation pneumonitis (RP) is one of the most common adverse events observed when thoracic tumors are treated with radiotherapy. Multi-institutional clinical data indicate a 5–37% rate of symptomatic RP in patients with lung cancer (1,2). Different degrees of RP result in significant disparities in characteristics and prognosis. The Common Terminology Criteria for Adverse Events (CTCAE) 5.0 classifies RP into five grades according to clinical characteristics and imaging (3). Severe RP including grade 3 or higher RP can significantly impact patient survival and manifest as severe dyspnea, hypoxia, cough, and coinfection. Retrospective studies conducted in Japan and China both found a 21% mortality rate in patients with lung cancer and steroids therapy for RP, with the majority of deaths resulting from respiratory failure from the entirety of the lung tissue being affected (4,5). Although glucocorticoids have been used as a symptomatic treatment for RP and shown to provide relief, with research indicating 93% of RP patients obtain symptomatic relief and 71% achieve remission after steroid therapy (4), there is no optimal drug regimen for treating RP (6). Therefore, effectively reducing the occurrence of severe RP and providing standardized treatment to decrease mortality in patients with RP remains an urgent clinical need.
Literature review
The application of emerging radiation technologies such as image-guided radiation therapy (IGRT) and four-dimensional (4D) computed tomography (CT) has significantly improved the accuracy of radiotherapy. In recent years, many studies have been carried out examining dosiomics, radiomics, and clinical characteristics to identify the factors affecting the occurrence of RP, with the aim of developing a more effective predictive model (7-9). It has been consistently demonstrated that age, history of radiotherapy, and radiotherapy parameters are especially significant factors associated with the development of RP (10,11). Moreover, the occurrence of grade 2 RP has been correlated with lung volume exceeding 30 Gy (V30) >20% (12). Furthermore, many types of drugs, including azithromycin, thymalfasin, and Chinese medicine, have been used in the treatment of RP in order to achieve a better therapeutic effect (13-15).
Study objective
Shanghai Pulmonary Hospital conducted a retrospective study in which we collected the clinical information of patients with lung cancer who underwent thoracic radiotherapy from August 2020 to August 2022, with or without other therapies. Multi-aspects of clinical data were collected to analyze to occurrence and influencing factors of grade 3 or high RP. The study objective is to uncover the best clinical predict model for severe RP. We present this article in accordance with the TRIPOD reporting checklist (available at https://tlcr.amegroups.com/article/view/10.21037/tlcr-24-328/rc).
Methods
We retrospectively reviewed the medical charts of lung cancer patients with radiotherapy (total dose >50 Gy and single dose 2 Gy) between August 2020 and August 2022. Screening for the presence of RP was performed by the CTCAE 5.0 and the Chinese consensus of RP (16). The grade of RP was evaluated by professional radiologists and respiratory physicians. We reviewed the radiotherapy treatment plans of patients with irradiation and the treatment methods of RP patients. We defined the steroids of RP mentioned in the Chinese consensus of RP as the standardized steroids of RP. Patients with metastatic lung cancer, incomplete radiotherapy, acute lung bacterial infection, drugs-related pneumonitis, active phase of pulmonary tuberculosis and therapy with long-term steroids or anti-inflammatory drugs were excluded. The study was conducted in accordance with the Declaration of Helsinki (as revised in 2013). The study was approved by the ethics board of Shanghai Pulmonary Hospital (No. L22-357), which was built by the clinical trial “The value of azithromycin combined Jinshuibao tablets in the treatment of radiation pneumonitis in patients with lung cancer: a prospective, randomized controlled exploratory clinical study” (ChiCTR2300073183), and informed consent was taken from all the patients.
Radiotherapy courses were delivered using three-dimensional (3D) conformal, intensity-modulated radiotherapy (IMRT), or volumetric arc therapy (VMAT) techniques. Each patient was immobilized in the supine position with a vacuum cushion. CT scans at intervals of 2.5–5.0 mm were performed for radiation planning, and a 4D-CT scan of the whole lung was performed to measure respiratory movement. Based on fluorodeoxyglucose positron emission tomography (FDG-PET) and the diagnostic CT images, gross tumor volume (GTV) was carefully contoured on the planning CT images. The National Comprehensive Cancer Network (NCCN) guidelines suggest the clinical target volume (CTV) was expanded from the internal GTV, and no prophylactic lymph node area was added. A planning target volume (PTV) margin of 5 mm was added for setup and interfractional uncertainty. Our study’s limited PTV margin was expanded by <10 mm from GTV without CTV for part of patients with advanced age, poor lung function, subclinical interstitial lung disease (ILD), and a history of pulmonary toxic drugs such as taxane and gemcitabine. IGRT was applied to real-time monitoring to abate set-up errors. The treatment dose was prescribed to cover 95% of the PTV. The mean lung dose (MLD), the percentage of total lung volume exceeding 20 Gy (V20) and 5 Gy (V5), and the dose constraints for normal tissue (spinal cord, esophagus, and heart) were limited appropriately according to the NCCN guidelines (17).
The standardized steroids application referred to the Chinese expert consensus on the diagnosis and treatment of RP (16). The standardized steroid therapy was administered for those with grade 2 RP with obvious symptoms and included oral prednisone at a dose of 0.5–1 mg/kg/d. If the illness improved or stabilized after 2–4 weeks, patients were permitted to steadily reduce the dose by 5–10 mg every week or every 2 weeks for 4–12 weeks. Grade 3 or higher RP was treated with intravenous injection of dexamethasone or methylprednisolone (the equivalent dose of methylprednisolone was 1–4 mg/kg/d). The dose could be decreased after the symptoms improved or stabilized (usually in 1–2 weeks). The recommendation is to subtract one-third to one-quarter of the original dose every 3 days until the minimum dose is reached. With the illness stable or improved to grade 2 or lower, switching from intravenous to oral for glucocorticoids was implemented, and the oral dose was gradually reduced.
The details regarding the diagnosis for RP refer to the Chinese consensus of RP (16). The incidence and grading of RP were assessed, and standardized use of glucocorticoid and symptomatic treatments was administered to patients according to their respective grades.
Model construction
The clinical model screening was completed using three methods: multivariate logistics regression (MLR), backward stepwise regression (BSR), and random forest regression (RFR). The MLR model was used to investigate the impact of several independent variables (X1, X2, …, Xk) on a single dependent variable (Y). BSR is an extension of linear regression models based on the Pearson correlation coefficient. The multiple regression model was as follows (18):
where Y is the dependent variable; X1, X2, …, Xk are independent variables; β1, β2, …, βk are parameters; and ε is the random component (the rest of the model).
The essential value of MLR model is based on the scientific information contained in the known equation. The applied stepwise regression was built to screen a minimum set of independent variables when the adjusted determination coefficient was maximum and the mean squared deviation was minimum in model. The detailed steps included building a model that contained all potential dependent variables, removing some variables one by one, screening the final variables to construct model with the highest determination coefficient (19). The RFR algorithm is an integrated supervised learning model that is implemented by random sampling of many independent decision trees and subsequently reports the total response of all decision trees. In our study, the model was built using the “RandomForest” package in R version 3.4.2 (The R Foundation for Statistical Computing, Vienna, Austria). Some of the key parameters controlling the performance of the model are the number of trees, the number of input variables, and the node size. The RFR model was further improved by optimizing these parameters (20).
Model validation
We estimated the over-optimism power in model development by dint of four validation methods, including the hold-out, 10-fold, leave-one-out, and bootstrap methods. Hold-out validation means the training set and validation set are filtrated after simple randomization of the total data to analyze the accuracy of the model. We used the 10-fold version of K-fold cross-validation for our cohorts. K-fold cross validation randomly partitions the dataset into K folders. Each fold has virtually the same number of class distributions. One of the folds was used for validation, while the remaining (K − 1) folds were used for training. This process was repeated 10 times until each fold was used only once as a validation set. Finally, the average results of 10 experiments were calculated (21). In leave-one-out cross-validation, one sample is left out of the test set each time, and the remaining samples are used as the training set. In bootstrap validation, a set of data is randomly resampled (with substitution; For example, when an item is sampled, it is immediately replaced) multiple times (up to 10,000 times or more), and statistical conclusions are drawn from this data collection (22). In our study, for each of the 1,000 predefined bootstraps, we fitted the logistic regression model coefficients on each bootstrap, and then computed its accuracy and κ value using the original non-bootstrapped development cohort. Accuracy and κ value were evaluated in all methods. As external validation, we evaluated the aforementioned models using the extra data of 70 patients. The processing of these data followed exactly the same procedure as that for the model development cohort, and none were used in any way during model construction.
The well-established calibration curve technique was used to assess the model’s goodness of fit. To facilitate clinical use and support fully independent validation of our model, a simple nomogram was generated for the three models. Finally, we evaluated the potential clinical utility of our model using decision curve analysis (DCA) (23).
Statistical analyses
The clinical characteristics of the continuous variables were expressed as mean ± standard deviation. Univariate ranking of clinical predictors was performed by Pearson chi-square test and Fisher exact test for categorical variables and logistic regression for continuous variables. Bilateral hypothesis test was used, and confidence level P=0.05 for the significance of clinical factors. The significant characteristics were then input into multivariate logistic regression. Feature selection, model construction, model performance evaluation, and DCA are all done in R 4.2.1. The Hosmer-Lemeshow test was used to assess adequate model calibration in both the training and validation data sets.
Results
Between August 2020 to August 2022, a total of 968 patients with lung cancer received thoracic radiotherapy. We collected basic clinical information and tracked the occurrence of RP over a 12-month follow-up period. Among those initially screened, 35 patients were excluded due to a lack of related information on RP, 115 patients were excluded and they did not reach the prescribed radiation dose planned by the doctor because of adverse events such as bone marrow suppression, coronavirus disease 2019 (COVID-19) epidemic situation, refusal to continue treatment, deterioration of disease. And 128 were excluded due to missing basic clinical or follow-up data. Finally, 690 patients were included in the study. The enrollment situation is showed in Figure 1. RP of different grades occurred in 453 patients (65.7%). The incidence of grade 2 RP was 28.7%, and the incidence of severe RP was 9.4%. The median time to the onset of RP was 113.0 days. Patients with grade 2 or above symptoms RP were treated with steroids in which there were 109 patients treated in our hospital and 87 patients treated in other hospitals. The results showed that whether steroid is standardized or not could affect the occurrence of severe RP. The probability of severe RP in the standardized steroids group was 14.8% (9/61), and the probability of severe RP was 34.8% (46/132) in the non-standardized steroids group (P=0.004). The results suggest that standardized steroids may contribute to reducing the incidence of severe RP. There were five patients who developed pneumocystis carinii pneumonia (PCP) and 6 patients who developed other fungal infections in all patients received long-term and high-dose steroids. The clinical characteristics of enrolled patients are listed in Table 1.
Table 1
Variables | Enrolled patients (n=690) |
---|---|
Age (years), median (IQR) | 64.0 (32.0–84.0) |
Age (years), n (%) | |
<70 | 529 (76.7) |
≥70 | 161 (23.3) |
Gender, n (%) | |
Female | 131 (19.0) |
Male | 559 (81.0) |
Smoker, n (%) | |
Yes | 390 (56.5) |
No | 300 (43.5) |
ECOG PS, n (%) | |
0 | 116 (16.8) |
1 | 525 (76.1) |
2 | 49 (7.1) |
Lung disease, n (%) | |
None | 451 (65.4) |
Subclinical ILD | 28 (4.1) |
COPD | 38 (5.5) |
Emphysema | 173 (25.1) |
Pathology, n (%) | |
NSCLC | 543 (78.7) |
SCLC | 145 (21.0) |
Mixed | 2 (0.3) |
Stage, n (%) | |
Stage I–III NSCLC | 430 (62.3) |
Stage IV NSCLC | 132 (19.1) |
LS-SCLC | 81 (11.7) |
ES-SCLC | 47 (6.8) |
FEV1 (%), mean ± SD | 81.6±0.90 |
DLCO (%), mean ± SD | 89.6±1.17 |
Lymphocyte counts before radiotherapy (×109/L), n (%) | |
<1.60 | 362 (52.5) |
≥1.60 | 328 (47.5) |
Therapeutic scheme, n (%) | |
CCRT | 87 (12.6) |
SCRT | 518 (75.1) |
Radiotherapy alone | 61 (8.8) |
Radiotherapy and others | 24 (3.5) |
Surgery, n (%) | |
Yes | 155 (22.5) |
No | 535 (77.5) |
Immunotherapy, n (%) | |
Yes | 162 (23.5) |
No | 528 (76.5) |
Targeted therapy, n (%) | |
Yes | 20 (2.9) |
No | 670 (97.1) |
Chemotherapy regimen, n (%) | |
Gemcitabine | 76 (11.0) |
Paclitaxel | 231 (33.5) |
Others | 317 (45.9) |
None | 66 (9.6) |
Steroids treatment, n (%) | |
Standardized | 61 (8.8) |
Non-standardized | 132 (19.1) |
No steroids | 497 (72.0) |
Limited PTV margin, n (%) | |
Yes | 255 (37.0) |
No | 354 (51.3) |
UK | 81 (11.7) |
Radiotherapy dose, n (%) | |
<54 Gy | 191 (27.7) |
≥54 and <60 Gy | 283 (41.0) |
≥60 Gy | 216 (31.3) |
IQR, interquartile range; ECOG, Eastern Cooperative Oncology Group; PS, performance status; ILD, interstitial lung disease; COPD, chronic obstructive pulmonary disease; NSCLC, non-small cell lung cancer; LS-SCLC, limited stage small cell lung cancer; ES-SCLC, extensive stage small cell lung cancer; FEV1, forced expiratory volume in 1 second; SD, standard deviation; DLCO, diffusing capacity of the lung for carbon monoxide; CCRT, concurrent chemoradiation therapy; SCRT, sequential chemoradiation therapy; PTV, planning target volume; UK, unknown.
Univariate and multivariate analyses of severe RP in enrolled patients are shown in Table 2. Univariate analysis showed that subclinical ILD, diffusing capacity of the lung for carbon monoxide (DLCO), surgery, lymphocyte count before radiotherapy, lymphocyte count after radiotherapy, whole lung volume, ipsilateral lung V30, and with or not standardized steroids were statistically significant (P<0.05) with the correlation of grade 3 or higher RP. The results of multivariate analysis showed that subclinical ILD, DLCO, and with or not standardized steroids were associated with the occurrence of severe RP. Therein, patients with subclinical ILD were more likely to develop grade 3 and higher RP. Patients with DLCO >87% were treated by clinicians with standardized steroids, which had a lower risk of grade 3 and a higher RP.
Table 2
Variables | Univariable analysis | Multivariable analysis | |||||
---|---|---|---|---|---|---|---|
OR | 95% CI | P value | OR | 95% CI | P value | ||
Age >70 years | 1.525 | 0.856–2.635 | 0.14 | ||||
Age | 1.031 | 0.999–1.066 | 0.06 | ||||
Gender | 0.839 | 0.461–1.621 | 0.58 | ||||
Smoker | 0.667 | 0.398–1.113 | 0.12 | ||||
ECOG PS | |||||||
0 | 0.571 | 0.232–1.206 | 0.18 | ||||
1 | 1.053 | 0.588–1.990 | 0.87 | ||||
2 | 1.995 | 0.834–4.259 | 0.09 | ||||
Lung disease | |||||||
Subclinical ILD | 3.471 | 1.321–8.156 | 0.007 | 25.938 | 1.754–700.714 | 0.02 | |
COPD | 0.816 | 0.193–2.351 | 0.74 | ||||
Emphysema | 1.239 | 0.697–2.132 | 0.45 | ||||
Surgery | 0.456 | 0.197–0.926 | 0.044 | 0.251 | 0.030–1.357 | 0.14 | |
Pathology | |||||||
NSCLC | 1.092 | 0.594–2.146 | 0.79 | ||||
SCLC | 0.934 | 0.475–1.716 | 0.83 | ||||
Stage | |||||||
Stage I–III NSCLC | 0.898 | 0.535–1.528 | 0.69 | ||||
Stage IV NSCLC | 1.179 | 0.610–2.147 | 0.60 | ||||
LS-SCLC | 1.234 | 0.551–2.487 | 0.58 | ||||
ES-SCLC | 0.639 | 0.152–1.818 | 0.46 | ||||
Treatment | |||||||
CCRT | 0.428 | 0.128–1.074 | 0.11 | ||||
SCRT | 1.365 | 0.745–2.675 | 0.34 | ||||
Radiotherapy alone | 1.515 | 0.640–3.181 | 0.30 | ||||
Radiotherapy and others | 0.409 | 0.023–1.991 | 0.39 | ||||
Chemotherapy | 0.624 | 0.306–1.410 | 0.22 | ||||
Chemotherapy regimen | |||||||
Gemcitabine | 1.078 | 0.460–2.231 | 0.85 | ||||
Paclitaxel | 1.098 | 0.634–1.858 | 0.73 | ||||
Immunotherapy | 1.071 | 0.575–1.901 | 0.82 | ||||
Targeted therapy | 0.498 | 0.028–2.463 | 0.50 | ||||
Lymphocyte before >1.60×109/L | 0.575 | 0.333–0.969 | 0.041 | 0.385 | 0.107–1.259 | 0.13 | |
Lymphocyte after >0.84×109/L | 0.523 | 0.298–0.892 | 0.02 | 0.957 | 0.278–3.336 | 0.94 | |
Standardized steroids | 0.026 | 0.011–0.051 | <0.001 | 0.004 | 0.000–0.024 | <0.001 | |
Radiotherapy dose >60 Gy | 0.758 | 0.414–1.326 | 0.35 | ||||
FEV1 >81.5% | 0.587 | 0.323–1.046 | 0.07 | ||||
DLCO >87% | 0.361 | 0.170–0.729 | 0.006 | 0.220 | 0.064–0.680 | 0.01 | |
Limited PTV margin | 0.690 | 0.380–1.215 | 0.21 | ||||
Whole lung volume >2,500 cm3 | 0.417 | 0.242–0.737 | 0.002 | 0.573 | 0.159–2.002 | 0.38 | |
Total lung V5 >35% | 1.500 | 0.871–2.676 | 0.16 | ||||
Total lung V20 >23% | 1.539 | 1.910–2.578 | 0.10 | ||||
Total lung V30 >16% | 1.117 | 0.659–1.869 | 0.68 | ||||
Total lung MLD >13 Gy | 1.467 | 0.740–2.727 | 0.25 | ||||
Contralateral lung V5 >26% | 1.263 | 0.704–2.190 | 0.42 | ||||
Contralateral lung V20 >4% | 1.078 | 0.645–1.780 | 0.77 | ||||
Contralateral lung V30 >2% | 1.423 | 0.677–1.910 | 0.61 | ||||
Contralateral lung MLD >2 Gy | 1.293 | 0.631–3.011 | 0.51 | ||||
Ipsilateral lung V5 >62% | 1.393 | 0.827–2.328 | 0.21 | ||||
Ipsilateral lung V20 >40% | 1.616 | 0.969–2.713 | 0.07 | ||||
Ipsilateral lung V30 >29% | 1.700 | 1.015–2.889 | 0.046 | 2.105 | 0.640–7.216 | 0.22 | |
Ipsilateral lung MLD >18 Gy | 1.643 | 0.974–2.837 | 0.07 |
RP, radiation pneumonitis; OR, odds ratio; CI, confidence interval; ECOG, Eastern Cooperative Oncology Group; PS, performance status; ILD, interstitial lung disease; COPD, chronic obstructive pulmonary disease; NSCLC, non-small cell lung cancer; SCLC, small cell lung cancer; LS-SCLC, limited stage small cell lung cancer; ES-SCLC, extensive stage small cell lung cancer; CCRT, concurrent chemoradiation therapy; SCRT, sequential chemoradiation therapy; FEV1, forced expiratory volume in 1 second; DLCO, diffusing capacity of the lung for carbon monoxide; PTV, planning target volume; V5, lung volume exceeding 5 Gy; V20, lung volume exceeding 20 Gy; V30, lung volume exceeding 30 Gy; MLD, mean lung dose.
To further explore the clinical prognostic factor of severe RP, we built a clinical model by dividing all the enrolled patients into a training set (621 patients) and a test set (69 patients). We constructed models using three screening methods and evaluated the models via external and internal validation in the training set. The test was also used to evaluate models via external validation. The overall model-building process is shown in Figure S1. The proportion of patients with severe RP in the training cohort and the testing cohort was 9.2% and 11.6%, respectively. The results of the univariate and multivariate analyses of the training set are listed in Table S1. Based on logistics regression results, three screening methods, including MLR, BSR, and RFR, were adopted to construct the model. The three models were as follows:
Model 1: subclinical ILD + DLCO >87% + standardized steroids;
Model 2: subclinical ILD + DLCO >87% + ipsilateral lung V20 >40% + standardized steroids;
Model 3: age + Eastern Cooperative Oncology Group (ECOG) performance status (PS) score of 2 + DLCO >87% + standardized steroids.
Model 1 from MLR was significantly associated with the occurrence of severe RP. Model 2 from BSR was screened according to the Akaike information criterion (AIC), which is a measure of the goodness of fit of a statistical model, with the lowest AIC value considered to have the best fit. In our study, the AIC value of model 2 was 80.23. Model 3 from RFR was screened according to the importance of characteristics using out-of-bag (OOB) error, an unbiased estimate of random forest generalization error, and an OOB rate of 10.33%. The detailed results of the models are shown in Figure 2, with the forest and bubble maps reflecting the results of the models, respectively.
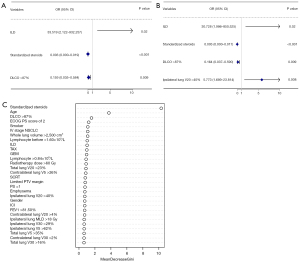
In order to determine which of the three models could best reflect the situation affecting the occurrence of grade 3 and higher RP, we conducted internal and external validation of the three models. The hold-out, 10-fold, leave-one-out, and bootstrap methods were used to verify the reproducibility of the model and to prevent overfitting. The accuracy and κ value were used to evaluate the validity and consistency of the models, respectively. The results are shown in Table 3. Three models all showed good accuracy and consistency than actual situation.
Table 3
Model | Cross-validation | Bootstrap | |||||||||
---|---|---|---|---|---|---|---|---|---|---|---|
Hold-out | 10-fold | Leave-one-out | |||||||||
Accuracy | κ | Accuracy | κ | Accuracy | κ | Accuracy | κ | ||||
Model 1 | 0.917 | 0.525 | 0.926 | 0.569 | 0.926 | 0.568 | 0.926 | 0.542 | |||
Model 2 | 0.902 | 0.479 | 0.905 | 0.457 | 0.872 | 0.496 | 0.907 | 0.470 | |||
Model 3 | 0.917 | 0.525 | 0.938 | 0.651 | 0.938 | 0.659 | 0.938 | 0.641 |
The receiver operating characteristic (ROC) curves of the three models are shown in Figure 3A, with the area under the ROC curve (AUC) of model 2 being the highest at 0.958 [95% confidence interval (CI): 0.932–0.985], indicating good predictive ability. The ROC curves of the models in the testing cohort are shown in Figure S2. A nomogram based on model 2 was built and is exhibited in Figure 3B, and the calibration curve of model 2 is shown in Figure 3C, which the predicted probability of RP was in good agreement with the actual observed probability. The nomograms and calibration curves of models 1 and 3 are displayed in Figure S3. The DCA (Figure 3D) showed that the best positive net benefit was provided at the threshold probability of Model 2, suggesting that a subset of patients could benefit clinically if the model 2 was used to aid clinical decision making.
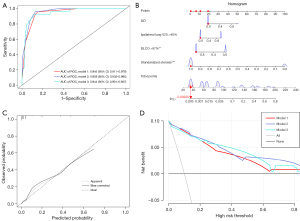
Discussion
Principal findings
Reducing the occurrence of severe RP is a critical goal for clinicians due to its high mortality. Several clinical studies have reported an incidence of severe RP of around 10–20% (24,25). To address this issue, our study collected clinical data from 690 patients with lung cancer over 2 years and analyzed the occurrence of RP. The results indicated that the incidence of severe RP was up to 9.4%. The standardized steroids for RP patients contributed to decreasing the risk of developing high-grade RP (14.8% vs. 34.8% in patients with steroids). The multivariate analysis of all patients indicated that subclinical ILD, DLCO, and with or not standardized steroids had correlation with the occurrence of severe RP. Model 2 by BSR was the best clinical prediction model for severe RP. Subclinical ILD, ipsilateral lung V20 >40% could increase the risk factors of severe RP, and standardized steroids and DLCO >87% could decrease the risk factors of severe RP. These findings indicated that individualized and precise radiotherapy regimens can significantly reduce adverse events to radiotherapy and that standardized steroids can improve the prognosis of RP and the quality of life of patients.
Strengths, limitations, and comparison with similar studies
In this study, individual lung dose limitation was applied for a specific population of patients with an age of 70 years or older, a history of taxane and gemcitabine use, poor basic lung function, or underlying lung diseases [idiopathic pulmonary fibrosis (IPF), usual interstitial pneumonia (UIP)] considering that they are at higher risk for developing RP, which were consistent with previous studies (26-28). According to the NCCN guideline (17), total lung V20 <35% was recommended and total lung V20 of the study was not exceed 30% that the highest value was 28.69%. Furthermore, the ipsilateral lung V20 >40% and ipsilateral lung MLD >18 Gy were considered as hazardous factors for severe RP in univariate analysis of training cohort and the ipsilateral lung V30 >29% also was a hazardous factor for severe RP in univariate analysis of all populations and training cohort. Similarly, several studies have suggested that V5, V30, and MLD can be used to predict the occurrence of RP (29-31). For instance, Tsujino et al. concluded in a retrospective study of severe RP in patients with concurrent chemoradiotherapy for non-small cell lung cancer that V5 and V20 are independent and significant risk factors for the occurrence of severe RP (32). In our study, however, the results of multivariate analysis for severe RP about V5 were not statistically significant. Here, we suggested that populations with an age ≥70 years, a history of taxane and gemcitabine chemotherapy, poor lung function, or subclinical ILD should be strictly limited according to the above dose range (ipsilateral lung V20, V30, MLD).
It is understanding that better DLCO was considered to decrease the risk factors of grade 3 or higher RP. According to a previous study, a diffusion deficit is considered to be a DLCO of less than 80% of the predicted value, meaning that these patients may have chronic inflammation of the lung with impairment of the gas-blood exchange (33). This reminded us that patients who received radiotherapy need to have better lung diffusion functions. An early decrease of DLCO has also been shown to be a predictive factor for severe RP development and thus could serve as a marker for early diagnosis and treatment modification (34). In our study, we found that subclinical ILD affected severe RP. Similarly, subclinical ILD participated in the occurrence of symptomatic and severe RP, was reported by others researchers (35,36). Thus, clinicians must be cautious about the risk of severe RP in patients with subclinical ILD and take specific measures in the early period of RP. The good PS maybe contributed to decrease the severe RP was showed by the RandomForest analysis which ECOG PS score of 2 could predict the risk of severe RP. This result was consistent with results of Liu et al.’ study for severe RP in which an ECOG PS score of 2–3, a forced expiratory volume in 1 second (FEV1) ≤65%, a previous PTV spanning the bilateral mediastinum, and V20 ≥30% on composite [previous radiation therapy (RT) + stereotactic ablative radiotherapy (SABR)] plans could predict the severe RP (37). Age also was regarded as a significant indicator in RandomForest mode. Though the final outcome didn’t involve the two indicators in the best model, other study pointed out that age and ECOG PS score of 2 could be associated with the grade ≥2 RP (38).
At present, there is no specific therapy for RP other than symptomatic treatment and steroid treatment. Based on the clinical treatment experience of our institution and previous clinical trials, our study selected the treatment scheme mentioned in the expert consensus of China on radiation-related pneumonitis (16). We found a reduction in the incidence of severe RP after administration of standardized steroid therapy from 34.8% to 14.8%, indicating the effectiveness of this treatment scheme. Here, we suggested the standardized steroids regimen should be applied for different grade RP, especially with or without steroids, dosage of steroids, and duration of steroids. Steroid and antibiotic treatment can trigger a decline in the immune system, resulting in the occurrence of fungal infections such as PCP (39). In our study, five patients developed PCP after receiving a long-term and high initial dose of steroids. Therefore, clinicians should be alert to fungal infection when using high-dose steroid therapy in patients with RP.
We further explored the risk factors that influenced severe RP by using a variety of screening approaches to construct models and identified the prediction model of which was most associated with RP. Conventional studies on indicators are mainly based on logistic regression methods (40-42). Using the BSR method, we found that ipsilateral lung V20 >40% was critically associated with severe RP. We used the RFR to construct a model and determined the association of age and ECOG PS score of 2 with severe RP. These methods have been reported in other literatures but was not further compared to which one was the best to evaluate the risk of severe RP (43-45). Furthermore, in our study, we compared the accuracy and κ value to identify the factors associated with the occurrence of severe RP, which the accuracy value was up to 80% above and κ value was 0.4 above was considered to have moderate consistency with actual situation. We also compared the model screening methods and found that BSR could both better screen and construct the model according to the ROC. We evaluated the performance of the models in three aspects, discrimination ability, calibration, and clinical application potential. Through internal and external verification of the three models, we found the model 2 verified the stability and fit of the model. The final comprehensive model had excellent calibration, with no significant overestimation or underestimation for different risk intervals. Finally, a good clinical practice model was constructed with the nomogram, and the clinical decision curve was used to evaluate the clinical application ability of the model. The model construction from design to assessment systematically described the prediction process of severe RP, which were also applied for others diseases (46,47).
Our study still had some limitations, which should be mentioned. First, as we employed a retrospective design, bias might have been introduced. Second, when patients received drug treatment including targeted and immune therapy besides radiotherapy, RP could be mixed with drugs-related pneumonitis which was involved in our study. Third, although lung dose limitation and standardized glucocorticoid therapy for patients receiving radiotherapy contributed to a reduction in the incidence of severe RP, some patients still experienced severe RP, and the reason for this needs to be further determined. Therefore, we look forward to identifying more effective measures for preventing and treating RP. And then, this study only followed up patients for up to 1 year after radiotherapy to observe RP, and thus data on their long-term survival status remains to be collected. Finally, imageomics were not included in the construction of the model, and only specific lung limiting doses, including V5, V20, V30, and MLD, were examined, which implies certain limitations for the prediction of RP. Previous studies have built models to predict RP by classifying and scoring a dose and volume histogram and using pulmonary CT, but the consideration of clinical factors was not as detailed as in this study (7,48,49). These factors will be incorporated into subsequent research.
Conclusions
Our study demonstrated that construction of clinical model could predict the risk of grade 3 or higher RP. Patients with subclinical ILD and ipsilateral lung V20 >40% of radiotherapy could increase the risk of severe RP, and patients with a better lung diffusion function and received standardized steroids treatment could decrease the risk of severe RP.
Acknowledgments
Funding: The study was supported by
Footnote
Reporting Checklist: The authors have completed the TRIPOD reporting checklist. Available at https://tlcr.amegroups.com/article/view/10.21037/tlcr-24-328/rc
Data Sharing Statement: Available at https://tlcr.amegroups.com/article/view/10.21037/tlcr-24-328/dss
Peer Review File: Available at https://tlcr.amegroups.com/article/view/10.21037/tlcr-24-328/prf
Conflicts of Interest: All authors have completed the ICMJE uniform disclosure form (available at https://tlcr.amegroups.com/article/view/10.21037/tlcr-24-328/coif). The authors have no conflicts of interest to declare.
Ethical Statement: The authors are accountable for all aspects of the work in ensuring that questions related to the accuracy or integrity of any part of the work are appropriately investigated and resolved. The study was conducted in accordance with the Declaration of Helsinki (as revised in 2013). The study was approved by the ethics board of Shanghai Pulmonary Hospital (No. L22-357), and informed consent was taken from all the patients.
Open Access Statement: This is an Open Access article distributed in accordance with the Creative Commons Attribution-NonCommercial-NoDerivs 4.0 International License (CC BY-NC-ND 4.0), which permits the non-commercial replication and distribution of the article with the strict proviso that no changes or edits are made and the original work is properly cited (including links to both the formal publication through the relevant DOI and the license). See: https://creativecommons.org/licenses/by-nc-nd/4.0/.
References
- Or M, Liu B, Lam J, et al. A systematic review and meta-analysis of treatment-related toxicities of curative and palliative radiation therapy in non-small cell lung cancer. Sci Rep 2021;11:5939. [Crossref] [PubMed]
- Zhuang H, Yuan Z, Chang JY, et al. Radiation pneumonitis in patients with non--small-cell lung cancer treated with erlotinib concurrent with thoracic radiotherapy. J Thorac Oncol 2014;9:882-5. [Crossref] [PubMed]
- Common Terminology Criteria for Adverse Events (CTCAE). Version 5.
0. 2017. Available online: https://ctep.cancer.gov/protocoldevelopment/electronic_applications/docs/CTCAE_v5_Quick_Reference_5x7.pdf - Sekine I, Sumi M, Ito Y, et al. Retrospective analysis of steroid therapy for radiation-induced lung injury in lung cancer patients. Radiother Oncol 2006;80:93-7. [Crossref] [PubMed]
- Xu H, Cao J, Wang J, et al. Management and outcome of symptomatic radiation induced lung injury in non-small cell lung cancer. Chinese Journal of Radiation Oncology 2013;22:201-4.
- Yamada M, Kudoh S, Hirata K, et al. Risk factors of pneumonitis following chemoradiotherapy for lung cancer. Eur J Cancer 1998;34:71-5. [Crossref] [PubMed]
- Zhang Z, Wang Z, Yan M, et al. Radiomics and Dosiomics Signature From Whole Lung Predicts Radiation Pneumonitis: A Model Development Study With Prospective External Validation and Decision-curve Analysis. Int J Radiat Oncol Biol Phys 2023;115:746-58. [Crossref] [PubMed]
- Huang Y, Feng A, Lin Y, et al. Radiation pneumonitis prediction after stereotactic body radiation therapy based on 3D dose distribution: dosiomics and/or deep learning-based radiomics features. Radiat Oncol 2022;17:188. [Crossref] [PubMed]
- Kraus KM, Oreshko M, Bernhardt D, et al. Dosiomics and radiomics to predict pneumonitis after thoracic stereotactic body radiotherapy and immune checkpoint inhibition. Front Oncol 2023;13:1124592. [Crossref] [PubMed]
- Shi LL, Yang JH, Yao HF. Multiple regression analysis of risk factors related to radiation pneumonitis. World J Clin Cases 2023;11:1040-8. [Crossref] [PubMed]
- Kita N, Tomita N, Takaoka T, et al. Clinical and dosimetric factors for symptomatic radiation pneumonitis after stereotactic body radiotherapy for early-stage non-small cell lung cancer. Clin Transl Radiat Oncol 2023;41:100648. [Crossref] [PubMed]
- Doshita K, Tabuchi Y, Kenmotsu H, et al. Incidence and Treatment Outcome of Radiation Pneumonitis in Patients With Limited-stage Small Cell Lung Cancer Treated With Concurrent Accelerated Hyperfractionated Radiation Therapy and Chemotherapy. Adv Radiat Oncol 2023;8:101129. [Crossref] [PubMed]
- Yan Y, Wu L, Li X, et al. Immunomodulatory role of azithromycin: Potential applications to radiation-induced lung injury. Front Oncol 2023;13:966060. [Crossref] [PubMed]
- Liu F, Qiu B, Xi Y, et al. Efficacy of Thymosin α1 in Management of Radiation Pneumonitis in Patients With Locally Advanced Non-Small Cell Lung Cancer Treated With Concurrent Chemoradiotherapy: A Phase 2 Clinical Trial (GASTO-1043). Int J Radiat Oncol Biol Phys 2022;114:433-43. [Crossref] [PubMed]
- Gao RW, Day CN, Yu NY, et al. Dosimetric predictors of pneumonitis in locally advanced non-small cell lung cancer patients treated with chemoradiation followed by durvalumab. Lung Cancer 2022;170:58-64. [Crossref] [PubMed]
- Zhou C, Yu J. Chinese expert consensus on diagnosis and treatment of radiation pneumonitis. Precision Radiation Oncology 2022;6:262-71.
- Ettinger DS, Wood DE, Aisner DL, et al. NCCN Guidelines Version 2.2024 Non-Small Cell Lung Cancer. National Comprehensive Cancer Network. 2024. Available online: https://www.nccn.org/patientresources/patient-resources/guidelines-for-patients
- Bugdol MD, Bugdol MN, Lipowicz AM, et al. Prediction of menarcheal status of girls using voice features. Comput Biol Med 2018;100:296-304. [Crossref] [PubMed]
- Cilla S, Macchia G, Lenkowicz J, et al. CT angiography-based radiomics as a tool for carotid plaque characterization: a pilot study. Radiol Med 2022;127:743-53. [Crossref] [PubMed]
- Wang Y, Du Y, Wang J, et al. Calibration of a low-cost PM(2.5) monitor using a random forest model. Environ Int 2019;133:105161. [Crossref] [PubMed]
- Mohr F, van Rijn JN. Fast and Informative Model Selection Using Learning Curve Cross-Validation. IEEE Trans Pattern Anal Mach Intell 2023;45:9669-80. [Crossref] [PubMed]
- Wang T, Russo DP, Bitounis D, et al. Integrating structure annotation and machine learning approaches to develop graphene toxicity models. Carbon N Y 2023;204:484-94. [Crossref] [PubMed]
- Van Calster B, Wynants L, Verbeek JFM, et al. Reporting and Interpreting Decision Curve Analysis: A Guide for Investigators. Eur Urol 2018;74:796-804. [Crossref] [PubMed]
- Wen J, Liu H, Wang L, et al. Potentially Functional Variants of ATG16L2 Predict Radiation Pneumonitis and Outcomes in Patients with Non-Small Cell Lung Cancer after Definitive Radiotherapy. J Thorac Oncol 2018;13:660-75. [Crossref] [PubMed]
- Roach M 3rd, Gandara DR, Yuo HS, et al. Radiation pneumonitis following combined modality therapy for lung cancer: analysis of prognostic factors. J Clin Oncol 1995;13:2606-12. [Crossref] [PubMed]
- Palma DA, Senan S, Tsujino K, et al. Predicting radiation pneumonitis after chemoradiation therapy for lung cancer: an international individual patient data meta-analysis. Int J Radiat Oncol Biol Phys 2013;85:444-50. [Crossref] [PubMed]
- Liang J, Bi N, Wu S, et al. Etoposide and cisplatin versus paclitaxel and carboplatin with concurrent thoracic radiotherapy in unresectable stage III non-small cell lung cancer: a multicenter randomized phase III trial. Ann Oncol 2017;28:777-83. [Crossref] [PubMed]
- Liu Y, Zhu Y, Wu R, et al. Stereotactic body radiotherapy for early stage non-small cell lung cancer in patients with subclinical interstitial lung disease. Transl Lung Cancer Res 2020;9:2328-36. [Crossref] [PubMed]
- Meng Y, Luo W, Wang W, et al. Intermediate Dose-Volume Parameters, Not Low-Dose Bath, Is Superior to Predict Radiation Pneumonitis for Lung Cancer Treated With Intensity-Modulated Radiotherapy. Front Oncol 2020;10:584756. [Crossref] [PubMed]
- Madani I, De Ruyck K, Goeminne H, et al. Predicting risk of radiation-induced lung injury. J Thorac Oncol 2007;2:864-74. [Crossref] [PubMed]
- Wang W, Xu Y, Schipper M, et al. Effect of normal lung definition on lung dosimetry and lung toxicity prediction in radiation therapy treatment planning. Int J Radiat Oncol Biol Phys 2013;86:956-63. [Crossref] [PubMed]
- Tsujino K, Hashimoto T, Shimada T, et al. Combined analysis of V20, VS5, pulmonary fibrosis score on baseline computed tomography, and patient age improves prediction of severe radiation pneumonitis after concurrent chemoradiotherapy for locally advanced non-small-cell lung cancer. J Thorac Oncol 2014;9:983-90. [Crossref] [PubMed]
- Wu X, Liu X, Zhou Y, et al. 3-month, 6-month, 9-month, and 12-month respiratory outcomes in patients following COVID-19-related hospitalisation: a prospective study. Lancet Respir Med 2021;9:747-54. [Crossref] [PubMed]
- Aso S, Navarro-Martin A, Castillo R, et al. Severity of radiation pneumonitis, from clinical, dosimetric and biological features: a pilot study. Radiat Oncol 2020;15:246. [Crossref] [PubMed]
- Ueki N, Matsuo Y, Togashi Y, et al. Impact of pretreatment interstitial lung disease on radiation pneumonitis and survival after stereotactic body radiation therapy for lung cancer. J Thorac Oncol 2015;10:116-25. [Crossref] [PubMed]
- Okumura M, Hojo H, Nakamura M, et al. Radiation pneumonitis after palliative radiotherapy in cancer patients with interstitial lung disease. Radiother Oncol 2021;161:47-54. [Crossref] [PubMed]
- Liu H, Zhang X, Vinogradskiy YY, et al. Predicting radiation pneumonitis after stereotactic ablative radiation therapy in patients previously treated with conventional thoracic radiation therapy. Int J Radiat Oncol Biol Phys 2012;84:1017-23. [Crossref] [PubMed]
- Huang BT, Lin PX, Wang Y, et al. Developing a Prediction Model for Radiation Pneumonitis in Lung Cancer Patients Treated With Stereotactic Body Radiation Therapy Combined With Clinical, Dosimetric Factors, and Laboratory Biomarkers. Clin Lung Cancer 2023;24:e323-e331.e2.
- Fu Z, Yang X, Bi N, et al. Radiation pneumonitis complicated by Pneumocystis carinii in patients with thoracic neoplasia: a clinical analysis of 7 cases. Cancer Commun (Lond) 2019;39:47. [Crossref] [PubMed]
- Guo XJ, Cai XT, Rong ZX, et al. Interstitial pneumonitis associated with combined regimen of immunotherapy and conventional therapies-pharmacovigilance database analysis with real-world data validation. BMC Med 2023;21:6. [Crossref] [PubMed]
- Nardi EA, Sun CL, Robert F, et al. Lung Cancer in Nonelderly Patients: Facility and Patient Characteristics Associated With Not Receiving Treatment. J Natl Compr Canc Netw 2019;17:931-9. [Crossref] [PubMed]
- Lindberg K, Grozman V, Karlsson K, et al. The HILUS-Trial-a Prospective Nordic Multicenter Phase 2 Study of Ultracentral Lung Tumors Treated With Stereotactic Body Radiotherapy. J Thorac Oncol 2021;16:1200-10. [Crossref] [PubMed]
- Harder EM, Park HS, Chen ZJ, et al. Pulmonary dose-volume predictors of radiation pneumonitis following stereotactic body radiation therapy. Pract Radiat Oncol 2016;6:e353-9. [Crossref] [PubMed]
- Zhang Y, Li Z, Xiao H, et al. Development and validation of a random forest model for predicting radiation pneumonitis in lung cancer patients receiving moderately hypofractionated radiotherapy: a retrospective cohort study. Ann Transl Med 2022;10:1264. [Crossref] [PubMed]
- O'Reilly S, Jain V, Huang Q, et al. Dose to Highly Functional Ventilation Zones Improves Prediction of Radiation Pneumonitis for Proton and Photon Lung Cancer Radiation Therapy. Int J Radiat Oncol Biol Phys 2020;107:79-87. [Crossref] [PubMed]
- Liu P, Xing Z, Peng X, et al. Machine learning versus multivariate logistic regression for predicting severe COVID-19 in hospitalized children with Omicron variant infection. J Med Virol 2024;96:e29447. [Crossref] [PubMed]
- Voss RK, Chiang YJ, Cromwell KD, et al. Do No Harm, Except to Ourselves? A Survey of Symptoms and Injuries in Oncologic Surgeons and Pilot Study of an Intraoperative Ergonomic Intervention. J Am Coll Surg 2017;224:16-25.e1. [Crossref] [PubMed]
- Yu H, Wu H, Wang W, et al. Machine Learning to Build and Validate a Model for Radiation Pneumonitis Prediction in Patients with Non-Small Cell Lung Cancer. Clin Cancer Res 2019;25:4343-50. [Crossref] [PubMed]
- Stella M, van Rooij R, Lam MGEH, et al. Lung Dose Measured on Postradioembolization (90)Y PET/CT and Incidence of Radiation Pneumonitis. J Nucl Med 2022;63:1075-80. [Crossref] [PubMed]