Assessing the transportability of radiomic models for lung cancer diagnosis: commercial vs. open-source feature extractors
Highlight box
Key findings
• Radiomic models using purportedly equivalent features from open-source and commercial platforms do not confer equivalent performances.
• Radiomic models from different platforms can provide equivalent results when building independent models with full flexibility in feature selection maximizing prediction accuracy.
• Open-source radiomic platform can perform equally to commercial extractor for the analysis of lung cancer in indeterminate pulmonary nodules.
What is known and what is new?
• Many radiomic feature extractors have shown promise in improving malignancy risk stratification.
• Our study gives insight on the transportability of radiomic models between different radiomic feature extractor platforms.
What is the implication, and what should change now?
• More efforts are necessary to improve transportability of models between radiomic platforms to allow for direct comparison of platform performances and for easier research collaboration.
• Development and usage of easily accessible, open-source radiomic tools should be encouraged.
Introduction
Lung cancer remains the most common and deadliest malignancy in the United States (US) and globally with an estimated 236,000 new cases and 132,000 annual deaths in the US (1). Lung cancer screening has been shown to reduce lung cancer mortality but there are a significant number of false positive scans (2-4). With the recently updated lung cancer screening eligibility guidelines, over 14 million individuals in the US are screening eligible and the burden of indeterminate pulmonary nodules (IPNs) with unclear malignancy potential will continue to increase (5-7).
IPNs are nodules that are up to 30 mm in size without features suggestive of benign etiology or metastatic cancer (8). In addition to screen detected IPNs, there are also nodules found incidentally on computed tomography (CT) scans, taken for unrelated indications, with an estimated 1.57 million nodules identified annually in the US (9). Professional societies such as the American College of Radiology and British Thoracic Society (BTS) have published guidelines for screen and incidentally detected lung nodules with recommendations for management strategies based on qualitative and quantitative estimates of malignancy probability (8,10-13). Using clinical variables and radiographic characteristics, nodules’ probability of malignancy is estimated by one of the available clinical risk calculators, of which the Mayo Clinic model is the most widely validated. However, using the clinical risk prediction models have shortcomings stemming from inconsistent agreement between radiologists’ interpretation of variables to patients’ estimates of factors such as family and smoking history. Furthermore, patients with nodules in the intermediate risk group (10–70% risk of malignancy) are associated with a very broad range of risk profiles. Following BTS recommendations, patients with intermediate risk group IPNs will undergo positron emission tomography (PET)/CT imaging, which has significant limitations due to suboptimal specificity, or will undergo invasive biopsies on an unacceptably high number of benign diagnoses (14-16).
One strategy to address these challenges has been the development of CT based imaging biomarkers in radiomics, which is a process of extracting and analyzing quantitative image features of a nodule otherwise imperceptible to the human observer with the goal of better characterizing the phenotype of the nodule (17). Numerous radiomic models have emerged with a great variability in image or region of interest (ROI) acquisition, features extraction, and statistical modeling. The most assessable are conventional radiomic platforms, which produce quantitative data from extraction of radiomic features of a pulmonary nodule. The most informative features are then selected to build a statistical model to predict the outcome of interest, which is the probability of malignancy in our case. HealthMyne (Madison, WI, USA) and PyRadiomics are two of the most prominent conventional radiomic platforms; the former is a commercial product while the latter is open-source. We previously published and validated a radiomic based model using HealthMyne features, which were selected by using least absolute shrinkage and selection operator (LASSO) regression, to diagnose lung cancer in IPNs (18). We set out to compare HealthMyne with PyRadiomics, to determine if the models derived by using HealthMyne to extract features could be transported to features extracted by PyRadiomics. A comparison in diagnostic accuracies between the two similar platforms is of utmost interest for the better understanding and optimization of radiomic feature development and selection in addition to reproducibility and validation of radiomic research. Furthermore, there are many benefits of having a viable open-source radiomic platform, such as broader accessibility, lower barriers to collaborations and clinical implementation, and higher likelihood of permanence.
While both platforms use multifeatured quantitative radiomics models to analyze pulmonary lesions, the radiomic features from each platform are developed independently. Even nominally identical features from the separate platforms can produce differing diagnostic accuracy, and significant efforts have been underway to standardize features, with image biomarker standardization initiative (IBSI) being the most notable (19). We performed a prospectively collected and retrospectively blinded study evaluating and comparing the diagnostic accuracies of the open-source and commercial radiomic platforms. We do this by comparing three newly developed open-source models to the validated commercial radiomic model. We present this article in accordance with the STARD reporting checklist (available at https://tlcr.amegroups.com/article/view/10.21037/tlcr-24-281/rc).
Methods
Patient selection
For this study, we used the same cohort used to train and validate the HealthMyne model in our previously published work (18), which includes four independent case-control patient populations. Prior article dealt with the development of the HealthMyne model whereas this manuscript deals with the development of new radiomic models using PyRadiomics, an open-source radiomic feature extractor. The training cohort consisted of patients (N=161) from Vanderbilt University Medical Center (VUMC) and Tennessee Valley Veterans Affair Hospital who consented to research between 2003 and 2017. Independent external cohorts include patients from the Detection of Early Cancer Among Military Personnel (DECAMP) (N=94, 2013–2017) from 12 clinical centers (20), University of Pittsburgh Medical Center (UPMC) (N=98, 2006–2015), and the University of Colorado Denver Hospital and Rocky Mountain Regional Veterans Affair Medical Center (UC Denver) (N=86, 2010–2018). The subjects included in this study were found to have IPNs between 6 and 30 mm in the largest axial diameter, CT chest scans with 3 mm and thinner slice thickness, and a definitive diagnosis determined by biopsy proven cancer or benign, or 2-year longitudinal follow up imaging showing no signs of growth for benign nodules. The study was conducted in accordance with the Declaration of Helsinki (as revised in 2013). The study was approved by Internal Review Board at Vanderbilt University (study #090781) and informed consent was obtained from all individual participants.
Radiomic model
IPNs were identified by a board-certified radiologist and then segmented using HealthMyne’s semi-automated segmentation tool. The same segmentation masks were then used for quantitative feature extraction using each radiomic platform’s feature extraction tools. For HealthMyne, the commercial platform, we used our previously published and validated model (ComRad) which was developed by using LASSO regression method with L1 penalty to select for the most informative features when training on the VUMC cohort (18).
Using features from PyRadiomics, the open-source platform, we developed three methodologically different radiomic (OpenRad) models. First, we selected open-source features independently from commercial features by dimension reduction through the same LASSO regression method as was used to create the ComRad model. The other two model building approaches were based on finding open-source platform features that most closely resemble to the features from our model from the published, commercial platform feature model (ComRad). The first of these approaches tried to match open-source to those ComRad features based upon nomenclature standards. When there were no exact matches based on IBSI standards, the most closely related features based on nomenclature were selected by expert opinion. Experts included three methodological experts as well as three lung cancer clinical specialists, whom all have extensive experiences in developing radiomic models. They all met during roundtable discussions to reach consensus regarding the selection of OpenRad features that did not have a direct nominal match with ComRad features, which consisted of 3 of the 11 total OpenRad features selected. The second method matched features solely on those with the highest Spearman’s correlation (Figures S1-S6 for models’ feature selection).
Statistical analysis
The three OpenRad models were all first trained on the VUMC cohort and then tested on the three external cohorts. The diagnostic accuracy of the risk prediction models was assessed by evaluating area under the curve (AUC) of the receiver operator characteristic (ROC) with 95% confidence interval (CI) for each radiomic platform. We also compared the three OpenRad models with ComRad by performing McNemar’s test at Youden’s cutoff in the validation cohorts. We also directly compared AUCs of models by bootstrap. Once we found the most comparable and best performing OpenRad model, we combined Mayo model scores to each of the radiomic models making integrated clinical radiomic (ClinRad) models. The Mayo model scores are based on patient age, smoking history, history of extra-thoracic cancer equal or greater than 5 years prior, nodule size, nodule location, and spiculation.
We then pooled the four cohorts (N=439) and fit the models and adjusted the prevalence of disease to 0.33 using Bayes offset. This value was chosen because of model’s intended use is in a population with an estimated prevalence of cancer at 33% in the real-world setting. We obtained AUCs of the ClinRad models on the full cohort. We then internally cross-validated the discrimination and calibration performance of each model by using 200 repeated 3-fold splitting (2/3rd of the combined cohort as the training set and 1/3rd of cohort as a test set). For each of the 200 repetitions, the radiomic based model and baseline Mayo were fitted to the training set and then calibrated to prevalence of 0.33. For each split, 500 bootstrap sampling of size 100 from 1/3 of cohort, each with approximately 0.33 prevalence, were taken from the test set to evaluate the models’ likely performance on test sample of nodules representing a similar patient population (Figures S1-S6 for model coefficients).
Reclassification
Mayo risk scores less than 0.1 considered to be low risk and above 0.7 considered high risk, and 0.1–0.7 to be intermediate risk for probability of cancer in accordance with the BTS guidelines. We used the Mayo model risk classification as a baseline estimate reference. Patients were then placed in “posttest” risk classification using the same cutoffs and their integrated model risk score. The bias-corrected clinical net reclassification index (cNRI) was then calculated for malignant cases and benign control subjects separately by comparing the integrated model (radiomic + Mayo) with the baseline Mayo classifications (21). This method allows for the utility evaluation of our model on the reclassifying IPNs into either high risk or low risk categories compared to Mayo model. The 95% CI of cNRIs for benign and malignant IPNs were estimated using 200 repeated 3-fold splitting samples of the data.
Results
A schema of the study approach is presented in Figure 1.

Study population
Four cohorts were assembled from VUMC and the Tennessee Valley VA healthcare Nashville Campus with 161 patients, UPMC with 98, the DECAMP consortium with 94 patients, and UC Denver with 86. The VUMC cohort had the highest percentage of malignancy with 71% of cohort, while the external cohorts had malignancy ranging from 41–55%. Majority of IPNs (67.7%) from cohorts were stratified into the intermediate risk group based on Mayo score (Table 1).
Table 1
Characteristics | VUMC (N=161) | UPMC (N=98) | DECAMP (N=94) | Denver (N=86) | |||||||
---|---|---|---|---|---|---|---|---|---|---|---|
Benign (n=46) | Cancer (n=115) | Benign (n=58) | Cancer (n=40) | Benign (n=47) | Cancer (n=47) | Benign (n=39) | Cancer (n=47) | ||||
Age, years | 63 [55–69] | 70 [63–76] | 68 [64–74] | 68 [62–75] | 64 [61–71] | 68 [63–73] | 66 [62–72] | 66 [63–70] | |||
Current/former smoker | 39 (84.8) | 109 (94.8) | 58 (100.0) | 40 (100.0) | 47 (100.0) | 47 (100.0) | 30 (76.9) | 38 (80.9) | |||
Pack-years | 28 [11–49] | 45 [30–71] | 47 [36–69] | 40 [31–58] | 40 [30–54] | 45 [36–60] | 32 [1–50] | 39 [16–53] | |||
Previous cancer | 11 (23.9) | 43 (37.4) | 2 (3.4) | 0 | 22 (46.8) | 22 (46.8) | 5 (12.8) | 6 (12.8) | |||
Located in upper lobe | 21 (45.7) | 67 (58.3) | 25 (43.1) | 29 (72.5) | 28 (59.6) | 26 (55.3) | 23 (59.0) | 37 (78.7) | |||
Size, mm | 13 [8.1–18.8] | 20 [14.5–23.1] | 11.2 [7.8–13.5] | 20.3 [15.9–25.8] | 11 [8–14] | 15 [11.2–19.5] | 13.1 [11.1–18.7] | 19.1 [14.8–23.9] | |||
Spiculated | 12 (26.1) | 48 (41.7) | 2 (3.4) | 11 (27.5) | 25 (53.2) | 21 (44.7) | 8 (20.5) | 18 (38.3) | |||
Sex, male | 27 (58.7) | 67 (58.3) | 36 (62.1) | 21 (52.5) | 35 (74.5) | 37 (78.7) | 26 (66.7) | 32 (68.1) | |||
BMI, kg/m2 | 28 [23–33] | 27 [23–31] | 29 [26–32] | 27 [23–30] | 25 [22–28] | 26 [23–30] | 28 [26–32] | 28 [24–31] | |||
Mayo model risk | 28.4 [15.3–45.9] | 63.3 [37.4–79.7] | 16 [10–32] | 51 [32–74] | 42 [25–69] | 51 [35–72] | 27 [17–54] | 51 [25–77] | |||
High risk: >70% | 5 (10.9) | 43 (37.4) | 0 | 12 (30.0) | 12 (25.5) | 13 (27.7) | 3 (7.7) | 14 (29.8) | |||
Intermediate risk: 10–70% | 32 (69.6) | 69 (60.0) | 43 (74.1) | 27 (67.5) | 33 (70.2) | 34 (72.3) | 30 (76.9) | 29 (61.7) | |||
Low risk: <10% | 9 (19.6) | 3 (2.6) | 15 (25.9) | 1 (2.5) | 2 (4.3) | 0 | 6 (15.4) | 4 (8.5) |
Data are expressed as median [interquartile range] or number (percentage). BMI, body mass index; DECAMP, Detection of Early Lung Cancer Among Military Personnel; Denver, University of Colorado Denver; UPMC, University of Pittsburgh Medical Center; VUMC, Vanderbilt University Medical Center.
Radiomic model diagnostic accuracy
The commercial platform derived radiomic model (ComRad) is a previously validated and published model based on 10 features selected using the LASSO method. A similar LASSO approach was used to select for 12 features to build the open-source platform model (OpenRad). IBSI OpenRad model was developed by choosing features equivalent to the ComRad features based on IBSI nomenclature standards resulted in the inclusion of 11 features. Lastly, we built a Spearman’s OpenRad model with 10 features by selecting open-source features with the highest Spearman’s correlation coefficient with ComRad features.
ComRad achieved an AUC of 0.76 (95% CI: 0.71–0.82). OpenRad had an AUC of 0.75 (95% CI: 0.69–0.81) for LASSO model, 0.71 (95% CI: 0.65–0.77) for IBSI feature model, and 0.74 (95% CI: 0.68–0.79) for Spearman’s correlation model (Figure 2A). Direct comparison of models’ AUC values using bootstrap method showed a significant difference between the ComRad and OpenRad IBSI model (P=0.048). We were not able to show a significant difference between ComRad with OpenRad Spearman’s model using direct comparison (P=0.157).

We also compared the three OpenRad models with ComRad model by performing McNemar’s test at Youden’s cutoff on the test cohort. This allows for the comparison of the different models at their optimal cutoffs, instead of the clinically relevant threshold of 0.1 and 0.7 per BTS for the construction of AUC values. Using McNemar’s test, comparisons of models differed significantly in their classifications between OpenRad Spearman’s model and ComRad model (P=0.003) as well as OpenRad LASSO vs. ComRad (P=0.017).
Mayo scores were added to OpenRad LASSO model, which was the OpenRad model that had the highest AUC, forming an open-source integrated clinical radiomics model (ClinRad). Similarly, the Mayo scores were added to ComRad, forming a commercial-based integrated ClinRad model. All four cohorts were combined and AUCs were estimated for the integrated models, resulting in AUCs of 0.80 (95% CI: 0.74–0.86) for open-source ClinRad model and 0.80 (95% CI: 0.74–0.86) for commercial ClinRad model (Figure 2B).
Calibration of prevalence adjusted model
Using the combined cohort, the models were recalibrated to the clinically relevant prevalence of 33%. The commercial model was appropriately fitted to the population with a slope of 1.023 (mean of 200 repeated 3-fold cross validation samples, 95% CI: 0.64–1.51) with an intercept of −0.007 (95% CI: −0.37 to 0.44). The open-source model was also appropriately fitted to the population with a slope of 1.023 (mean of 200 repeated 3-fold cross validation samples, 95% CI: 0.61–1.57) with an intercept of −0.005 (95% CI: −0.37 to 0.45).
Reclassification of prevalence adjusted model
Using categories defined by decision thresholds of 0.1 and 0.7 in accordance with BTS guidance, nodules were deemed reclassified if their radiomic based model score put them in a different risk group than the Mayo model. We observed cNRI bias-corrected cNRI of 0.09 (0.02–0.15) for benign controls and cNRI of 0.07 (0.00–0.13) for malignancy cases using commercial ClinRad model compared to Mayo. We saw similar improvements for open-source ClinRad model with cNRI of 0.09 (0.02–0.15) for benign controls and cNRI of 0.06 (0.00–0.12) for malignancy cases (Table 2). The baseline Mayo clinic model is calibrated to the training set, then adjusted to a prevalence of cancer of 0.33. All values reported as mean (95% CI) of the test set in the 200 repeated threefold splitting procedures.
Table 2
Performance metrics | Commercial clinical radiomics model (Mayo + radiomics) | Open-source clinical radiomics model (Mayo + radiomics) |
---|---|---|
AUC (95% CI) | 0.80 (0.74–0.86) | 0.80 (0.74–0.86) |
cNRI cancer (95% CI) | 0.07 (0.00–0.13) | 0.06 (0.00–0.12) |
cNRI benign (95% CI) | 0.09 (0.02–0.15) | 0.09 (0.02–0.15) |
AUC, area under the curve; CI, confidence interval; cNRI, bias-corrected clinical net reclassification index; Mayo, Mayo model; LASSO, least absolute shrinkage and selection operator.
Discussion
Radiomics has shown promise to impact clinical care by correctly distinguishing patients with malignant nodules from those with benign process, potentially improving outcomes by speeding time to diagnosis and treatment for cancer and reducing overtreatment for benign nodules. Many radiomic models for IPN diagnosis have been published (18,22-25), but radiomics has yet to become routinely used clinically. One chief reason for this is the lack of generalizability and/or transportability of radiomic models. An abundance of radiomics tools, platforms, and approaches positively serves science by enabling rapid advancement and model development but serves as a hindrance when a radiomic model derived using one tool in a specific study population cannot be replicated using a different tool in a different population. For these reasons, our own work has evaluated the transportability of radiomics, specifically: can a radiomic model derived using radiomic features (such as size, shape, density, and texture) extracted from a CT scan using one platform transport to nominally equivalent features extracted from a different radiomic analysis platform?
Different radiomic platforms may have different methods calculating the same “feature”, so this approach would be impractical. However, much work has been done through the IBSI to standardize how many commonly used features are calculated (19). Despite great success in harmonization of how features are calculated, not all platforms have implemented these recommendations. Additionally, image-preprocessing steps can change the method by which a ROI is presented to the algorithm for feature extraction. Lastly, while the formula for calculating a specific feature may be standardized, the method the ROI is presented to it can differ. For example, as shown in Figure 3, the grey level distance zone matrix (GLDZM) features can be calculated on each 2D slice within the ROI, then the resulting GLDZM features can be averaged to arrive at an aggregate value. Or, as shown in Figure 3B, the intensity values for each slice can be averaged, then the GLDZM can be calculated on the “average” slice. Lastly, the GLDZM can be calculated on the volume, but this can introduce artifacts based upon variable slice thicknesses. Taken together, while the IBSI has shown correlation between features calculated across radiomic platforms, the impact of all these variable factors on the practical outcome of specific radiomic-based prediction models has not been evaluated.
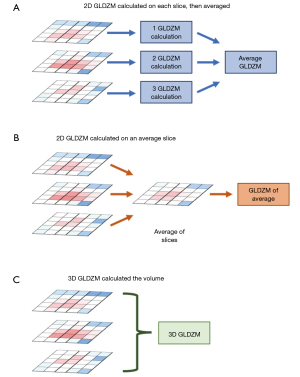
We evaluated this approach by testing the how well a previously published radiomic model based upon 10 radiomic features from the commercial HealthMyne platform can be transported to the open-source PyRadiomics feature extractor. We selected for PyRadiomics features to include in our model that could be considered equivalent to HealthMyne features based on IBSI nomenclature standardization recommendations as well as Spearman’s rank correlation. We found that using both methods to form models with ostensibly the same set of features resulted in divergent diagnostic performances and IPN risk stratifications. It was only when we developed an independent model using LASSO regression and allowing for full flexibility in feature selection to maximize prediction accuracy did the diagnostic performance between the two platforms mirror one another. These findings demonstrate the failure of direct transportability between radiomic feature extractor platforms due to the variability in features; however, equivalent outcomes can be achieved by building independent models using methods that maximizes diagnostic performances.
Furthermore, our findings also support the open-source radiomic platform as a viable tool for radiomics analysis of IPNs. The lower cost associated with open-source platforms allows for more equitable access and collaborative efforts that may accelerate the development of radiomics. There is also security in the sustainability of open-source platforms that will not be threatened by financial insolvency, which is a reality that commercial radiomic platforms face.
Our study had a number of limitations. The cohorts included are mostly from large academic medical centers with referrals from surrounding communities, suggesting higher cancer prevalence than seen in the IPN population, thus limiting generalizability. Our cohorts also demonstrated a higher percentage of malignancy than the desired intended use prevalence of 33% malignant IPNs used for our prediction model. This was adjusted with statistical bootstrap methods, but results may differ in a local, unique population. Furthermore, while we were able to observe different AUC values by each model, many of the models’ CIs overlap, which, as methodologist have pointed out, do not mean the models are equivalent (26). However, when performing direct comparison of the different models using bootstrap method, we were only able to find a significant difference between ComRad and OpenRad IBSI models’ AUCs. The direct comparisons of the other models’ AUCs did not show significant differences. That being said, testing the difference between AUCs, like all hypothesis testing methods, depends on other factors beyond the test itself, such as sample size and variance. Lastly, CT chest scans, especially for incidentally found nodules, can have a significant amount of variation in slice thickness, contrast, and quality. These factors can limit the generalizability of our study. Furthermore, a significant limiting component in radiomics generally and a cause of variability across readers and cohorts is method of segmentation. There are manual, semi-automated, and fully automated segmentation tools available, with less automated methods having more likelihood of inter and intra user variability. We accounted for this in our study by using the same segmentations, created using HealthMyne’s semi-automated segmenter, for feature extraction by both platforms. Both HealthMyne and PyRadiomics are traditional radiomic platforms and differ from the fully automated Optellum (Lung Cancer Prediction Convolutional Neural Network model). Optellum offers some benefits to traditional radiomics by reportedly having slightly higher diagnostic accuracies, less onerous process with no need of manual segmentation, and greater reproducibility. Future research should directly compare the respective diagnostic accuracies and efficiencies of fully automated platforms such as Optellum to traditional, open-source platforms.
Conclusions
Our study contributes to the growing body of evidence demonstrating the utility of radiomics, including an open-source platform, for improving lung malignancy risk stratification. Furthermore, we revealed that the transportability of radiomic models across platforms directly does not conserve performance, but radiomic platforms can provide equivalent results when building de novo models. Efforts to improve transportability between radiomic platforms is paramount for direct comparison of model diagnostic performances, which is necessary for finding the best models and improving upon them for clinical implementation ultimately. Furthermore, additional work is necessary to evaluate how specific platform features, segmentation tools and techniques, image quality, and nodule characteristics contribute to diagnostic accuracy of radiomic tools.
Acknowledgments
The authors thank the DECAMP Consortium. They also thank Katie Dickerson of HealthMyne for her technical support of incredible service throughout the project.
Funding: This work was supported by
Footnote
Reporting Checklist: The authors have completed the STARD reporting checklist. Available at https://tlcr.amegroups.com/article/view/10.21037/tlcr-24-281/rc
Data Sharing Statement: Available at https://tlcr.amegroups.com/article/view/10.21037/tlcr-24-281/dss
Peer Review File: Available at https://tlcr.amegroups.com/article/view/10.21037/tlcr-24-281/prf
Conflicts of Interest: All authors have completed the ICMJE uniform disclosure form (available at https://tlcr.amegroups.com/article/view/10.21037/tlcr-24-281/coif). D.X. reports receiving support from an NIH grant (No. T32CA106183-19). A.E.B. reports receiving support from an NIH grant (No. R01CA252964). S.A.D. reports receiving support from an NIH grant (No. U01CA152662). E.L.G. reports receiving support from NIH grants (Nos. R01CA252964 and U01CA152662). The other authors have no conflicts of interest to declare.
Ethical Statement: The authors are accountable for all aspects of the work in ensuring that questions related to the accuracy or integrity of any part of the work are appropriately investigated and resolved. The study was conducted in accordance with the Declaration of Helsinki (as revised in 2013). The study was approved by Internal Review Board at Vanderbilt University (Study #090781) and informed consent was obtained from all individual participants.
Open Access Statement: This is an Open Access article distributed in accordance with the Creative Commons Attribution-NonCommercial-NoDerivs 4.0 International License (CC BY-NC-ND 4.0), which permits the non-commercial replication and distribution of the article with the strict proviso that no changes or edits are made and the original work is properly cited (including links to both the formal publication through the relevant DOI and the license). See: https://creativecommons.org/licenses/by-nc-nd/4.0/.
References
- Mariotto AB, Enewold L, Zhao J, et al. Medical Care Costs Associated with Cancer Survivorship in the United States. Cancer Epidemiol Biomarkers Prev 2020;29:1304-12. [Crossref] [PubMed]
- Pastorino U, Sverzellati N, Sestini S, et al. Ten-year results of the Multicentric Italian Lung Detection trial demonstrate the safety and efficacy of biennial lung cancer screening. Eur J Cancer 2019;118:142-8. [Crossref] [PubMed]
- de Koning HJ, van der Aalst CM, de Jong PA, et al. Reduced Lung-Cancer Mortality with Volume CT Screening in a Randomized Trial. N Engl J Med 2020;382:503-13. [Crossref] [PubMed]
- National Lung Screening Trial Research Team. Reduced lung-cancer mortality with low-dose computed tomographic screening. N Engl J Med 2011;365:395-409. [Crossref] [PubMed]
- Jonas DE, Reuland DS, Reddy SM, et al. Screening for Lung Cancer With Low-Dose Computed Tomography: Updated Evidence Report and Systematic Review for the US Preventive Services Task Force. JAMA 2021;325:971-87. [Crossref] [PubMed]
- American Cancer Society. American Cancer Society: Cancer Facts and Figures 2021. Atlanta, GA: American Cancer Society; 2021.
- Jemal A, Fedewa SA. Lung Cancer Screening With Low-Dose Computed Tomography in the United States-2010 to 2015. JAMA Oncol 2017;3:1278-81. [Crossref] [PubMed]
- Gould MK, Donington J, Lynch WR, et al. Evaluation of individuals with pulmonary nodules: when is it lung cancer? Diagnosis and management of lung cancer, 3rd ed: American College of Chest Physicians evidence-based clinical practice guidelines. Chest 2013;143:e93S-e120S.
- Gould MK, Tang T, Liu IL, et al. Recent Trends in the Identification of Incidental Pulmonary Nodules. Am J Respir Crit Care Med 2015;192:1208-14. [Crossref] [PubMed]
- MacMahon H, Naidich DP, Goo JM, et al. Guidelines for Management of Incidental Pulmonary Nodules Detected on CT Images: From the Fleischner Society 2017. Radiology 2017;284:228-43. [Crossref] [PubMed]
- Baldwin DR, Callister MEGuideline Development Group. The British Thoracic Society guidelines on the investigation and management of pulmonary nodules. Thorax 2015;70:794-8. [Crossref] [PubMed]
- American College of Radiology. [cited 2022 Aug 8]. Lung CT Screening Reporting & Data System (Lung-RADS). Available online: https://www.acr.org/Clinical-Resources/Reporting-and-Data-Systems/Lung-Rads
- NCCN. NCCN Lung Cancer Screening Guideline V2.2022 [cited 2022 Aug 8]. Available online: https://www.nccn.org/guidelines/guidelines-detail?category=2&id=1441
- Tanner NT, Aggarwal J, Gould MK, et al. Management of Pulmonary Nodules by Community Pulmonologists: A Multicenter Observational Study. Chest 2015;148:1405-14. [Crossref] [PubMed]
- Deppen SA, Blume JD, Kensinger CD, et al. Accuracy of FDG-PET to diagnose lung cancer in areas with infectious lung disease: a meta-analysis. JAMA 2014;312:1227-36. [Crossref] [PubMed]
- Maiga AW, Deppen SA, Mercaldo SF, et al. Assessment of Fluorodeoxyglucose F18-Labeled Positron Emission Tomography for Diagnosis of High-Risk Lung Nodules. JAMA Surg 2018;153:329-34. [Crossref] [PubMed]
- Gillies RJ, Kinahan PE, Hricak H. Radiomics: Images Are More than Pictures, They Are Data. Radiology 2016;278:563-77. [Crossref] [PubMed]
- Kammer MN, Lakhani DA, Balar AB, et al. Integrated Biomarkers for the Management of Indeterminate Pulmonary Nodules. Am J Respir Crit Care Med 2021;204:1306-16. [Crossref] [PubMed]
- Zwanenburg A, Vallières M, Abdalah MA, et al. The Image Biomarker Standardization Initiative: Standardized Quantitative Radiomics for High-Throughput Image-based Phenotyping. Radiology 2020;295:328-38. [Crossref] [PubMed]
- Billatos E, Duan F, Moses E, et al. Detection of early lung cancer among military personnel (DECAMP) consortium: study protocols. BMC Pulm Med 2019;19:59. [Crossref] [PubMed]
- Paynter NP, Cook NR. A bias-corrected net reclassification improvement for clinical subgroups. Med Decis Making 2013;33:154-62. [Crossref] [PubMed]
- Massion PP, Antic S, Ather S, et al. Assessing the Accuracy of a Deep Learning Method to Risk Stratify Indeterminate Pulmonary Nodules. Am J Respir Crit Care Med 2020;202:241-9. [Crossref] [PubMed]
- Ardila D, Kiraly AP, Bharadwaj S, et al. End-to-end lung cancer screening with three-dimensional deep learning on low-dose chest computed tomography. Nat Med 2019;25:954-61. Erratum in: Nat Med 2019;25:1319. [Crossref] [PubMed]
- Maldonado F, Duan F, Raghunath SM, et al. Noninvasive Computed Tomography-based Risk Stratification of Lung Adenocarcinomas in the National Lung Screening Trial. Am J Respir Crit Care Med 2015;192:737-44. [Crossref] [PubMed]
- Schabath MB, Gillies RJ. Noninvasive Quantitative Imaging-based Biomarkers and Lung Cancer Screening. Am J Respir Crit Care Med 2015;192:654-6. [Crossref] [PubMed]
- Nicholls A. Confidence limits, error bars and method comparison in molecular modeling. Part 2: comparing methods. J Comput Aided Mol Des 2016;30:103-26. [Crossref] [PubMed]