Heterogeneity of lymphocyte subsets in predicting immune checkpoint inhibitor treatment response in advanced lung cancer: an analysis across different pathological types, therapeutic drugs, and age groups
Highlight box
Key findings
• This study identifies specific lymphocyte subsets that correlate with better responses to ICIs in various lung cancer subtypes. Lower CD8+ T cell and higher CD4+ T cell counts are associated with improved treatment efficacy, particularly in younger patients under 60 years.
What is known and what is new?
• Prior research has shown that immune profiles, including lymphocyte subsets, play a role in predicting responses to immunotherapy in cancer treatment.
• This study provides detailed insights into how different lymphocyte ratios affect treatment outcomes across lung cancer subtypes, offering a more nuanced understanding of immune-based stratification in immunotherapy.
What is the implication, and what should change now?
• The findings suggest that immune profiling, particularly lymphocyte subset analysis, can be an effective tool for tailoring immunotherapy in lung cancer, potentially leading to more personalized treatment approaches. Oncology treatment protocols should consider incorporating routine lymphocyte subset profiling for patients undergoing immunotherapy. This can help optimize treatment decisions and improve patient outcomes by identifying those most likely to benefit from specific immunotherapeutic agents.
Introduction
Immune checkpoint inhibitor (ICI) is an emerging approach in tumor treatment that utilizes antibodies to obstruct inhibitory signals between tumor cells (TC) and immune cells (IC), thereby enhancing the immune system’s ability to effectively target and eliminate cancer cells. The advent of ICIs has altered the therapeutic paradigm for solid tumors, showing marked efficacy across various cancer types. Pivotal data from the Checkmate-024 trial underscore that pembrolizumab significantly extends progression-free survival (PFS) and overall survival (OS) in comparison to conventional chemotherapy for advanced lung cancer patients with elevated anti-programmed cell death ligand 1 (PD-L1) expression (1). Moreover, patients who respond to immunotherapy typically achieve a longer median PFS compared to those treated with conventional cytotoxic agents or even molecular-targeted drugs (2).
Despite the considerable advancements achieved by ICIs in lung cancer treatment, the Keynote-042 study demonstrated that only 27.3% of PD-L1-positive patients derived benefit from pembrolizumab monotherapy. Even among those with PD-L1 expression ≥50%, the objective response rate (ORR) was merely 39.1% (3). In contrast, patients exhibiting low or negative PD-L1 expression may also potentially attain favorable therapeutic outcomes from immunotherapy interventions (4,5). Although tissue biopsy remains the gold standard for tumor molecular profiling, the invasive nature of sampling, the need for repeated biopsies, and the limited accessibility of tumor tissue in certain advanced cases make continuous assessment of ICI treatments challenging. Consequently, there is a significant need for reliable and dynamic monitoring methods to gauge the efficacy of ICIs. In this context, novel biomarkers for ICI treatment assessment are of utmost clinical importance.
Increasing evidence suggests a correlation between peripheral blood lymphocyte subsets and the immune status of cancer patients (6-8). Non-invasive peripheral blood lymphocyte subset analysis could serve as a valuable tool for assessing treatment efficacy and prognosis in cancer patients (9-13). However, few studies have analyzed the heterogeneity of the predictive ability of different subsets of lymphocytes for ICIs treatment in different subgroups, especially considering different pathological types, different immunotherapy drugs, and different age groups. In this study, we collected baseline peripheral blood lymphocyte subsets from advanced lung cancer patients before immunotherapy and analyzed their relationship with short-term treatment response and prognosis in different subsets. This will provide evidence for investigating lymphocyte subsets as biomarkers for predicting the efficacy of ICI therapy. We present this article in accordance with the REMARK reporting checklist (available at https://tlcr.amegroups.com/article/view/10.21037/tlcr-24-109/rc).
Methods
Study population
This investigation enrolled 146 patients diagnosed with advanced-stage lung cancer, all of whom received ICI therapy at Shandong Cancer Hospital and Institute from May 2020 to December 2022. The study was meticulously conducted in strict accordance with the ethical guidelines and principles of the Helsinki Declaration (as revised in 2013), and was approved by the Ethics Review Committee of Shandong Cancer Hospital and Institute (approval No. SDTHEC2023011009). To ensure privacy and confidentiality, patient data were anonymized rigorously. The study adhered to stringent confidentiality protocols during all data analyses. Informed consent was obtained from each participant in written form, following a comprehensive explanation of the study’s purpose and procedures.
Inclusion criteria
- Histopathological or cytological confirmation of lung cancer diagnosis;
- Age ≥18 years with an Eastern Cooperative Oncology Group (ECOG) performance status of 0–2;
- Clinical staging of III–IV according to the American Joint Committee on Cancer (AJCC) guidelines;
- Availability of comprehensive and analyzable lymphocyte subset data and follow-up records;
- Assessment of epidermal growth factor receptor (EGFR) gene mutation and PD-L1 expression levels at the time of admission;
- No history of autoimmune diseases or previous treatments that could influence immune modulation;
- Absence of acute pneumonia or other respiratory infections at admission, and no inflammation resulting from additional treatment modalities, such as radiotherapy.
Exclusion criteria
- Patients with clinical or pathological evidence of non-lung cancer diagnoses;
- Missing or incomplete clinical or pathological data during the follow-up phase;
- Presence of autoimmune diseases or a history of treatments affecting immune function, including prior immunostimulatory interventions.
Data collection
- Patient demographics: detailed collection of patient demographics, encompassing gender, age, tumor pathology, and Tumor Node Metastasis (TNM) staging as per tumor classification guidelines;
- Treatment regimens and medication details: comprehensive documentation of each patient’s treatment plan, including specific medications used. This covers aspects such as dosage, duration, route of administration, and any combination therapies employed;
- Peripheral blood sample collection: blood samples were collected via peripheral venipuncture from each participant within the initial 2 weeks preceding the commencement of ICI therapy. Blood was treated with erythrocyte lysis buffer to remove red blood cells, leaving a suspension of leukocytes. Staining protocol: the leukocytes were then stained with fluorochrome-conjugated antibodies. The antibodies were sourced from Tongshengshidai (Z6410010, Z6410002; Beijing, China), Beckman (6607073; Brea, CA, USA), and BD (665343; Franklin Lakes, NJ, USA). Staining was conducted according to the manufacturer’s protocols. Flow cytometry analysis: following staining, cells were analyzed using a flow cytometer (Beckman Coulter DxFLEX, Brea, CA, USA). These samples were analyzed to determine the proportions of various lymphocyte subsets, including CD3−CD56+ [natural killer (NK) cells], CD3−CD19+ (B cells), CD3+ (total T lymphocytes), CD3+CD4+ [helper T (Th) cells], and CD3+CD8+ [cytotoxic and suppressor T (Tc/Ts) cells], along with the CD4+/CD8+ ratio.
Clinical outcome assessment
The evaluation of initial disease response post-ICI or combined treatment administration was a key focus. For patients receiving combination chemotherapy, response assessment was conducted after the completion of two treatment cycles. Those undergoing concomitant radiotherapy were evaluated 1 month post-radiotherapy completion. In cases of immunotherapy monotherapy, assessments were performed within the initial four treatment cycles. We diligently tracked PFS for each patient, defined as the duration from the onset of treatment to either any tumor progression or death due to any cause.
Treatment efficacy was determined through a combination of imaging studies and tumor marker evaluations. Each imaging result underwent a thorough review by at least two senior radiologists. To standardize the evaluation of treatment responses, the Response Evaluation Criteria In Solid Tumors (RECIST) version 1.1 was employed. This included categorizing responses into complete response (CR), partial response (PR), stable disease (SD), and progressive disease (PD). Treatment outcomes classified as CR, PR, or SD were considered effective, while PD was indicative of ineffective treatment.
Statistical analysis
Treatment efficacy was assessed using the t-test across patient cohorts and subgroups. The median value divided observations into higher and lower groups. Median survival time was determined via the Kaplan-Meier method, with survival distributions compared using the log-rank test. Receiver operating characteristic (ROC) curves were used to test the sensitivity. A univariate Cox proportional hazards regression model was employed to evaluate individual variables’ influence on PFS, showing hazard ratio (HR) with 95% confidence interval (CI) without adjusting for selected factors. Analyses were bidirectional, with P values <0.05 indicating significance. Data analysis used R version 4.0.3 software (https://www.r-project.org/), unless specified. Survival curves and violin plots were created using Prism GraphPad Software 8.0 (San Diego, CA, USA).
Results
Patient characteristics
A total of 146 patients diagnosed with advanced lung cancer were enrolled in this study. The baseline clinical and pathological characteristics of the patients are summarized in Table 1. These details, including gender, age, histological type, receipt of combination therapy, and administration of PD-L1 or programmed death 1 (PD-1) inhibitors, were presented as counts and percentages. These baseline features were utilized as covariates in subsequent correlation analyses. Of the enrolled patients, 10 presented with atypical pathological results, divergent from common classifications such as squamous, adenocarcinoma, or small cell carcinoma. These included 3 cases of large cell neuroendocrine carcinoma, 2 of adenocarcinoma combined with sarcomatoid carcinoma, 1 adenoid cystic carcinoma, and 4 with unspecified pathology. Data from these ten patients were excluded from statistical analyses pertinent to histological subtypes. Importantly, the study period did not record any patient deaths, thereby concentrating on the short-term outcomes post-treatment.
Table 1
Patients’ characteristics | N | % |
---|---|---|
Gender | ||
Male | 122 | 83.56 |
Female | 24 | 16.44 |
Age, years | ||
≤60 | 64 | 43.84 |
>60 | 82 | 56.16 |
Histotype | ||
Squamous cell lung cancer | 34 | 23.29 |
Adenocarcinoma | 57 | 39.04 |
Small cell | 45 | 30.82 |
Others | 10 | 6.85 |
ICI | ||
PD-L1 inhibitor | 56 | 38.36 |
PD-1 inhibitor | 90 | 61.64 |
Treatment | ||
Single ICIs | 33 | 22.6 |
With chemotherapy | 68 | 46.58 |
With radiotherapy | 4 | 2.74 |
With targeted therapy | 4 | 2.74 |
With chemotherapy and targeted therapy | 11 | 7.53 |
With radiochemotherapy | 26 | 17.81 |
Clinical stages | ||
III | 41 | 28.08 |
IV | 105 | 71.92 |
ICI, immune checkpoint inhibitor; PD-L1, programmed cell death ligand 1; PD-1, programmed death 1.
Correlation between lymphocyte subsets and the efficacy of ICIs
The findings demonstrated that a higher levels of CD3+ CD8+ cells within the lymphocyte population was associated with diminished immunotherapy efficacy. Conversely, a lower levels of CD3+CD8+ T cells correlated with improved treatment response, exhibiting a significant negative correlation with short-term efficacy (P=0.004, Figure 1A). Additionally, a higher CD4+/CD8+ ratio was found to be significantly associated with enhanced immunotherapy efficacy (P=0.02, Figure 1B). However, no significant associations were identified between other lymphocyte subsets, including NK cells, B cells, total T lymphocytes, Th cells, and immunotherapy efficacy in the overall analysis. Additionally, the ROC analysis demonstrated that the baseline levels of CD3+CD8+ cells and the CD4+/CD8+ ratio were predictive of immunotherapy efficacy, with area under the curve (AUC) values of 0.64 (P=0.004, Figure 1C) and 0.63 (P=0.006, Figure 1D), respectively.
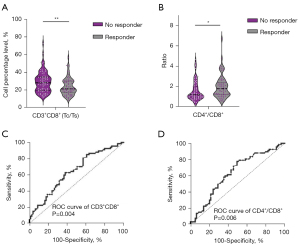
Differential analysis of patients receiving anti-PD-L1/anti-PD-1 drugs and lymphocyte subsets
This study further conducted a detailed analysis of lymphocyte subset distribution in patients receiving treatment with anti-PD-L1 or anti-PD-1 drugs. In our sample, 56 patients received PD-L1 drug therapy (atezolizumab: 18 patients, durvalumab: 33 patients, avelumab: 5 patients), accounting for 38.36% of the total sample. Additionally, 90 patients received PD-1 drug therapy (pembrolizumab: 21 patients, nivolumab: 3 patients, cemiplimab: 4 patients, toripalimab: 24 patients, sintilimab: 38 patients), accounting for 61.64% of the total sample. Statistical analysis revealed no significant correlation between the efficacy of PD-1 drugs and the cellular distribution of lymphocyte subsets. No statistically significant associations were observed between any indicators and the immunotherapy efficacy. However, in patients receiving anti-PD-L1 drug therapy, our analysis revealed a correlation between lower CD3+CD8+ T cell levels (P=0.002, Figure 2A) and a higher CD4+/CD8+ ratio (P=0.04, Figure 2B) with better treatment efficacy. The ROC curves demonstrate that in patients receiving anti-PD-L1 drug treatment, the AUC areas for baseline CD3+CD8+ cells and CD4+/CD8+ ratio are 0.73 (P=0.003, Figure 2C) and 0.67 (P=0.03, Figure 2D), respectively. No significant trends were observed between the proportions of other lymphocyte subsets and treatment efficacy. Furthermore, we conducted a separate analysis focusing on the used PD-L1 inhibitor, durvalumab (33 patients), and found a more significant correlation between lower CD3+CD8+ T cell levels and better treatment efficacy (P<0.0001, Figure S1).
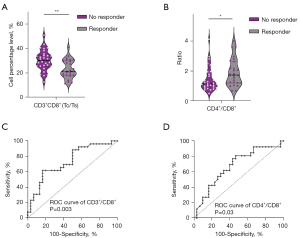
Correlation between pathological types and lymphocyte subsets
Comprehensive subgroup analyses were performed to explore the relationship between lymphocyte subsets and treatment efficacy across three histological types of lung cancer: squamous cell carcinoma, adenocarcinoma, and small cell carcinoma. In squamous cell carcinoma patients, a lower level of CD3+CD8+ T lymphocytes (P=0.01, Figure S2A) and a higher CD4+/CD8+ T cell ratio were associated with treatment efficacy (P=0.02, Figure S2A). In addition, in small cell carcinoma patients, the difference in treatment efficacy was more pronounced with lower levels of CD3+CD8+ T lymphocytes (P=0.009, Figure S2B). However, for adenocarcinoma patients, we did not observe a significant association between lymphocyte subset proportions and treatment efficacy (Figure S2C). An integration of the three histological types and CD3+CD8+ T lymphocyte levels was performed (Figure 3A). ROC working curves show that the baseline levels of CD3+CD8+ cells predict the efficacy of immunotherapy in small cell carcinoma, squamous cell carcinoma, and adenocarcinoma with AUC values of 0.75 (P=0.008, Figure 3B), 0.70 (P=0.050, Figure 3C), and 0.51 (P=0.90, Figure 3D), respectively.
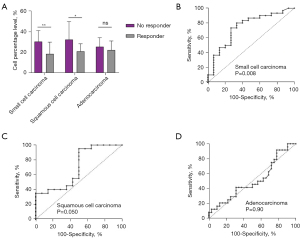
Correlation between age and prediction of lymphocyte subset efficacy
Given that the human immune status is influenced by a multitude of factors, including age and gender, this study sought to elucidate the relationship between the proportions of lymphocyte subsets and immunotherapy efficacy across different age cohorts. The analysis was conducted on both older (≥60 years) and younger (<60 years) patient groups within the cohort of 146 individuals.
We found a significant statistical association between higher levels of CD3+CD4+ T lymphocytes (P=0.03) and lower levels of CD3+CD8+ T lymphocytes (P=0.006) and treatment efficacy in patients younger than 60 years. The results indicated that patients with a relatively higher CD4+/CD8+ ratio demonstrated better treatment efficacy (P=0.006) (Figure 4A). Creating ROC curves, the predictive values for treatment efficacy of CD3+CD4+ T lymphocyte levels, CD3+CD8+ T lymphocyte levels, and CD4+/CD8+ ratio in the group aged less than 60 years were 0.66 (P=0.02, Figure 4B), 0.69 (P=0.008, Figure 4C), and 0.71 (P=0.003, Figure 4D), respectively. Conversely, in the cohort of patients aged 60 years or older, the analysis did not reveal any significant correlations between lymphocyte subset distributions and treatment efficacy.
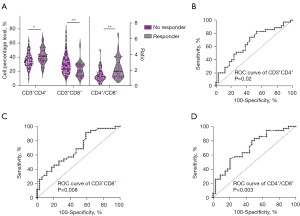
Prediction of PFS of ICIs treatment by lymphocyte subsets
This study evaluated the prognostic significance of various lymphocyte subsets in lung cancer patients receiving ICI therapy, as determined by peripheral blood lymphocyte analyses. In the context of survival analysis, a HR of less than 1 is indicative of a protective factor, suggesting that patients with higher frequency values of specific lymphocyte subsets may experience extended PFS. Our findings revealed a significant correlation between the frequency values of peripheral blood CD3+CD4+ T cells and CD3+CD8+ T cells and the PFS of patients undergoing immunotherapy. However, for other lymphocyte subsets, no statistical differences in PFS were observed (as illustrated in the forest plot, Figure 5A). The Kaplan-Meier survival curve analysis underscored the relationship between the frequency values of CD3+CD4+ T cells and PFS. According to the log-rank test, the median PFS was markedly different between groups, being 145 days in the lower CD3+CD4+ T cell group versus 230 days in the higher CD3+CD4+ T cell group (HR =0.50, P=0.006, Figure 5B). Similarly, the median PFS was 203 days for patients with lower CD3+CD8+ T cell levels, compared to 146 days for those with higher levels (HR =1.78, P=0.02, Figure 5C). The Kaplan-Meier survival curve analysis also examined the relationship between the CD4+/CD8+ cell ratio and PFS. In this analysis, the median PFS was 154 days in the group with a lower CD4+/CD8+ ratio and 193 days in the group with a higher ratio. Although a trend towards separation between the two survival curves was observed, the difference did not reach statistical significance (HR =0.70, P=0.18, Figure 5D).
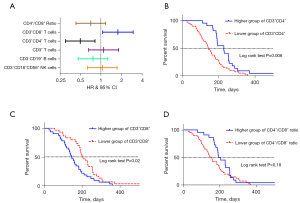
Discussion
The advent of immunotherapy has markedly enhanced survival outcomes for lung cancer patients. Nonetheless, the accurate prediction of immunotherapy efficacy remains an intricate challenge. Identifying the specific patient demographics that would most benefit from immunotherapy is critically important for optimizing clinical outcomes (14). Currently, the primary biomarkers employed for predicting the response to ICIs in lung cancer include PD-L1 expression and tumor mutational burden (TMB) (15). However, it is noteworthy that these markers have not demonstrated significant predictive value in lung cancer trials involving immunotherapy (16,17).
Nevertheless, the assessment of immunotherapy efficacy poses certain challenges due to the dynamic nature of PD-L1 expression during invasive procedures like pathological biopsies, as well as the spatial heterogeneity within tumors (18). Consequently, the exploration of dynamic and non-invasive biomarkers capable of monitoring the therapeutic response to immunotherapy holds significant clinical value (19,20). In this context, the analysis of peripheral blood lymphocyte subsets has emerged as a particularly promising approach. This method offers a non-invasive alternative for evaluating patients undergoing ICI treatment, potentially providing valuable insights into their therapeutic response and aiding in the more precise tailoring of immunotherapy strategies.
Lymphocyte subsets play a critical role in both the initiation and progression of cancer (21). Among peripheral blood T lymphocytes, CD4+ T cells and CD8+ T cells are pivotal. CD4+ T cells are instrumental in enhancing antibody production by B cells and regulating immune responses mediated by other T cells. On the other hand, CD8+ T cells possess cytotoxic abilities and serve as effector cells for cell-mediated cytotoxicity. The homeostatic balance between these subsets is essential for sustaining a robust immune response. A decrease in the CD4+/CD8+ ratio often indicates an immunosuppressive state, typically seen in cancer patients, where cellular immune function is compromised, reducing the body’s ability to recognize and eliminate mutated cells (22). In our study, a positive correlation was observed between higher CD4+/CD8+ ratios and enhanced efficacy of immunotherapy. This finding highlights the significant impact of both cellular and humoral immunity on the outcomes of immunotherapy treatments. Furthermore, CD8+ T cells can be subdivided into distinct subsets based on CD28 expression, which either positively or negatively regulates the immune response. An increase in CD8+CD28− or CD8+CD28+ cells is associated with impaired immune function (23,24). Notably, elevated levels of CD8+CD28+ T cells have been identified as an independent predictor of OS in lung cancer patients (25). Our findings reinforce the link between a patient’s immune status and their response to ICIs, as evidenced by the reduced efficacy of ICIs in patients with higher levels of CD8+ T lymphocytes.
The tumor immune microenvironment is intricately linked with both the genetic background and molecular pathological characteristics of tumors (25). Clinical studies have demonstrated notable variations in the therapeutic efficacy of ICIs across different histological types of lung cancer (26,27). This variation implies that tumors of distinct pathological types possess significant differences in their immune microenvironments, which in turn are associated with variations in peripheral blood lymphocyte subpopulations (28). Our study identified a correlation between lower levels of CD3+CD8+ T lymphocytes and improved efficacy of ICIs in patients with squamous cell carcinoma. However, such a relationship between lymphocyte subpopulations and treatment efficacy was not observed in patients with adenocarcinoma. Thus, it appears that the effectiveness of ICIs is linked not only to the overall immune status but also to the specific immune microenvironment within the tumor tissue (29). A large-scale phase III study suggests a notable distinction in PD-L1 expression patterns between non-small cell lung cancer (NSCLC) and small cell lung cancer (SCLC), with PD-L1 expression more prevalent on IC rather than TC in SCLC (5). Tumors displaying PD-L1 expression on both TC and IC exhibit distinct histological and molecular profiles. Importantly, ICs are crucial in modulating T cell responses, independent of TC PD-L1 expression (30). Additionally, circulating lymphocytes are pivotal in mediating sustained anti-tumor responses. Research on T-cell dynamics reveals that CD8+ T cells can proliferate and evolve into cytotoxic T lymphocytes (CTLs), which then migrate towards tumor sites through the peripheral bloodstream (31-33). These insights may elucidate the observed enhanced sensitivity of lymphocyte levels in predicting the efficacy of immunotherapy in SCLC in our study. Consequently, this suggests that the prognostic value of peripheral blood T cells in patients with advanced lung cancer may differ according to their histological type.
With advancing age, the human immune system experiences a progressive decline, particularly marked by a significant reduction in both the number and function of lymphocytes after the age of 60 years (34-36). This decline is partly attributed to the thymus, the primary organ responsible for T cell differentiation, development, and maturation, as its function diminishes with age, leading to a decrease in T cell production (37). Concurrently, aging is associated with an increase in the senescent phenotype of peripheral T cells and a rise in the secretion of pro-inflammatory factors (38). Aging-induced metabolic disorders in T cells disrupt pro-inflammatory pathways, potentially impacting the efficacy of treatments (39). Despite the general decline in immune function observed in the elderly, immunotherapy has been shown to remain effective in older cancer patients (40). However, our study revealed no significant correlation between lymphocyte subpopulations and the efficacy of immunotherapy in patients over the age of 60 years. This finding suggests that in elderly patients, who generally possess a weakened immune state, the efficacy of ICIs may not be determined solely by their immune status. Instead, it might be more closely associated with the molecular characteristics and the specific microenvironment of the tumor.
This study observed that patients with higher levels of CD4+ T cells in peripheral blood experienced significantly prolonged PFS. While a similar trend of extended PFS was noted in patients with higher CD4+/CD8+ ratios, this observation did not reach statistical significance. In contrast, an inverse relationship was identified between high levels of CD8+ T cells in peripheral blood and longer PFS. These data are consistent with a previous report by Li et al. (10). Furthermore, Li et al. demonstrated in their study that peripheral CD4+ T lymphocytes and the CD4+/CD8+ ratio were prognostic factors for therapeutic efficacy in breast cancer patients receiving chemotherapy combined with ICIs (11). This suggests that lymphocyte subpopulations can serve as potential biomarkers for predicting the efficacy of immune therapy. However, this study also found a positive correlation between lower levels of CD8+ and longer PFS, which may be related to the expression of various molecular markers associated with T cell exhaustion (PD-1, CTLA-4, 2B4, CD160) and T cell senescence (CD57, lack of CD28), thereby affecting the efficacy of immune therapy (41). In addition, we suggest that lower peripheral CD8+ T cell counts may reflect a more “primed” immune system that is potentially more responsive to further activation by ICIs. This could be due to the migration of activated CD8+ T cells from the periphery into the tumor site, a process which ICIs could potentially enhance. Clearly, this area requires further investigation to fully understand the mechanisms behind these observations.
In this retrospective study, we analyzed 146 advanced lung cancer patients who underwent ICI treatment. We collected baseline data on lymphocyte subpopulations and clinical information. Through subgroup analyses, we investigated the predictive role and PFS relevance of differences in the levels of lymphocyte subsets across pathology types, age groups, and immunotherapy drugs. Our findings suggest that lymphocyte status and the efficacy of immunotherapy differ significantly in these subgroups. This highlights the substantial individual variability in lymphocyte subpopulations and underscores the necessity of tailoring immunotherapy based on diverse immune states. Notably, certain lymphocyte subpopulations were found to be effective predictors of PFS in immunotherapy, suggesting the potential clinical value of non-invasive peripheral blood sampling for predicting treatment outcomes (42). This could lead to more personalized and effective treatment strategies for lung cancer patients, ensuring that patients receive the most suitable immunotherapeutic interventions based on their unique immunological landscape.
Conclusions
This study substantiates the role of CD3+CD8+ and CD3+CD4+ T lymphocytes in peripheral blood as predictive biomarkers for the efficacy of ICIs in advanced lung cancer. Our findings reveal significant variability in lymphocyte subpopulations across diverse patient groups, underlining the necessity for personalized immunotherapy approaches. The use of peripheral blood lymphocyte subsets as non-invasive biomarkers holds promise for enhancing the prediction and efficacy of immunotherapy in lung cancer.
However, it is important to recognize the limitations of our study, including its retrospective nature and the focus on short-term outcomes such as PFS, without a comprehensive analysis of OS. Additionally, the absence of detailed subgroup analysis and subclonal lymphocyte analysis suggests a direction for future research. Therefore, we advocate for prospective, large-scale clinical studies to delve deeper into the functions and mechanisms of lymphocyte subclones in immunotherapy. Such studies could further refine our understanding and enhance the clinical application of lymphocyte subpopulations, paving the way for advancements in cancer immunotherapy.
Acknowledgments
Funding: This study was supported by
Footnote
Reporting Checklist: The authors have completed the REMARK reporting checklist. Available at https://tlcr.amegroups.com/article/view/10.21037/tlcr-24-109/rc
Data Sharing Statement: Available at https://tlcr.amegroups.com/article/view/10.21037/tlcr-24-109/dss
Peer Review File: Available at https://tlcr.amegroups.com/article/view/10.21037/tlcr-24-109/prf
Conflicts of Interest: All authors have completed the ICMJE uniform disclosure form (available at https://tlcr.amegroups.com/article/view/10.21037/tlcr-24-109/coif). The authors have no conflicts of interest to declare.
Ethical Statement: The authors are accountable for all aspects of the work in ensuring that questions related to the accuracy or integrity of any part of the work are appropriately investigated and resolved. The study was meticulously conducted in strict accordance with the ethical guidelines and principles of the Helsinki Declaration (as revised in 2013), and was approved by the Ethics Review Committee of Shandong Cancer Hospital and Institute (approval No. SDTHEC2023011009). Informed consent was obtained from each participant in written form, following a comprehensive explanation of the study’s purpose and procedures.
Open Access Statement: This is an Open Access article distributed in accordance with the Creative Commons Attribution-NonCommercial-NoDerivs 4.0 International License (CC BY-NC-ND 4.0), which permits the non-commercial replication and distribution of the article with the strict proviso that no changes or edits are made and the original work is properly cited (including links to both the formal publication through the relevant DOI and the license). See: https://creativecommons.org/licenses/by-nc-nd/4.0/.
References
- Shukuya T, Carbone DP. Predictive Markers for the Efficacy of Anti-PD-1/PD-L1 Antibodies in Lung Cancer. J Thorac Oncol 2016;11:976-88. [Crossref] [PubMed]
- Reck M, Rodríguez-Abreu D, Robinson AG, et al. Pembrolizumab versus Chemotherapy for PD-L1-Positive Non-Small-Cell Lung Cancer. N Engl J Med 2016;375:1823-33. [Crossref] [PubMed]
- Mok TSK, Wu YL, Kudaba I, et al. Pembrolizumab versus chemotherapy for previously untreated, PD-L1-expressing, locally advanced or metastatic non-small-cell lung cancer (KEYNOTE-042): a randomised, open-label, controlled, phase 3 trial. Lancet 2019;393:1819-30. [Crossref] [PubMed]
- Kanabar SS, Tiwari A, Soran V, et al. Impact of PD1 and PDL1 immunotherapy on non-small cell lung cancer outcomes: a systematic review. Thorax 2022;77:1163-74. [Crossref] [PubMed]
- Liu SV, Reck M, Mansfield AS, et al. Updated Overall Survival and PD-L1 Subgroup Analysis of Patients With Extensive-Stage Small-Cell Lung Cancer Treated With Atezolizumab, Carboplatin, and Etoposide (IMpower133). J Clin Oncol 2021;39:619-30. [Crossref] [PubMed]
- Postow MA, Chasalow SD, Kuk D, et al. Absolute lymphocyte count as a prognostic biomarker for overall survival in patients with advanced melanoma treated with ipilimumab. Melanoma Res 2020;30:71-5. [Crossref] [PubMed]
- Watanabe J, Saito M, Horimoto Y, et al. A maintained absolute lymphocyte count predicts the overall survival benefit from eribulin therapy, including eribulin re-administration, in HER2-negative advanced breast cancer patients: a single-institutional experience. Breast Cancer Res Treat 2020;181:211-20. [Crossref] [PubMed]
- Sjoquist KM, Renfro LA, Simes RJ, et al. Personalizing Survival Predictions in Advanced Colorectal Cancer: The ARCAD Nomogram Project. J Natl Cancer Inst 2018;110:638-48. [Crossref] [PubMed]
- Liu A, Xia Y, Li W, et al. The Predictive Value of Changes in the Absolute Counts of Peripheral Lymphocyte Subsets for Progression and Prognosis in Breast Cancer Patients. Contrast Media Mol Imaging 2022;2022:3444360. [Crossref] [PubMed]
- Li P, Qin P, Fu X, et al. Associations between peripheral blood lymphocyte subsets and clinical outcomes in patients with lung cancer treated with immune checkpoint inhibitor. Ann Palliat Med 2021;10:3039-49. [Crossref] [PubMed]
- Li M, Xu J, Jiang C, et al. Predictive and Prognostic Role of Peripheral Blood T-Cell Subsets in Triple-Negative Breast Cancer. Front Oncol 2022;12:842705. [Crossref] [PubMed]
- Miao K, Zhang X, Wang H, et al. Peripheral Blood Lymphocyte Subsets Predict the Efficacy of Immune Checkpoint Inhibitors in Non-Small Cell Lung Cancer. Front Immunol 2022;13:912180. [Crossref] [PubMed]
- Lee KH, Kim SJ, Woo JS, et al. Prognostic significances of PD-L1- and CTLA-4-positive T cells and positive correlations of immunosuppressive marker expression between cancer tissue and peripheral blood in patients with gastric cancer. Front Immunol 2023;14:1138743. [Crossref] [PubMed]
- Wang X, Qiao Z, Aramini B, et al. Potential biomarkers for immunotherapy in non-small-cell lung cancer. Cancer Metastasis Rev 2023;42:661-75. [Crossref] [PubMed]
- Du W, Sirbu C, Lucas BD Jr, et al. A Retrospective Study of Brain Metastases From Solid Malignancies: The Effect of Immune Checkpoint Inhibitors. Front Oncol 2021;11:667847. [Crossref] [PubMed]
- Rudin CM, Awad MM, Navarro A, et al. Pembrolizumab or Placebo Plus Etoposide and Platinum as First-Line Therapy for Extensive-Stage Small-Cell Lung Cancer: Randomized, Double-Blind, Phase III KEYNOTE-604 Study. J Clin Oncol 2020;38:2369-79. [Crossref] [PubMed]
- Gandara DR, Paul SM, Kowanetz M, et al. Blood-based tumor mutational burden as a predictor of clinical benefit in non-small-cell lung cancer patients treated with atezolizumab. Nat Med 2018;24:1441-8. [Crossref] [PubMed]
- Teng F, Meng X, Kong L, et al. Progress and challenges of predictive biomarkers of anti PD-1/PD-L1 immunotherapy: A systematic review. Cancer Lett 2018;414:166-73. [Crossref] [PubMed]
- Kim ES, Velcheti V, Mekhail T, et al. Blood-based tumor mutational burden as a biomarker for atezolizumab in non-small cell lung cancer: the phase 2 B-F1RST trial. Nat Med 2022;28:939-45. [Crossref] [PubMed]
- Li T, Wang H, Xu J, et al. TGFBR2 mutation predicts resistance to immune checkpoint inhibitors in patients with non-small cell lung cancer. Ther Adv Med Oncol 2021;13:17588359211038477. [Crossref] [PubMed]
- Wang YY, Zhou N, Liu HS, et al. Circulating activated lymphocyte subsets as potential blood biomarkers of cancer progression. Cancer Med 2020;9:5086-94. [Crossref] [PubMed]
- Niwińska A, Olszewski WP. The role of stromal immune microenvironment in the progression of ductal carcinoma in situ (DCIS) to invasive breast cancer. Breast Cancer Res 2021;23:118. [Crossref] [PubMed]
- Ceeraz S, Thompson CR, Beatson R, et al. Harnessing CD8(+)CD28(-) Regulatory T Cells as a Tool to Treat Autoimmune Disease. Cells 2021;10:2973. [Crossref] [PubMed]
- Reina-Campos M, Scharping NE, Goldrath AW. CD8(+) T cell metabolism in infection and cancer. Nat Rev Immunol 2021;21:718-38. [Crossref] [PubMed]
- Chu Y, Dai E, Li Y, et al. Pan-cancer T cell atlas links a cellular stress response state to immunotherapy resistance. Nat Med 2023;29:1550-62. [Crossref] [PubMed]
- Lu S, Huang D, Chen X, et al. 1290P RATIONALE 304: Tislelizumab (TIS) plus chemotherapy (chemo) vs chemo alone as first-line (1L) treatment for non-squamous (non-sq) non-small cell lung cancer (NSCLC) in patients (pts) who are smokers vs non-smokers. Ann Oncol 2021;32:S1000-1. [Crossref]
- Yu X, Wang J, Lu S, et al. 1297P RATIONALE 307: Tislelizumab (TIS) plus chemotherapy (chemo) vs chemo alone as first-line (1L) treatment for advanced squamous non-small cell lung cancer (sq NSCLC) in patients (pts) who were smokers vs non-smokers. Ann Oncol 2021;32:S1005. [Crossref]
- Kim H, Park S, Han KY, et al. Clonal expansion of resident memory T cells in peripheral blood of patients with non-small cell lung cancer during immune checkpoint inhibitor treatment. J Immunother Cancer 2023;11:e005509. [Crossref] [PubMed]
- Lin G, Li C, Li P, et al. Immune heterogeneity in adenocarcinoma and squamous cell carcinoma components of lung adenosquamous carcinoma. J Clin Oncol 2021;39:e20523. [Crossref]
- Kowanetz M, Zou W, Gettinger SN, et al. Differential regulation of PD-L1 expression by immune and tumor cells in NSCLC and the response to treatment with atezolizumab (anti-PD-L1). Proc Natl Acad Sci U S A 2018;115:E10119-26. [Crossref] [PubMed]
- Kamphorst AO, Pillai RN, Yang S, et al. Proliferation of PD-1+ CD8 T cells in peripheral blood after PD-1-targeted therapy in lung cancer patients. Proc Natl Acad Sci U S A 2017;114:4993-8. [Crossref] [PubMed]
- Kim KH, Cho J, Ku BM, et al. The First-week Proliferative Response of Peripheral Blood PD-1(+)CD8(+) T Cells Predicts the Response to Anti-PD-1 Therapy in Solid Tumors. Clin Cancer Res 2019;25:2144-54. [Crossref] [PubMed]
- Kagamu H, Kitano S, Yamaguchi O, et al. CD4(+) T-cell Immunity in the Peripheral Blood Correlates with Response to Anti-PD-1 Therapy. Cancer Immunol Res 2020;8:334-44. [Crossref] [PubMed]
- Elyahu Y, Monsonego A. Thymus involution sets the clock of the aging T-cell landscape: Implications for declined immunity and tissue repair. Ageing Res Rev 2021;65:101231. [Crossref] [PubMed]
- Shinzawa M, Moseman EA, Gossa S, et al. Reversal of the T cell immune system reveals the molecular basis for T cell lineage fate determination in the thymus. Nat Immunol 2022;23:731-42. [Crossref] [PubMed]
- Luan D, Willard-Gallo K, Garaud S, et al. Transcription Factors and Checkpoint Inhibitor Expression with Age: Markers of Immunosenescence? Blood 2016;128:5983. [Crossref]
- Bao X, Qin Y, Lu L, et al. Transcriptional Regulation of Early T-Lymphocyte Development in Thymus. Front Immunol 2022;13:884569. [Crossref] [PubMed]
- Sprenger HG, MacVicar T, Bahat A, et al. Cellular pyrimidine imbalance triggers mitochondrial DNA-dependent innate immunity. Nat Metab 2021;3:636-50. [Crossref] [PubMed]
- Duong E, Fessenden TB, Lutz E, et al. Type I interferon activates MHC class I-dressed CD11b(+) conventional dendritic cells to promote protective anti-tumor CD8(+) T cell immunity. Immunity 2022;55:308-323.e9. [Crossref] [PubMed]
- Xu Q, Zhang X, Huang M, et al. Comparison of Efficacy and Safety of Single and Double Immune Checkpoint Inhibitor-Based First-Line Treatments for Advanced Driver-Gene Wild-Type Non-Small Cell Lung Cancer: A Systematic Review and Network Meta-Analysis. Front Immunol 2021;12:731546. [Crossref] [PubMed]
- Zelle-Rieser C, Thangavadivel S, Biedermann R, et al. T cells in multiple myeloma display features of exhaustion and senescence at the tumor site. J Hematol Oncol 2016;9:116. [Crossref] [PubMed]
- Puig-Saus C, Sennino B, Peng S, et al. Neoantigen-targeted CD8(+) T cell responses with PD-1 blockade therapy. Nature 2023;615:697-704. [Crossref] [PubMed]