CT-based radiomic consensus clustering association with tumor biological behavior in clinical stage IA adenocarcinoma: a retrospective study
Highlight box
Key findings
• Consensus clusters stratified by the radiomic features (RFs) of clinical stage IA adenocarcinoma exhibited distinctions in tumor biological behavior.
What is known and what is new?
• In lung adenocarcinoma, RFs are potential non-invasive biomarkers for predicting a spectrum of heterogeneous biological behaviors, however, the relationship between RFs and the biological behavior of early-stage lung adenocarcinoma, particularly clinical stage IA adenocarcinoma, remains unclear.
• RF analysis of primary stage IA adenocarcinomas was performed and a larger number of cases were used for a more robust verification. Patients were clustered into groups based on RFs using consensus cluster analysis. Three distinct clusters with distinct tumor biological behavior were identified, which indicates that RFs can reflect the biological behavior of tumors.
What is the implication, and what should change now?
• In clinical stage IA adenocarcinoma patients, extracted RFs can be used to demonstrate biological characteristics of tumors, thus contributing to patient diagnosis and subsequent treatment.
Introduction
The widespread adoption and advocacy of low-dose chest computed tomography (CT) screening have notably increased the detection rate of early-stage lung adenocarcinoma (1). Early-stage lung adenocarcinoma displays heterogeneity with varying biological behaviors among tumors, including differences in tumor invasion, histological grade, oncogene mutation, pleural invasion, and lymph node metastasis (LNM) (2). These varied traits are pivotal for customizing treatment plans and predicting the prognosis of patients with early-stage lung adenocarcinoma.
Researchers have explored radiomics features (RFs) to study the biological behavior of lung adenocarcinoma, demonstrating their utility in diagnosing its invasiveness (3,4). In the context of lung adenocarcinoma, RFs are potential non-invasive biomarkers capable of predicting a spectrum of heterogeneous biological behaviors, including malignancy, histological subtype, invasiveness, histological grade, pleural invasion, genetic expression, and LNM (4-8). Unfortunately, the application of radiomics models in clinical settings is challenging, primarily because of the limited reproducibility and repeatability observed in radiomics studies (9,10). Additionally, other factors hinder the widespread use of radiomics in clinical practice, such as the low interpretability of RFs and the unclear association between RFs and biological tumor behavior. The conventional RFs study process emphasizes statistical concepts for feature selection, prioritizing predictive power over the biological significance of RFs. This approach, coupled with the susceptibility of various machine learning models to overfitting, has sparked a controversy in RF studies. These shortcomings contribute to a growing disparity between decision-making in routine clinical practice and the interpretation of images by RFs. This disconnection is expected to have repercussions that ultimately hinder its widespread integration into routine clinical imaging (11,12).
The association between RFs and the biological behavior of early-stage lung adenocarcinoma, particularly clinical stage IA adenocarcinoma, remains unexplored. To bridge this knowledge gap, our investigation focused on unveiling the connections between RFs and the biological behavior of tumors in clinical stage IA adenocarcinoma through consensus clustering derived from CT-based radiomics analyses of primary tumors. We present this article in accordance with the STROBE reporting checklist (available at https://tlcr.amegroups.com/article/view/10.21037/tlcr-24-283/rc).
Methods
Study participants
Our analysis encompassed cases of clinical stage IA lung adenocarcinomas that underwent surgical resection within the timeframe of May 2005 to December 2018. Exclusion criteria comprised the absence of enhanced high-resolution CT (HRCT) images or positron emission tomography CT scans within 2 weeks before surgery, clinical staging beyond IA, unavailability of clinicopathological data, utilization of preoperative therapies such as chemotherapy, radiotherapy, targeted therapy, or immunotherapy, history of previous malignancy with detectable disease within the past 5 years, fewer than 3 hilar/peripheral and 3 mediastinal lymph node stations (13), and a follow-up duration of less than 6 months without occurrence of metastasis or death. A total cohort comprising 669 patients was subject to our analysis.
The study was conducted in accordance with the Declaration of Helsinki (as revised in 2013). The retrospective nature of this investigation received approval from the Institutional Review Board of the Cancer Hospital, Chinese Academy of Medical Sciences (approval No. NCCN2021C-213), with the necessity for informed consent being waived.
Clinicopathological characteristics
Our study involved the assessment of clinical characteristics such as sex, age, and history of smoking. The classification of tumors adhered to the standards set by the International Association for Lung Cancer Grading System (14). The identification of epidermal growth factor receptor (EGFR) mutations was conducted on tumor tissue and plasma DNA samples through the utilization of either an amplification refractory mutation system or direct DNA sequencing method.
CT image acquisition, interpretation, and RF extraction
HRCT scans were conducted utilizing spiral CT scanners with 8-, 16-, or 64-channel configurations (LightSpeed Ultra, ProSpeed or Discovery ST, and LightSpeed VCT, respectively, all from GE Medical Systems). All participants underwent enhanced HRCT assessments, with intravenous contrast (60 to 80 mL) administered at rates of 2.0 to 2.5 mL/s, followed by image acquisition 25 to 30 seconds post-infusion. Parameters for HRCT imaging were set at 120 kVp and 250–350 mA, with reconstruction performed using a standard algorithm. Slice thickness ranged between 0.625 to 1.25 mm, with intervals of 0.8 to 1.0 mm.
The primary tumor’s morphological characteristics, including tumor diameter, nodule consistency [pure ground glass nodule (pGGN), part solid nodule (PSN), solid nodule (SN)], solid component size, consolidation to tumor ratio (CTR), lobar and tumor location [central (the inner third) or peripheral (the outer two-thirds of the lung fields)], were evaluated by two experienced chest CT interpreters (L.Z. and M.L.) using the Carestream GCRIS 2.1 PACS workstation (Carestream Health, Shenzhen, China). Both radiologists, each possessing over 10 years of experience, analyzed the data in consensus, blinded to all clinical and outcome details. Considering the different biological behaviors of PSNs with CTR >0.5 and PSNs with CTR ≤0.5, these two groups of PSNs were analyzed separately (15).
The primary tumor underwent manual segmentation by a thoracic radiologist (L.Z.) and was validated by another thoracic radiologist (M.L.) independently. In cases of conflicting tumor boundaries, a final agreement was reached through a group discussion. Tumor regions of interest were delineated using an open-source software (ITK-SNAP; http://www.itksnap.org/pmwiki/pmwiki.php), with lung window settings across all two-dimensional sections in the axial view. Adjustments to window and level settings were made as necessary to accurately outline nodule borders, particularly in cases where nodules were adjacent to the mediastinum or chest wall.
The extraction of RFs was conducted using Artificial Intelligence Kit software (A.K. V3.0.0. R, GE Healthcare), adhering to the standards of the Image Biomarker Standardization initiative (16). Initially, linear interpolation was employed to resample all images to a consistent voxel size of 1 mm × 1 mm × 1 mm to mitigate variations in layer thickness. Subsequently, a grayscale discretization process (bin width =25 for CT) was applied to convert continuous images into discrete values. Gaussian Laplacian and wavelet image filters were then utilized to remove mixed noise during image digitization, thereby isolating low- or high-frequency features. A total of 107 quantitative features were extracted, comprising 18 first-order features, 16 grey-level run-length matrix features, 16 grey-level size-zone matrix features, 24 grey-level co-occurrence matrix features, 14 shape [three-dimensional (3D)] features, five neighboring grey-tone difference matrix features, and 14 grey-level dependence matrix features. The calculation formula for the radiomics signatures can be found on the official documentation website (https://pyradiomics.readthedocs.io/en/latest/features.html).
Consensus clustering
Consensus clustering was employed to categorize the extracted RFs. This method is resampling-based, aiming to gauge the consensus across multiple clustering iterations and to determine the optimal number of clusters (17). We repetitively sampled 80% of the original dataset, hierarchically clustering each subsample. We then assessed how frequently each sample co-occurred with others in the same cluster to construct a consensus heat map, tracing plot, corresponding empirical cumulative distribution function curve, and chart showing shifts in the area under the curve. This enabled us to identify the most suitable number of clusters.
Follow-up strategy
Following sublobar resection or lobectomy, all patients underwent post-operative follow-up starting from the day after surgery. Survival outcomes and disease progression data were acquired through medical record review and telephone interviews conducted by trained staff members. In cases the patients or their family members were unreachable at the scheduled follow-up date, the date and survival information were censored according to the last follow-up. Recurrence-free survival (RFS) served as the primary endpoint, defined as the duration between the surgery date and the occurrence of local, regional, or metastatic relapse.
Statistical analyses
Frequency distribution and descriptive statistics were calculated for all variables. The data were presented as mean ± standard deviation in cases of normal distribution, and as median [interquartile range (IQR)] when normality assumptions were not met. To test the normality assumptions, the Kolmogorov-Smirnov test was employed. Age and tumor diameter variations across different clusters were assessed using the t-test and Wilcoxon rank-sum test for parametric and nonparametric continuous variables, and the Chi-squared test or Fisher’s exact test for categorical variables. The RFS was analyzed using the Kaplan-Meier method, and the comparison of the assigned clusters was done using the log-rank test. The hazard ratios (HRs) for metastasis or recurrence based on the assigned clusters were evaluated through Cox proportional hazards analysis. Statistical analyses were conducted by M.W.L. and Y.M.W. using SPSS software (version 25; IBM Corp., Armonk, NY, USA) and R software (version 4.1.1; The R Foundation for Statistical Computing, Vienna, Austria), with P<0.05 considered as statistically significant.
Results
Clinicopathologic characteristics
In total, 669 patients were included in this study (Figure 1). The median age was 58 years (IQR, 50–64 years), 257 patients (38.4%) were male and 412 (61.6%) were female. Most patients were non-smokers [n=498 (74.4%)]. The median tumor size was 1.6 cm (IQR, 1.1–2.2 cm). Of the 669 patients with nodules 121 (18.1%) had pGGNs, 225 (33.6%) had PSNs with CTR ≤0.5, 155 (23.2%) had PSNs with CTR >0.5, and 168 (25.1%) had SNs. In total, 149 patients (22.3%) had central-type tumors and 520 patients (77.7%) had peripheral-type tumors. Most patients had pathological stage I disease [n=469 (70.1%)]; 82 (12.3%) had stage II disease, 65 (9.7%) had stage 0 disease, and 53 (7.9%) had stage III disease. Of all patients, 129 (19.3%) had pleural involvement. Most tumors on histological rating were grade 2 [n=308 (55.1%)], followed by grade 1 [n=171 (30.6%)], and grade 3 [n=80 (14.3%)]. Among the 318 patients with EGFR gene test, 118 (37.1%) were EGFR mutation-negative and 200 (62.9%) were EGFR mutation-positive (Table 1).
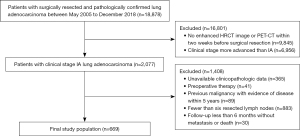
Table 1
Characteristic | Values |
---|---|
Sex | |
Male | 257 (38.4) |
Female | 412 (61.6) |
Age (years) | 58 [50–64] |
Smoking status | |
Non-smoker | 498 (74.4) |
Smoker | 171 (25.6) |
Diameter (cm) | 1.6 [1.1–2.2] |
Nodule consistency | |
pGGN | 121 (18.1) |
PSN | 380 (56.8) |
SN | 168 (25.1) |
Solid component size of PSNs (cm) | 0.4 [0.3–0.6] |
CTR | |
0 | 121 (18.1) |
0< CTR ≤0.5 | 225 (33.6) |
0.5< CTR <1.0 | 155 (23.2) |
1.0 | 168 (25.1) |
Lobar location | |
Right upper lobe | 235 (35.1) |
Right middle lobe | 55 (8.2) |
Right lower lobe | 115 (17.2) |
Left upper lobe | 175 (26.2) |
Left lower lobe | 89 (13.3) |
Tumor location | |
Central | 149 (22.3) |
Peripheral | 520 (77.7) |
Surgical procedure | |
Sublobectomy | 94 (14.1) |
Lobectomy | 575 (85.9) |
Adjuvant therapy | |
Surgery alone | 545 (81.5) |
Surgery plus adjuvant therapy | 124 (18.5) |
Pleural invasion | |
Negative | 540 (80.7) |
Positive | 129 (19.3) |
Pathological T stage# | |
Tis | 65 (9.7) |
T1a | 182 (27.2) |
T1b | 198 (29.6) |
T1c | 93 (13.9) |
T2a | 131 (19.6) |
Pathological N stage# | |
N0 | 534 (79.8) |
N1 | 82 (12.3) |
N2 | 53 (7.9) |
Pathological stage# | |
0 (Tis) | 65 (9.7) |
IA1 | 180 (26.9) |
IA2 | 155 (23.2) |
IA3 | 49 (7.3) |
IB | 85 (12.7) |
IIB | 82 (12.3) |
IIIA | 53 (7.9) |
Grading system of lung adenocarcinoma | |
1 | 171 (30.6) |
2 | 308 (55.1) |
3 | 80 (14.3) |
EGFR mutation | |
Negative | 118 (37.1) |
Positive | 200 (62.9) |
Data are presented as median [interquartile range] or n (%). Pathology slides of 110 patients cannot be reviewed and 318 patients undergo genetic testing. #, 8th staging classification. pGGN, pure ground glass nodule; PSN, part solid nodule; SN, solid nodule; CTR, consolidation to tumor ratio; EGFR, epidermal growth factor receptor.
Comparison of characteristics between different clusters
Consensus clustering was evaluated from k=2 to k=6, and k=3 was selected as the best cluster because it produced the best data split (Figure 2A). The cumulative distribution function for these clusters was assessed using various consensus indices, with k=3 being the most stable across all consensus indices (Figure 2B). There were 310 patients (46.3%) in Cluster 1, 324 (48.4%) in Cluster 2, and 35 (5.2%) in Cluster 3. The representative CT and pathology images for each cluster are shown in (Figure 3). A total of 45 cases in Cluster 1, 157 in Cluster 2, and 17 in Cluster 3 increased in stages. The reason for the pathologic upstaging was pleural invasion, occult lymph node metastasis (ONM), and both pleural invasion and ONM (Table S1). The highest percentages of SNs [119 of 324 (36.7%), P<0.001], central tumors [91 of 324 (28.1%), P=0.002], pleural invasion [87 of 324 (26.9%), P<0.001], ONM [106 of 324 (32.7%), P<0.001], and EGFR mutation-positive [122 of 176 (69.3%), P=0.03], were found in Cluster 2. Compared with other Clusters [Cluster 1, 1.1 cm (IQR, 0.8–1.4 cm), Cluster 3, 1.6 cm (IQR, 1.4–1.8 cm)], Cluster 2 had a bigger median nodule diameter [Cluster 2, 2.1 cm (IQR, 1.7–2.5 cm), P<0.001]. Cluster 3 exhibited the highest rate of histological grade 3 [8 of 34 (23.5%), P<0.001], compared with the other clusters [Cluster 1, 11 of 248 (4.4%); Cluster 2, 61 of 277 (22.0%)]. Cluster 1 was associated with pGGN [184 of 310 (59.4%), P<0.001], no pleural invasion [276 of 310 (89.0%), P<0.001], histological grade 1 [114 of 248 (46.0%), P<0.001], and no ONM [292 of 310 (94.2%), P<0.001] (Figure 4, Table 2). There is no statistically significant difference in the distribution of part-solid nodules based on CTR (≤0.5 vs. >0.5) within the cluster (Table S2).
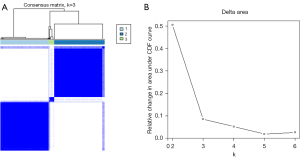
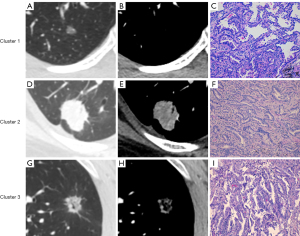
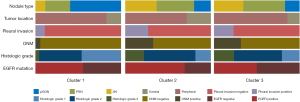
Table 2
Characteristic | Cluster 1 (n=310) | Cluster 2 (n=324) | Cluster 3 (n=35) | P value |
---|---|---|---|---|
Sex | <0.001 | |||
Male | 97 (31.3) | 150 (46.3) | 10 (28.6) | |
Female | 213 (68.7) | 174 (53.7) | 25 (71.4) | |
Age (years) | 55 [48–61] | 59 [52–66] | 58 [52–67] | <0.001 |
Smoking status | 0.002 | |||
Non-smoker | 249 (80.3) | 221 (68.2) | 28 (80.0) | |
Smoker | 61 (19.7) | 103 (31.8) | 7 (20.0) | |
Nodule consistency | <0.001 | |||
pGGN | 184 (59.4) | 63 (19.4) | 6 (17.1) | |
PSN | 90 (29.0) | 142 (43.8) | 17 (48.6) | |
SN | 36 (11.6) | 119 (36.7) | 12 (34.3) | |
Diameter (cm) | 1.1 [0.8–1.4] | 2.1 [1.7–2.5] | 1.6 [1.4–1.8] | <0.001 |
CTR | <0.001 | |||
0 | 99 (31.9) | 22 (6.8) | 0 | |
0< CTR ≤0.5 | 106 (34.2) | 107 (33.0) | 12 (34.3) | |
0.5< CTR <1.0 | 68 (21.9) | 76 (23.5) | 11 (31.4) | |
1.0 | 37 (11.9) | 119 (36.7) | 12 (34.3) | |
Tumor location | 0.002 | |||
Central | 53 (17.1) | 91 (28.1) | 5 (14.3) | |
Peripheral | 257 (82.9) | 233 (71.9) | 30 (85.7) | |
Pleural invasion | <0.001 | |||
Negative | 276 (89.0) | 237 (73.1) | 27 (77.1) | |
Positive | 34 (11.0) | 87 (26.9) | 8 (22.9) | |
Histologic grade | <0.001 | |||
1 | 114 (46.0) | 50 (18.1) | 7 (20.6) | |
2 | 123 (49.6) | 166 (59.9) | 19 (55.9) | |
3 | 11 (4.4) | 61 (22.0) | 8 (23.5) | |
ONM | <0.001 | |||
Negative | 292 (94.2) | 218 (67.3) | 24 (68.6) | |
Positive | 18 (5.8) | 106 (32.7) | 11 (31.4) | |
EGFR mutation | 0.03 | |||
Negative | 58 (44.6) | 54 (30.7) | 6 (50.0) | |
Positive | 72 (55.4) | 122 (69.3) | 6 (50.0) |
Data are presented as median [interquartile range] or n (%). Pathology slides of 110 patients could not be reviewed, distributed as follows: 62 in Cluster 1, 47 in Cluster 2, and 1 in Cluster 3. 318 patients underwent genetic testing, distributed as follows: 130 in Cluster 1, 176 in Cluster 2, 12 in Cluster 3. pGGN, pure ground glass nodule; PSN, part solid nodule; SN, solid nodule; CTR, consolidation to tumor ratio; ONM, occult lymph node metastasis; EGFR, epidermal growth factor receptor.
Survival outcomes
During a median (IQR) follow-up period of 62 [55–72] months, 12 (3.9%) cases of metastasis or recurrence occurred in Cluster 1, 62 (19.1%) in Cluster 2, and 1 (2.9%) in Cluster 3. There were significant differences in metastasis and recurrence among the three clusters (P<0.001) (Figure 5). Cluster 2 was associated with the highest risk, with an HR of 5.294 (95% confidence interval, 2.853–9.825) compared with Cluster 1 (Table 3).
Table 3
Outcome | Cluster 1 (n=310) | Cluster 2 (n=324) | Cluster 3 (n=35) | P value |
---|---|---|---|---|
Metastasis or recurrence | <0.001 | |||
No | 298 (96.1) | 262 (80.9) | 34 (97.1) | |
Yes | 12 (3.9) | 62 (19.1) | 1 (2.9) | |
HR* | Reference | 5.294 (2.853–9.825) | 0.670 (0.087–5.162) |
Unless otherwise indicated, data are presented as n (%). *, data in parentheses are presented as 95% confidence interval. Hazard ratio refers to the risk of metastasis or recurrence in each cluster compared to a reference group. HR, hazard ratio.
Discussion
Instead of using a machine learning model to predict certain biological behaviors, as in previous radiomics studies, we focused on whether radiomics and biological behavior are truly related. In this study, cluster analysis of RFs was conducted to establish different groups and compare the differences in the biological behaviors of tumors in each group to positively identify the correlation between RFs and the biological behaviors of tumors, filling the gap in the current research. We classified the extracted RFs into three clusters using consensus clustering and demonstrated that radiomics can reflect the biological behavior of tumors.
Radiomics contains redundant and irrelevant information as high-dimensional data. The feature screening process not only screens out the features that truly reflect clinical information but may also randomly screen out irrelevant or redundant features (18,19). Under different experimental conditions, even if the same true features can be obtained, the same irrelevant and redundant features cannot be screened, which may be one of the reasons for the lack of reproducibility and repeatability of RFs. Moreover, due to the low interpretability and ambiguous correlation between RFs and clinical outcomes, it is impossible to determine which features are required. Clustering is a method for organizing objects into distinct groups, where objects within the same cluster exhibit notable similarities, while those in separate clusters demonstrate considerable dissimilarities (20). In our study, we used a standardized process to extract RFs and clustered these features. Four distinct clusters are identified. This does not imply that all true features are included in the same cluster. Rather, true, irrelevant, and redundant features with certain similarities are clustered together. We believe that a cluster of RFs is more representative than a single or a few features. The results of our study are also validated by those of previous studies, which differ in that we focused on clinical stage IA adenocarcinoma and included a larger number of cases for a more robust verification (21,22).
In lung adenocarcinoma, invasiveness is closely related to its subtype. Tumor invasiveness increases according to the subtypes of adenocarcinoma in situ, from minimally invasive to invasive adenocarcinoma (23), and the clinical strategy varies from follow-up to surgery. On CT images, ground-glass opacity represents components of the mural growth of tumor cells, and radiomics can further distinguish the subtype and invasiveness (3,24-27). Besides tumor invasiveness, adjacent visceral pleural invasion can upgrade the tumor to the T2 stage regardless of the tumor size (28). However, pleural indentation on CT images cannot accurately predict visceral pleural invasion, with an accuracy of 28.3% to 70.8% depending on the different patterns of the pleural tag sign (29). The joint prediction model containing CT morphological and RFs can significantly improve the accuracy of prediction, with an area under the curve of 0.894 in clinical stage IA lung cancer (30). The biological behaviors of tumors are also related to oncological outcomes. In our study, we demonstrated that some specific RFs are related to ground-glass opacity and pleural invasion.
The most common metastasis of early cT1N0M0 lung adenocarcinoma is ONM, which necessitates lobectomy with mediastinal lymph node dissection as the standard surgical procedure (31). The rate of ONM in clinical N0 stage non-small cell lung cancer is only 23.1% (32), indicating that a large number of patients underwent an unnecessarily aggressive surgical approach, which increased the risk of tissue damage and longer postoperative recovery time (33,34). Moreover, the accuracy of ONM diagnosis affects the implementation of less invasive treatments, such as stereotactic ablative radiotherapy or segmentectomy, in patients with early-stage non-small cell lung cancer patients (35-37). Studies have evaluated the relationship between CT features of the primary tumor and ONM, such as tumor size, solid component size, central tumor location, and pleural indentation, which are all risk predictors of LNM (38-41). Haque et al. (41) found that the probability of ONM increased by 10–14% when tumor size increased by 1 cm. Although different guidelines define central tumors differently (42-44), Casal et al. (38) found that identifying the center as the inner 1/3 or 2/3 was associated with upstaging from cN0 to any N. This is consistent with our findings, in which Cluster 2 had the highest percentage of ONM [106 of 324 (32.7%), P<0.001], and tumors in this cluster were more likely to be large (P<0.001), radiologically solid adenocarcinomas (P<0.001) and to have pleural involvement (P<0.001).
Grade 3 tumors are associated with aggressive features, including ONM and visceral pleural invasion (45). Histological grade 3 tumors are mainly adenocarcinomas containing >20% solid and/or micropapillary components that are confirmed to be associated with ONM (8,46,47). However, in our study, we observed that the percentage of grade 3 tumors in Cluster 3 [8 of 34 (23.5%)] surpassed that in Cluster 2 [61 of 277 (22.0%)]. This discrepancy may be attributed to the extended time period from 2005 to 2018, and that the pathological slides of 110 patients could not be reviewed. Some slides were not located, and others were poorly preserved, making them inaccessible for retrospective examination.
Our study identified that the high-risk cluster, Cluster 2, is significantly more likely to have larger median nodule diameter, pleural metastasis, ONM, EGFR mutation positivity, and poor prognosis. These findings have important clinical implications for treatment strategies. Patients in the high-risk cluster should be considered for thorough lymph node dissection due to the high likelihood of ONM. This extensive surgical intervention could help manage the higher tumor burden and improve staging accuracy, potentially enhancing patient outcomes. The poor prognosis associated with the high-risk cluster underscores the need for intensified adjuvant therapy. More aggressive preoperative or postoperative adjuvant therapy regimens might be necessary to reduce recurrence risk and improve survival time. Our findings support a more aggressive postoperative treatment regimen for high-risk patients. Future research should refine these predictive models to ensure they integrate both statistical robustness and biological significance, improving their alignment with clinical practice.
The limitations of our study include the unavailability of certain pathological sections stemming from the inclusion of cases with a follow-up time exceeding 5 years, and in some cases, surpassing 10 years. This limitation may have impacted the results of the cluster analysis. In addition, almost half of the patients did not undergo genetic testing, highlighting the need for further studies.
Conclusions
In summary, using a new cluster analysis method, this study validated that RFs could reflect tumor biological behaviors in clinical stage IA adenocarcinomas.
Acknowledgments
Funding: This work was supported by
Footnote
Reporting Checklist: The authors have completed the STROBE reporting checklist. Available at https://tlcr.amegroups.com/article/view/10.21037/tlcr-24-283/rc
Data Sharing Statement: Available at https://tlcr.amegroups.com/article/view/10.21037/tlcr-24-283/dss
Peer Review File: Available at https://tlcr.amegroups.com/article/view/10.21037/tlcr-24-283/prf
Conflicts of Interest: All authors have completed the ICMJE uniform disclosure form (available at https://tlcr.amegroups.com/article/view/10.21037/tlcr-24-283/coif). The authors have no conflicts of interest to declare.
Ethical Statement: The authors are accountable for all aspects of the work in ensuring that questions related to the accuracy or integrity of any part of the work are appropriately investigated and resolved. The study was conducted in accordance with the Declaration of Helsinki (as revised in 2013). The retrospective nature of this investigation received approval from the Institutional Review Board of the Cancer Hospital, Chinese Academy of Medical Sciences (approval No. NCCN2021C-213), with the necessity for informed consent being waived.
Open Access Statement: This is an Open Access article distributed in accordance with the Creative Commons Attribution-NonCommercial-NoDerivs 4.0 International License (CC BY-NC-ND 4.0), which permits the non-commercial replication and distribution of the article with the strict proviso that no changes or edits are made and the original work is properly cited (including links to both the formal publication through the relevant DOI and the license). See: https://creativecommons.org/licenses/by-nc-nd/4.0/.
References
- National Lung Screening Trial Research Team. Reduced lung-cancer mortality with low-dose computed tomographic screening. N Engl J Med 2011;365:395-409. [Crossref] [PubMed]
- Tavernari D, Battistello E, Dheilly E, et al. Nongenetic Evolution Drives Lung Adenocarcinoma Spatial Heterogeneity and Progression. Cancer Discov 2021;11:1490-507. [Crossref] [PubMed]
- Zhu M, Yang Z, Wang M, et al. A computerized tomography-based radiomic model for assessing the invasiveness of lung adenocarcinoma manifesting as ground-glass opacity nodules. Respir Res 2022;23:96. [Crossref] [PubMed]
- Wu G, Woodruff HC, Shen J, et al. Diagnosis of Invasive Lung Adenocarcinoma Based on Chest CT Radiomic Features of Part-Solid Pulmonary Nodules: A Multicenter Study. Radiology 2020;297:451-8. [Crossref] [PubMed]
- Cheng B, Deng H, Zhao Y, et al. Predicting EGFR mutation status in lung adenocarcinoma presenting as ground-glass opacity: utilizing radiomics model in clinical translation. Eur Radiol 2022;32:5869-79. [Crossref] [PubMed]
- Dong H, Yin L, Chen L, et al. Establishment and validation of a radiological-radiomics model for predicting high-grade patterns of lung adenocarcinoma less than or equal to 3 cm. Front Oncol 2022;12:964322. [Crossref] [PubMed]
- Zha X, Liu Y, Ping X, et al. A Nomogram Combined Radiomics and Clinical Features as Imaging Biomarkers for Prediction of Visceral Pleural Invasion in Lung Adenocarcinoma. Front Oncol 2022;12:876264. [Crossref] [PubMed]
- Zhang L, Lv L, Li L, et al. Radiomics Signature to Predict Prognosis in Early-Stage Lung Adenocarcinoma (≤3 cm) Patients with No Lymph Node Metastasis. Diagnostics (Basel) 2022;12:1907. [Crossref] [PubMed]
- Berenguer R, Pastor-Juan MDR, Canales-Vázquez J, et al. Radiomics of CT Features May Be Nonreproducible and Redundant: Influence of CT Acquisition Parameters. Radiology 2018;288:407-15. [Crossref] [PubMed]
- Peng X, Yang S, Zhou L, et al. Repeatability and Reproducibility of Computed Tomography Radiomics for Pulmonary Nodules: A Multicenter Phantom Study. Invest Radiol 2022;57:242-53. [Crossref] [PubMed]
- Tomaszewski MR, Gillies RJ. The Biological Meaning of Radiomic Features. Radiology 2021;298:505-16. [Crossref] [PubMed]
- Kurz FT, Schlemmer HP. Imaging in translational cancer research. Cancer Biol Med 2022;19:1565-85. [Crossref] [PubMed]
- Rami-Porta R. editor. IASLC Staging Handbook in Thoracic Oncology, 2nd edn. North Fort Myers, FL: Editorial Rx Press; 2016.
- Moreira AL, Ocampo PSS, Xia Y, et al. A Grading System for Invasive Pulmonary Adenocarcinoma: A Proposal From the International Association for the Study of Lung Cancer Pathology Committee. J Thorac Oncol 2020;15:1599-610. [Crossref] [PubMed]
- Nakao M, Oikado K, Sato Y, et al. Prognostic Stratification According to Size and Dominance of Radiologic Solid Component in Clinical Stage IA Lung Adenocarcinoma. JTO Clin Res Rep 2022;3:100279. [Crossref] [PubMed]
- Zwanenburg A, Vallières M, Abdalah MA, et al. The Image Biomarker Standardization Initiative: Standardized Quantitative Radiomics for High-Throughput Image-based Phenotyping. Radiology 2020;295:328-38. [Crossref] [PubMed]
- Monti S, Tamayo P, Mesirov J, et al. Consensus Clustering: A Resampling-Based Method for Class Discovery and Visualization of Gene Expression Microarray Data. Machine Learning 2003;52:91-118. [Crossref]
- Maciejewski H. editor. Risk of Selection of Irrelevant Features from High-Dimensional Data with Small Sample Size. Stochastic Models, Statistics and Their Applications; 2015; Cham: Springer International Publishing; 2015.
- Chung IF, Chen YC, Pal NR. Feature Selection With Controlled Redundancy in a Fuzzy Rule Based Framework. IEEE Transactions on Fuzzy Systems 2018;26:734-48. [Crossref]
- Wang H, Wei W, Yang J, et al., editors. Clustering by pattern similarity in large data sets. International Conference on Management of Data; 2002.
- Lin P, Lin YQ, Gao RZ, et al. Integrative radiomics and transcriptomics analyses reveal subtype characterization of non-small cell lung cancer. Eur Radiol 2023;33:6414-25. [Crossref] [PubMed]
- Perez-Johnston R, Araujo-Filho JA, Connolly JG, et al. CT-based Radiogenomic Analysis of Clinical Stage I Lung Adenocarcinoma with Histopathologic Features and Oncologic Outcomes. Radiology 2022;303:664-72. [PubMed]
- Travis WD, Brambilla E, Noguchi M, et al. International association for the study of lung cancer/american thoracic society/european respiratory society international multidisciplinary classification of lung adenocarcinoma. J Thorac Oncol 2011;6:244-85. [Crossref] [PubMed]
- Kao TN, Hsieh MS, Chen LW, et al. CT-Based Radiomic Analysis for Preoperative Prediction of Tumor Invasiveness in Lung Adenocarcinoma Presenting as Pure Ground-Glass Nodule. Cancers (Basel) 2022;14:5888. [Crossref] [PubMed]
- Wu L, Gao C, Ye J, et al. The value of various peritumoral radiomic features in differentiating the invasiveness of adenocarcinoma manifesting as ground-glass nodules. Eur Radiol 2021;31:9030-7. [Crossref] [PubMed]
- Jiang Y, Che S, Ma S, et al. Radiomic signature based on CT imaging to distinguish invasive adenocarcinoma from minimally invasive adenocarcinoma in pure ground-glass nodules with pleural contact. Cancer Imaging 2021;21:1. [Crossref] [PubMed]
- Wu L, Gao C, Xiang P, et al. CT-Imaging Based Analysis of Invasive Lung Adenocarcinoma Presenting as Ground Glass Nodules Using Peri- and Intra-nodular Radiomic Features. Front Oncol 2020;10:838. [Crossref] [PubMed]
- Travis WD, Asamura H, Bankier AA, et al. The IASLC Lung Cancer Staging Project: Proposals for Coding T Categories for Subsolid Nodules and Assessment of Tumor Size in Part-Solid Tumors in the Forthcoming Eighth Edition of the TNM Classification of Lung Cancer. J Thorac Oncol 2016;11:1204-23.
- Hsu JS, Han IT, Tsai TH, et al. Pleural Tags on CT Scans to Predict Visceral Pleural Invasion of Non-Small Cell Lung Cancer That Does Not Abut the Pleura. Radiology 2016;279:590-6. [Crossref] [PubMed]
- Wei SH, Zhang JM, Shi B, et al. The value of CT radiomics features to predict visceral pleural invasion in ≤3 cm peripheral type early non-small cell lung cancer. J Xray Sci Technol 2022;30:1115-26. [Crossref] [PubMed]
- Ginsberg RJ, Rubinstein LV. Randomized trial of lobectomy versus limited resection for T1 N0 non-small cell lung cancer. Lung Cancer Study Group. Ann Thorac Surg 1995;60:615-22; discussion 622-3. [Crossref] [PubMed]
- Beyaz F, Verhoeven RLJ, Schuurbiers OCJ, et al. Occult lymph node metastases in clinical N0/N1 NSCLC; A single center in-depth analysis. Lung Cancer 2020;150:186-94. [Crossref] [PubMed]
- Darling GE, Allen MS, Decker PA, et al. Randomized trial of mediastinal lymph node sampling versus complete lymphadenectomy during pulmonary resection in the patient with N0 or N1 (less than hilar) non-small cell carcinoma: results of the American College of Surgery Oncology Group Z0030 Trial. J Thorac Cardiovasc Surg 2011;141:662-70. [Crossref] [PubMed]
- Shayani J, Flores RM, Hakami A. Mediastinal lymph node dissection: the debate is not resolved. J Thorac Dis 2017;9:1848-50. [Crossref] [PubMed]
- Chang JY, Mehran RJ, Feng L, et al. Stereotactic ablative radiotherapy for operable stage I non-small-cell lung cancer (revised STARS): long-term results of a single-arm, prospective trial with prespecified comparison to surgery. Lancet Oncol 2021;22:1448-57. [Crossref] [PubMed]
- Saji H, Okada M, Tsuboi M, et al. Segmentectomy versus lobectomy in small-sized peripheral non-small-cell lung cancer (JCOG0802/WJOG4607L): a multicentre, open-label, phase 3, randomised, controlled, non-inferiority trial. Lancet 2022;399:1607-17. [Crossref] [PubMed]
- Suzuki K, Saji H, Aokage K, et al. Comparison of pulmonary segmentectomy and lobectomy: Safety results of a randomized trial. J Thorac Cardiovasc Surg 2019;158:895-907. [Crossref] [PubMed]
- Casal RF, Sepesi B, Sagar AS, et al. Centrally located lung cancer and risk of occult nodal disease: an objective evaluation of multiple definitions of tumour centrality with dedicated imaging software. Eur Respir J 2019;53:1802220. [Crossref] [PubMed]
- Song CY, Kimura D, Sakai T, et al. Novel approach for predicting occult lymph node metastasis in peripheral clinical stage I lung adenocarcinoma. J Thorac Dis 2019;11:1410-20. [Crossref] [PubMed]
- He XQ, Luo TY, Li X, et al. Clinicopathological and computed tomographic features associated with occult lymph node metastasis in patients with peripheral solid non-small cell lung cancer. Eur J Radiol 2021;144:109981. [Crossref] [PubMed]
- Haque W, Singh A, Park HS, et al. Quantifying the rate and predictors of occult lymph node involvement in patients with clinically node-negative non-small cell lung cancer. Acta Oncol 2022;61:403-8. [Crossref] [PubMed]
- Silvestri GA, Gonzalez AV, Jantz MA, et al. Methods for staging non-small cell lung cancer: Diagnosis and management of lung cancer, 3rd ed: American College of Chest Physicians evidence-based clinical practice guidelines. Chest 2013;143:e211S-50S.
- De Leyn P, Dooms C, Kuzdzal J, et al. Revised ESTS guidelines for preoperative mediastinal lymph node staging for non-small-cell lung cancer. Eur J Cardiothorac Surg 2014;45:787-98. [Crossref] [PubMed]
- Ettinger DS, Wood DE, Aisner DL, et al. Non-Small Cell Lung Cancer, Version 3.2022, NCCN Clinical Practice Guidelines in Oncology. J Natl Compr Canc Netw 2022;20:497-530. [Crossref] [PubMed]
- Deng C, Zheng Q, Zhang Y, et al. Validation of the Novel International Association for the Study of Lung Cancer Grading System for Invasive Pulmonary Adenocarcinoma and Association With Common Driver Mutations. J Thorac Oncol 2021;16:1684-93. [Crossref] [PubMed]
- Moon Y, Kim KS, Lee KY, et al. Clinicopathologic Factors Associated With Occult Lymph Node Metastasis in Patients With Clinically Diagnosed N0 Lung Adenocarcinoma. Ann Thorac Surg 2016;101:1928-35. [Crossref] [PubMed]
- Dezube AR, Mazzola E, Deeb A, et al. Mandatory Nodal Evaluation During Resection of Clinical T1a Non-Small Cell Lung Cancers. Ann Thorac Surg 2022;113:1583-90. [Crossref] [PubMed]