Prognostic role of dynamic changes in inflammatory indicators in patients with non-small cell lung cancer treated with immune checkpoint inhibitors—a retrospective cohort study
Highlight box
Key findings
• Elevated dynamic changes in the neutrophil-to-lymphocyte ratio and platelet-to-lymphocyte ratio are associated with lower response rates and shorter progression-free survival in patients with metastatic non-small cell lung cancer (NSCLC) treated with immune checkpoint inhibitors (ICIs).
What is known and what is new?
• Many studies have confirmed that programmed cell death ligand 1 (PD-L1) expression is the most useful biomarker in predicting the responses and outcomes of the programmed death protein 1/PD-L1 blockade. Microsatellite instability and the tumor mutation burden are being explored as predictive and prognostic biomarkers for the activity of ICIs in different tumor types. Tumor-infiltrating lymphocytes and immune specific signatures have also been investigated with promising results. However, all of the aforementioned methods require invasive procedures that place heavy physical and psychological burdens on patients.
• Simple, effective, and definitive biomarkers of responses to ICIs were identified in the blood of NSCLC patients.
What is the implication, and what should change now?
• The findings could help to identify patients with NSCLC who could benefit from ICI treatment.
Introduction
The incidence and mortality rates of lung cancer are increasing year by year, and it continues to be the most common cause of cancer-related death in China and around the world (1,2). Non-small cell lung cancer (NSCLC) is the most common pathological subtype of lung cancer, and accounts for about 85% of all lung cancers (3). In recent years, the anti-programmed cell death 1/ligand 1 (anti-PD-1/PD-L1) antibody, immune checkpoint inhibitors (ICIs), have greatly improved the prognosis of lung cancer patients. ICI monotherapy or chemo-immunotherapy is the preferred treatment option for advanced lung cancer patients without oncogenic drivers (3). However, immunotherapy is expensive and has limited effectiveness against lung cancer as a second-line treatment. It may also lead to an increase in the incidence and severity of treatment-related adverse events (4-6). Thus, further research is needed to identify additional biomarkers and revise existing biomarkers to both optimize patient selection and improve treatment outcomes for NSCLC patients.
Under normal circumstances, the PD-1/PD-L1 axis is necessary to maintain human immune homeostasis (7). PD1, an immune inhibitory receptor, is expressed in many immune cells, including T cells (7). When PD1 is combined with its ligand PD-L1, an inhibitory signal is activated that suppresses T-cell proliferation and protects normal cells from collateral damage (8,9). Tumor cells highly express the ligand PD-L1, which inhibits the function of immune cells by binding to PD1 to evade immune surveillance and achieve immune escape (10). PD-L1 expression is an effective biomarker in predicting the responses and outcomes of the PD-1/PD-L1 blockade (11). However, several factors can limit its reliability, including the use of various antibodies for detecting PD-L1 expression, different procedures for biopsy collection and storage, the lack of defined thresholds for quantifying PD-L1 expression, and intra-tumor heterogeneity in PD-L1 expression. Recently, research has explored the use of microsatellite instability (MSI) and the tumor mutation burden (TMB) as predictive and prognostic biomarkers for the activity of ICIs in different tumor types (12). Tumor-infiltrating lymphocytes (TILs) and immune specific signatures have been investigated with promising results (13,14). In addition, a previous study revealed that thymic density changes were observed in nearly all NSCLC patients undergoing immunotherapy, with decreased density associated with longer overall survival (OS) (15). However, most of the aforementioned methods require invasive procedures that place heavy physical and psychological burdens on patients. Therefore, it is necessary to identify simple, effective, and definitive biomarkers of response to ICIs.
Pathologic response, such as inflammation, has been proposed as a major driver of the development and progression of various types of tumor (16). Immune cells are key components of the tumor microenvironment, and their presence is most commonly associated with the outcomes of patients (17,18). As many studies have reported, cytotoxic CD8 T cells are strongly associated with improved clinical outcomes in patients, while some immune cells, such as neutrophils, M2 polarized macrophages, and Forkhead Box P3 (FOXP3) positive regulatory T cells, are risk factors for tumor progression and are related to a poor prognosis (19-22). Similarly, hematological inflammatory parameters can also reflect the immune status of patients and have been established as important predictive markers associated with the prognosis of tumors (23-27). An increased neutrophil-to-lymphocyte ratio (NLR) and platelet-to-lymphocyte ratio (PLR) have been reported to be independent poor prognostic indicators in several tumor types, including NSCLC (23-25,28). Recently, many studies have showed that the NLR and PLR are also associated with worse outcomes in patients treated with ICIs (29-31). However, most of studies only indicated the prognostic effect of the static level, they fail to show the real-time association of survival with dynamic changes in these markers over time, which potentially reflects a combined time- and treatment-dependent variation in the underlying disease process (32,33).
In this study, we investigated the usefulness of the NLR and PLR in predicting the clinical outcomes of NSCLC patients undergoing treatments with PD-1 inhibitors. The prediction model, which was constructed based on hematological inflammatory parameters, may be an effective, cheap and readily available method for assessing the prognostic markers of NSCLC patients treated with ICIs. We present this article in accordance with the REMARK reporting checklist (available at https://tlcr.amegroups.com/article/view/10.21037/tlcr-24-637/rc).
Methods
Patients selection
The sample size was calculated using the Raosoft calculator. In total, 95 patients were included in the present study. We retrospectively reviewed the data of patients who were treated with ICIs (pembrolizumab, nivolumab, atezolizumab, or camrelizumab) between January 2015 and March 2018 at Shanghai Pulmonary Hospital. The patients who received ICI-based combination therapy (camrelizumab in combination with pemetrexed/carboplatin or apatinib) were also identified. Patients who had been treated with immunotherapeutic agents previously, or who had died within 4 weeks of their first ICI treatment were excluded from the analysis. The study was approved by the Ethics Committee of Shanghai Pulmonary Hospital (No. K24-264Y). Informed consent for participation in the study and the publication of the results was obtained from each patient, parent, or guardian. The study was conducted in accordance with the Declaration of Helsinki (as revised in 2013).
Data collection
The following data were extracted from the medical records of all the patients: demographic characteristics, Eastern Cooperative Oncology Group-performance status (ECOG-PS) score at the time of initiating the ICI therapy, smoking history, histology, molecular alterations of EGFR/ALK/ROS1/BRAFV600E/Kras/HER-2/RET/c-MET (when available), metastatic sites at the time of initiating the ICI therapy, prior lines of chemotherapy and radiation therapy, baseline complete blood count [defined as the results obtained at the time (−3/0 days) of initiating the ICI therapy, including the absolute neutrophil count (ANC), absolute lymphocyte count (ALC), and platelet count to calculate the NLR and PLR]. Derived NLR (dNLR) was calculated as follows: dNLR = ANC/(WBC − ANC). Cycle 3 (C3) complete blood count (which was defined as the results obtained before the C3 of ICIs) was also collected and calculated in the present study. Responses were assessed by computed tomography (CT) imaging and classified according to the Response Evaluation Criteria in Solid Tumors (RECIST) version 1.1 every 6–12 weeks during treatment.
Statistical analysis
The Mann-Whitney U test was used to compare the continuous variables, and Fisher’s exact test or the Chi-square test was used to compare the categorical variables. Progression-free survival (PFS) was defined as the time from ICI treatment to disease progression or death from any cause. The Statistical Package for the Social Sciences software (version 23.0, SPSS Inc., Chicago, IL, USA) was used to analyze all the statistical data. Kaplan-Meier survival curves were compared using the log-rank test. Univariate and multivariate analyses were performed using the Cox proportional hazards regression model. The hazard ratios (HRs) and 95% confidence intervals (95% CIs) for the variables were calculated using the Cox proportional hazards model. We reported 95% CIs with two-sided P<0.05 being set as a threshold for significance.
Results
Patient characteristics and overall clinical outcomes
In total, 95 patients were included in the present study. The baseline characteristics of the patients are listed in Table 1. The median age of the enrolled patients was 61 years (range, 36 to 82 years). In brief, the majority of patients were male (83.2%, 79/95), 62.1% (59/95) were former or current smokers, 98.9% (94/95) had a good ECOG-PS score (of 0 or 1), 66.3% (63/95) had adenocarcinoma, 93.7% (89/95) had stage IV disease, and 87.4% were without molecular alterations (EGFR/ALK/ROS1/BRAFV600E/Kras/HER-2/RET/c-MET). In relation to the sites of the metastatic lesions in the 95 patients, 14.7% presented in the brain, 16.8% in the liver, and 44.2% in the bone. Additionally, 15.8% (15/95) of the patients had previously received chest radiation, 8.4% (8/95) had previously received cranial radiation, and 14.7% (14/95) had previously received bone radiation.
Table 1
Characteristic | All patients (N=95) |
---|---|
Age (years) | |
≤65 | 59 (62.1) |
>65 | 36 (37.9) |
Gender | |
Male | 79 (83.2) |
Female | 16 (16.8) |
Smoking history | |
Non-smoker | 36 (37.9) |
Former or current smoker | 59 (62.1) |
ECOG-PS | |
0 | 11 (11.6) |
1 | 83 (87.4) |
2 | 1 (1.1) |
Histologic subtypes | |
Adenocarcinoma | 63 (66.3) |
Squamous cell carcinoma | 21 (22.1) |
Other† | 11 (11.6) |
TNM stage | |
IIIB | 6 (6.3) |
IV | 89 (93.7) |
Molecular alterations | |
WT (EGFR/ALK/ROS1/BRAFV600E/Kras/HER-2/RET/c-MET) | 83 (87.4) |
Kras | 7 (7.4) |
HER-2 | 1 (1.1) |
BRAFV600E | 2 (2.1) |
ROS1 | 1 (1.1) |
Chest radiation | |
Yes | 15 (15.8) |
No | 80 (84.2) |
Cranial radiation | |
Yes | 8 (8.4) |
No | 87 (91.6) |
Bone radiation | |
Yes | 14 (14.7) |
No | 81 (85.3) |
Types of immune checkpoint inhibitors | |
Pembrolizumab | 34 (35.8) |
Nivolumab | 14 (14.7) |
Atezolizumab | 1 (1.1) |
Camrelizumab plus pemetrexed/carboplatin‡ | 28 (29.5) |
Camrelizumab plus apatinib§ | 18 (18.9) |
Lines of treatment | |
1 | 36 (37.9) |
2 | 26 (27.4) |
>2 | 33 (34.7) |
Brain metastasis | 14 (14.7) |
Liver metastasis | 16 (16.8) |
Bone metastasis | 42 (44.2) |
Pretreatment NLR | |
≥5 | 23 (24.2) |
<5 | 72 (75.8) |
Pretreatment derived NLR | |
≥3 | 30 (31.6) |
<3 | 65 (68.4) |
Pretreatment PLR | |
<150 | 46 (48.4) |
150–300 | 40 (42.2) |
>300 | 9 (9.4) |
Data are presented as n (%). †, including 11 cases of NSCLC, 1 case of large cell carcinoma, and 1 case of adenosquamous case; ‡, 1 patient treated with pembrolizumab plus abraxane; §, 1 patient treated with pembrolizumab plus apatinib, 1 patient treated with nivolumab plus bevacizumab. ECOG-PS, Eastern Corporation Oncology Group-performance status; TNM, tumor-node-metastasis; WT, wild-type; NLR, neutrophil-to-lymphocyte ratio; PLR, platelet-to-lymphocyte ratio; NSCLC, non-small cell lung cancer.
In relation to the types of ICIs administered to the 95 patients, 35.8% of the patients received pembrolizumab, 14.7% received nivolumab, 1.1% received atezolizumab, 29.5% received camrelizumab plus pemetrexed/carboplatin (including 1 patient treated with pembrolizumab plus abraxane), and 18.9% received camrelizumab plus apatinib (including 1 patient treated with pembrolizumab plus apatinib, and 1 patient treated with nivolumab plus bevacizumab). The majority of the patients received ICI therapy as a first-line/second-line therapy (37.9%/27.4%).
At the baseline, 23 (24.2%) patients had a NLR ≥5, and 30 (31.6%) patients had a dNLR ≥3, while 46 (48.4%) had a PLR <150, and 40 (42.2%) had a PLR falling between 150 and 300. The cut-off values were consistent with that reported in most of the literature.
The overall response rate (ORR) of the cohort was 32.6% and the median PFS was 7.1 months. The ORRs of the monotherapy and combination therapy groups were 27.7% and 37.5%, respectively. While the median PFS of the two cohorts were 5.5 and 8.3 months, respectively.
The association between the NLR and clinical outcomes
When a baseline NLR value of 5 was used as the cut-off value, patients with a baseline NLR ≥5 had a similar ORR (30.4% versus 33.3%, P=0.79 and median PFS as those with a NLR <5 (6.7 vs. 7.1 months, HR: 1.01, 95% CI: 0.55–1.87; P=0.96) (Figure 1A).
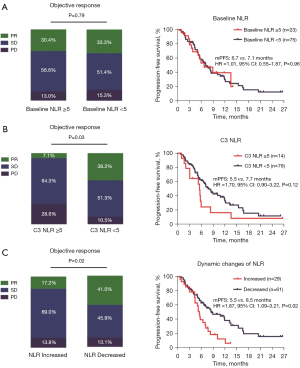
When 5 was used as the C3 NLR cut-off value, patients with a C3 NLR <5 (84.4%, 76/90) had a better ORR than those with a C3 NLR ≥5 (38.2% vs. 7.1%, P=0.03). There was also a trend toward better median PFS in patients with a C3 NLR <5; however, the difference was not statistically significant (7.7 vs. 5.5 months, HR: 1.70, 95% CI: 0.90–3.22; P=0.12) (Figure 1B).
To further clarify the relationship between dynamic changes in the NLR and clinical outcomes, the patients were divided into two groups. The patients who had an increased NLR (C3 vs. the baseline) were defined as the increased group, while those who had a decreased NLR were defined as the decreased group. Interestingly, the patients who had an increased NLR (32.2%, 29/90) had an inferior ORR (17.2% vs. 41.0%, P=0.02) and median PFS (5.5 vs. 8.5 months, HR: 1.87, 95% CI: 1.09–3.21; P=0.02) than those who had a decreased NLR (67.8%, 61/90) (Figure 1C).
The association between the dNLR and clinical outcomes
When performing the same analyses using 3 as the cut-off value for the baseline dNLR, no significant difference was found between the two groups (≥3 vs. <3) in terms of both the ORR and median PFS (Figure 2A). The patients with a C3 dNLR <3 had a borderline statistically significant better ORR (38.6% vs. 15.0%, P=0.06) and median PFS (8.2 vs. 5.6 months, HR: 1.67, 95% CI: 0.94–2.97; P=0.08) than those with a C3 dNLR ≥3 (Figure 2B). In relation to the dynamic changes in the dNLR, the patients who had an increased dNLR (28.9%, 26/90) had a lower ORR (15.4% vs. 40.3%, P=0.02) and inferior median PFS (5.6 vs. 8.4 months, HR: 1.49, 95% CI: 0.87–2.57; P=0.15) than those who had a decreased dNLR (68.9%, 62/90) (Figure 2C).
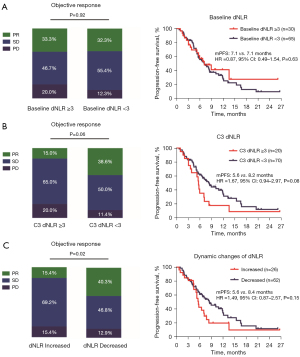
The association between the PLR and clinical outcomes
The patients were divided into three groups based on their PLR (i.e., the <150, 150–300, and >300 PLR groups). As Figure 3A shows, the baseline PLR was not associated with the clinical outcomes of ICI therapy. There was a trend toward a better ORR (20.0% vs. 27.8% vs. 40.9%, P=0.29) and median PFS (5.5 vs. 7.0 vs. 8.3 months, P=0.10) in patients with a lower C3 PLR, but the difference was not statistically significant (Figure 3B). Interestingly, the patients who had a decreased PLR (52.2%, 47/90) had a better ORR (42.6% vs. 23.3%, P=0.052) and median PFS (11.8 vs. 5.5 months, HR: 2.28, 95% CI: 1.32–3.94; P=0.003) than those who had an increased PLR (47.8%, 43/90) (Figure 3C).
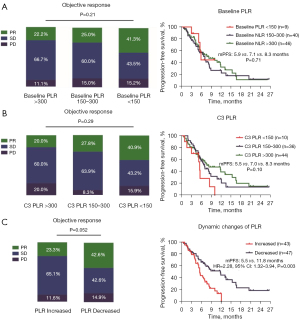
The association between dynamic changes in the NLR and PLR and clinical outcomes
Based on the dynamic changes in the NLR and PLR, the patients were further stratified into the three groups based on whether they had both an increased NLR and PLR (22.2%, 20/90), either an increased NLR or PLR (35.6%, 32/90), or both a decreased NLR and PLR (42.2%, 38/90). As Figure 4 shows, patients who had both a decreased NLR and PLR had a better ORR (47.4% vs. 28.1% vs. 15.0%, P=0.03) and prolonged median PFS (11.8 vs. 5.5 vs. 5.6 months, P=0.003) than those with either an increased NLR or PLR, or both an increased NLR and PLR.
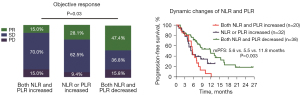
Univariate and multivariate analyses of median PFS
The univariate analysis identified previous chest radiation, the lines of treatment, the number of metastatic sites, and dynamic changes in the NLR, C3 PLR, and PLR as being significantly associated with median PFS. The multivariate analysis revealed that dynamic changes in the PLR was an independent predictive factor of PFS (HR: 2.27, 95% CI: 1.10–4.71, P=0.03), as was previous chest radiation (HR: 0.39, 95% CI: 0.18–0.85, P=0.02), and the number of metastatic sites (HR: 2.53, 95% CI: 1.25–5.12, P=0.01) (Table 2).
Table 2
Variables | Univariate analysis | Multivariate analysis | |||
---|---|---|---|---|---|
HR (95% CI) | P | HR (95% CI) | P | ||
Female vs. male | 1.72 (0.92–3.20) | 0.09* | 1.60 (0.89–2.87) | 0.11 | |
Age (≤65 vs. >65) years | 1.26 (0.76–2.08) | 0.37 | |||
Smokers vs. non-smokers | 0.74 (0.45–1.23) | 0.24 | |||
Histologic subtypes | |||||
SQCC vs. ADC | 0.93 (0.45–1.92) | 0.83 | |||
Other vs. ADC | 1.35 (0.60–3.07) | 0.47 | |||
TNM (stage IV vs. stage IIIB) | 1.59 (0.64–3.98) | 0.32 | |||
Molecular alteration (mutant vs. WT) | 1.08 (0.49–2.37) | 0.85 | |||
Chest radiation (yes vs. no) | 0.31 (0.17–0.57) | <0.001* | 0.39 (0.18–0.85) | 0.02* | |
Cranial radiation (yes vs. no) | 0.51 (0.23–1.12) | 0.09* | 0.95 (0.35–2.59) | 0.92 | |
Bone radiation (yes vs. no) | 0.70 (0.36–1.35) | 0.28 | |||
Types of ICIs | |||||
ICIs + chemotherapy vs. ICIs monotherapy | 0.63 (0.33–1.18) | 0.14 | |||
ICIs + apatinib vs. ICIs monotherapy | 0.97 (0.51–1.86) | 0.93 | |||
Lines of treatment (>1 vs. first-line) | 1.46 (1.08–1.96) | 0.01* | 1.26 (0.87–1.80) | 0.22 | |
The number of metastatic sites (>2 vs. ≤2) | 2.07 (1.33–3.90) | 0.003* | 2.53 (1.25–5.12) | 0.01* | |
Baseline NLR (≥5 vs. <5) | 1.01 (0.55–1.87) | 0.96 | |||
C3 NLR (≥5 vs. <5) | 1.70 (0.90–3.22) | 0.10 | |||
Dynamic changes in the NLR | |||||
Increased versus decreased | 1.87 (1.09–3.21) | 0.02* | 1.30 (0.70–2.42) | 0.40 | |
Baseline dNLR (≥3 vs. <3) | 0.87 (0.49–1.54) | 0.63 | |||
C3 dNLR (≥3 vs. <3) | 1.67 (0.94–2.97) | 0.08* | 1.91 (0.92–3.96) | 0.08* | |
Dynamic changes in dNLR | |||||
Increased versus decreased | 1.49 (0.87–2.57) | 0.15 | |||
Baseline PLR | |||||
150–300 vs. <150 | 1.21 (0.73–2.01) | 0.46 | |||
>300 vs. <300 | 0.92 (0.32–2.63) | 0.87 | |||
C3 PLR | |||||
150–300 vs. <150 | 1.24 (0.71–2.14) | 0.45 | 1.20 (0.38–3.79) | 0.76 | |
>300 vs. <300 | 2.24 (1.00–5.03) | 0.05* | 0.95 (0.36–2.50) | 0.92 | |
Dynamic changes in the PLR | |||||
Increased versus decreased | 2.28 (1.32–3.94) | 0.003* | 2.27 (1.10–4.71) | 0.03* |
*, P value is less than 0.1. PFS, progression-free survival; SQCC, squamous cell carcinoma; ADC, adenocarcinoma; TNM, tumor-node-metastasis; WT, wild-type; ICIs, immune checkpoint inhibitors; NLR, neutrophil-to-lymphocyte ratio; C3, cycle 3; dNLR, derived neutrophil-to-lymphocyte ratio; PLR, platelet-to-lymphocyte ratio; HR, hazard ratio; CI, confidence interval.
Discussion
The development of ICIs has altered the treatment landscape of NSCLC with remarkable success. Inflammation serves as an important factor in tumor development and progression (16). A wide variety of molecules, which are produced in the process of inflammation, can serve as potential predictive prognostic markers in NSCLC patients (34,35). In our study, dynamic changes in the NLR, dNLR, and PLR were significantly associated with the clinical outcomes of metastatic NSCLC patients treated with ICIs. Further, dynamic changes in the PLR could potentially be used to predict the efficacy of ICI therapy.
As is well known, inflammation plays an important role in the spreading of all kinds of tumors, and related molecules can be used to predict patient prognosis. High levels of peripheral neutrophils and neutrophil extracellular traps have been reported to be associated with tumor development and progression (36). Specifically, the inflammatory factors and growth factors released by neutrophils promote the growth of tumor cells, inhibit apoptosis, and promote angiogenesis, and ultimately result in tumor progression (36). Conversely, lymphocytes mainly exert anti-tumor effects via the regulation of cellular immunity (37). Platelets play an important role in tumor blood metastases by releasing platelet-derived growth factor (PDGF) and platelet-reactive protein (38,39). These findings suggest that hematological inflammatory parameters could serve as potential prognostic markers in cancer patients.
In this study, after two cycles of ICI treatment, the patients with a lower NLR and dNLR had a better ORR and median PFS than those with a higher NLR and dNLR. Moreover, the patients who had an increased NLR, dNLR, and PLR (C3 versus the baseline) had a lower ORR and an inferior median PFS than those with a decreased NLR, dNLR, and PLR. As previously reported, the prognostic value of pretreatment indexes is questionable (32,40). Our study results also indicated that the baseline NLR, dNLR, and PLR were not associated with the clinical outcomes of ICI therapy. These results highlight the vital role of the dynamic changes (rather than the baseline levels) of inflammatory parameters in predicting responses to ICIs and clinical outcomes.
Together with targeted therapies, ICIs have revolutionized the treatment and become an indispensable part of the standard treatment of all stages of NSCLC, including metastatic, locally advanced, and even early stage disease as part of a neoadjuvant therapy (3,41). Moreover, as a previous study have reported, serious immune-related adverse side effects after ICI treatment still occur in 10–30% of cases (42). Therefore, effective and definitive biomarkers are still needed to confirm which patients are more likely to respond to treatments and to dynamically monitor the occurrence of toxic side effects.
At present, the expression of PDL1 in tumor tissues is still mainly used to predict responses to the PD-1/PD-L1 blockade in both clinical research and clinical practice (11). In addition to PD-L1, the proportion or TILs and the TMB have also been proposed as important biomarkers. The different proportion or abundance of TILs can accurately predict the survival outcomes of patients with a variety of tumors (13,43). NSCLC patients with high TMB tumors have an improved ORR and improved PFS after ICI treatment (44). A TMB >10 mutations/megabase has been shown to predict the efficacy of ICI therapy (45). However, all of these measures require the availability of tumor tissues from patients, which is invasive and places risks and burdens on patients. Our results revealed that dynamic change in the PLR was an independent predictive factor of PFS in NSCLC patients who received ICI therapy. As is well known, regular blood routine examination is necessary for cancer patients after therapy. Thus, building a predictive model using peripheral blood biomarkers is practically feasible, and such a model could be used to facilitate the longitudinal follow-up of ICI treatment responses and monitor the occurrence of immune-related side effects.
Platelet elevation accelerates cancer progression and metastasis mainly by promoting angiogenesis and the production of adhesion molecules (38,39). Many studies have shown that platelet quantity is associated with prognosis and response to immunotherapy in NSCLC, colorectal cancer, and other tumors (46-49). Among them, the prognosis prediction efficacy of the platelet volume, platelet distribution width, PLR, platelet-related genes, and platelet-derived microparticles in circulating blood has been confirmed (30,50-52). In the present study, only dynamic change in the PLR was identified as an independent predictive factor of PFS in NSCLC patients receiving ICI treatment. This result may emphasize the more important role of platelets in the evaluation of the ICI efficacy, especially in NSCLC. In a recent study, researchers found that platelets from the blood of NSCLC patients could inhibit T-cell infiltration in tumors through PD-L1 expression (46). Platelets can also serve as a physical barrier surrounding tumor cells, preventing natural killer cells from killing tumor cells (53,54). In addition, platelet-derived growth factors, such as PDGF and transforming growth factor-beta (TGF-β), promote tumor growth by accelerating tumor proliferation and promoting angiogenesis (38,55). The above findings provide some evidence of the value of the PLR in predicting immunotherapy efficacy. However, the specific mechanisms by which platelets affect the efficacy of immunotherapy in lung cancer require further studies.
Conclusions
A decreased NLR and PLR is related to a better ORR and prolonged median PFS in NSCLC patients who receive ICI treatment. A further analysis revealed that dynamic change in the PLR was an independent factor in predicting PFS. This could help to identify patients with NSCLC who could benefit from ICI treatment.
Acknowledgments
Funding: This project was supported by
Footnote
Reporting Checklist: The authors have completed the REMARK reporting checklist. Available at https://tlcr.amegroups.com/article/view/10.21037/tlcr-24-637/rc
Data Sharing Statement: Available at https://tlcr.amegroups.com/article/view/10.21037/tlcr-24-637/dss
Peer Review File: Available at https://tlcr.amegroups.com/article/view/10.21037/tlcr-24-637/prf
Conflicts of Interest: All authors have completed the ICMJE uniform disclosure form (available at https://tlcr.amegroups.com/article/view/10.21037/tlcr-24-637/coif). The authors have no conflicts of interest to declare.
Ethical Statement: The authors are accountable for all aspects of the work in ensuring that questions related to the accuracy or integrity of any part of the work are appropriately investigated and resolved. The study was approved by the Ethics Committee of Shanghai Pulmonary Hospital (No. K24-264Y). Informed consent for participation in the study and the publication of the results was obtained from each patient, parent, or guardian. The study was conducted in accordance with the Declaration of Helsinki (as revised in 2013).
Open Access Statement: This is an Open Access article distributed in accordance with the Creative Commons Attribution-NonCommercial-NoDerivs 4.0 International License (CC BY-NC-ND 4.0), which permits the non-commercial replication and distribution of the article with the strict proviso that no changes or edits are made and the original work is properly cited (including links to both the formal publication through the relevant DOI and the license). See: https://creativecommons.org/licenses/by-nc-nd/4.0/.
References
- Chen W, Zheng R, Baade PD, et al. Cancer statistics in China, 2015. CA Cancer J Clin 2016;66:115-32. [Crossref] [PubMed]
- Sung H, Ferlay J, Siegel RL, et al. Global Cancer Statistics 2020: GLOBOCAN Estimates of Incidence and Mortality Worldwide for 36 Cancers in 185 Countries. CA Cancer J Clin 2021;71:209-49. [Crossref] [PubMed]
- Punekar SR, Shum E, Grello CM, et al. Immunotherapy in non-small cell lung cancer: Past, present, and future directions. Front Oncol 2022;12:877594. [Crossref] [PubMed]
- Conroy M, Naidoo J. Immune-related adverse events and the balancing act of immunotherapy. Nat Commun 2022;13:392. [Crossref] [PubMed]
- Shankar B, Zhang J, Naqash AR, et al. Multisystem Immune-Related Adverse Events Associated With Immune Checkpoint Inhibitors for Treatment of Non-Small Cell Lung Cancer. JAMA Oncol 2020;6:1952-6. [Crossref] [PubMed]
- Xu M, Xu L, Hao Y, et al. Clinical characteristics and prognostic implications of immune-related hepatitis in patients with advanced non-small cell lung cancer treated with immune checkpoint inhibitors: a retrospective study. J Thorac Dis 2024;16:1900-10. [Crossref] [PubMed]
- Ahmadzadeh M, Johnson LA, Heemskerk B, et al. Tumor antigen-specific CD8 T cells infiltrating the tumor express high levels of PD-1 and are functionally impaired. Blood 2009;114:1537-44. [Crossref] [PubMed]
- Butte MJ, Keir ME, Phamduy TB, et al. Programmed death-1 ligand 1 interacts specifically with the B7-1 costimulatory molecule to inhibit T cell responses. Immunity 2007;27:111-22. [Crossref] [PubMed]
- Keir ME, Liang SC, Guleria I, et al. Tissue expression of PD-L1 mediates peripheral T cell tolerance. J Exp Med 2006;203:883-95. [Crossref] [PubMed]
- Salmaninejad A, Khoramshahi V, Azani A, et al. PD-1 and cancer: molecular mechanisms and polymorphisms. Immunogenetics 2018;70:73-86. [Crossref] [PubMed]
- Reck M, Rodríguez-Abreu D, Robinson AG, et al. Pembrolizumab versus Chemotherapy for PD-L1-Positive Non-Small-Cell Lung Cancer. N Engl J Med 2016;375:1823-33. [Crossref] [PubMed]
- Yarchoan M, Hopkins A, Jaffee EM. Tumor Mutational Burden and Response Rate to PD-1 Inhibition. N Engl J Med 2017;377:2500-1. [Crossref] [PubMed]
- Shi L, Lu J, Zhong D, et al. Clinicopathological and predictive value of MAIT cells in non-small cell lung cancer for immunotherapy. J Immunother Cancer 2023;11:e005902. [Crossref] [PubMed]
- Liu H, Luo Y, Zhao S, et al. A reactive oxygen species-related signature to predict prognosis and aid immunotherapy in clear cell renal cell carcinoma. Front Oncol 2023;13:1202151. [Crossref] [PubMed]
- Li Y, Gong B, Lou J, et al. Association between thymus density loss and efficacy in non-small cell lung cancer patients treated with immune checkpoint inhibitors. Transl Lung Cancer Res 2024;13:1544-58. [Crossref] [PubMed]
- Mantovani A, Allavena P, Sica A, et al. Cancer-related inflammation. Nature 2008;454:436-44. [Crossref] [PubMed]
- Dai J, Su Y, Zhong S, et al. Exosomes: key players in cancer and potential therapeutic strategy. Signal Transduct Target Ther 2020;5:145. [Crossref] [PubMed]
- Frisbie L, Buckanovich RJ, Coffman L. Carcinoma-Associated Mesenchymal Stem/Stromal Cells: Architects of the Pro-tumorigenic Tumor Microenvironment. Stem Cells 2022;40:705-15. [Crossref] [PubMed]
- Quail DF, Amulic B, Aziz M, et al. Neutrophil phenotypes and functions in cancer: A consensus statement. J Exp Med 2022;219:e20220011. [Crossref] [PubMed]
- Sica A, Mantovani A. Macrophage plasticity and polarization: in vivo veritas. J Clin Invest 2012;122:787-95. [Crossref] [PubMed]
- Yukawa T, Shimizu K, Maeda A, et al. Cyclooxygenase-2 genetic variants influence intratumoral infiltration of Foxp3-positive regulatory T cells in non-small cell lung cancer. Oncol Rep 2015;33:74-80. [Crossref] [PubMed]
- Raskov H, Orhan A, Christensen JP, et al. Cytotoxic CD8(+) T cells in cancer and cancer immunotherapy. Br J Cancer 2021;124:359-67. [Crossref] [PubMed]
- Yamamoto T, Kawada K, Obama K. Inflammation-Related Biomarkers for the Prediction of Prognosis in Colorectal Cancer Patients. Int J Mol Sci 2021;22:8002. [Crossref] [PubMed]
- Jiang Y, Xu D, Song H, et al. Inflammation and nutrition-based biomarkers in the prognosis of oesophageal cancer: a systematic review and meta-analysis. BMJ Open 2021;11:e048324. [Crossref] [PubMed]
- Schobert IT, Savic LJ, Chapiro J, et al. Neutrophil-to-lymphocyte and platelet-to-lymphocyte ratios as predictors of tumor response in hepatocellular carcinoma after DEB-TACE. Eur Radiol 2020;30:5663-73. [Crossref] [PubMed]
- Tang J, Fu Y, Song Y, et al. Increasing serum complement component 1q is associated with worse prognosis in advanced non-small cell lung cancer treated with immune checkpoint inhibitors: a single-center, retrospective study. J Thorac Dis 2024;16:3251-9. [Crossref] [PubMed]
- Weng J, Huang J, Yu W, et al. Combination of albumin concentration and neutrophil-to-lymphocyte ratio for predicting overall survival of patients with non-small cell lung cancer. J Thorac Dis 2021;13:5508-16. [Crossref] [PubMed]
- Mandaliya H, Jones M, Oldmeadow C, et al. Prognostic biomarkers in stage IV non-small cell lung cancer (NSCLC): neutrophil to lymphocyte ratio (NLR), lymphocyte to monocyte ratio (LMR), platelet to lymphocyte ratio (PLR) and advanced lung cancer inflammation index (ALI). Transl Lung Cancer Res 2019;8:886-94. [Crossref] [PubMed]
- Muhammed A, Fulgenzi CAM, Dharmapuri S, et al. The Systemic Inflammatory Response Identifies Patients with Adverse Clinical Outcome from Immunotherapy in Hepatocellular Carcinoma. Cancers (Basel) 2021;14:186. [Crossref] [PubMed]
- Sánchez-Gastaldo A, Muñoz-Fuentes MA, Molina-Pinelo S, et al. Correlation of peripheral blood biomarkers with clinical outcomes in NSCLC patients with high PD-L1 expression treated with pembrolizumab. Transl Lung Cancer Res 2021;10:2509-22. [Crossref] [PubMed]
- Lin X, Deng H, Yang Y, et al. Peripheral Blood Biomarkers for Early Diagnosis, Severity, and Prognosis of Checkpoint Inhibitor-Related Pneumonitis in Patients With Lung Cancer. Front Oncol 2021;11:698832. [Crossref] [PubMed]
- Diem S, Schmid S, Krapf M, et al. Neutrophil-to-Lymphocyte ratio (NLR) and Platelet-to-Lymphocyte ratio (PLR) as prognostic markers in patients with non-small cell lung cancer (NSCLC) treated with nivolumab. Lung Cancer 2017;111:176-81. [Crossref] [PubMed]
- Fang T, Wang Y, Yin X, et al. Diagnostic Sensitivity of NLR and PLR in Early Diagnosis of Gastric Cancer. J Immunol Res 2020;2020:9146042. [Crossref] [PubMed]
- Young RJ, Tin AW, Brown NJ, et al. Analysis of circulating angiogenic biomarkers from patients in two phase III trials in lung cancer of chemotherapy alone or chemotherapy and thalidomide. Br J Cancer 2012;106:1153-9. [Crossref] [PubMed]
- Zhao L, Ji W, Zhang L, et al. Changes of circulating transforming growth factor-beta1 level during radiation therapy are correlated with the prognosis of locally advanced non-small cell lung cancer. J Thorac Oncol 2010;5:521-5. [Crossref] [PubMed]
- Zhu T, Zou X, Yang C, et al. Neutrophil extracellular traps promote gastric cancer metastasis by inducing epithelial-mesenchymal transition. Int J Mol Med 2021;48:127. [Crossref] [PubMed]
- Lin B, Du L, Li H, et al. Tumor-infiltrating lymphocytes: Warriors fight against tumors powerfully. Biomed Pharmacother 2020;132:110873. [Crossref] [PubMed]
- De Palma M, Biziato D, Petrova TV. Microenvironmental regulation of tumour angiogenesis. Nat Rev Cancer 2017;17:457-74. [Crossref] [PubMed]
- Menter DG, Kopetz S, Hawk E, et al. Platelet "first responders" in wound response, cancer, and metastasis. Cancer Metastasis Rev 2017;36:199-213. [Crossref] [PubMed]
- Truffi M, Sottotetti F, Gafni N, et al. Prognostic Potential of Immune Inflammatory Biomarkers in Breast Cancer Patients Treated with Neoadjuvant Chemotherapy. Cancers (Basel) 2022;14:5287. [Crossref] [PubMed]
- Kang J, Zhang C, Zhong WZ. Neoadjuvant immunotherapy for non-small cell lung cancer: State of the art. Cancer Commun (Lond) 2021;41:287-302. [Crossref] [PubMed]
- Ramos-Casals M, Brahmer JR, Callahan MK, et al. Immune-related adverse events of checkpoint inhibitors. Nat Rev Dis Primers 2020;6:38. [Crossref] [PubMed]
- Rizzo A, Ricci AD. Biomarkers for breast cancer immunotherapy: PD-L1, TILs, and beyond. Expert Opin Investig Drugs 2022;31:549-55. [Crossref] [PubMed]
- Rizvi NA, Hellmann MD, Snyder A, et al. Cancer immunology. Mutational landscape determines sensitivity to PD-1 blockade in non-small cell lung cancer. Science 2015;348:124-8. [Crossref] [PubMed]
- Anagnostou V, Bardelli A, Chan TA, et al. The status of tumor mutational burden and immunotherapy. Nat Cancer 2022;3:652-6. [Crossref] [PubMed]
- Hinterleitner C, Strähle J, Malenke E, et al. Platelet PD-L1 reflects collective intratumoral PD-L1 expression and predicts immunotherapy response in non-small cell lung cancer. Nat Commun 2021;12:7005. [Crossref] [PubMed]
- Zhao S, Gong H, Liang W. Characterization of platelet-related genes and constructing signature combined with immune-related genes for predicting outcomes and immunotherapy response in lung squamous cell carcinoma. Aging (Albany NY) 2023;15:6969-92. [Crossref] [PubMed]
- Chen S, Zhang L, Chen L, et al. Comprehensive analysis of glycoprotein VI-mediated platelet activation signaling pathway for predicting pan-cancer survival and response to anti-PD-1 immunotherapy. Comput Struct Biotechnol J 2023;21:2873-83. [Crossref] [PubMed]
- Zhang G. Platelet-Related Molecular Subtype to Predict Prognosis in Hepatocellular Carcinoma. J Hepatocell Carcinoma 2022;9:423-36. [Crossref] [PubMed]
- Song Y, Tian J, Yang L, et al. Prognostic value of preoperative platelet-related parameters and plasma fibrinogen in patients with non-muscle invasive bladder cancer after transurethral resection of bladder tumor. Future Oncol 2022;18:2933-42. [Crossref] [PubMed]
- Deng D, Li X, Qi T, et al. A novel platelet risk score for stratifing the tumor immunophenotypes, treatment responses and prognosis in bladder carcinoma: results from real-world cohorts. Front Pharmacol 2023;14:1187700. [Crossref] [PubMed]
- Liu T, Wang J, Liu Y, et al. Prediction of the Therapeutic Effects of Pembrolizumab and Nivolumab in Advanced Non-Small Cell Lung Cancer by Platelet-Derived Microparticles in Circulating Blood. Technol Cancer Res Treat 2021;20:1533033821997817. [Crossref] [PubMed]
- Schmied L, Höglund P, Meinke S. Platelet-Mediated Protection of Cancer Cells From Immune Surveillance - Possible Implications for Cancer Immunotherapy. Front Immunol 2021;12:640578. [Crossref] [PubMed]
- Goubran HA, Burnouf T, Radosevic M, et al. The platelet-cancer loop. Eur J Intern Med 2013;24:393-400. [Crossref] [PubMed]
- Peterson JE, Zurakowski D, Italiano JE Jr, et al. VEGF, PF4 and PDGF are elevated in platelets of colorectal cancer patients. Angiogenesis 2012;15:265-73. [Crossref] [PubMed]