Tumor mutation burden and FAT3 mutation influence long-term survival in surgically resected small cell lung cancer
Highlight box
Key findings
• A high non-synonymous tumor mutation burden (TMB) and the FAT3 mutation could potentially influence long-term survival (LTS) in surgically resected small cell lung cancer (SCLC).
What is known and what is new?
• Only part of the population of patients with SCLC resection achieved LTS, and it is unknown whether genetic alterations impact on the prognosis of patients with LTS.
• Compared with short-term survival (STS) patients, LTS patients had higher concentrations of TMB and the FAT3 mutation.
What is the implication, and what should change now?
• Gene alteration represented the survival difference between LTS and STS in resected SCLC; high TMB may be a prognostic biomarker for LTS in resected SCLC, regardless of disease stage. FAT3 may be a potential and interesting gene to study. And these findings deserve further investigation.
Introduction
Lung cancer is the leading cause of cancer mortality worldwide and in China (1,2). Small cell lung cancer (SCLC) accounts for about 15% of all lung cancers, and it is recognized as a highly aggressive and lethal malignancy, which is usually characterized by early progression and extensive metastasis at diagnosis, striking recurrence and progressive evolution during treatment (3-5). Consequently, SCLC has a miserable prognosis and very rare opportunities of long-term survival (LTS), with a 5-year survival rate of less than 7% (3). Based on its clinical stages, SCLC patients can be divided into limited-stage (LS) and extensive-stage (ES). ES-SCLC patients are theoretically incurable. The median overall survival (mOS) of ES-SCLC patients who received current standard first-line therapeutic regimen as immunotherapy combined with chemotherapy is approximately 12.3–15.5 months (6-9). While the mOS of patients with LS is approximately 28.5–30.1 months, with 5-year OS of about 30% (10). Thus, there is a chance that LS patients could be cured by standardized chemoradiotherapy and preventive intracranial irradiation (PCI). Additionally, it is noteworthy that the mOS has been reported to range from 29–91 months for early stage SCLC patients who receive surgically resected as the main treatment and adjuvant treatments; thus, only a portion of the population achieve LTS (11-14), and the potentially reasons deserve further exploration.
Despite the existence of rare long-term survivors of SCLC, there is still a lack of prognostic factors other than disease staging and performance status (PS). In addition, at present, it is not yet known why some advanced SCLC patients achieve LTS, and why some early stage SCLC patients relapse. In the absence of adjusted multivariate analyses, it is a challenge to evaluate the prognostic significance of reported biomarkers beyond clinical variables. New SCLC subtypes based on key transcription regulators ASCL1-high (SCLC-A), NEUROD1-high (SCLC-N), POU2F3-high (SCLC-P), and YAP1-high (SCLC-Y) as well as on certain inflammatory characteristics (SCLC-I) were defined (15,16) and analyzed in relation to tumor evolution or immunotherapy effect (16,17). Prognostic relevance of transcription subtypes in surgically resected SCLC found high POU2F3 expression is associated with improved survival whereas elevated ASCL1 expression is an independent negative prognosticator (18). But the mechanism of long survival is still not convincingly explained. At present, the determinants of the LTS of SCLC patients are largely unknown. Therefore, comprehensive genomic profiling is required to establish more robust prognostic markers for operable SCLC.
To explore potential genetic alterations and identify prognostic biomarkers beyond stage for LTS, we performed a retrospective study comparing surgically resected tumors for late-stage (stage III or IIB) LTS with tumors for early stage (stage I) short-term survival (STS). We investigated the genomic profiling and tumor mutation burden (TMB) of LTS and STS using a gene panel (OrigiMed, Shanghai, China) for resected SCLCs. The gene panel covers all the coding exons of 450 cancer-related genes and 64 selected introns in 39 genes that are frequently rearranged in solid tumors. We hypothesized that genetic differences contribute to survival. Our data provide the first potential evidence for distinguishing between the genetic differences of LTS and STS resected SCLC patients. We present this article in accordance with the REMARK reporting checklist (available at https://tlcr.amegroups.com/article/view/10.21037/tlcr-24-467/rc).
Methods
Sample collection
All 52 patients were retrospectively collected, diagnosed with conventional SCLC, and underwent surgery without neoadjuvant chemotherapy or chemoradiotherapy at Zhejiang Cancer Hospital (Hangzhou, China) between April 2008 and December 2017 (19), and were followed up for at least 2 years. The pathological diagnoses for SCLC were based on standard criteria defined by the World Health Organization’s classification system (20). Tumor stage was defined according to the lung cancer tumor, node, and metastasis (TNM) classification system, eighth edition (21). The selection process for the SCLC patients is shown in Figure 1. We defined LTS as an overall survival (OS) ≥4 years and STS as an OS <2 years. We screened LTS patients in stages IIB through III. In total, six patients with stage IIB–III met the standard for LTS, and five patients with stage I met the standard for STS (Figure 1). All six patients received the same adjuvant therapy of four cycles of etoposide combined with cisplatin after surgery.
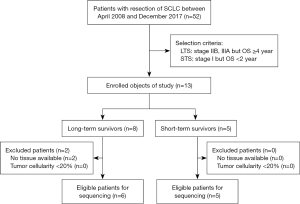
The study was conducted in accordance with the Declaration of Helsinki (as revised in 2013). Our research was approved by the Medical Ethics Committee of the Zhejiang Cancer Hospital [reference number IRB-2016-86 (Ke)]. Informed consent was waived due to the retrospective nature of the study.
Sample preparation
Tumor-rich tissue and normal lung tissue were fixed in formalin, embedded in paraffin and sectioned at 4 µm. DNA was isolated from at least 1 cm3 of tumor tissue using the Cobas R DNA Sample Preparation Kit (Roche Molecular Systems, Pleasanton, CA, USA), according to the manufacturer’s protocol. The concentration of double-stranded DNA (dsDNA) was determined using the Qubit R 2.0 Fluorometer and the Qubit R 2.0 dsDNA HS Assay Kit (both from ThermoFisher Scientific, Waltham, MA, USA). The quality of the sample DNA was evaluated using a specimen control-size ladder test (Invivoscribe Technologies, San Diego, CA, USA).
NGS
Genomic information was obtained by employing a next-generation sequencing (NGS)-based YuanSuTM 450 gene panel (OrigiMed, Shanghai, China) that covers all the coding exons of 450 cancer-related genes and 64 selected introns of genes frequently rearranged in 39 solid tumors. Using Illumina NextSeq 500 (Illumina Inc., San Diego, CA, USA), the genes were captured and sequenced, with a mean depth of 800×. Genomic alterations (GAs) were identified by aligning sequences from tumor tissues and matched normal lung tissue, according to previously reported methods (22). Single-nucleotide variants (SNVs) were identified by MuTect (v.1.7) (23), and insertion-deletions (Indels) were identified by PINDEL (v.0.2.5) (24). The functional effect of the GAs was annotated by SnpEff3.0 (25). Copy number variation regions were identified by Control-FREEC (v.9.7) (26) with the following parameters: window =50,000; and step =10,000. Gene fusions were detected by an in-house developed pipeline and assessed using an Integrative Genomics Viewer (27). The TMB was calculated by counting the somatic mutations containing SNVs and Indels in each million base detection sequence of each patient. Driver mutations and recorded germline alterations were not counted.
Statistical analyses
The statistical analyses were performed using SPSS (v.22.0, SPSS Inc., IL, USA). The significance of differences was analyzed using Fisher’s exact test or Wilcoxon rank sum test. A P value less than 0.05 (P<0.05) indicated a statistically significant difference.
Follow-up period
The follow-up deadline was March 17, 2020. As of this date, five patients were alive, six patients were deceased, and no patient had been lost follow-up. Survival time was calculated from the date of the pathological diagnosis to the follow-up deadline.
Results
Patient characteristics
The mOS of the LTS group was 87 months (range, 51–143 months), and that of the STS group was 18 months (range, 16–22 months). Patient characteristics are set out in Table 1. The LTS group comprised three male and three female patients, while the STS group comprised five male patients only. The median age of patients in the LTS group was 57 years (range, 49–63 years), while that of patients in the STS group was 57.8 years (range, 38–76 years). Six patients were smokers, and the five remaining patients had no history of smoking (Table 1).
Table 1
Characteristics | LTS (N=6) | STS (N=5) |
---|---|---|
Age, years | ||
Median [range] | 57 [49–63] | 57.8 [38–76] |
Sex, n (%) | ||
Male | 3 (50.0) | 5 (100.0) |
Female | 3 (50.0) | 0 |
TMB, mutations/Mb | ||
Median | 16.4 | 8.5 |
Mean ± SD | 19.25±9.48 | 9.88±5.35 |
Smoking history, n (%) | ||
Yes | 2 (33.3) | 4 (80.0) |
No | 4 (66.7) | 1 (20.0) |
Overall survival, months | ||
Median | >48 | 18 |
Mean ± SD | 19±2.83 |
LTS, long-term survival; STS, short-term survival; TMB, tumor mutation burden; SD, standard deviation.
TMB in the LTS and STS groups
The median TMBs of the LTS and STS groups were 16.4 (19.25±9.48) and 8.5 (9.88±5.35) mutations/Mb, respectively. We defined 10 mutations/Mb as the cut-off value (28). A value higher than 10 (TMB >10 mutations/Mb) was considered high, and a value lower than and/or equal to 10 (TMB ≤10 mutations/Mb) was considered low. Differences between the LTS and STS groups were assessed using Wilcoxon test. The P value was 0.08 (Figure 2). The results of the univariate analysis also showed that the OS of the high tumor mutational burden (TMB-H) patients was significantly longer than that of the low tumor mutational burden (TMB-L) patients [not available (N/A) vs. 17 months, P=0.007] (Figure 3). Additionally, the results of the univariate analysis indicated that a TMB-H was a prognostic factor for OS (hazard ratio =0.12, 95% confidence interval: 0.02–0.76, P=0.024). The clinical characteristics and TMB value of each patient are provided in Table 2.
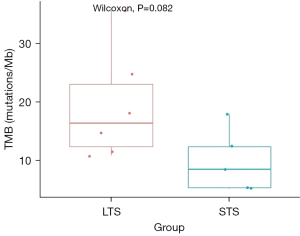
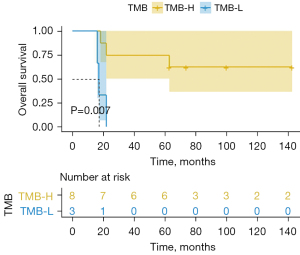
Table 2
Case | Sex | Age (years) | Smoking (pack-years) | pTNM | pStage | TMB value (mutations/Mb) | TMB (H/L) | DFS (months) | OS (months) |
---|---|---|---|---|---|---|---|---|---|
1 | Male | 49 | 0.0 | pT2aN2M0 | IIIA | 14.7 | TMB-H | 143 | 143 |
2 | Male | 59 | 7.5 | pT3N1M0 | IIIA | 35.6 | TMB-H | 143 | 143 |
3 | Male | 42 | 40.0 | pT1cN0M0 | IA3 | 8.5 | TMB-L | 8 | 16 |
4 | Male | 38 | 12.0 | pT1cN0M0 | IA3 | 5.4 | TMB-L | 5 | 22 |
5 | Female | 63 | 0.0 | pT2bN1M0 | IIB | 24.7 | TMB-H | 41 | 63 |
6 | Male | 50 | 0.0 | pT1cN2M0 | IIIA | 10.8 | TMB-H | 74 | 74 |
7 | Female | 61 | 60.0 | pT3N1M0 | IIIA | 11.6 | TMB-H | 100 | 100 |
8 | Female | 60 | 0.0 | pT1cN2M0 | IIIA | 18.1 | TMB-H | 63 | 63 |
9 | Male | 64 | 120.0 | pT2aN0M0 | IB | 12.4 | TMB-H | 8 | 22 |
10 | Male | 69 | 0.0 | pT1bN0M0 | IA2 | 17.9 | TMB-H | 12 | 18 |
11 | Male | 76 | 40.0 | pT1cN0M0 | IA3 | 5.2 | TMB-L | 10 | 17 |
TMB, tumor mutation burden; TMB-L, low TMB <10 mutations/Mb; TMB-H, high TMB >10 mutations/Mb; pTNM, pathological tumor, node, and metastasis; DFS, disease-free survival; OS, overall survival.
Sequence analysis and mutation identification of LTS and STS groups
In total, 29 somatic mutated genes were identified in the entire 11 samples (which comprised 6 LTS samples and 5 STS samples). All of the SCLC samples carried a minimum of 5.5 gene mutations. The top 10 genes with the highest mutation frequency were TP53 (N=11, 100.0%), RB1 (N=8, 73.0%), KMT2D (N=5, 45.0%), NOTCH1 (N=5, 45.0%), FAM135B (N=4, 36.0%), FAT3 (N=4, 36.0%), LRP1B (N=4, 36.0%), CDKN2C (N=3, 27.3%), H3F3A (N=3, 27.3%), and FAT4 (N=3, 27.3%) (Figure 4). For these mutated genes, in each sample, the median of mutated genes in the LTS group was 10 (range, 6–13), and that of the STS group was 7 (range, 3–8), while the medians of the mutated genes in the LTS and STS groups were 28 and 17, respectively. FAT3, CDKN2C, H3F3A, ARAF, B2M, CUL3, EPHA3, FAT1, FLT4, PRKDC, and STAT3 were only discovered in the LTS patients, and PTEN was unique to the STS patients (Table 3). The FAT3 gene was only detected in four LTS patients, and the corresponding P value, as determined by Fisher’s exact test, was 0.06. The association between the FAT3 gene and survival is shown in Figure 5.
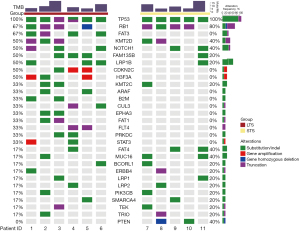
Table 3
Gene | Mutated samples, n [%] | |
---|---|---|
LTS (N=6) | STS (N=5) | |
TP53 | 6 [100] | 5 [100] |
RB1 | 4 [67] | 4 [80] |
FAT3 | 4 [67] | 0 [0] |
KMT2D | 3 [50] | 2 [40] |
NOTCH1 | 3 [50] | 2 [40] |
FAM135B | 3 [50] | 1 [20] |
LRP1B | 3 [50] | 1 [20] |
CDKN2C | 3 [50] | 0 [0] |
H3F3A | 3 [50] | 0 [0] |
KMT2C | 2 [33] | 1 [20] |
ARAF | 2 [33] | 0 [0] |
B2M | 2 [33] | 0 [0] |
CUL3 | 2 [33] | 0 [0] |
EPHA3 | 2 [33] | 0 [0] |
FAT1 | 2 [33] | 0 [0] |
FLT4 | 2 [33] | 0 [0] |
PRKDC | 2 [33] | 0 [0] |
STAT3 | 2 [33] | 0 [0] |
FAT4 | 1 [17] | 2 [40] |
MUC16 | 1 [17] | 2 [40] |
BCORL1 | 1 [17] | 1 [20] |
ERBB4 | 1 [17] | 1 [20] |
LRP1 | 1 [17] | 1 [20] |
LRP2 | 1 [17] | 1 [20] |
PIK3CB | 1 [17] | 1 [20] |
SMARCA4 | 1 [17] | 1 [20] |
TEK | 1 [17] | 1 [20] |
TRIO | 1 [17] | 1 [20] |
PTEN | 0 [0] | 2 [40] |
LTS, long-term survival; STS, short-term survival.
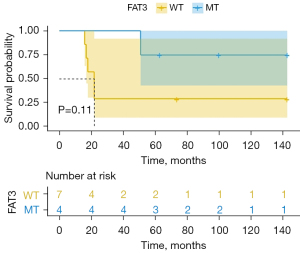
The correlation between the TMB and FAT3 mutation
The FAT3 gene mutation was detected in 4 of the 6 patients in the LTS group. None of the STS patients had the FAT3 mutation. The TMB values of the four LTS patients with FAT3 mutation were 18.1, 14.7, 24.7, 11.6 mutations/Mb, indicating that they were a TMB-H population. The correlation analysis between the TMB and FAT3 mutation was determined by a Wilcoxon test, and the P value was 0.23 (Figure 6). Thus, no significant correlation was found between FAT3 and TMB.
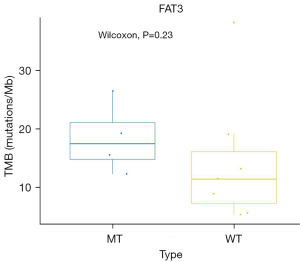
Discussion
Distinct individual differences in survival cannot be accurately predicted using existing prognostic variables (29). It was hypothesized that the genetic profiling of tumors in these patients would lead to the discovery of new prognostic markers. Based on our data, we propose that a TMB-H may be a prognostic biomarker for LTS in resected SCLC, regardless of disease stage. Interestingly, we found that the FAT3 mutation could be used to distinguish between the patients in the LTS and STS groups. The number of samples included in the current study is limited; however, our data revealed a promising discovery that deserves further investigation.
We explored genetic characteristic differences between the LTS and STS patients and found a TMB-H in the tumors from the LTS group. Specifically, we found that the LTS patients had a higher median TMB than the STS patients [16.4 (range, 10.8–35.6) vs. 8.5 (range, 5.2–17.9) mutations/Mb]. We also observed a notable trend in the median TMBs of the LTS and STS groups, approaching a statistically significant difference (P=0.08) (Figure 2). An exploratory analysis suggested that the TMB may have a significant predictive effect on OS (P=0.007) (Figure 3). In relation to the patients with a TMB-H and a TMB-L, the former group did not reach the mOS, while the latter group survived 17 months. These findings provide valuable evidence of the favorable prognostic value of a TMB-H.
Multiple studies demonstrate TMB has potential prognostic value in surgically resected SCLCs. This is different in ES-SCLCs with first-line immunotherapy combined with chemotherapy (30-32). A recent study from Liu et al. derived from the genomic profiling of 112 surgical resected SCLC and found TMB-high (a median TMB 5.45 mutations/Mb as cut-off) patients tended to have a better OS (P=0.0068) (33). Zhou et al. (34) collected 120 tumor samples from 40 stage I–III surgically resected SCLC patients and found a median TMB of 10.2 (range, 1.1–51.7) mutations/Mb. A higher TMB per cluster was associated with better disease-free survival (DFS), while the SNV intertumoral heterogeneity was linked to worse OS. Yuan et al. (35) analyzed surgically resected tumor tissues from 50 SCLC patients and reported similar results, which suggests that patients with a TMB-H (≥7 mutations/Mb) have a better prognosis than those with a TMB-L (P=0.0053). Zhou et al. (36) analyzed the correlation between clinical outcomes and GAs in 53 SCLC samples (of which 24 cases were LS and 29 cases were ES), and reported that a TMB-H (>21 mutations/Mb) was connected with a favorable OS (21.7 vs. 10.4 months, P=0.012). These results were consistent with our findings, suggesting the potential prognostic value of TMB in SCLC. Conversely, Kachroo et al. (37) recently found that there was no difference in the TMB survival rate of 186 SCLC patients. In their study, 71% of the cases were ES, and the mOS from the time of diagnosis was 14.9 and 14.7 months for the TMB-H and non-TMB-H patients, respectively. The reason for the inconsistencies in these results may be that the patients in these studies were at different stages of the disease.
We compared the genomic profiling data obtained in our study with the data obtained from a recent study, and we found that the FAT3 mutation displayed the most significant differences in the data set. Our results indicated that the top 10 genes with the highest mutation frequency were TP53 (100.0%), RB1 (73.0%), KMT2D (45.0%), NOTCH1 (45.0%), FAM135B (36.0%), FAT3 (36.0%), LRP1B (36.0%), CDKN2C (27.3%), H3F3A (27.3%), and FAT4 (27.3%). Liu et al. study reported that the most frequently altered top 10 genes in SCLC were TP53 (72%), RB1 (56%), KMT2C (21%), ZFHX3 (19%), KMT2D (16%), FAT1 (12%), FAT4 (12%), NOTCH1 (12%), NOTCH3 (12%), CREBBP (12%). The analysis of identification and validation of proteomic prognostic biomarkers revealed that HMGB3 showed elevated expression in tumors and was correlated with worse survival, whereas CASP10 showed decreased expression and was associated with a better prognosis (33). Based on our results, it is obvious that the FAT3 mutation was enriched in the tumor tissue of the LTS patients. Intriguingly, when we explored the genetic characteristic differences between LTS and STS, the FAT3 mutation was found in tumors from the LTS group. In the LTS group, the median number of mutated genes was 10 (range, 6–13) per sample, and the samples harbored 60 gene mutations in the following 28 genes: TP53 (n=6), RB1 (n=4), FAT3 (n=4), KMT2D (n=3), NOTCH1 (n=3), FAM135B (n=3), LRP1B (n=3), CDKN2C (n=3), and H3F3A (n=3). For the mutated genes per sample in the STS group, the median number was 7 (range, 3–8), and 30 mutations were found for 17 genes: TP53 (n=5), RB1 (n=4), KMT2D (n=2), NOTCH1 (n=2), FAT4 (n=2), MUC16 (n=2), PTEN (n=2), FAM135B (n=1), LRP1B (n=1), and KMT2C (n=1). The FAT3 mutation only occurred in the LTS group. The P value, as determined by Fisher’s exact test, from the comparison with the STS group was 0.06, indicating that the role of FAT3 in SCLC is meaningful and worthy of further study. The contrastive analysis indicated that FAT3 could be used to identify LTS patients.
In recent studies using targeted deep sequencing, the FAT3 mutation has been found to be associated with a high level of TMB, neoantigens, and tumor-infiltrating immunity, which may predict the efficacy of immunotherapy (37-42). Kachroo et al. performed a retrospective analysis of 179 SCLC patients and found that FAT3, along with LRP1B, MLL3, MED12, and NOTCH3, are significantly associated with the TMB-H (37). Moreover, FAT3, along with KRAS, TTN, RYR2, MUC16, TP53, USH2A, ZFHX4, KEAP1, STK11, NAV3, and EGFR, are the most commonly mutated genes with predictive neoantigens in patients with lung adenocarcinoma, which may have significance for immune-associated prognostic biomarkers and vaccine design (38). Using 506 lung adenocarcinoma samples from The Cancer Genome Atlas (TCGA) database, co-mutations of FAT3 and LRP1B were screened out, which increased the somatic mutational load, facilitated lymphocyte infiltration, and predicted the efficacy of immunotherapy (39). Another study drew a similar conclusion, co-mutations of FAT3 and LRP1B have potential to be used as biomarkers to predict the positive effectiveness of immunotherapy for NSCLC (40). Similarly, co-mutation of FAT3 and LRP1B not only leads to activation of the immune state, but also represents a subgroup with an improved prognosis, particularly in the microsatellite instability-high (MSI-H) subtype (41). A study of identification of signature of tumor-infiltrating CD8+ T lymphocytes in prognosis and immunotherapy of colon cancer by machine learning from GEO database, showed co-mutations of FAT3 and UNC13C can predict the better prognosis and the higher immunotherapy response (42). However, the association between FAT3 mutation and survival was not investigated in the first study of SCLC. The correlation between FAT3 mutation or co-mutation with other genes and immunotherapy efficacy or immune status deserves further dissection in SCLC.
Two potential explanations may explain why the FAT3 mutation is enriched in LTS patients. First, the FAT3 mutation is associated with neoantigens, which have higher immunogenicity than activating T cells in stimulating the immune response. Second, while current understandings are limited, the FAT3 mutation may be involved in the regulation of CD8+ T cell infiltration in the tumor immune microenvironment. Therefore, people with these characters have a better prognosis.
Our study derived retrenchment from its design and specific patient selection. Genomic profiling data were compared between two groups of patients that differed distinctly in terms of their survival status. To emphasize the role of our genomic analysis, our retrospective study was designed to select LTS patients with advanced (stage IIB or III) SCLC, who underwent surgery to remove tumors, for comparison with STS patients with early (stage I) SCLC. It should be noted that all specimens were obtained from surgery. Therefore, combined SCLC patients were able to be excluded, and the molecular profiles were more accurately reflective than the biopsy specimens. We encountered difficulties in obtaining sufficient SCLC tumor samples, which is a dilemma for all translational research on SCLC, as there is a limited supply of tissue samples for molecular studies. In our study, sections with sufficient tumor cellularity were available, and high-quality DNA was isolated in abundant concentrations.
Our study had several limitations. The small sample size represents the biggest limitation of this study, as it made it somewhat difficult to achieve strong statistical significance. The intention of our study is to explore the genetic characteristics of LTS in surgically resected III stage SCLC, the population with high-risk relapse, theoretically. As a result, the number of patients meeting these criteria is rare. Due to the low sample size of the clinical patients and the subsequent insufficient power for statistical significance, it is difficult to ascertain whether a similar strategy could be applied for clinical patients. Fortunately, our results were very consistent with previous research findings, high TMB having a better prognosis in surgically resected SCLC (33-35). Additionally, the study was retrospective and uncontrolled. Selection bias of the study sample is inevitable, such as gender and smoking history. There were few surgical specimens due to less opportunities for surgery. Due to the lack of tissue availability, scientific studies on SCLC molecular profiles are hampered. In addition, to completely ignore the effect of stage on prognosis, we selected two extreme cohorts of LTS (stages IIB–III) and STS (stage I) as the focus of our study. All the patients in our study had a follow-up period longer than 2 years. Therefore, the number of patients meeting the study conditions was very small. Five cases of STS with early-stage SCLC were added for a control comparison; however, the small sample size severely limited the statistical effect and the extent to which conclusive trends could be observed. Nevertheless, our study was able to provide a new discovery of LTS in SCLC, and the FAT3 gene deserves further attention. Moreover, our study focuses on the genetic characteristics of LTS postoperative patients and does not involve immunotherapy efficacy. The correlation of FAT3 gene mutations and the potential therapeutic benefits of immune checkpoint inhibitors (ICIs) in ES-SCLC is well worth further discussion.
Conclusions
In conclusion, we studied the genetic alterations profiles and TMB for LTS and STS patients. Our results indicate that a high non-synonymous TMB and the FAT3 mutation could serve as useful prognostic biomarkers for LTS in resected SCLC. Further research needs to be conducted to confirm these observations and explore the mechanisms underlying this association.
Acknowledgments
The authors would like to thank Qiuyue Pan, Lei Wang from OrigiMed for their excellent work in providing statistical support.
Funding: None.
Footnote
Reporting Checklist: The authors have completed the REMARK reporting checklist. Available at https://tlcr.amegroups.com/article/view/10.21037/tlcr-24-467/rc
Data Sharing Statement: Available at https://tlcr.amegroups.com/article/view/10.21037/tlcr-24-467/dss
Peer Review File: Available at https://tlcr.amegroups.com/article/view/10.21037/tlcr-24-467/prf
Conflicts of Interest: All authors have completed the ICMJE uniform disclosure form (available at https://tlcr.amegroups.com/article/view/10.21037/tlcr-24-467/coif). The authors have no conflicts of interest to declare.
Ethical Statement: The authors are accountable for all aspects of the work in ensuring that questions related to the accuracy or integrity of any part of the work are appropriately investigated and resolved. The study was conducted in accordance with the Declaration of Helsinki (as revised in 2013). This study was approved by the Medical Ethics Committee of the Zhejiang Cancer Hospital [reference number IRB-2016-86 (Ke)]. Informed consent was waived due to the retrospective nature of the study.
Open Access Statement: This is an Open Access article distributed in accordance with the Creative Commons Attribution-NonCommercial-NoDerivs 4.0 International License (CC BY-NC-ND 4.0), which permits the non-commercial replication and distribution of the article with the strict proviso that no changes or edits are made and the original work is properly cited (including links to both the formal publication through the relevant DOI and the license). See: https://creativecommons.org/licenses/by-nc-nd/4.0/.
References
- Bray F, Laversanne M, Sung H, et al. Global cancer statistics 2022: GLOBOCAN estimates of incidence and mortality worldwide for 36 cancers in 185 countries. CA Cancer J Clin 2024;74:229-63. [Crossref] [PubMed]
- He S, Xia C, Li H, et al. Cancer profiles in China and comparisons with the USA: a comprehensive analysis in the incidence, mortality, survival, staging, and attribution to risk factors. Sci China Life Sci 2024;67:122-31. [Crossref] [PubMed]
- Megyesfalvi Z, Gay CM, Popper H, et al. Clinical insights into small cell lung cancer: Tumor heterogeneity, diagnosis, therapy, and future directions. CA Cancer J Clin 2023;73:620-52. [Crossref] [PubMed]
- George J, Maas L, Abedpour N, et al. Evolutionary trajectories of small cell lung cancer under therapy. Nature 2024;627:880-9. [Crossref] [PubMed]
- Petty WJ, Paz-Ares L. Emerging Strategies for the Treatment of Small Cell Lung Cancer: A Review. JAMA Oncol 2023;9:419-29. [Crossref] [PubMed]
- Horn L, Mansfield AS, Szczęsna A, et al. First-Line Atezolizumab plus Chemotherapy in Extensive-Stage Small-Cell Lung Cancer. N Engl J Med 2018;379:2220-9. [Crossref] [PubMed]
- Paz-Ares L, Dvorkin M, Chen Y, et al. Durvalumab plus platinum-etoposide versus platinum-etoposide in first-line treatment of extensive-stage small-cell lung cancer (CASPIAN): a randomised, controlled, open-label, phase 3 trial. Lancet 2019;394:1929-39. [Crossref] [PubMed]
- Cheng Y, Fan Y, Zhao Y, et al. Tislelizumab Plus Platinum and Etoposide Versus Placebo Plus Platinum and Etoposide as First-Line Treatment for Extensive-Stage SCLC (RATIONALE-312): A Multicenter, Double-Blind, Placebo-Controlled, Randomized, Phase 3 Clinical Trial. J Thorac Oncol 2024; Epub ahead of print. [Crossref]
- Cheng Y, Han L, Wu L, et al. Effect of First-Line Serplulimab vs Placebo Added to Chemotherapy on Survival in Patients With Extensive-Stage Small Cell Lung Cancer: The ASTRUM-005 Randomized Clinical Trial. JAMA 2022;328:1223-32. [Crossref] [PubMed]
- Bogart J, Wang X, Masters G, et al. High-Dose Once-Daily Thoracic Radiotherapy in Limited-Stage Small-Cell Lung Cancer: CALGB 30610 (Alliance)/RTOG 0538. J Clin Oncol 2023;41:2394-402. [Crossref] [PubMed]
- Varlotto JM, Recht A, Flickinger JC, et al. Lobectomy leads to optimal survival in early-stage small cell lung cancer: a retrospective analysis. J Thorac Cardiovasc Surg 2011;142:538-46. [Crossref] [PubMed]
- Leuzzi G, Lococo F, Alessandrini G, et al. Prognostic Impact of Node-Spreading Pattern in Surgically Treated Small-Cell Lung Cancer: A Multicentric Analysis. Lung 2017;195:107-14. [Crossref] [PubMed]
- Zhu H, Zhou Z, Xue Q, et al. Treatment modality selection and prognosis of early stage small cell lung cancer: retrospective analysis from a single cancer institute. Eur J Cancer Care (Engl) 2013;22:789-96. [Crossref] [PubMed]
- Yokouchi H, Ishida T, Yamazaki S, et al. Prognostic impact of clinical variables on surgically resected small-cell lung cancer: Results of a retrospective multicenter analysis (FIGHT002A and HOT1301A). Lung Cancer 2015;90:548-53. [Crossref] [PubMed]
- Rudin CM, Poirier JT, Byers LA, et al. Molecular subtypes of small cell lung cancer: a synthesis of human and mouse model data. Nat Rev Cancer 2019;19:289-97. [Crossref] [PubMed]
- Gay CM, Stewart CA, Park EM, et al. Patterns of transcription factor programs and immune pathway activation define four major subtypes of SCLC with distinct therapeutic vulnerabilities. Cancer Cell 2021;39:346-360.e7. [Crossref] [PubMed]
- Ireland AS, Micinski AM, Kastner DW, et al. MYC Drives Temporal Evolution of Small Cell Lung Cancer Subtypes by Reprogramming Neuroendocrine Fate. Cancer Cell 2020;38:60-78.e12. [Crossref] [PubMed]
- Megyesfalvi Z, Barany N, Lantos A, et al. Expression patterns and prognostic relevance of subtype-specific transcription factors in surgically resected small-cell lung cancer: an international multicenter study. J Pathol 2022;257:674-86. [Crossref] [PubMed]
- Lu H, Qin J, Xu H, et al. O(6)-methyl-guanine-DNA methyltransferase methylation and IDH1/2 mutation in small cell lung cancer. Exp Ther Med 2017;14:398-402. [Crossref] [PubMed]
- Travis WD, Brambilla E, Nicholson AG, et al. The 2015 World Health Organization Classification of Lung Tumors: Impact of Genetic, Clinical and Radiologic Advances Since the 2004 Classification. J Thorac Oncol 2015;10:1243-60. [Crossref] [PubMed]
- Goldstraw P, Chansky K, Crowley J, et al. The IASLC Lung Cancer Staging Project: Proposals for Revision of the TNM Stage Groupings in the Forthcoming (Eighth) Edition of the TNM Classification for Lung Cancer. J Thorac Oncol 2016;11:39-51. [Crossref] [PubMed]
- Hu J, Wang Y, Zhang Y, et al. Comprehensive genomic profiling of small cell lung cancer in Chinese patients and the implications for therapeutic potential. Cancer Med 2019;8:4338-47. [Crossref] [PubMed]
- Cibulskis K, Lawrence MS, Carter SL, et al. Sensitive detection of somatic point mutations in impure and heterogeneous cancer samples. Nat Biotechnol 2013;31:213-9. [Crossref] [PubMed]
- Ye K, Schulz MH, Long Q, et al. Pindel: a pattern growth approach to detect break points of large deletions and medium sized insertions from paired-end short reads. Bioinformatics 2009;25:2865-71. [Crossref] [PubMed]
- Cingolani P, Platts A. A program for annotating and predicting the effects of single nucleotide polymorphisms, SnpEff: SNPs in the genome of Drosophila melanogaster strain w1118; iso-2; iso-3. Fly (Austin) 2012;6:80-92. [Crossref] [PubMed]
- Boeva V, Popova T, Bleakley K, et al. Control-FREEC: a tool for assessing copy number and allelic content using next-generation sequencing data. Bioinformatics 2012;28:423-5. [Crossref] [PubMed]
- Thorvaldsdóttir H, Robinson JT, Mesirov JP. Integrative Genomics Viewer (IGV): high-performance genomics data visualization and exploration. Brief Bioinform 2013;14:178-92. [Crossref] [PubMed]
- Valero C, Lee M, Hoen D, et al. Response Rates to Anti-PD-1 Immunotherapy in Microsatellite-Stable Solid Tumors With 10 or More Mutations per Megabase. JAMA Oncol 2021;7:739-43. [Crossref] [PubMed]
- Zhang D, Lu B, Liang B, et al. Interpretable deep learning survival predictive tool for small cell lung cancer. Front Oncol 2023;13:1162181. [Crossref] [PubMed]
- Zhu L, Qin J. Predictive biomarkers for immunotherapy response in extensive-stage SCLC. J Cancer Res Clin Oncol 2024;150:22. [Crossref] [PubMed]
- Paz-Ares L, Garassino MC, Chen Y, et al. Durvalumab ± Tremelimumab + Platinum-Etoposide in Extensive-Stage Small Cell Lung Cancer (CASPIAN): Outcomes by PD-L1 Expression and Tissue Tumor Mutational Burden. Clin Cancer Res 2024;30:824-35. [Crossref] [PubMed]
- Liu SV, Mok TSK, Nabet BY, et al. Clinical and molecular characterization of long-term survivors with extensive-stage small cell lung cancer treated with first-line atezolizumab plus carboplatin and etoposide. Lung Cancer 2023;186:107418. [Crossref] [PubMed]
- Liu Q, Zhang J, Guo C, et al. Proteogenomic characterization of small cell lung cancer identifies biological insights and subtype-specific therapeutic strategies. Cell 2024;187:184-203.e28. [Crossref] [PubMed]
- Zhou H, Hu Y, Luo R, et al. Multi-region exome sequencing reveals the intratumoral heterogeneity of surgically resected small cell lung cancer. Nat Commun 2021;12:5431. [Crossref] [PubMed]
- Yuan T, Wang X, Sun S, et al. Profiling of 520 Candidate Genes in 50 Surgically Treated Chinese Small Cell Lung Cancer Patients. Front Oncol 2021;11:644434. [Crossref] [PubMed]
- Zhou M, Fan J, Li Z, et al. Prognostic impact of tumor mutation burden and the mutation in KIAA1211 in small cell lung cancer. Respir Res 2019;20:248. [Crossref] [PubMed]
- Kachroo S, Shao C, Desai K, et al. Association of clinico-genomic characteristics with tumor mutational burden in small cell lung cancer patients. Future Oncol 2021;17:423-33. [Crossref] [PubMed]
- Cai W, Zhou D, Wu W, et al. MHC class II restricted neoantigen peptides predicted by clonal mutation analysis in lung adenocarcinoma patients: implications on prognostic immunological biomarker and vaccine design. BMC Genomics 2018;19:582. [Crossref] [PubMed]
- Zhu M, Zhang L, Cui H, et al. Co-Mutation of FAT3 and LRP1B in Lung Adenocarcinoma Defines a Unique Subset Correlated With the Efficacy of Immunotherapy. Front Immunol 2021;12:800951. [Crossref] [PubMed]
- Cai R, Zhu H, Liu Y, et al. To be, or not to be: the dilemma of immunotherapy for non-small cell lung cancer harboring various driver mutations. J Cancer Res Clin Oncol 2023;149:10027-40. [Crossref] [PubMed]
- Jiang Z, Zhu M, Zhang L, et al. Antitumor immunity and prognosis value elicited by FAT3 and LRP1B co-mutation in endometrial cancer. Gynecol Oncol 2024; Epub ahead of print. [Crossref]
- Liao K, Yang Q, Xu Y, et al. Identification of signature of tumor-infiltrating CD8 T lymphocytes in prognosis and immunotherapy of colon cancer by machine learning. Clin Immunol 2023;257:109811. [Crossref] [PubMed]