Identifying genetically-supported drug repurposing targets for non-small cell lung cancer through mendelian randomization of the druggable genome
Highlight box
Key findings
• We identified druggable genes for non-small cell lung cancer (NSCLC), including CD33, ENG, ICOSLG and IL18R1 for lung adenocarcinoma and VSIR, FSTL1 and TIMP2 for lung squamous cell carcinoma.
What is known and what is new?
• Targeted therapy has made promising advancements in systemic treatment for NSCLC over the last two decades. Current established targets include epidermal growth factor receptor (EGFR), Kirsten rat sarcoma viral oncogene (KRAS), anaplastic lymphoma kinase (ALK), c-Ros oncogene 1 (ROS1), v-Raf murine sarcoma viral oncogene homolog B (BRAF), mesenchymal-to-epithelial transition (MET), and rearranged during transfection (RET), which has benefited numerous patients with these gene alterations.
• There is an urgent need to explore other potential drug targets to expand the beneficiary group. This study identified several potential targets using the mendelian randomization of the druggable genomes.
What is the implication, and what should change now?
• Drugs with genetic support are more likely to be effective and successful in clinical trials. We provide compelling genetic evidence to prioritize drug development in NSCLC.
Introduction
Lung cancer is the second most common cancer and the leading cause of cancer-related death worldwide (1). Despite the declining morbidity and mortality rates attributable to tobacco control measures, the incidence and prevalence of lung cancer remain high (2).
In recent years, the clinical application of targeted therapy has significantly extended the survival time of lung cancer patients, particularly those with non-small cell lung cancer (NSCLC) (3). NSCLC is characterized by possessing various genetic drivers that tyrosine kinase inhibitors can specifically target. Established actionable drivers include the epidermal growth factor receptor (EGFR), Kirsten rat sarcoma viral oncogene (KRAS), anaplastic lymphoma kinase (ALK), c-Ros oncogene 1 (ROS1), v-Raf murine sarcoma viral oncogene homolog B (BRAF), mesenchymal-to-epithelial transition (MET), and rearranged during transfection (RET) (4-9). Each of these gene alterations plays a crucial role in driving tumor growth and progression at the genetic level, and tyrosine kinase inhibitors tailored toward these genes have significantly improved patient outcomes by cutting off the driving power behind tumorigenesis. However, most targeted drugs under development have failed in preclinical trials, leading to a significant waste of resources. It is estimated that the average cost of successfully developing a new drug is up to $1.3 billion (10). Therefore, there is an urgent need to develop cost-effective methods to identify gene targets with greater potential for clinical success before devoting resources to clinical trials of their targeted drugs.
With the advancement of genomics, the concept of the “druggable genome” has been proposed. This concept encompasses human genes that encode drug targets, including proteins targeted by approved or drugs in clinical-trial phase, proteins similar to approved drug targets, and proteins accessible to monoclonal antibodies or drug-like small molecules in vivo (11). Drugs supported by genetic evidence are more likely to succeed in clinical treatment (12). Genome-wide association studies (GWAS) have been applied in drug development, increasing the proportion of preclinical stage drugs from 2.0% to 8.2% (13). However, GWAS could not directly illustrate the causal effects of genes or reliably pinpoint novel drug targets.
Two-sample mendelian randomization (MR) study is a novel approach that can evaluate causality between two traits by using genetic variants [single nucleotide polymorphisms (SNPs)] as instrumental variables (IVs). These SNPs are randomly allocated at conception and are largely independent of confounders, including environmental and other genetic variants. Therefore, the MR study may have a similar impact on evaluating causality as randomized controlled trials (14,15). A trait determined by multiple genes, each having a minor effect on the trait, is known as a quantitative trait loci (QTL). SNPs related to the expression level of druggable genes or the circulating level of encoded proteins are named expression quantitative trait locus (eQTL) and protein quantitative trait locus (pQTL), respectively. The protein encoded by eQTL or pQTL might be analogous to a lifelong exposure that can be targeted by medication (16).
This study aimed to identify the expression levels of druggable genes and the circulating level of encoded proteins to predict the efficacy and prognostic value of the corresponding targeted drugs in NSCLC. Our research seeks to enhance the understanding of genetically repurposed drug targets for NSCLC and explore candidate druggable genes with causal association to NSCLC. We present this article in accordance with the STROBE-MR reporting checklist (available at https://tlcr.amegroups.com/article/view/10.21037/tlcr-24-65/rc) (17).
Methods
Ethical approval
As this study used publicly available data, requirement for separate ethical approval was waived, and the study was conducted in accordance with the Declaration of Helsinki (as revised in 2013).
Study design
Figure 1 illustrates the entire research design scheme. We employed a two-sample MR design to identify eQTL and pQTL and predict the efficacy of the corresponding targeted drugs on NSCLC. To investigate the potential effects of druggable genes on different histological types of NSCLC, analyses were performed separately for lung adenocarcinoma (LUAD) and lung squamous cell carcinoma (LUSC).
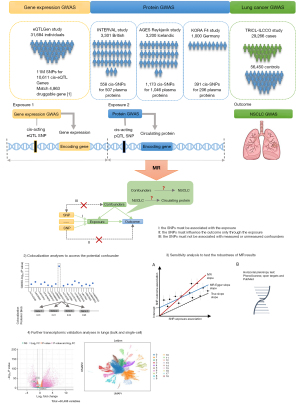
Data sources
SNPs located within 1 Mb upstream and downstream of druggable genes (cis-SNPs) provide more credible genetic evidence for these genes (18). GWAS summary data of cis-eQTL were obtained from the eQTLGen consortium (https://eqtlgen.org/) (18). We obtained data on 4,863 druggable genes from the original publication (19) and selected independent cis-eQTL (r2≤0.001) related SNPs that are significantly associated with each druggable gene (P<5×10−8).
SNPs associated with circulating protein levels could model drug target effects (20). We identified three studies that met the following criteria: (I) reported significant pQTLs in individuals of European descent; (II) provided all the SNP information required for MR; and (III) included SNPs relevant to NSCLC outcomes. We obtained three independent pQTL GWAS summary datasets from INTERVAL, AGES Reykjavik, and KORA F4 studies, respectively (21-23). These large-scale pQTL studies (21-23) found that the genetic determinants of circulating proteins are located in cis to the encoding genes. This is because cis-acting SNPs strongly associated with the encoding gene tend to influence the gene’s transcription and circulating protein level. The location proximity from each gene boundary to determine the cis-pQTL-related SNPs among the three studies were 10, 1 and 0.3 Mb in Suhre et al. (23), Sun et al. (21) and Emilsson et al. (22) respectively. The GWAS summary data for NSCLC were obtained from the Transdisciplinary Research In Cancer of the Lung and the International Lung Cancer Consortium (TRICL-ILCCO) and the Lung Cancer Cohort Consortium (LC3) (24). These data represent the meta-analysis results aimed at identifying new lung cancer susceptibility loci.
Statistical analysis
MR study is based on three main assumptions: (I) the genetic variant(s) must be reliably associated with the exposure; (II) the genetic variant(s) must influence the outcome only through the exposure of interest; and (III) the genetic variant(s) must be independent of any measured or unmeasured confounders.
The same SNPs associated with the exposure can be extracted from NSCLC GWAS data to evaluate the causal effect. MR analyses were performed using the R package (Version 4.2.0) “TwoSampleMR” (Version 0.5.6) (25). Clumping was performed under linkage disequilibrium (LD) conditions with r2<0.001 and 10,000 kb using European samples from the 1000 Genomes Project panel 3 of European-ancestry (26). The exposure and outcome data were harmonized using in-built functions. The Wald estimator (βoutcome/βexposure) was used for single cis-SNPs, while multiple random-effect inverse variance weighted (IVW) analysis was used for multiple cis-SNPs (27). Bonferroni correction was applied to adjust for multiple eQTL SNPs (adjusted P≤0.05/4,863).
Candidate NSCLC-influencing druggable genes supported by MR were evaluated via colocalization analyses using the coloc R package (28). Colocalization analysis assesses the probability that two traits share the same causal variant, rather than the variant being coincidentally shared due to correlation through LD (28). Default prior was used, including p1=10−4, p2=10−4, and p12=10−5, where p1, p2 and p12 represent the prior probabilities that a SNP in the tested region is significantly associated with the exposure, the outcome, or both, respectively. The colocalization analysis yields posterior probabilities corresponding to one of the five hypotheses: PPH0, no association with either trait; PPH1, association with the exposure but not the outcome; PPH2, association with the outcome, but not the exposure; PPH3, association with both traits with distinct causal variants; and PPH4, association with both traits with a shared causal variant (29). The formula PPH4/(PPH3 + PPH4) is often used to represent the probability of PPH4 when only a few SNPs are included in the colocalization analysis (30). For colocalization, regions within 1 Mb of the SNPs were selected.
Sensitivity analysis
Sensitivity analyses were performed for multiple cis-SNPs following the MR analyses. Cochran’s Q and I2 methods were used to assess the overall heterogeneity among Wald ratios (31). The MR-Egger regression method relaxes the assumption by not restricting the y-intercept. Directional pleiotropy is indicated if the MR-Egger y-intercept significantly deviates from zero. If more than two SNPs were available, pleiotropic effects were evaluated using the MR-Egger regression method (32). To further detect the presence of horizontal pleiotropy, each cis-SNP of the druggable genes was examined using PhenoScanner (33) and Open Targets (34). The parameters set in the PhenoScanner database were “P value <5E−8, Proxies = EUR, r2≥0.8 and Build 37” for gene expression, proteins, traits, and diseases. MR analyses were then performed between these traits and NSCLC to explore potential confounders. Further assessment for potential horizontal pleiotropy and external validation of druggable genes was conducted by searching Open Targets (34). In the search result for Open Targets datasets, none of the traits related to these SNPs were associated with NSCLC. To verify whether the causal direction from the exposure to the outcome in MR results, the “mr_steiger” function in the “TwoSampleMR” package was used. The SNPs were removed if the direction was “FALSE”. Bidirectional MR was performed to assess the orientation of causality.
Transcriptomic RNA sequencing (RNA-seq) data from lung tissue
The bulk RNA-seq analysis of The Cancer Genome Atlas (TCGA) pre-processed counts and fragments per kilobase million (FPKM) RNA-seq datasets for LUAD and LUSC, as well as the clinical information of each sample, were obtained from UCSC Xena platform (https://xenabrowser.net/datapages/) (35). The FPKM values were converted to the transcripts per kilobase million (TPM) value for further visualization and survival analysis. Differentially expressed genes (DEGs) were determined using R package “DESeq2” (36), all DEG analyses were conducted by comparing tumor tissue to normal tissue. Genes with |log2FoldChange| >1 and adjusted P value <0.05 were considered as DEGs. The high- and low-expression groups were divided by the median of the TPM value of specific genes for subsequent survival analysis. Overall survival curves were constructed using the Kaplan-Meier methodology, and log-rank tests were performed to identify survival-related genes, with P value <0.05 was considered as statistically significant.
We further analyzed single-cell RNA sequencing (scRNA-seq) data. The Lambrechts et al. dataset (37) was downloaded from BioStudies website (https://www.ebi.ac.uk/biostudies/arrayexpress/studies/E-MTAB-6149). Raw scRNA-seq data were processed using CellRanger (v7.0.1, 10xGenomics). Reads were aligned to the human genome to produce gene counts across barcodes, with all CellRanger parameters set to default. Quality control (QC) and clustering were performed using Scanpy (v1.9) (38) in Python 3.8. The QC procedure was based on gene numbers, the percentage of mitochondrial genes, and ribosomal genes to remove cells with low-quality RNA-seq data. Basic filtering included genes expressed in ≥20 cells and cells with at least 200 detected genes. Doublets were removed using Scrublet (39) software. The origin counts were converted to counts per million (CPM) values and normalized for further analysis. The “pp.highly_variable_genes” function in Scanpy was used to screen the highly variable genes for Uniform Manifold Approximation and Projection (UMAP) dimension reduction. The Leiden method was adopted for clustering. Malignant cancer cells were identified using infercnvpy (https://github.com/broadinstitute/inferCNV). The marker genes of each cell population were determined using the “tl.rank_genes_groups” function in Scanpy. Differential expression of the druggable genes was evaluated by Kruskal-Wallis’ test with Dunn post hoc tests. P value was adjusted using Bonferroni method.
Results
Cohort data source
The GWAS summary data for eQTL were obtained from the eQTLGen study (18), which incorporated 37 datasets comprising a total of 31,684 individuals and 11 million SNPs. SNPs related to 4,863 druggable genes were used to model the exposure to medicines targeting the encoded proteins (19). The GWAS summary data for circulating proteins from the INTERVAL study by Sun et al. (21), included 3,301 participants of European descent from England. The GWAS summary data for circulating protein from the AGES Reykjavik study by Emilsson et al. (22), consisted of 3,200 Icelanders. The GWAS summary data for circulating protein from the KORA F4 study (23) included 1,000 Germans (Table S1).
The GWAS summary data for NSCLC were obtained from the TRICL-CCO and LC3 consortia (24). In total, 29,266 cases and 56,450 controls were included in the analyses. These data were genotyped using the OncoArray, followed by imputation and meta-analysis, with detailed procedures described previously (24). In this study, LUAD and LUSC were the two major histological subgroups in NSCLC, comprising 11,273 cases versus 55,483 controls, and 7,426 cases versus 55,627 controls, respectively. Demographic characteristics can be found in Table S2.
Statistical analysis
After anchoring 4,863 druggable genes using cis-SNPs from eQTLGen (18), the cis-SNPs of eQTL were used for MR scanning analyses in LUAD and LUSC respectively. In total, 2,491 druggable genes with available SNPs were included in the MR analyses. Of these, 106 and 136 druggable genes were causally related to LUAD and LUSC respectively (Bonferroni adjusted P value ≤1.03×10−5). All analysis results are presented in the online tables (available at https://cdn.amegroups.cn/static/public/tlcr-24-65-1.xlsx, and https://cdn.amegroups.cn/static/public/tlcr-24-65-2.xlsx). All the cis-eQTL SNPs included in this study can be found in the online tables (available at https://cdn.amegroups.cn/static/public/tlcr-24-65-3.xlsx, and https://cdn.amegroups.cn/static/public/tlcr-24-65-4.xlsx). A total of 1,072 proteins, 453 of which were overlapping, were used for MR scanning analyses. According to MR estimates (Wald ratio or IVW) with a nominal P value <0.05, a total of 139 and 146 proteins were causally associated with LUAD and LUSC, respectively. The results can be found in the online tables (available at https://cdn.amegroups.cn/static/public/tlcr-24-65-5.xlsx, and https://cdn.amegroups.cn/static/public/tlcr-24-65-6.xlsx), while the cis-pQTL SNPs included in this study were available at https://cdn.amegroups.cn/static/public/tlcr-24-65-7.xlsx. By intersecting the data to establish a strict causal association at both the eQTL and pQTL levels, seven candidate druggable genes were confirmed, including CD33, ENG, ICOSLG, IL18R1 in LUAD and VSIR (also named C10orf54 in the original study), FSTL1, TIMP2 in LUSC. All these confirmed genes exhibited the same directional effect at both eQTL and pQTL level, except for ICOSLG and TIMP2 (Figure 2 and Table S3). For instance, a 1 − standard-deviation (1 − SD) genetically determined increase in CD33 expression and circulating protein levels was associated with an average 12% and 2% lower risk of developing LUAD, respectively (OR =0.88; 95% CI: 0.84–0.92; P=2×10−8; and OR =0.98; 95% CI: 0.97–0.99; P=0.02) (Figure 2). MR evidence for ICOSLG in LUAD was inconsistent between gene expression level (OR =1.05; 95% CI: 1.04–1.06; P=1.39×10−30) and circulating protein level (OR =0.992; 95% CI: 0.987–0.999; P=0.02). The causal effect of TIMP2 in LUSC was also divided (OR =1.27; 95% CI: 1.14–1.41; P=7.27×10−6 at the gene expression level; OR =0.83; 95% CI: 0.72–0.95; P=0.007 at the protein level). Detailed results can be found in the online tables (available at https://cdn.amegroups.cn/static/public/tlcr-24-65-1.xlsx, and https://cdn.amegroups.cn/static/public/tlcr-24-65-6.xlsx). It is worth mentioning that CD33 and VSIR were causally related to both LUAD and LUSC at the gene expression level while ENG was causally associated with both LUAD and LUSC at the protein level (Figure 2 and Table S3).
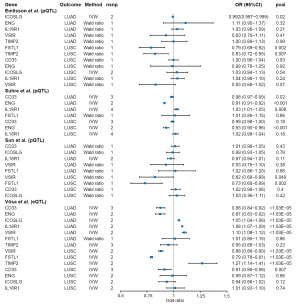
We performed colocalization analyses based on the GWAS summary data of the eQTL and pQTL for CD33, ENG, ICOSLG, IL18R1 VSIR, FSTL1 and TIMP2 to assess potential confounding due to LD. All these candidate genes were well colocalized with LUAD and LUSC using the coloc package (28) and the formula PPH4/(PPH3 + PPH4) with the lowest posterior probability being 98.98% (Tables 1,2).
Table 1
Gene | Outcome (type) | eQTL GWAS | pQTL GWAS | |||||||||
---|---|---|---|---|---|---|---|---|---|---|---|---|
Sign with Wald or IVW | Cochran’s Q test | I2 test | pQTL evidence | MR-Egger_intercept | Sign with Wald or IVW | Cochran’s Q test |
I2 test | MR-Egger_intercept | Coloc | |||
CD33 | LUAD | √ | √ | × | √ | √ | √ | √ | √ | √ | √ | |
ENG | LUAD | √ | √ | √ | √ | NA | √ | √ | × | NA | √ | |
ICOSLG | LUAD | √ | √ | √ | √ | NA | √ | √ | √ | NA | √ | |
IL18R1 | LUAD | √ | √ | × | √ | NA | √ | √ | √ | √ | √ | |
VSIR | LUSC | √ | √ | √ | √ | NA | √ | √ | NA | NA | √ | |
FSTL1 | LUSC | √ | √ | × | √ | NA | √ | √ | NA | NA | √ | |
TIMP2 | LUSC | √ | √ | √ | √ | NA | √ | √ | NA | NA | √ |
The Wald ratio method was used when SNP =1, IVW method for SNP ≥2. These drugs are either approved or in clinical trial phase, the drug-gene interactions based on https://dgidb.org, Indications and Clinical phase based on https://clinicaltrials.gov/, the mechanisms and direction of effect were confirmed in https://go.drugbank.com/. √, pass; ×, fail to test. Coloc, colocalization; LUAD, lung adenocarcinoma; LUSC, lung squamous cell carcinoma; IVW, inverse variance weighted; MR, Mendelian randomization; eQTL, expression quantitative trait locus; GWAS, genome-wide association study; pQTL, protein quantitative trait locus; Sign, significant; SNP, single nucleotide polymorphism; NA, not applicable.
Table 2
Gene | PhenoScanner | Drug name | Query score | Interaction score | Indications/usages | Clinical phase | ClinicalTrials.gov identifier/PMID | Supplement | ||
---|---|---|---|---|---|---|---|---|---|---|
CD33 | √ | Lintuzumab (SGN-33) | 8.61 | 20.61 | Acute myeloid leukemia | Phase 2 | NCT00528333 | |||
Myelodysplastic syndrome | Phase 1 | NCT00502112 | ||||||||
M195 | 4.3 | 10.3 | Relapsed and refractory myeloid leukemia | Phase 1B | 8142644 | |||||
AMV-564 (CNTO-3953) | 4.3 | 10.3 | Acute myeloid leukemia | Phase 1 | NCT03144245 | |||||
Locally advanced or metastatic solid tumors | Phase 1 | NCT04128423 | ||||||||
Myelodysplastic syndrome | Phase 1 | NCT03516591 | ||||||||
Oncolysin M | 4.3 | 10.3 | NA | NA | NA | |||||
Vadastuximab talirine (SGN-CD33A; 33A) | 4.3 | 10.3 | Acute myeloid leukemia | Phase 3 | NCT02785900 | Terminated (due to safety; specifically, a higher rate of deaths, including fatal infections, in the SGN33A arm versus the control arm) | ||||
Myelodysplastic syndrome | Phase 2 | NCT02706899 | Terminated (sponsor decision based on portfolio prioritization) | |||||||
Gemtuzumab ozogamicin | 2.15 | 5.15 | Acute myeloblastic leukemia | Phase 4 | NCT01041040 | |||||
ENG | × | Carotuximab (TRC105) | 4.3 | 61.83 | Non-small cell lung cancer | Phase 1 | NCT05401110 | |||
Castration-resistant prostate cancer | Phase 2 | NCT05534646 | ||||||||
Advanced soft tissue sarcoma | Phase 2 | NCT01975519 | ||||||||
ICOSLG | √ | NA | NA | NA | NA | NA | NA | |||
IL18R1 | √ | NA | NA | NA | NA | NA | NA | |||
VSIR | √ | Onvatilimab (JNJ-61610588) | 4.3 | 61.83 | Advanced cancer | Phase 1 | NCT02671955 | Terminated (Janssen business decision) | ||
FSTL1 | √ | NA | NA | NA | NA | NA | NA | |||
TIMP2 | √ | NA | NA | NA | NA | NA | NA |
√, pass; ×, fail to test. NA, not applicable.
Sensitivity analyses
After validating the druggable genes, we conducted sensitivity analyses to assess potential heterogeneity and pleiotropy caused by confounders. All sensitivity analyses of druggable genes are shown in Table 2, with detailed results can be found in Table S4. All the genes passed Cochran’s Q test (P>0.05). The MR-Egger intercept estimates for CD33 and IL18R1 were close to the null, suggesting no evidence of directional pleiotropy (P>0.05). However, CD33, IL18R1 and FSTL1 showed bias with I2>0.50. Bidirectional MR, used as a sensitivity analysis to assess the correct orientation of MR estimates, provided no evidence that NSCLC influences these gene expression levels or protein levels in this study. The PhenoScanner dataset (33) identified some traits associated with druggable genes such as CD33, ENG, IL18R1 and VSIR. For example, cis-SNP rs10421385 of CD33 was closely related to the expression level of SIGLEC20P, along with rs12459419 associated with SIGLEC22P and SIGLECL1. The cis-SNP rs651007 of ENG was associated with multiple traits, including angiopoietin-1 receptor, beta-1,4-galactosyltransferase 1, and lithostathine-1-alpha. Detailed SNP-related traits can be found in the online table (available at https://cdn.amegroups.cn/static/public/tlcr-24-65-8.xlsx). Since we used cis-SNPs for these candidate genes, these pleiotropic effects on other molecules were more likely to represent vertical pleiotropy, where SNPs affect levels of other molecules. However, vertical pleiotropy does not violate the assumptions of MR. Hence, we conducted MR analyses between these traits (as exposure) and NSCLC (as outcome) to reduce the possibility of MR estimates bias caused by horizontal pleiotropy. When the MR estimated the expression levels of SIGLEC20P, SIGLEC22P and SIGLECL1 on LUAD, none of these traits showed evidence of a causal effect on LUAD (P value for Wald ratio were: 0.14, 0.58 and 0.43, respectively). We did find some traits causally associated with LUAD, including: ABO, angiopoietin-1 receptor, and beta-1,4-galactosyltransferase 1, rs651007 and rs8176749 were identified for these traits. Detailed results can be found in the online table (available at https://cdn.amegroups.cn/static/public/tlcr-24-65-9.xlsx).
Transcriptomic RNA sequencing analyses in lung tissue
In the bulk-RNA-seq based transcriptomic data from the TCGA dataset, the majority of candidate druggable genes were found to be differentially expressed between tumor and normal tissue, except for ICOSLG in LUAD and FSTL1 in LUSC. In the LUAD dataset, CD33, ENG and IL18R1 were significantly down-regulated in tumor tissue. In the LUSC dataset, VSIR and TIMP2 were significantly down-regulated in tumor tissue (Figure S1A,S1B and Table S5). Kaplan-Meier survival analysis revealed that in LUAD, lower expression levels of CD33 and ENG were associated with poor prognosis (log-rank test P=0.006 for CD33 and 0.021 for ENG) (Figure 3), other genes-related survival analysis results can be found in Figure S2. In LUSC, higher expression levels of TIMP2 indicated poor prognosis (log-rank test P=0.02) (Figure 3). Therefore, CD33, ENG, and TIMP2 could serve as prognostic factors. ScRNA-seq analysis revealed that in certain cell clusters of the tumor microenvironment, candidate druggable genes showed significantly differential expression between tumor and normal tissue. This evidence further confirmed the drug-targeting potential of genes screened by MR methods.
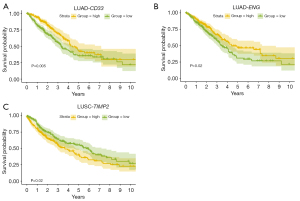
Single-cell RNA sequencing data of lung tissue
Because of the lack of cellular-resolution in bulk RNA-seq analysis, we further evaluate the expression of the DEGs in scRNA-seq dataset (Figures S3-S5 and table available at https://cdn.amegroups.cn/static/public/tlcr-24-65-10.xlsx). The dataset from Lambrechts et al. (37) included 6 LUAD samples, 6 LUSC samples, and 4 normal tissue samples. ScRNA-seq analysis revealed that in LUAD, candidate druggable genes were mainly expressed in alveolar macrophages [with high expression of MACRO, FABP4 and MCEMP1 (40)], endothelial cells, fibroblasts, mast cells, and monocyte-derived macrophages (40) (Figures S5A,S6). In some of these cells, druggable genes showed significantly differential expression. For instance, the expression level of IL18R1 was lower in tumor tissue compared to normal tissue in both mast cells and endothelial cells (adjusted P value =6.79×10−14 for mast cell and 1.22×10−8 for endothelial cells). CD33 was lower expressed in monocyte-derived macrophages in tumor tissue (adjusted P value =6.16×10−12). However, ENG was highly expressed in endothelial cells in tumor tissue (adjusted P value =1.51×10−24) (available at https://cdn.amegroups.cn/static/public/tlcr-24-65-10.xlsx). This challenged the previous conclusion obtained from bulk RNA-seq analysis; one possible explanation is the much lower abundance of alveolar macrophage in tumor tissue, leading to the overall low expression levels of ENG and CD33. In LUSC, candidate druggable genes were mainly expressed in non-tumor epithelial cells, monocyte-derived macrophage clusters [where APOE and C1QB were highly expressed, C1Q+ macrophages (41)], monocyte-derived macrophage cluster (where CD163 was lowly expressed), alveolar macrophages, dendritic cells (DCs), and fibroblasts (Figures S5B,S7). Intriguingly, TIMP2 was significantly lower expressed in cancer cells compared to non-tumor epithelial cells (adjusted P value =8.91×10−6), suggesting that TIMP2 may play a role in tumorigenesis and tumor cells growth. TIMP2 was also expressed at lower levels in the macrophage cluster (adjusted P value =1.54×10−30), alveolar macrophages (adjusted P value =2.01×10−13) and DCs (adjusted P value =0.13). VSIR was down-regulated in the macrophage cluster as well (adjusted P value =8.10×10−7). Overall, targeting TIMP2 may directly affect the tumor cell in LUSC. Other druggable genes may play their roles by intervening with other tumor microenvironment components in both LUAD and LUSC.
Discussion
We conducted a two-sample MR analysis to simulate the causal effect of druggable genes on LUAD and LUSC using accessible GWAS summary data from multiple consortia. Our findings provide strong MR evidence for the repurposing value of candidate druggable genes in NSCLC, including CD33, ENG, IL18R1 in LUAD and VSIR, FSTL1 in LUSC (Table 1 and Figure 2).
We identified two genes encoding the targets with existing drugs that warrant further discussion by searching DGIdb (42), Clinicaltrials, DrugBank (43) and PubMed. All results are summarized in Table 1. Gemtuzumab ozogamicin is a recombinant humanized IgG4 kappa antibody against CD33 conjugated with a calicheamicin derivative, a cytotoxic antitumor antibiotic isolated from the fermentation of Micromonospora echinospora ssp. (44). The Food and Drug Administration (FDA) approved this drug on May 17, 2000, as a first-line treatment for patients aged 60 years or older with CD33-positive acute myeloid leukemia (AML) (45). Subsequent studies have made significant progress in treating AML by targeting CD33 in combination with other targets, such as CD123 (46) and CAR-T cells (47). Our MR results showed that increased expression of CD33 at the gene level can reduce the risk of NSCLC, a finding confirmed by transcriptome analysis. ScRNA-seq revealed that CD33 is lowerly expressed in monocyte-derived macrophages of LUAD tumor tissue. However, the efficacy of the drugs targeting CD33 in NSCLC and the specific mechanisms involved remain inexplicit. Carotuximab (TRC105) is a drug targeting the protein encoded by ENG, which is currently in phase I clinical trials and is also being investigated in NSCLC (NCT05401110). Other drugs or targets are either in the clinical trial stage or awaiting investigation (Table 1). Despite advancements in targeted therapy, discovering new genetic targets remains challenging. Many barriers to the clinical success of targeted drugs include tumor heterogeneity, genetic complexity, and technological limitations.
Since most clinically successful drugs target proteins rather than gene expression, genetic variants associated with protein levels may more accurately resemble drug target effects than eQTLs. Therefore, MR results for protein levels were prioritized for evaluation (20). Consequently, the MR evidence for ICOSLG in LUAD at the protein level is considered more significant.
When conducting a PhenoScanner search to assess potential confounders, we found that CD33, IL18R1 and VSIR showed no evidence of horizontal pleiotropy. However, ENG influenced LUAD through diverse pathways (also known as confounders), such as ABO (48), angiopoietin-1 receptor (49), and beta-1,4-galactosyltransferase 1 (21). These analyses allowed us to explore pleiotropy due to confounders (28,32,50).
The two-sample MR design allows us to explore the expression levels and circulating proteins levels of candidate genes in LUAD (18,21-23) and LUSC (24). Each type of NSCLC has its own biological characteristics, suggesting that different druggable genes may have different causal effects. Notably, some candidate druggable genes were also supported by transcriptomic data. Moreover, CD33, ENG and TIMP2 showed differences in the survival curves, and the direction of the effect was consistent with MR results. Therefore, the prognostic value of these genes in targeted therapy for NSCLC is worth further investigation.
Our study has several valuable advantages compared to previous MR studies. Firstly, the SNPs of eQTLs were sufficiently large to conduct robust MR analyses (18). Multiple sources of pQTL (21-23) could be mutually verified, and sufficient GWAS data were available when conducting external verification using PhenoScanner (33). Additionally, we further explored the role of druggable genes in different subtypes of the NSCLC, including LUAD and LUSC, since the current practices in LUAD and LUSC are significantly different. Secondly, this study employed a novel MR approach, using QTL-related SNPs as IVs and anchoring of the druggable gene sites to translate drug effects into gene-level effects (51). By utilizing genetic variants as IVs, MR can address confounding and reverse causation and provide more reliable insights into genetic associations. Lastly, the rapid development of genomics and the growth in publicly available druggable gene resources provide valuable genetic data for discovering novel drug targets (19,52,53). If genetic evidence from MR can shed more light on the clinical successful rate of potential targeted drugs and simplify the screening steps for the clinical trials, it could significantly lower the cost of drug development (16,54).
However, there are some limitations in this study. A key limitation is that MR cannot fully replicate the conditions of a randomized controlled trial (RCT). MR mimics lifelong low-dose exposure to a drug and assumes a linear relationship between exposure and outcome, whereas, RCTs typically investigate higher doses of a drug over a much shorter timeframe (55). The MR result may not directly correspond to the effect size in practice and does not perfectly predict the effect of a drug. Independent cohorts are required to validate scientific findings from the MR approach and eliminate false positives. Secondly, a preventative agent would need to have high tolerability and a reasonable safety profile. However, our approach is not well-suited for systematically evaluating the safety aspects of the proposed candidate genes in this study. Thirdly, the eQTL cohorts included some non-European individuals (18), and the sample size of the pQTL study is far smaller than that of the eQTL study (21-23). Larger sample sizes are needed to generate a better ability to detect QTLs. Lastly, the accuracy of the results is limited by the original data and the methodology since the causal link in this study is based on the secondary analysis of mixed models. Despite of the limitations, two-sample MR analysis is a time- and cost-effective adjuvant to RCTs, considering the current success rate for drugs proceeding from phase I trials to approval is 13.8% approximately (56).
Conclusions
Our research provides genetic evidence of druggable genes in NSCLC, and we hope that these data will serve as a valuable resource for prioritizing drug development efforts.
Acknowledgments
Funding: This study was supported by
Footnote
Reporting Checklist: The authors have completed the STROBE-MR reporting checklist. Available at https://tlcr.amegroups.com/article/view/10.21037/tlcr-24-65/rc
Peer Review File: Available at https://tlcr.amegroups.com/article/view/10.21037/tlcr-24-65/prf
Conflicts of Interest: All authors have completed the ICMJE uniform disclosure form (available at https://tlcr.amegroups.com/article/view/10.21037/tlcr-24-65/coif). W.L. serves as the unpaid Associate Editor-in-Chief of Translational Lung Cancer Research from May 2024 to April 2025. The other authors have no conflicts of interest to declare.
Ethical Statement: The authors are accountable for all aspects of the work in ensuring that questions related to the accuracy or integrity of any part of the work are appropriately investigated and resolved. The study was conducted in accordance with the Declaration of Helsinki (as revised in 2013).
Open Access Statement: This is an Open Access article distributed in accordance with the Creative Commons Attribution-NonCommercial-NoDerivs 4.0 International License (CC BY-NC-ND 4.0), which permits the non-commercial replication and distribution of the article with the strict proviso that no changes or edits are made and the original work is properly cited (including links to both the formal publication through the relevant DOI and the license). See: https://creativecommons.org/licenses/by-nc-nd/4.0/.
References
- Siegel RL, Giaquinto AN, Jemal A. Cancer statistics, 2024. CA Cancer J Clin 2024;74:12-49. [Crossref] [PubMed]
- Siegel RL, Miller KD, Fuchs HE, et al. Cancer statistics, 2022. CA Cancer J Clin 2022;72:7-33. [Crossref] [PubMed]
- Goldstraw P, Chansky K, Crowley J, et al. The IASLC Lung Cancer Staging Project: Proposals for Revision of the TNM Stage Groupings in the Forthcoming (Eighth) Edition of the TNM Classification for Lung Cancer. J Thorac Oncol 2016;11:39-51. [Crossref] [PubMed]
- Planchard D, Jänne PA, Cheng Y, et al. Osimertinib with or without Chemotherapy in EGFR-Mutated Advanced NSCLC. N Engl J Med 2023;389:1935-48. [Crossref] [PubMed]
- Wu YL, Dziadziuszko R, Ahn JS, et al. Alectinib in Resected ALK-Positive Non-Small-Cell Lung Cancer. N Engl J Med 2024;390:1265-76. [Crossref] [PubMed]
- Drilon A, Camidge DR, Lin JJ, et al. Repotrectinib in ROS1 Fusion-Positive Non-Small-Cell Lung Cancer. N Engl J Med 2024;390:118-31. [Crossref] [PubMed]
- Riely GJ, Smit EF, Ahn MJ, et al. Phase II, Open-Label Study of Encorafenib Plus Binimetinib in Patients With BRAF(V600)-Mutant Metastatic Non-Small-Cell Lung Cancer. J Clin Oncol 2023;41:3700-11. [Crossref] [PubMed]
- Riedel R, Fassunke J, Scheel AH, et al. MET Fusions in NSCLC: Clinicopathologic Features and Response to MET Inhibition. J Thorac Oncol 2024;19:160-5. [Crossref] [PubMed]
- Zhou C, Solomon B, Loong HH, et al. First-Line Selpercatinib or Chemotherapy and Pembrolizumab in RET Fusion-Positive NSCLC. N Engl J Med 2023;389:1839-50. [Crossref] [PubMed]
- Galkina Cleary E, Jackson MJ, Zhou EW, et al. Comparison of Research Spending on New Drug Approvals by the National Institutes of Health vs the Pharmaceutical Industry, 2010-2019. JAMA Health Forum 2023;4:e230511. [Crossref] [PubMed]
- Minikel EV, Painter JL, Dong CC, et al. Refining the impact of genetic evidence on clinical success. Nature 2024;629:624-9. [Crossref] [PubMed]
- Su WM, Gu XJ, Dou M, et al. Systematic druggable genome-wide Mendelian randomisation identifies therapeutic targets for Alzheimer's disease. J Neurol Neurosurg Psychiatry 2023;94:954-61. [Crossref] [PubMed]
- Rasooly D, Peloso GM, Pereira AC, et al. Genome-wide association analysis and Mendelian randomization proteomics identify drug targets for heart failure. Nat Commun 2023;14:3826. [Crossref] [PubMed]
- Zheng G, Chattopadhyay S, Sundquist J, et al. Antihypertensive drug targets and breast cancer risk: a two-sample Mendelian randomization study. Eur J Epidemiol 2024;39:535-48. [Crossref] [PubMed]
- Bourgault J, Abner E, Manikpurage HD, et al. Proteome-Wide Mendelian Randomization Identifies Causal Links Between Blood Proteins and Acute Pancreatitis. Gastroenterology 2023;164:953-965.e3. [Crossref] [PubMed]
- Ochoa D, Karim M, Ghoussaini M, et al. Human genetics evidence supports two-thirds of the 2021 FDA-approved drugs. Nat Rev Drug Discov 2022;21:551. [Crossref] [PubMed]
- Skrivankova VW, Richmond RC, Woolf BAR, et al. Strengthening the Reporting of Observational Studies in Epidemiology Using Mendelian Randomization: The STROBE-MR Statement. JAMA 2021;326:1614-21. [Crossref] [PubMed]
- Võsa U, Claringbould A, Westra HJ, et al. Large-scale cis- and trans-eQTL analyses identify thousands of genetic loci and polygenic scores that regulate blood gene expression. Nat Genet 2021;53:1300-10. [Crossref] [PubMed]
- Finan C, Gaulton A, Kruger FA, et al. The druggable genome and support for target identification and validation in drug development. Sci Transl Med 2017;9:eaag1166. [Crossref] [PubMed]
- Folkersen L, Gustafsson S, Wang Q, et al. Genomic and drug target evaluation of 90 cardiovascular proteins in 30,931 individuals. Nat Metab 2020;2:1135-48. [Crossref] [PubMed]
- Sun BB, Maranville JC, Peters JE, et al. Genomic atlas of the human plasma proteome. Nature 2018;558:73-9. [Crossref] [PubMed]
- Emilsson V, Ilkov M, Lamb JR, et al. Co-regulatory networks of human serum proteins link genetics to disease. Science 2018;361:769-73. [Crossref] [PubMed]
- Suhre K, Arnold M, Bhagwat AM, et al. Connecting genetic risk to disease end points through the human blood plasma proteome. Nat Commun 2017;8:14357. [Crossref] [PubMed]
- McKay JD, Hung RJ, Han Y, et al. Large-scale association analysis identifies new lung cancer susceptibility loci and heterogeneity in genetic susceptibility across histological subtypes. Nat Genet 2017;49:1126-32. [Crossref] [PubMed]
- Hemani G, Zheng J, Elsworth B, et al. The MR-Base platform supports systematic causal inference across the human phenome. Elife 2018;7:e34408. [Crossref] [PubMed]
- 1000 Genomes Project Consortium; Abecasis GR, Auton A, et al. An integrated map of genetic variation from 1,092 human genomes. Nature 2012;491:56-65.
- Burgess S, Davey Smith G, Davies NM, et al. Guidelines for performing Mendelian randomization investigations: update for summer 2023. Wellcome Open Res 2023;4:186. [Crossref] [PubMed]
- Giambartolomei C, Vukcevic D, Schadt EE, et al. Bayesian test for colocalisation between pairs of genetic association studies using summary statistics. PLoS Genet 2014;10:e1004383. [Crossref] [PubMed]
- Wang G, Sarkar A, Carbonetto P, et al. A simple new approach to variable selection in regression, with application to genetic fine mapping. J R Stat Soc Series B Stat Methodol 2020;82:1273-300. [Crossref] [PubMed]
- Zuber V, Grinberg NF, Gill D, et al. Combining evidence from Mendelian randomization and colocalization: Review and comparison of approaches. Am J Hum Genet 2022;109:767-82. [Crossref] [PubMed]
- Sanderson E, Glymour MM, Holmes MV, et al. Mendelian randomization. Nat Rev Methods Primers 2022;2:6. [Crossref] [PubMed]
- Burgess S, Thompson SG. Interpreting findings from Mendelian randomization using the MR-Egger method. Eur J Epidemiol 2017;32:377-89. [Crossref] [PubMed]
- Kamat MA, Blackshaw JA, Young R, et al. PhenoScanner V2: an expanded tool for searching human genotype-phenotype associations. Bioinformatics 2019;35:4851-3. [Crossref] [PubMed]
- Mountjoy E, Schmidt EM, Carmona M, et al. An open approach to systematically prioritize causal variants and genes at all published human GWAS trait-associated loci. Nat Genet 2021;53:1527-33. [Crossref] [PubMed]
- Goldman MJ, Craft B, Hastie M, et al. Visualizing and interpreting cancer genomics data via the Xena platform. Nat Biotechnol 2020;38:675-8. [Crossref] [PubMed]
- Love MI, Huber W, Anders S. Moderated estimation of fold change and dispersion for RNA-seq data with DESeq2. Genome Biol 2014;15:550. [Crossref] [PubMed]
- Lambrechts D, Wauters E, Boeckx B, et al. Phenotype molding of stromal cells in the lung tumor microenvironment. Nat Med 2018;24:1277-89. [Crossref] [PubMed]
- Wolf FA, Angerer P, Theis FJ. SCANPY: large-scale single-cell gene expression data analysis. Genome Biol 2018;19:15. [Crossref] [PubMed]
- Wolock SL, Lopez R, Klein AM. Scrublet: Computational Identification of Cell Doublets in Single-Cell Transcriptomic Data. Cell Syst 2019;8:281-291.e9. [Crossref] [PubMed]
- Kim N, Kim HK, Lee K, et al. Single-cell RNA sequencing demonstrates the molecular and cellular reprogramming of metastatic lung adenocarcinoma. Nat Commun 2020;11:2285. [Crossref] [PubMed]
- Revel M, Sautès-Fridman C, Fridman WH, et al. C1q+ macrophages: passengers or drivers of cancer progression. Trends Cancer 2022;8:517-26. [Crossref] [PubMed]
- Freshour SL, Kiwala S, Cotto KC, et al. Integration of the Drug-Gene Interaction Database (DGIdb 4.0) with open crowdsource efforts. Nucleic Acids Res 2021;49:D1144-51. [Crossref] [PubMed]
- Wishart DS, Feunang YD, Guo AC, et al. DrugBank 5.0: a major update to the DrugBank database for 2018. Nucleic Acids Res 2018;46:D1074-82. [Crossref] [PubMed]
- Ali S, Dunmore HM, Karres D, et al. The EMA Review of Mylotarg (Gemtuzumab Ozogamicin) for the Treatment of Acute Myeloid Leukemia. Oncologist 2019;24:e171-9. [Crossref] [PubMed]
- Bross PF, Beitz J, Chen G, et al. Approval summary: gemtuzumab ozogamicin in relapsed acute myeloid leukemia. Clin Cancer Res 2001;7:1490-6.
- Pizzitola I, Anjos-Afonso F, Rouault-Pierre K, et al. Chimeric antigen receptors against CD33/CD123 antigens efficiently target primary acute myeloid leukemia cells in vivo. Leukemia 2014;28:1596-605. [Crossref] [PubMed]
- Kim MY, Yu KR, Kenderian SS, et al. Genetic Inactivation of CD33 in Hematopoietic Stem Cells to Enable CAR T Cell Immunotherapy for Acute Myeloid Leukemia. Cell 2018;173:1439-1453.e19. [Crossref] [PubMed]
- Joehanes R, Zhang X, Huan T, et al. Integrated genome-wide analysis of expression quantitative trait loci aids interpretation of genomic association studies. Genome Biol 2017;18:16. [Crossref] [PubMed]
- Folkersen L, Fauman E, Sabater-Lleal M, et al. Mapping of 79 loci for 83 plasma protein biomarkers in cardiovascular disease. PLoS Genet 2017;13:e1006706. [Crossref] [PubMed]
- Hemani G, Bowden J, Davey Smith G. Evaluating the potential role of pleiotropy in Mendelian randomization studies. Hum Mol Genet 2018;27:R195-208. [Crossref] [PubMed]
- Schmidt AF, Finan C, Gordillo-Marañón M, et al. Genetic drug target validation using Mendelian randomisation. Nat Commun 2020;11:3255. [Crossref] [PubMed]
- Gaziano L, Giambartolomei C, Pereira AC, et al. Actionable druggable genome-wide Mendelian randomization identifies repurposing opportunities for COVID-19. Nat Med 2021;27:668-76. [Crossref] [PubMed]
- Jacobs BM, Taylor T, Awad A, et al. Summary-data-based Mendelian randomization prioritizes potential druggable targets for multiple sclerosis. Brain Commun 2020;2:fcaa119. [Crossref] [PubMed]
- King EA, Davis JW, Degner JF. Are drug targets with genetic support twice as likely to be approved? Revised estimates of the impact of genetic support for drug mechanisms on the probability of drug approval. PLoS Genet 2019;15:e1008489. [Crossref] [PubMed]
- Gill D, Cameron AC, Burgess S, et al. Urate, Blood Pressure, and Cardiovascular Disease: Evidence From Mendelian Randomization and Meta-Analysis of Clinical Trials. Hypertension 2021;77:383-92. [Crossref] [PubMed]
- Wouters OJ, McKee M, Luyten J. Estimated Research and Development Investment Needed to Bring a New Medicine to Market, 2009-2018. JAMA 2020;323:844-53. [Crossref] [PubMed]