Establishing a predictive model for tumor mutation burden status based on 18F-FDG PET/CT and clinical features of non-small cell lung cancer patients
Highlight box
Key findings
• Our study demonstrated a significant correlation between maximum standardized uptake value (SUVmax) and tumor mutation burden (TMB) status. The efficacy of the predictive model based on positron emission tomography (PET) parameters and clinical features provides a non-invasive method to assess TMB status effectively. The nomogram, established by combining SUVmax, metabolic tumor volume, pathologic type, carbohydrate antigen 125 level, showed a good diagnostic efficacy (area under the curve =0.822) and performed well in distinguishing the status of TMB-high and TMB-low.
What is known and what is new?
• TMB is a significant biomarker for immune checkpoint inhibitors efficacy. The detection of TMB requires whole exome sequencing, a high-cost method with invasive procedures that is unaffordable and unacceptable for most patients. The predictive model, for the first time, combined PET parameters and clinical features, offering a novel non-invasive approach to assess TMB status.
What is the implication, and what should change now?
• 18F-fluorodeoxyglucose PET/computed tomography is a new imaging method to detect TMB status. The nomogram has the potential to offer supplementary insights into the possibilities and strategies concerning immunotherapy treatment, enhancing TMB’s predictive value for immune checkpoint inhibitors response.
Introduction
Lung cancer is the primary cause of cancer-related deaths worldwide. Non-small cell lung cancer (NSCLC) is the main type of lung cancer, accounting for 80–85% of lung cancer patients (1,2). Therapeutic options for NSCLC treatment, including surgery, radiation therapy, chemotherapy, and targeted drug therapy, have greatly improved the survival of some advanced NSCLC patients. In recent years, immune checkpoint inhibitor (ICI) therapy has become one of the standard therapeutic options for metastatic NSCLC patients, either as monotherapy or combined with other standard therapies (3,4). However, some patients with NSCLC fail to respond to ICI monotherapy. Finding reliable biomarkers to predict the response to ICI therapy has become a huge challenge for clinical application (5).
Tumor mutation burden (TMB), defined as the total number of somatic/acquired mutations per coding area of a tumor genome (mutations/Mb), has emerged as a promising predictive biomarker of response to ICIs. It has been acknowledged that a higher TMB status is associated with higher response rates and longer progression-free survival (6-9). The detection of TMB requires whole exome sequencing (WES), a high-cost method with invasive procedures that is unaffordable and unacceptable for most patients. Therefore, it is of great clinical significance to explore non-invasive and cost-effective biomarkers for TMB.
18F-fluorodeoxyglucose (FDG) positron emission tomography/computed tomography (PET/CT), a noninvasive imaging modality, is widely used in the diagnosis, staging, and therapeutic efficacy evaluation of patients with NSCLC. A few studies have shown that baseline metabolic parameters (MPs) of NSCLC may be predictive factors for TMB (6,10,11). However, these studies still have certain limitations: (I) the cutoff for TMB-high was different; (II) they failed to establish a predictive model for TMB based on MPs of NSCLC patients; (III) these studies had a relatively small sample size. In this study, we established a predictive model for TMB based on MPs and clinical features of NSCLC patients with a larger sample size, aiming to provide a potential non-invasive detection method for TMB, making it a better predictive biomarker of response to ICIs. We present this article in accordance with the TRIPOD reporting checklist (available at https://tlcr.amegroups.com/article/view/10.21037/tlcr-24-416/rc).
Methods
Study patients
We retrospectively included 223 patients diagnosed with NSCLC at the First Affiliated Hospital of Chongqing Medical University from January 2019 to September 2023. The study was conducted in accordance with the Declaration of Helsinki (as revised in 2013). The study was approved by the Ethics Committee of The First Affiliated Hospital of Chongqing Medical University (No. K2023-644) and individual consent for this analysis was waived due to the retrospective nature of the study. The inclusion criteria are as follows: (I) complete clinical data available; (II) pathologically confirmed NSCLC; (III) baseline 18F-FDG PET/CT scans performed before treatment; (IV) baseline TMB data of the primary tumor tissue on biopsy/surgery samples obtained before therapy, excluding TMB data of metastases and lymph nodes; (V) time interval between biopsy/surgery and 18F-FDG PET/CT scan was within 3 months according to previous studies (12,13). We retrospectively collected the clinical features and serological targets from the medical record.
18F-FDG PET/CT acquisition
All patients underwent whole-body PET/CT scans according to the standard protocol of our hospital. All patients fasted for at least 6 hours before the scan with fasting blood glucose levels ≤8.1 mmol/L. Intravenous injection of 18F-FDG imaging agent was administered at a calculated dose of 3.70–5.55 MBq/kg. Each patient was requested to rest quietly for 60 minutes before PET/CT imaging, with a scanning range from the skull base to the middle thigh. 18F-FDG is produced by the cyclotron accelerator of the First Affiliated Hospital of Chongqing Medical University. The patients were scanned with the Philips Gemini 64 PET-CT scanner (Philips Medical Systems, Netherlands). The CT scanning parameters were as follows: voltage, 120 kV; current, 100 mA; layer thickness, 4.0 mm. The PET scanning parameters were as follows: layer thickness, 4.0 mm, 1 minute per bed. The scanned data was attenuated by a computer and reconstructed through iterative methods to obtain maximum intensity projection and fused images.
Image analysis
As shown in Figure 1, 18F-FDG PET/CT imaging data, including PET and CT images, were imported into the open-source software LIFEx (version 5.2.0) (14) in Digital Imaging and Communications in Medicine (DICOM) format. For patients with multiple lesions, only the single primary lesion used for TMB detection was included in the image analysis, excluding other primary and metastatic lesions. The region of interest (ROI) of the primary tumor on 18F-FDG PET/CT fused images was semi-automatically recognized and segmented using a threshold method set at 40% of the maximum standardized uptake value (SUVmax) (14,15). The maximum diameter of the primary tumor was measured on a lung window with CT images data. The above operations were jointly decided by two senior radiologists. Disagreements were resolved by negotiation. The following parameters of the primary tumor were obtained through the above methods: SUVmax, SUVmean, metabolic tumor volume (MTV), and total lesion glycolysis (TLG).
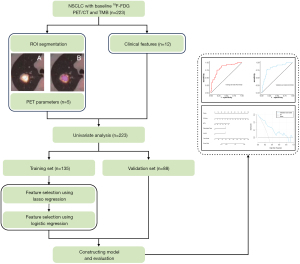
TMB detection
The formalin-fixed paraffin-embedded (FFPE) tumor samples were obtained from primary tumor tissue of 223 patients. Capture-based targeted sequencing was performed at the Clinical Molecular Medicine Testing Center, the First Affiliated Hospital of Chongqing Medical University using a panel consisting of 520 cancer-related genes spanning 1.64 megabases (Mb) of the human genome. The indexed samples were sequenced on an Illumina NextSeq 500 paired-end system (Illumina, Inc., USA) with an average sequencing depth of 1,698× (16,17). TMB per patient was computed as a ratio between the total number of nonsynonymous mutations detected and the coding region size of the panel. TMB-high is defined as TMB >4 mutations/Mb and TMB-low is defined as TMB ≤4 mutations/Mb according to a previous study (18,19).
Statistical analysis
Patient clinical characteristics and imaging parameters were evaluated to determine their association with TMB status. Categorical variables were assessed using the chi-square test or Fisher’s exact test, while continuous variables were described using either mean (± standard deviation) and compared using the t-test for normally distributed data, or median (interquartile range) and compared using the Wilcoxon rank sum test for non-normally distributed data.
Univariate analysis was performed in all patients to reveal the preliminary associations between variables and TMB status. Based on the ratio of 6:4, 60% of patients were randomly selected as the training set, and the remaining patients were used as the validation set. One hundred and thirty-five patients in the training set were included for variable selection with lasso regression and stepwise regression methods. Following variable selection, the patient cohort in the training set was used for developing the diagnostic prediction model, and 88 patients in the validation set for internal model validation. The diagnostic performance of the model was evaluated using receiver operating characteristic (ROC) curves and area under the curve (AUC). Model calibration was assessed using the Hosmer-Lemeshow test, while clinical utility was evaluated using decision curve analysis (DCA).
All statistical analyses and graphical preparations were conducted using R software (version 4.3.0) and packages, including “CBCgrps”, “cowplot”, “rms”, “pROC”, “rmda”, and “tidyverse”. A P value less than 0.05 was considered statistically significant.
Results
Baseline characteristics of patients
The clinical information and PET parameters of all 223 enrolled patients are shown in Tables 1,2. Patients were grouped according to their TMB status, with 127 cases in the TMB-low group and 96 cases in the TMB-high group. Statistical analysis indicated significant differences (P<0.05) in sex, age, pathology type, smoking history, CA125 level, Cyfra21-1 level, lymphatic metastasis, SUVmax, SUVmean, and TLG. Compared to the TMB-low group, the proportion of males, smokers, and patients with pathological type adenocarcinoma was higher in the TMB-high group. Regarding tumor marker levels, patients with high CA125 and Cyfra21-1 accounted for a larger proportion in the TMB-high group. For PET parameters, Table 2 showed that most baseline MPs, including SUVmax, SUVmean, and TLG, were higher in the TMB-high group compared with the TMB-low group. In Table S1, we provide a detailed description of the distribution of various metrics in the training and validation set. The results indicate that there are no statistically significant differences between the two groups for these metrics. Therefore, the data from both cohorts are free from systematic bias and are suitable for model development and validation.
Table 1
Clinical features | All patients | TMB-low | TMB-high | P value |
---|---|---|---|---|
Sex | 0.003 | |||
Male | 122 [55] | 58 [46] | 64 [67] | |
Female | 101 [45] | 69 [54] | 32 [33] | |
Age, years | 63±8.94 | 61.59±9.84 | 64.86±7.23 | 0.005 |
Pathology type | 0.005 | |||
SCC | 38 [17] | 30 [24] | 8 [8] | |
ADC | 185 [83] | 97 [76] | 88 [92] | |
Clinical stage | 0.76 | |||
I | 63 [28] | 39 [31] | 24 [25] | |
II | 19 [9] | 11 [9] | 8 [8] | |
III | 35 [16] | 18 [14] | 17 [18] | |
IV | 106 [48] | 59 [46] | 47 [49] | |
Smoking history | <0.001 | |||
Nonsmoker | 72 [32] | 54 [43] | 18 [19] | |
Smoker | 151 [68] | 73 [57] | 78 [81] | |
Family malignant tumor history | 0.17 | |||
Yes | 53 [24] | 35 [28] | 18 [19] | |
No | 170 [76] | 92 [72] | 78 [81] | |
Multiple primary lung cancer | 0.16 | |||
Yes | 43 [19] | 29 [23] | 14 [15] | |
No | 180 [81] | 98 [77] | 82 [85] | |
BMI, kg/m2 | 21.5 (20.2–23.15) | 21.5 (20.05–23.25) | 21.6 (20.3–22.92) | 0.79 |
CEA | 0.08 | |||
Normal | 118 [53] | 74 [58] | 44 [46] | |
High | 105 [47] | 53 [42] | 52 [54] | |
CA125 | <0.001 | |||
Normal | 168 [75] | 109 [86] | 59 [61] | |
High | 55 [25] | 18 [14] | 37 [39] | |
Cyfra21-1 | 0.01 | |||
Normal | 90 [40] | 61 [48] | 29 [30] | |
High | 133 [60] | 66 [52] | 67 [70] | |
Lymphatic metastasis | 0.009 | |||
Yes | 93 [42] | 43 [34] | 50 [52] | |
No | 130 [58] | 84 [66] | 46 [48] |
Data are expressed as n [%], mean ± standard deviation or median (interquartile range). Upper limits of normal were 5.0 ng/mL for CEA, 35.0 U/mL for CA125, 3.3 ng/mL for Cyfra21-1. SCC, squamous cell carcinoma; ADC, adenocarcinoma; BMI, body mass index; CEA, carcinoembryonic antigen; CA125, carbohydrate antigen 125; Cyfra21-1, cytokeratin 19 fragment; NSCLC, non-small cell lung cancer; TMB, tumor mutation burden.
Table 2
PET parameters | All patients | TMB low | TMB high | P value |
---|---|---|---|---|
SUVmax | 7.1 [4.25–10.7] | 5.5 [2.5–8.95] | 9.75 [7–13.6] | <0.001 |
SUVmean | 4.5 [2.55–6.8] | 3.5 [1.55–5.6] | 6 [4.2–8.5] | <0.001 |
MTV | 8.5 [4.6–18.55] | 8 [4.7–15.9] | 10.75 [4.5–22.1] | 0.24 |
TLG | 35.28 [10.49–109.65] | 22.68 [6.82–63.24] | 46.54 [18.76–170.88] | <0.001 |
Size*, cm | 27 [20–39] | 26 [19–34] | 29.5 [20–44] | 0.07 |
The data are expressed as median [interquartile range]. Size*, maximum diameter of the tumor. PET, positron emission tomography; SUVmax, maximum standardized uptake value; SUVmean, mean standardized uptake value; MTV, metabolic tumor volume; TLG, total lesion glycolysis; NSCLC, non-small cell lung cancer; TMB, tumor mutation burden.
The performance of individual factors for discriminating between TMB-high and TMB-low
We plotted ROC curves to evaluate the ability of individual factors to distinguish between TMB-high and TMB-low (Figure 2A). It was observed that SUVmax was the most accurate biomarker in distinguishing TMB-high from TMB-low, with an AUC >0.75 (Figure 2A,2B). The remaining factors had AUCs below 0.75 in differentiating between the groups (Figure 2B). Therefore, we hope to combine multiple indicators to establish a predictive model to improve the prediction performance of TMB status.
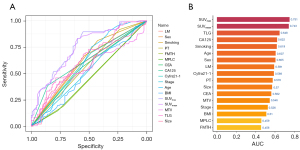
Collinearity among variables and variable selection
Based on a ratio of 6:4, 135 patients were randomly selected as the training set, and the remaining 88 patients were used as the validation set. Recognizing the significant collinearity among the PET parameters, which indicated varying degrees of correlation among multiple variables (Figure 3), we adopted a two-stage method to address this issue. Initially, we applied lasso regression directly to all variables instead of performing traditional univariate analysis. lasso regression reduces complexity and mitigates multicollinearity by penalizing the absolute size of the regression coefficients, compressing some coefficients and setting others to zero, effectively selecting more relevant variables (Figure 4). Subsequently, the variables that remained significant were further analyzed through stepwise regression, specifically a bidirectional approach that iteratively added and removed predictors to find the optimal model. This combination of lasso followed by stepwise regression allows for more robust feature selection in the presence of multicollinearity. After the variable selection process, four variables were used to construct the predictive model: SUVmax (OR =1.265, 95% CI: 1.144–1.420, P<0.001), MTV (OR =0.976, 95% CI: 0.953–0.996, P=0.02), PT (OR =7.301, 95% CI: 1.876–38.682, P=0.008), and CA125 level (OR =6.736, 95% CI: 2.594–19.242, P<0.001).
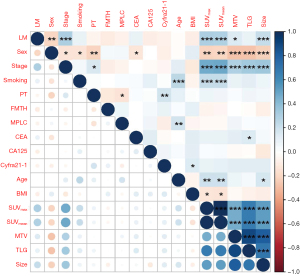
Predictive model and evaluation of model
These variables, selected through lasso regression followed by stepwise regression, were incorporated into a predictive model for TMB status. The predictive performance of the model was assessed using ROC curves. The AUC for the model was 0.822 (95% CI, 0.751–0.894) in the training set and also 0.822 (95% CI, 0.731–0.912) in the validation set (Figure 5). This consistent performance across both datasets underscores the model’s robustness. The model was further visualized through a nomogram (Figure 6), designed to calculate the probability of NSCLC being classified as TMB-high. The calculation method involved assigning scores to each variable based on their contribution, summed across all selected factors. The total points were then used to determine the corresponding predicted probability of being TMB-high, as depicted on the nomogram’s lower scale.
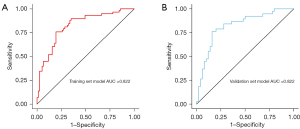
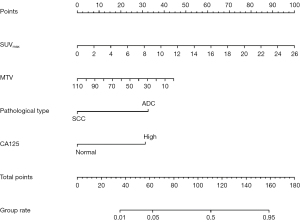
Figure 7 illustrates the calibration curve of the nomogram. The alignment of the prediction curve with the diagonal suggests a good correspondence between predicted and actual probabilities (the Hosmer-Lemeshow test, P>0.05, suggesting that it is of good fit). Decision curves for the predictive model (Figure 8) show the clinical utility across various risk thresholds, recommending risk-based interventions when the probability thresholds range from 10% to 70% in the training set and from 10% to 80% in the validation set. These thresholds provide a guideline for clinical decision-making, suggesting the model’s practical application in patient management strategies.
Discussion
Immunotherapy has significantly transformed treatment strategies for various cancers, including NSCLC. However, response heterogeneity presents a substantial challenge (20,21). Consequently, identifying effective predictive biomarkers for ICIs is critical to improving prognosis in NSCLC patients (22,23).
PD-L1 expression is commonly used as a biomarker for selecting ICI therapy (24,25), yet its predictive efficacy is marred by inconsistencies due to methodological discrepancies and inherent limitations (26-28). Importantly, some studies have documented significant ICI responses in patients with PD-L1 expression below 1% (29,30).
Advancements in sequencing technologies have highlighted the role of non-synonymous mutations in creating neoantigens, which affect tumor immunogenicity and the response to ICIs (9). TMB, which measures the total number of non-synonymous mutations per DNA megabase, has emerged as a promising predictive biomarker for ICI response (29,30). Next-generation sequencing (NGS) is the preferred method for assessing TMB, providing a quantitative analysis across various tumor types (31). However, the clinical utility of NGS is hindered by its technical complexities, extended processing times, and invasiveness (12).
Radiological imaging, specifically 18F-FDG PET/CT, offers a non-invasive alternative for predicting TMB status, circumventing the limitations associated with invasive biopsies and the lengthy durations of NGS cycles. Previous studies have investigated the relationship between baseline MPs from 18F-FDG PET/CT and TMB status, though these studies have been limited by small sample sizes and inconsistent TMB-high cutoffs. Moon et al. found no significant relationship between SUVmax and TMB status in lung cancer patients (13), possibly due to ignoring the time interval between 18F-FDG PET-CT and biopsy, which could impact the correlation. Addressing this issue, Haghighat Jahromi et al. (11) demonstrated a significant positive correlation between SUVmax and TMB status in multiple advanced cancers by limiting the interval to within six months. Furthermore, Zhang et al. (6) confirmed that SUVmax values were higher in the TMB-high group compared to the TMB-low group in NSCLC patients. Based on the previous study (12), we propose that an elevated mutation burden, indicated by TMB, could be linked to metabolic remodeling and immune inflammatory response. These characteristics, in turn, may correlate with increased SUVmax.
In our study, we established a predictive model from the training set of 135 patients and evaluated the model’s performance in an independent validation set of 88 patients. AUC for the model was 0.822 (95% CI, 0.751–0.894) in the training set and 0.822 (95% CI, 0.731–0.912) in the validation set, respectively. This development marks the first time a predictive model combining 18F-FDG PET/CT parameters and clinical features has demonstrated such significant predictive capability, representing a major innovation in our research.
Compared to previous studies, our study has the following improvements: (I) we have more strictly limited the interval between 18F-FDG PET/CT and biopsy to within three months, ensuring temporal consistency between PET parameters and TMB status; (II) we have expanded the sample size; (III) for the first time, we have used PET parameters and clinical features to establish a prediction model for TMB levels; (IV) we explored a different cutoff for TMB status, as there is no uniform cutoff value for high and low TMB groups. Zhang et al. (6) used TMB >10 mutations/Mb for grouping in their study, but this is not suitable for constructing a predictive model because the majority of patients have TMB status less than 10 mutations/Mb. Based on previous research (18,19), we chose 4 as the cutoff between high and low TMB groups, making the high TMB group and low TMB group more balanced and enhancing the credibility of the prediction model.
Additionally, we innovated in statistical methods. Instead of traditional univariate analysis with backward stepwise elimination, we applied lasso regression followed by stepwise variable screening directly. This method proved more effective, particularly since the MTV variable, previously insignificant in univariate analysis, emerged as a key predictor in both lasso and stepwise regression. This underscores the robustness and efficacy of lasso regression in comprehensively considering interactions and overall effects among variables, thereby optimizing the variable selection process. The predictive model demonstrates a significant correlation between SUVmax and TMB status, providing a non-invasive method to assess TMB status effectively.
While our results are promising, the study is not without limitations. Being conducted within a single institution, future research should aim for multicenter studies to validate and generalize the findings. The retrospective nature of our study may also introduce potential biases, underscoring the need for prospective application of our predictive model in clinical settings. Furthermore, the correlation between driver mutations such as EGFR and TP53 and TMB status remains unexplored and will be addressed in subsequent research efforts.
Conclusions
In our study, we successfully developed a predictive model that integrates 18F-FDG PET/CT baseline MPs with clinical features to evaluate the TMB status of NSCLC patients effectively. The model was visualized through a nomogram that demonstrated robust predictive accuracy and substantial clinical value, offering a non-invasive approach to assess TMB status.
This model’s non-invasive nature is particularly advantageous in the clinical setting, as it reduces the need for invasive biopsies and expedites the assessment process, which is vital for timely therapeutic decision-making. In the future, we aim to enhance this model by incorporating it into a multivariate efficacy prediction framework that includes TMB and other significant predictors relevant to the efficacy of ICI therapies. Such advancements could provide clinicians with a more comprehensive tool for making informed decisions regarding combination therapy, ultimately aiming to improve the survival rates and quality of life for NSCLC patients.
Acknowledgments
Funding: This work was supported by Research on
Footnote
Reporting Checklist: The authors have completed the TRIPOD reporting checklist. Available at https://tlcr.amegroups.com/article/view/10.21037/tlcr-24-416/rc
Data Sharing Statement: Available at https://tlcr.amegroups.com/article/view/10.21037/tlcr-24-416/dss
Peer Review File: Available at https://tlcr.amegroups.com/article/view/10.21037/tlcr-24-416/prf
Conflicts of Interest: All authors have completed the ICMJE uniform disclosure form (available at https://tlcr.amegroups.com/article/view/10.21037/tlcr-24-416/coif). The authors have no conflicts of interest to declare.
Ethical Statement: The authors are accountable for all aspects of the work in ensuring that questions related to the accuracy or integrity of any part of the work are appropriately investigated and resolved. The study was conducted in accordance with the Declaration of Helsinki (as revised in 2013). The study was approved by the Ethics Committee of the First Affiliated Hospital of Chongqing Medical University (No. K2023-644) and individual consent for this retrospective analysis was waived due to the retrospective nature of the study.
Open Access Statement: This is an Open Access article distributed in accordance with the Creative Commons Attribution-NonCommercial-NoDerivs 4.0 International License (CC BY-NC-ND 4.0), which permits the non-commercial replication and distribution of the article with the strict proviso that no changes or edits are made and the original work is properly cited (including links to both the formal publication through the relevant DOI and the license). See: https://creativecommons.org/licenses/by-nc-nd/4.0/.
References
- Dantoing E, Piton N, Salaün M, et al. Anti-PD1/PD-L1 Immunotherapy for Non-Small Cell Lung Cancer with Actionable Oncogenic Driver Mutations. Int J Mol Sci 2021;22:6288. [Crossref] [PubMed]
- Jemal A, Bray F, Center MM, et al. Global cancer statistics. CA Cancer J Clin 2011;61:69-90. [Crossref] [PubMed]
- Brueckl WM, Ficker JH, Zeitler G. Clinically relevant prognostic and predictive markers for immune-checkpoint-inhibitor (ICI) therapy in non-small cell lung cancer (NSCLC). BMC Cancer 2020;20:1185. [Crossref] [PubMed]
- Lahiri A, Maji A, Potdar PD, et al. Lung cancer immunotherapy: progress, pitfalls, and promises. Mol Cancer 2023;22:40. [Crossref] [PubMed]
- Vanguri RS, Luo J, Aukerman AT, et al. Multimodal integration of radiology, pathology and genomics for prediction of response to PD-(L)1 blockade in patients with non-small cell lung cancer. Nat Cancer 2022;3:1151-64. [Crossref] [PubMed]
- Zhang Q, Tao X, Yuan P, et al. Predictive value of (18) F-FDG PET/CT and serum tumor markers for tumor mutational burden in patients with non-small cell lung cancer. Cancer Med 2023;12:20864-77. [Crossref] [PubMed]
- Hellmann MD, Ciuleanu TE, Pluzanski A, et al. Nivolumab plus Ipilimumab in Lung Cancer with a High Tumor Mutational Burden. N Engl J Med 2018;378:2093-104. [Crossref] [PubMed]
- Carbone DP, Reck M, Paz-Ares L, et al. First-Line Nivolumab in Stage IV or Recurrent Non-Small-Cell Lung Cancer. N Engl J Med 2017;376:2415-26. [Crossref] [PubMed]
- Zheng M. Tumor mutation burden for predicting immune checkpoint blockade response: the more, the better. J Immunother Cancer 2022;10:e003087. [Crossref] [PubMed]
- Ono A, Terada Y, Kawata T, et al. Assessment of associations between clinical and immune microenvironmental factors and tumor mutation burden in resected nonsmall cell lung cancer by applying machine learning to whole-slide images. Cancer Med 2020;9:4864-75. [Crossref] [PubMed]
- Haghighat Jahromi A, Barkauskas DA, Zabel M, et al. Relationship between tumor mutational burden and maximum standardized uptake value in 2-[18F]FDG PET (positron emission tomography) scan in cancer patients. EJNMMI Res 2020;10:150. [Crossref] [PubMed]
- Wang J, Wang J, Huang X, et al. CT radiomics-based model for predicting TMB and immunotherapy response in non-small cell lung cancer. BMC Med Imaging 2024;24:45. [Crossref] [PubMed]
- Moon SH, Kim J, Joung JG, et al. Correlations between metabolic texture features, genetic heterogeneity, and mutation burden in patients with lung cancer. Eur J Nucl Med Mol Imaging 2019;46:446-54. [Crossref] [PubMed]
- Nioche C, Orlhac F, Boughdad S, et al. LIFEx: A Freeware for Radiomic Feature Calculation in Multimodality Imaging to Accelerate Advances in the Characterization of Tumor Heterogeneity. Cancer Res 2018;78:4786-9. [Crossref] [PubMed]
- Cheebsumon P, Yaqub M, van Velden FH, et al. Impact of [18F]FDG PET imaging parameters on automatic tumour delineation: need for improved tumour delineation methodology. Eur J Nucl Med Mol Imaging 2011;38:2136-44. [Crossref] [PubMed]
- Ye S, Li Q, Wu Y, et al. Integrative genomic and transcriptomic analysis reveals immune subtypes and prognostic markers in ovarian clear cell carcinoma. Br J Cancer 2022;126:1215-23. [Crossref] [PubMed]
- Li F, Yang Y, Xu Y, et al. Comparative study of the genomic landscape and tumor microenvironment among large cell carcinoma of the lung, large cell neuroendocrine of the lung, and small cell lung cancer. Medicine (Baltimore) 2023;102:e32781. [Crossref] [PubMed]
- Wang X, Kong C, Xu W, et al. Decoding tumor mutation burden and driver mutations in early stage lung adenocarcinoma using CT-based radiomics signature. Thorac Cancer 2019;10:1904-12. [Crossref] [PubMed]
- Yin W, Wang W, Zou C, et al. Predicting Tumor Mutation Burden and EGFR Mutation Using Clinical and Radiomic Features in Patients with Malignant Pulmonary Nodules. J Pers Med 2022;13:16. [Crossref] [PubMed]
- Fumet JD, Truntzer C, Yarchoan M, et al. Tumour mutational burden as a biomarker for immunotherapy: Current data and emerging concepts. Eur J Cancer 2020;131:40-50. [Crossref] [PubMed]
- Freedman AN, Klabunde CN, Wiant K, et al. Use of Next-Generation Sequencing Tests to Guide Cancer Treatment: Results From a Nationally Representative Survey of Oncologists in the United States. JCO Precis Oncol 2018;2:PO.18.00169.
- Samstein RM, Lee CH, Shoushtari AN, et al. Tumor mutational load predicts survival after immunotherapy across multiple cancer types. Nat Genet 2019;51:202-6. [Crossref] [PubMed]
- Yarchoan M, Albacker LA, Hopkins AC, et al. PD-L1 expression and tumor mutational burden are independent biomarkers in most cancers. JCI Insight 2019;4:e126908. [Crossref] [PubMed]
- Galvano A, Gristina V, Malapelle U, et al. The prognostic impact of tumor mutational burden (TMB) in the first-line management of advanced non-oncogene addicted non-small-cell lung cancer (NSCLC): a systematic review and meta-analysis of randomized controlled trials. ESMO Open 2021;6:100124. [Crossref] [PubMed]
- D'Arcangelo M, D'Incecco A, Ligorio C, et al. Programmed death ligand 1 expression in early stage, resectable non-small cell lung cancer. Oncotarget 2019;10:561-72. [Crossref] [PubMed]
- Büttner R, Longshore JW, López-Ríos F, et al. Implementing TMB measurement in clinical practice: considerations on assay requirements. ESMO Open 2019;4:e000442. [Crossref] [PubMed]
- Prestipino A, Zeiser R. Clinical implications of tumor-intrinsic mechanisms regulating PD-L1. Sci Transl Med 2019;11:eaav4810. [Crossref] [PubMed]
- Bregni G, Sticca T, Camera S, et al. Feasibility and clinical impact of routine molecular testing of gastrointestinal cancers at a tertiary centre with a multi-gene, tumor-agnostic, next generation sequencing panel. Acta Oncol 2020;59:1438-46. [Crossref] [PubMed]
- Provencio M, Ortega AL, Coves-Sarto J, et al. Atezolizumab Plus Bevacizumab as First-line Treatment for Patients With Metastatic Nonsquamous Non-Small Cell Lung Cancer With High Tumor Mutation Burden: A Nonrandomized Controlled Trial. JAMA Oncol 2023;9:344-53. [Crossref] [PubMed]
- Slansky JE, Spellman PT. Alternative Splicing in Tumors - A Path to Immunogenicity? N Engl J Med 2019;380:877-80. [Crossref] [PubMed]
- Chalmers ZR, Connelly CF, Fabrizio D, et al. Analysis of 100,000 human cancer genomes reveals the landscape of tumor mutational burden. Genome Med 2017;9:34. [Crossref] [PubMed]