A multi-level investigation of the genetic relationship between gastroesophageal reflux disease and lung cancer
Highlight box
Key findings
• This study represents the first comprehensive investigation into the shared genetic architecture between gastroesophageal reflux disease (GERD) and lung cancer (LC), providing valuable insights into this complex genetic interplay.
What is known and what is new?
• Observational studies have revealed a potential association between GERD and LC, but the genetic role in their comorbidity have not been fully elucidated.
• This study provides valuable evidence of genetic correlation, identifying pleiotropic loci, and suggesting a potential causal association between GERD and LC.
What is the implication, and what should change now?
• This study conveys a crucial public health message: managing individuals with GERD may potentially contribute to reducing the long-term burden of malignant diseases.
Introduction
Gastroesophageal reflux disease (GERD), characterized by troublesome symptoms and complications caused by the reflux of duodenogastric contents, is a common and chronic condition affecting approximately 2.5% to 33.1% of the global population (1,2). Recurrent micro-aspiration from the refluxed contents is associated with higher risk of multiple lung diseases, including pneumonia, asthma, bronchiolitis obliterans syndrome, idiopathic pulmonary fibrosis, and chronic obstructive pulmonary disease (3-6). Additionally, underlying links between GERD and non-esophageal cancer have been also recognized (7). Lung cancer (LC), as one of the most prevalent malignancies, remains the leading cause in both incidence rate and mortality worldwide (8). Recent epidemiological studies have observed a significant phenotypic association between GERD and LC. Leveraging data from the National Health Insurance Research Database of Taiwan (15,412 cases and 60,957 controls), Hsu et al. found that patients with GERD were associated with significantly elevated risk of LC in comparison to those without (9). More recently, a multinational cohort study enrolled 812,617 patients with GERD to investigate the impact of anti-reflux surgery on the risk of distinct histological types of LC (10). Similarly, this study found that anti-reflux surgery significantly decreased the risk of small-cell lung cancer (SCLC) and lung squamous cell carcinoma (LUSC), and showed a protective trend for lung adenocarcinoma (LUAD). Despite this, phenotypic correlations revealed in conventional epidemiological studies were susceptible to potential biases, confounding factors, and reverse causality due to the observational nature (11).
Utilizing genetic data for phenotypic correlation analysis offers a distinct advantage over observational studies, as it can effectively circumvent the issue of reverse causality and can also minimize the potential confounding with meticulous design. With the increasing sample size of genome-wide association studies (GWAS), previous studies have identified a substantial number of genetic variants [single nucleotide polymorphisms (SNPs)] associated with GERD (88 SNPs) and LC (56 SNPs) (12,13). Furthermore, utilizing the design of the twin study, heritability of GERD and LC has been estimated as 30–31% (14,15) and 18–26% (16,17), respectively. This underscores a significant genetic component in disease susceptibility.
In this context, several Mendelian randomization (MR) studies have been conducted using genetic variants as instrumental variables (IVs), and consistently identified a causal association between GERD and LC, with odds ratio (OR) ranging from 1.25 to 1.37 (18-20). Nonetheless, multiple significant gaps in previous investigations remain to be filled. Firstly, prior MR studies used GWAS data with relatively small sample sizes (18,19), particularly for SCLC, which restricted the statistical power. Secondly, the insufficient sensitivity analyses did not guarantee the core model assumptions, thereby impeding the robustness of results (21). Finally, the adoption of limited confounders, such as smoking status and obesity, may not comprehensively account for potential pleiotropy in complex traits (20,22).
Therefore, a novel statistical genetic tool named genome-wide cross-trait analysis was utilized to dissect shared genetic components in complex traits, using summary data from the large-scale GWAS studies (11,23). Specifically, we measured the genetic correlation, identified the shared loci, and finally inferred a putative causal association through the bidirectional two-sample MR analysis. Figure 1 illustrates the overall study design. We present this article in accordance with the STREGA reporting checklist (available at https://tlcr.amegroups.com/article/view/10.21037/tlcr-24-345/rc) (24).
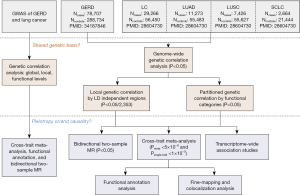
Methods
GWAS summary datasets
In the study, summary data from the hitherto largest GWAS of GERD and LC were leveraged for genetic analyses, both exclusively focused on individuals of European ancestry. The detailed information for the GWAS data of both traits is shown in Table S1.
The largest GWAS study on GERD derived from the meta-analyzing data by Ong et al. in 2022 (12), which combined up to 367,441 (78,707 cases) European individuals from the UK Biobank (UKBB) study (35,4285 individuals) and Queensland Sun and Health Study (QSKIN) study (13,156 individuals). GERD was defined based on a combination of self-reported GERD symptoms such as heartburn, the use of GERD medication, and hospital records [The International Classification of Diseases, Tenth Revision (ICD-10)]. The Haplotype Reference Consortium (HRC) reference panel was used to impute the genotype data. To combine the GWAS data from the UKB and QSKIN cohorts, a fixed-effect inverse variance-weighted (IVW) meta-analysis was performed.
For overall LC and subtype-specific LC, the largest GWAS data were a meta-analysis of data from McKay et al. in 2017 (25). The GWAS summary data from the International Lung Cancer Consortium (ILCCO) combined a total of 29,266 LC cases and 56,450 controls, which included 11,273 LUAD, 7,426 LUSC, and 2,664 SCLC. Imputation was performed on variants based on the 1000 Genomes Project (1KGP) Phase 3 panel. The fixed-effect IVW meta-analysis was carried out to combine the GWAS data. The study was conducted in accordance with the Declaration of Helsinki (as revised in 2013).
Statistical analyses
Genome-wide genetic correlation analysis
We performed linkage disequilibrium (LD) score regression (LDSC) analysis to evaluate the genome-wide genetic correlations between two traits, utilizing GWAS summary statistics in the calculation (26). LDSC estimates genetic correlation (rg) on a scale from −1 to +1. It leverages the fact that when estimating the effect size of a specific variant, the combined effects of all variants that are in LD with that variant were considered. Thus, the idea of substituting the χ2 statistics with the product of z-scores from two traits and the LD scores allows us to calculate genetic correlations between traits: , where Ni represents the sample size of each trait, M represents number of SNPs, ρg represents the genetic covariance, lj represents the LD scores, ρ represents the phenotypic link within overlapping samples, Ns represents the overlapping sample size. By performing a regression of the product of z-scores from two GWASs based on the reference LD scores from 1KGP European ancestry with SNPs mapped in the Hapmap3 reference panel, the genetic covariance between two traits can be estimated. Then, the genetic correlation can be calculated as , where represents the heritability for each trait. Given the potential overlap in population between the GWAS data of GERD and LC, we also conducted LDSC with a constrained intercept, which is more robust in handling sample overlap (26). For multiple testing, the false discovery rate (Benjamini-Hochberg correction) was employed.
Local genetic correlation analysis
The global genetic correlation offers an assessment of the collective impact of genome-wide variants. However, it is conceivable that, despite exhibiting minimal global genetic correlation, certain regions of the genome may still have an impact on both traits. Thus, we computed pairwise local genetic correlation using SUPERGNOVA (27). This algorithm separates the entire genome into approximately 2,353 independent regions, with each averaging about 1.6 centimorgans in length. It then measures the genetic correlation specific to each of these genomic regions. To account for multiple testing, Bonferroni correction (P<0.05/2,353) was applied.
Partitioned LDSC analysis
Using partitioned LDSC (28), we investigated the genetic correlation between GERD and LC in multiple functional categories. This study included 14 common functional categories, including coding region, conserved region, DNase I digital genomic foot-printing region (DGF), DNase I hypersensitive sites (DHS), fetal DHS, intron, promotor, super enhancer, transcription factor-binding site (TFBS), transcribed region, and histone marks H3K27ac, H3K4me1, H3K4me3, and H3K9ac (28,29). For SNPs classified within each specific category, recalculated LD scores were utilized to estimate the genetic correlation between GERD and LC within that functional category.
Cross-trait meta-analysis
A significant genetic correlation suggests the presence of either horizontal pleiotropy (pleiotropy) or vertical pleiotropy (causality). To further investigate the pleiotropic SNPs associated with both traits (GERD and LC), we performed a cross-phenotypic association (CPASSOC) analysis (30). Utilizing summary data from single SNP-trait associations in GWAS, CPASSOC provides two estimates, SHom and SHet. Representing the maximum of the weighted sum of trait-specific genetic effects, SHom employs a fixed-effect meta-analysis approach, which was more powerful when genetic effect sizes cross traits were homogenous. SHet, as an extension of SHom, assumes the presence of heterogeneity and computes corresponding P value via a sample size-weighted meta-analysis of GWAS summary data. For this analysis, we adopted the SHet method to correct for potential heterogeneity and ensure more robust results.
After CPASSOC analysis, independent loci were obtained using software PLINK (v1.9) with parameters: --clump-p1 5E-8 --clump-p2 1E-5 --clump-r2 0.2 --clump-kb 500 (31). SNPs with the lowest P value within each independent locus were defined as index SNPs. Significant pleiotropic SNPs were defined as having PCPASSOC <5×10−8 and Psingle-trait <1×10−3 in both traits. These SNPs were further classified into four groups: (I) “known” shared SNPs, referring to SNPs that reach genome-wide significance in both traits (PGERD <5×10−8 and PLC <5×10−8); (II) “single-trait-driven” shared SNPs, referring to SNPs reaching genome-wide significance in one of the two traits, either PGERD <5×10−8 or PLC <5×10−8; (III) “LD-Tagged” shared SNPs, referring to SNPs not reaching genome-wide significance in both traits (PGERD >5×10−8 and PLC >5×10−8), but showing LD (r2≥0.2) with index SNPs previously identified by single-trait GWAS; and (IV) novel shared SNPs, referring to significant pleiotropic SNPs that did not reach genome-wide significance in both traits (5×10−8< Psingle-trait <1×10−3) and were not in LD with previously identified SNPs in single-trait GWAS (r2<0.2) (32). To gain further insights into the biological implications in the shared SNPs, the linear closest genes of pleiotropic loci were annotated using the Ensemble Variant Effect Predictor (VEP) (33).
Fine-mapping credible set analysis
Index SNPs may not always be causal variants due to the complex LD patterns across SNPs. To obtain a credible set of causal variants that have a 99% likelihood of encompassing causal variants for each shared loci, we employed the Bayesian fine-mapping algorithm—FM-summary (34). For each shared locus, variants located within 500 kb of the index SNP were extracted (35). The FM-summary prioritizes the primary signal and applies a flat prior along with a steepest descent approximation (36).
Colocalization analysis
To determine whether the association signals for GERD and LC co-occurred at identified shared loci, we performed the colocalization analysis using the R package Coloc (37). Coloc employs the Bayesian algorithm to obtain five posterior probabilities for five different hypotheses: (I) H0, no causal variant; (II) H1 or H2, causal variant only for one trait; (III) H3, two distinct variants associated with both traits; and (IV) H4, shared variant correlated with both traits. The posterior probability for H4 (PPH4) was calculated using summary data for variants near loci shared between GERD and LC that were within 500 kb of the index SNP. If PPH4 exceeded 0.5, a locus was labeled as a co-localized genetic variant.
Transcriptome-wide association studies (TWASs)
Many genetic variants have an effect on complex phenotypes by modulating gene expression. Therefore, determining overlapping genes underlying GERD and LC may shed light on the underlying causal mechanisms. Utilizing FUSION (38), the TWAS was performed to identify associations between GERD and LC regarding gene expression in multiple tissues. This involved integrating expression weights obtained from 49 tissues sourced from GTEx (version 8) with GWAS summary data (39). To obtain an independent set of gene-tissue pairs, a total of 49 TWASs for each trait were systematically conducted, focusing on one tissue-trait pairing at a time. Subsequently, by intersecting across traits, shared gene-tissue pairs were identified. The Benjamini-Hochberg correction was used to correct TWAS P values, and a false discovery rate <0.05 was deemed significant.
Bidirectional MR analysis
Next, we investigated the putative causal association between GERD and LC through the bidirectional two-sample MR analysis. For GERD, genome-wide significant SNPs (P<5×10−8) were selected and clumped for independent IVs (r2=0.01 and window size =10 Mb). For LC, SNPs with P value <5×10−8 were obtained and clumped using parameters: r2=0.01 and window size =10 Mb. F-statistic was calculated to assess strength of selected IVs, where a value less than 10 indicates a weak instrument (40). Additionally, the statistical power of MR was evaluated using an online calculator (https://shiny.cnsgenomics.com/mRnd/) (41).
We implemented the IVW method as the principal approach, assumes all IVs to be valid and offers the highest statistical power (42). Additionally, we performed several complementary sensitivity analyses to evaluate the robustness: (I) MR-Egger regression, identifying and mitigating bias resulting from directional pleiotropy (43); (II) weighted median, offering a consistent estimate of causality even with more than 50% invalid IVs (44); (III) Causal Analysis Using Summary Effect estimates (CAUSE) and MR-Pleiotropy Residual Sum and Outlier (MR-PRESSO), evaluating and adjusting for the potential correlated and uncorrelated horizontal pleiotropy (45,46); (IV) removing pleiotropic IVs associated with potential confounding factors based on the Phenoscanner (47); (V) removing palindromic IVs with strand ambiguity; and (VI) leave-one-out analyses, evaluating the potential impact of each SNP on the IVW estimate. We further utilized multivariable MR (MVMR) (48) to adjust for influence of significant confounding factors, including body mass index (BMI) (49), smoking status (50), alcohol consumption (50), physical activity (51), and sleep duration (52). These confounders were integrated individually as well as collectively with GERD to ensure a comprehensive analysis. Finally, a reverse-direction MR analysis was carried out to determine if genetic predisposition to LC has a causal impact on GERD.
All MR analyses were carried out utilizing the following R packages: “TwoSampleMR” (v0.5.6), “MRPRESSO” (v1.0), “CAUSE” (v1.2.0), and “MVMR” (v0.3), in R software (v4.2.3).
Results
Global genetic correlation
We observed a strongly significant global genetic correlation between GERD and overall LC (rg=0.33, P=1.58×10−14) after adjusting for multiple testing (Table 1). The genetic correlation continued to be significant in subtype-specific LC (LUAD: rg=0.19, P=6.64×10−6; LUSC: rg=0.39, P=2.22×10−12; SCLC: rg=0.39, P=5.27×10−12). Given the potential sample overlap in GWAS data, the intercepts of genetic covariance were constrained to zero, which exhibited increased power, while also marginally reducing standard errors. Using constrained LDSC, the genetic correlation remained significant for LC (rg=0.36, P=3.28×10−37) as well as subtype-specific LC (LUAD: rg=0.20, P=5.27×10−12; LUSC: rg =0.41, P=1.53×10−34; SCLC: rg=0.39, P=7.31×10−25).
Table 1
Trait 1 | Trait 2 | Unconstrained LDSC | Constrained LDSC | |||||
---|---|---|---|---|---|---|---|---|
rg | rg_se | P value | rg | rg_se | P value | |||
GERD | Overall LC | 0.33 | 0.04 | 1.58×10−14 | 0.36 | 0.03 | 3.28×10−37 | |
GERD | LUAD | 0.19 | 0.04 | 6.64×10−6 | 0.20 | 0.03 | 5.27×10−12 | |
GERD | LUSC | 0.39 | 0.05 | 2.22×10−12 | 0.41 | 0.03 | 1.53×10−34 | |
GERD | SCLC | 0.39 | 0.06 | 5.27×10−12 | 0.39 | 0.03 | 7.31×10−25 |
GERD, gastroesophageal reflux disease; LC, lung cancer; LDSC, linkage disequilibrium score regression; LUAD, lung adenocarcinoma; LUSC, lung squamous cell carcinoma; SCLC, small-cell lung cancer; rg, genetic correlation; se, standard error.
Local genetic correlation
After separating the genome into multiple LD-independent regions, 5 local regions with significant genetic correlation were detected, including 1 region (Chr2: 103,264,434–104,481,488) shared by overall LC and LUAD, and 1 region (Chr9: 126,927,204–128,926,989) shared by overall LC and LUSC (Figure 2). The most significant region was located at 9q33.3 (Chr9: 126,927,204–128,926,989, P=6.76×10−10), which harbors PBX3, a factor interacts with the promoter of tumor suppressor p53 associated with LC tumorigenesis (53,54).
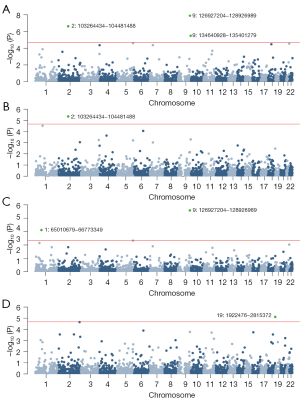
Partitioned genetic correlation
We further partitioned genetic correlation across 14 distinct functional categories, considering the highly positive genetic correlations observed between GERD and LC (Figure 3, Table S2). In 13 of the 14 functional categories, GERD was significantly correlated with overall LC, of which rg values ranged from 0.17 (super enhancer) to 0.38 (conserved regions). Extending to subtype-specific LC, we noted significant associations in 10/14, 12/14, and 12/14 functional categories for LUAD, LUSC, and SCLC, respectively. Notably, the conserved region (rg=0.30), conserved region (rg=0.38), and promotor (rg=0.41) displayed strongest genetic correlation for LUAD, LUSC, and SCLC, respectively.
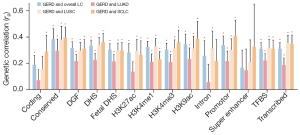
Cross-trait meta-analysis and pleiotropic loci
The strong genetic correlation inspired us to locate pleiotropic loci between GERD and LC by performing CPASSOC. Cross-trait meta-analysis included a total of 2,194,995, 2,197,591, 2,197,145, and 2,202,470 SNPs shared between GERD and overall LC, LUAD, LUSC, and SCLC, respectively. Finally, CPASSOC identified 22 independent loci with genome-wide significance (PCPASSOC <5×10−8 and Psingle-trait <1×10−3), including 14 pleiotropic loci between GERD and overall LC, 4 pleiotropic loci between GERD and LUAD, 8 pleiotropic loci between GERD and LUSC, and 2 pleiotropic loci between GERD and SCLC (Table 2, Figure S1). Near these shared loci, some widely reported oncogenes, such as PTPRF, PBX3, RAB5B, and TCF4 (related SNPs: rs2782641, rs10156445, rs773109, and rs4500831), were observed.
Table 2
SNP | CHR: position | A1/A2 | Beta | Psingle‑trait | PCPASSOC | Gene† | |||
---|---|---|---|---|---|---|---|---|---|
GERD | LC | GERD | LC | ||||||
GERD and over all LC | |||||||||
rs17391694 | Chr1: 78623626 | C/T | −0.04 | 0.11 | 2.54×10−7 | 2.62×10−8 | 7.94×10−10 | – | |
rs2782641 | Chr1: 44013355 | G/A | −0.03 | −0.04 | 4.33×10−8 | 6.26×10−4 | 5.67×10−10 | PTPRF | |
rs6711584 | Chr2: 104421692 | G/A | −0.03 | 0.04 | 2.66×10−11 | 1.52×10−4 | 2.13×10−13 | – | |
rs329122 | Chr5: 133864599 | G/A | 0.03 | −0.05 | 3.05×10−9 | 1.69×10−5 | 2.35×10−11 | JADE2 | |
rs13207689 | Chr6: 27369704 | C/G | 0.05 | 0.14 | 9.32×10−10 | 9.29×10−11 | 1.35×10−14 | ZNF391, RP1-153G14.4 | |
rs13220495 | Chr6: 26441640 | C/T | 0.04 | 0.13 | 1.96×10−8 | 7.74×10−9 | 2.91×10−12 | BTN3A3 | |
rs17526722 | Chr6: 25918855 | G/A | 0.03 | 0.13 | 5.59×10−5 | 1.26×10−8 | 1.58×10−8 | SLC17A2 | |
rs2232423 | Chr6: 28366151 | A/G | 0.05 | 0.15 | 1.37×10−11 | 8.04×10−12 | 2.54×10−17 | ZSCAN12 | |
rs537160‡ | Chr6: 31916400 | A/G | −0.03 | 0.05 | 5.08×10−8 | 3.98×10−5 | 8.46×10−10 | CFB, NELFE, C2, CYP21A2 | |
rs215614 | Chr7: 32347335 | G/A | 0.03 | 0.04 | 4.08×10−11 | 4.29×10−4 | 1.31×10−13 | – | |
rs10156445‡ | Chr9: 128617244 | A/G | −0.02 | −0.04 | 6.33×10−7 | 7.81×10−4 | 1.51×10−8 | PBX3 | |
rs9328534 | Chr9: 134874805 | C/T | 0.03 | 0.04 | 1.35×10−8 | 4.67×10−4 | 1.25×10−10 | MED27 | |
rs773109 | Chr12: 56374695 | G/A | 0.04 | −0.04 | 8.71×10−14 | 5.14×10−4 | 5.40×10−16 | RAB5B, RP11-603J24.7 | |
rs4500831 | Chr18: 53097544 | G/A | 0.03 | 0.05 | 1.21×10−7 | 3.42×10−4 | 1.47×10−9 | TCF4 | |
GERD and LUAD | |||||||||
rs6695572 | Chr1: 77945635 | G/A | −0.02 | 0.12 | 4.09×10−4 | 8.34×10−9 | 2.14×10−8 | AK5 | |
rs17391694‡ | Chr1: 78623626 | C/T | −0.04 | 0.14 | 2.54×10−7 | 3.83×10−7 | 8.34×10−9 | – | |
rs6711584 | Chr2: 104421692 | G/A | −0.03 | 0.07 | 2.66×10−11 | 2.89×10−5 | 8.68×10−13 | – | |
rs537160‡ | Chr6: 31906797 | A/G | −0.03 | 0.06 | 5.08×10−8 | 7.89×10−4 | 8.21×10−9 | CFB, NELFE, C2, CYP21A2 | |
GERD and LUSC | |||||||||
rs2782641 | Chr1: 44013355 | G/A | −0.03 | −0.07 | 4.33×10−8 | 2.76×10−4 | 4.98×10−9 | PTPRF | |
rs329122 | Chr5: 133864599 | G/A | 0.03 | −0.08 | 3.05×10−9 | 2.28×10−5 | 5.12×10−10 | JADE2 | |
rs13191445 | Chr6: 26015489 | G/A | 0.03 | 0.25 | 5.35×10−5 | 1.06×10−11 | 5.56×10−11 | HIST1H1A, HIST1H1PS2, U91328.22 | |
rs9379899 | Chr6: 26603015 | T/A | 0.04 | 0.11 | 1.25×10−9 | 2.17×10−4 | 1.07×10−10 | ABT1 | |
rs3922717 | Chr6: 27030924 | A/G | 0.04 | 0.08 | 5.35×10−13 | 3.75×10−4 | 3.81×10−14 | VN1R13P | |
rs13219181 | Chr6: 27136225 | A/G | 0.03 | 0.11 | 1.32×10−8 | 2.56×10−5 | 7.00×10−10 | – | |
rs200968 | Chr6: 27859568 | T/C | 0.04 | 0.11 | 3.94×10−11 | 4.25×10−5 | 1.62×10−12 | HIST1H2BO, HIST1H3J, HIST1H2AM | |
rs2232426 | Chr6: 28360659 | G/C | 0.05 | 0.22 | 1.39×10−11 | 1.02×10−10 | 1.63×10−14 | ZSCAN12 | |
GERD and SCLC | |||||||||
rs3172494 | Chr3: 48731487 | G/T | 0.05 | −0.15 | 6.71×10−9 | 9.28×10−4 | 5.12×10−9 | IP6K2 | |
rs2232423 | Chr6: 28366151 | A/G | 0.05 | 0.20 | 1.37×10−11 | 2.14×10−4 | 2.02×10−12 | ZSCAN12 |
†, gene symbol mapped by VEP; ‡, novel SNPs, defined as shared SNPs that are neither driven by a single trait nor in LD with index SNPs identified in single-trait GWAS (LD r2<0.2). SNPs, single nucleotide polymorphisms; CPASSOC, cross-phenotypic association; CHR, chromosome; A1, effect allele; A2, alternative allele; GERD, gastroesophageal reflux disease; LC, lung cancer; LUAD, lung adenocarcinoma; LUSC, lung squamous cell carcinoma; SCLC, small-cell lung cancer; VEP, Variant Effect Predictor; LD, linkage disequilibrium; GWAS, genome-wide association study.
After removing loci identified in previously reported single-trait GWASs or loci in LD (r2≥0.2) with previously identified loci, 3 loci were categorized novel pleiotropic loci: 2 shared between GERD and overall LC, and 2 shared between GERD and LUAD, with 1 locus overlapped between overall LC and LUAD. The most significant novel locus was rs537160, which was mapped to complement factor B (CFB), a pivotal component of the alternative signaling pathway in complement activation (55). rs10156445, as the second most significant novel locus, was near PBX3, a member of the PBX family interacting with the promoter of tumor suppressor p53 (54).
Identification of causal variants and colocalization
Using FM-summary algorithm, each of the identified pleiotropic variants established a 99% credible set of causal variants, which offers potential targets for subsequent experimental research (Tables S3-S6). As a result, we identified a set of 352, 42, 219, and 18 causal variants for overall LC, LUAD, LUSC, and SCLC. For the novel pleiotropic loci, we identified 1, 3, 11, 75 causal variants for rs17391694, rs537160, rs537160, and rs10156445, respectively.
To evaluate whether genetic variants influencing the association across traits were shared or distinct, the colocalization analysis was further performed. Approximately a half of pleiotropic loci showed colocalization at same candidate causal variants with PPH4 >0.5: 8/14 between GERD and overall LC, 2/4 between GERD and LUAD, 4/8 between GERD and LUSC, and 1/2 between GERD and SCLC (Table S7).
TWASs
After multiple testing (FDR <0.05) and intersecting the single-trait TWAS results across traits, multiple TWAS-significant gene-tissue pairs shared between GERD and LC were identified, including 30 genes shared between GERD and overall LC, 10 genes shared between GERD and LUAD, 11 genes shared between GERD and LUSC, and 12 genes shared between GERD and SCLC (Tables S8-S11, Figure S2). Among these gene-tissue pairs between GERD and overall LC, ERAP1, FUBP1, and CEP57 were most frequently identified genes and simultaneously discovered in lung tissues and esophagus tissues (i.e., esophagus mucosa, esophagus muscularis, and esophagus gastroesophageal junction). As a member of the M1 family of aminopeptidases, ERAP1 was previously implicated in autoimmunity and signals a role in susceptibility of LC (56). As a versatile DNA and RNA binding protein, FUBP1 plays a role in multiple biological processes, and serves as an oncoprotein associated with multiple malignancies, including LC (57,58). CEP57, a centrosomal protein, is involved in the processes of microtubule nucleation and bundling associated with cell division error and thus potentially promote malignant progression (59,60). Additionally, PBX3, a factor interacting with the promoter of p53 (54), was frequently identified in gene-tissue pairs between GERD and LUSC.
Bidirectional MR
Finally, we evaluated the causal association between GERD and LC by performing a two-sample MR. We identified a total of 91 GERD-associated SNPs as IVs, and F-statistics calculated >10 suggested strong IVs (Table S12). Utilizing the IVW method, GERD was found to be significantly associated with the risk of overall LC (OR =1.34, P=1.33×10−6), which remained consistent in weight median (OR =1.28, P=2.03×10−4), MR-PRESSO (OR =1.37, P=1.51×10−7), and MR-CAUSE (OR =1.30, P=6.11×10−3) (Figure 4, Table S13, Figures S3-S6). The estimates continued to be directionally consistent with MR-Egger regression, despite no significance (OR =1.08, P=0.82). Consistent results were also observed after excluding pleiotropic SNPs (OR =1.30, P=1.34×10−5) or palindromic SNPs (OR =1.40, P=2.80×10−7). No significant horizontal pleiotropy was observed (PMR-Egger intercept =0.54), and leave-one-out analyses detected no obvious outlying variants (Figure S7). Looking into the subtype-specific LC, significant causal associations also were identified in LUAD (IVW OR =1.25, P=2.71×10−3), LUSC (IVW OR =1.52, P=9.59×10−9), and SCLC (IVW OR =1.76, P=1.27×10−5), which were further confirmed in sensitivity analyses except MR-Egger regression. Additionally, the power of all MR analyses was calculated to be 100% using estimates from IVW, suggesting a satisfactory statistical power (Table S14). Potential confounders were accounted for using MVMR, yielding estimates that exhibit a more pronounced magnitude and statistical significance, which suggests that the causal relationship between GER and LC remains independent of common confounding factors (Figure S8).
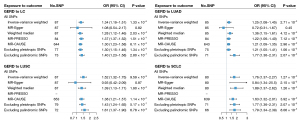
In the reverse-direction MR analysis, we identified a total of 14, 15, 13, and 4 SNPs for overall LC, LUAD, LUSC, and SCLC as IVs, with all F-statistics >10 suggesting strong IVs (Table S15). We observed no significant causal effect of LC on GERD: overall LC (IVW OR =1.02, P=0.24), LUAD (IVW OR =1.00, P=0.95), LUAD (IVW OR =0.99, P=0.46), and SCLC (IVW OR =0.98, P=0.27) (Figure 5).
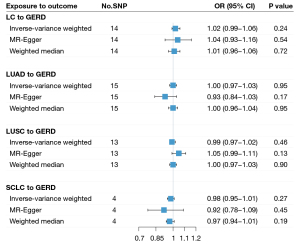
Discussion
As far as we know, this genome-wide cross-trait analysis represents the first comprehensive investigation into the genetic correlation, pleiotropic loci, association between gene expression and trait, and causal relationship between GERD and LC, providing valuable insights into this complex genetic interplay. Our findings revealed a significantly genetic correlation underlying GERD and overall LC. After partitioning the whole genome, significant genetic correlations were identified within five genomic regions and multiple functional categories (e.g., conserved region, and promotor). The underlying genetic link was further divided into two categories: pleiotropy and causality, corresponding with the identified pleiotropic loci from CPASSOC, the shared genes from TWAS, and the causal association through the bidirectional MR analysis. For subtype-specific LC, similar results were also observed for LUAD, LUSC, and SCLC. Taken together, these findings advance our understanding of the intricate link between a digestive disease and a respiratory malignancy, offering valuable implications for LC prevention in individuals with GERD.
Despite a significant global genetic correlation detected in LDSC, the estimated intercept of genetic covariance ranging from 0.001 to 0.008 suggested a presence of potential bias from sample overlap. Therefore, we employed LDSC with a constrained intercept to address this issue, and similarly, detected a significant global genetic correlation between GERD and LC (26). When separating the entire genome into approximately 2,353 distinct regions, a significant local genetic correlation between GERD and overall LC, as well as LUSC, was identified, specifically at 9q33.3. This genomic region contains PBX3, which has previously been reported to be associated with GERD and LC (12,53,54). Furthermore, we observed significant genetic correlations in multiple annotated regions of the genome using stratified LDSC. Notably, the conserved region exhibited the highest partitioned rg, while other non-coding regions, including specific histone modification marks, such as H3K4me1 and H3K27me3, and histone acetylation marks, such as H3K27ac, H3K9ac, also showed significant partitioned rg. These findings align with prior studies highlighting the crucial role of epigenetic modification in LC development (61,62).
Through the cross-trait meta-analysis, we revealed 22 pleiotropic loci between GERD and LC, among which 18 loci have been reported to be associated with either one or both traits. For instance, the shared SNP rs4500831 (18q21.2) showed LD with rs1942262 (r2=0.21) previously identified in the GWAS study of GERD (12), which was mapped to TCF4 implicated in the development of LC (63). Additionally, several pleiotropic loci were mapped to genes associated with risks of various carcinomas, such as PTPRF, JADE2, SLC17A2, MED27, and RAB5B. Multiple genes, including PTPRF, JADE2, ZNF391, SLC17A2, MED27, RAB5B, and ZSCAN12, exhibited significant evidence of colocalization (PPH4 >0.5), indicating etiological correlations. Cross-trait meta-analysis has the advantage of revealing signals that have not reached genome-wide significance in a single-trait analysis (64). Within these loci in our study, we identified four novel loci associated with both GERD and LC, among which we highlight two genes (CFB and NELFE), both mapped by the same locus (index SNP: rs537160).
CFB is a factor that binds C3 to form C3B in the alternative pathway, playing a pivotal role in labeling target particles and thereby contributing to effective target clearance (65). Through the integration of proteomic analysis, CFB has been identified as a potential biomarker for pancreatic cancer (66). Also, a recent study found that elevated CFB expression serves as an independent predictor of long-term survival of LUAD (65). Furthermore, complementary pathway may play a critical role in the development of GERD. Previous studies reported that the transcription factor NF-κB is associated with the development of GERD, and the activation of NF-κB is mediated through the alternative pathway (67,68). These observations underscore the potential etiology of CFB underlying GERD and LC.
Additionally, NELFE is RNA-binding protein that plays a role in tumor biology and progression (69,70). Prior study has revealed that NELFE has the potential to induce hepatocellular carcinoma by regulating the MYC signaling pathway (71). Furthermore, NELFE may promote the tumorigenesis and metastasis of pancreatic cancer via the Wnt/β-catenin signaling pathway (72). Through the whole-exome sequencing of early non-smokers with LUAD, NELFE was also identified as a candidate driver marker (73). Nevertheless, further study is warranted to validate and explore the biological mechanism of NELFE in the tumorigenesis of LC.
The TWAS analysis evaluated pleiotropy at the level of gene expression by combining GTEx tissue-specific expression data and GWAS summary data. Specifically, both CPASSOC and TWAS identified PTPRF and PBX3 as relevant genes. Furthermore, PBX3, located at 9q33.3, was also identified in the local genetic correlation analysis. Two shared genes, CEP57 and FUBP1, were also identified by TWAS, and have been reported to have a direct or indirect association with GERD and LC (12,56,58). In summary, these shared biological targets between GERD and LC suggest potential therapeutic strategies for the coexisting groups in clinical practice. Further studies are warranted to elucidate the underlying mechanisms.
Utilizing a comprehensive bidirectional MR analysis, our results revealed a significant causal association between GERD and LC, further extending to subtype-specific LC. Of note, the strength of the causal estimates between GERD and LC largely aligns with the genetic correlation; specifically, the correlation is strongest between GERD and LUSC/SCLC, whereas the correlation with LUAD is the weakest. Compared with prior MR studies, our research significantly advances previous findings in several crucial aspects (18-20). We leveraged the GWAS of LC with an expanded sample size, substantially enhancing the statistical ability to discover causal relationships. For example, the causal association between GERD and SCLC was not discovered from the MR study by Liu et al., who utilized limited sample size GWAS data from the FinnGen database (only 461 cases) (18). Additionally, the sensitivity analyses were performed to scrutinize the assumptions of MR, thereby offering further support for the reliability of our main findings. To ensure causal estimates independent of potential confounding factors, comprehensive MVMR analyses were carried out. Through a reverse directional MR design, we found no significant causal association of genetically predicted LC on the risk of GERD, which had not been previously explored in prior MR studies (18-20). Taken together, the estimated causal effects were consistently affirmed among multiple sensitivity analyses and statistical approaches, indicating its robustness. In line with our findings, previous population-based epidemiologic studies also reported positive associations of GERD and LC (9,10,74). Interestingly, a large-scale cohort study reported that anti-reflux surgery led to a significant reduction in the risk of LUSC [standardized incidence ratio (SIR) =0.75, 95% CI: 0.60–0.92] and SCLC (SIR =0.63, 95% CI: 0.44–0.90), with a protective trend in LUAD (SIR =0.80, 95% CI: 0.62–1.03) (10). These observations closely resemble the findings of our study, finding a significantly positive causal effect between GERD and LUSC/SCLC, while the correlation with LUAD is marginally significant.
Several limitations in the current study should be acknowledged. Firstly, to mitigate potential bias from population stratification, we focused exclusively on individuals of European ancestry for our genetic data. However, it is important to note that the incidence of GERD may exhibit racial disparities (75), suggesting the need for further research to validate the generalizability of our findings in other ethnic populations. Secondly, our study was limited to data from autosomes due to existing limitations in the analytical software, which does not support the analysis of sex chromosomes. Thirdly, while we mapped pleiotropic SNPs to relevant genes, further investigations are warranted to pinpoint the causal genes responsible for the observed signals. Finally, our study relied on summary-level data rather than individual-level data, determined by data limitations. While summary-level data provide a larger sample size, leading to increased statistical power in causal estimates (42), it is important to acknowledge its drawbacks. Compared with individual-level data, summary-level data do not account for some important confounders for each individual, such as local socioeconomic, medical situations, and other factors.
Conclusions
In summary, using a novel statistical genetic approach based on the hitherto largest GWAS summary data, the study sheds light on the observational association between GERD and LC. These findings provide valuable evidence of genetic correlation, identifying pleiotropic loci, and suggesting a potential causal association between GERD and LC. This study conveys a crucial public health message: managing individuals with GERD may potentially contribute to reducing the long-term burden of malignant diseases.
Acknowledgments
Funding: This study was supported by
Footnote
Reporting Checklist: The authors have completed the STREGA reporting checklist. Available at https://tlcr.amegroups.com/article/view/10.21037/tlcr-24-345/rc
Peer Review File: Available at https://tlcr.amegroups.com/article/view/10.21037/tlcr-24-345/prf
Conflicts of Interest: All authors have completed the ICMJE uniform disclosure form (available at https://tlcr.amegroups.com/article/view/10.21037/tlcr-24-345/coif). The authors have no conflicts of interest to declare.
Ethical Statement: The authors are accountable for all aspects of the work in ensuring that questions related to the accuracy or integrity of any part of the work are appropriately investigated and resolved. The ethical approval for each summary-level data can be found from the corresponding studies. The study was conducted in accordance with the Declaration of Helsinki (as revised in 2013).
Open Access Statement: This is an Open Access article distributed in accordance with the Creative Commons Attribution-NonCommercial-NoDerivs 4.0 International License (CC BY-NC-ND 4.0), which permits the non-commercial replication and distribution of the article with the strict proviso that no changes or edits are made and the original work is properly cited (including links to both the formal publication through the relevant DOI and the license). See: https://creativecommons.org/licenses/by-nc-nd/4.0/.
References
- Vakil N, van Zanten SV, Kahrilas P, et al. The Montreal definition and classification of gastroesophageal reflux disease: a global evidence-based consensus. Am J Gastroenterol 2006;101:1900-20; quiz 1943. [Crossref] [PubMed]
- The global, regional, and national burden of gastro-oesophageal reflux disease in 195 countries and territories, 1990-2017: a systematic analysis for the Global Burden of Disease Study 2017. Lancet Gastroenterol Hepatol 2020;5:561-81. [Crossref] [PubMed]
- Broers C, Tack J, Pauwels A. Review article: gastro-oesophageal reflux disease in asthma and chronic obstructive pulmonary disease. Aliment Pharmacol Ther 2018;47:176-91. [Crossref] [PubMed]
- Hayes D Jr. A review of bronchiolitis obliterans syndrome and therapeutic strategies. J Cardiothorac Surg 2011;6:92. [Crossref] [PubMed]
- Gaillet G, Favelle O, Guilleminault L, et al. Gastroesophageal reflux disease is a risk factor for severity of organizing pneumonia. Respiration 2015;89:119-26. [Crossref] [PubMed]
- Baqir M, Vasirreddy A, Vu AN, et al. Idiopathic pulmonary fibrosis and gastroesophageal reflux disease: A population-based, case-control study. Respir Med 2021;178:106309. [Crossref] [PubMed]
- Herbella FA, Neto SP, Santoro IL, et al. Gastroesophageal reflux disease and non-esophageal cancer. World J Gastroenterol 2015;21:815-9. [Crossref] [PubMed]
- Siegel RL, Miller KD, Jemal A. Cancer statistics, 2019. CA Cancer J Clin 2019;69:7-34. [Crossref] [PubMed]
- Hsu CK, Lai CC, Wang K, et al. Risk of lung cancer in patients with gastro-esophageal reflux disease: a population-based cohort study. PeerJ 2016;4:e2753. [Crossref] [PubMed]
- Yanes M, Santoni G, Maret-Ouda J, et al. Antireflux surgery and risk of lung cancer by histological type in a multinational cohort study. Eur J Cancer 2020;138:80-8. [Crossref] [PubMed]
- Zhu Z, Hasegawa K, Camargo CA Jr, et al. Investigating asthma heterogeneity through shared and distinct genetics: Insights from genome-wide cross-trait analysis. J Allergy Clin Immunol 2021;147:796-807. [Crossref] [PubMed]
- Ong JS, An J, Han X, et al. Multitrait genetic association analysis identifies 50 new risk loci for gastro-oesophageal reflux, seven new loci for Barrett's oesophagus and provides insights into clinical heterogeneity in reflux diagnosis. Gut 2022;71:1053-61. [Crossref] [PubMed]
- Wang C, Dai J, Qin N, et al. Analyses of rare predisposing variants of lung cancer in 6,004 whole genomes in Chinese. Cancer Cell 2022;40:1223-1239.e6. [Crossref] [PubMed]
- Cameron AJ, Lagergren J, Henriksson C, et al. Gastroesophageal reflux disease in monozygotic and dizygotic twins. Gastroenterology 2002;122:55-9. [Crossref] [PubMed]
- Mohammed I, Cherkas LF, Riley SA, et al. Genetic influences in gastro-oesophageal reflux disease: a twin study. Gut 2003;52:1085-9. [Crossref] [PubMed]
- Mucci LA, Hjelmborg JB, Harris JR, et al. Familial Risk and Heritability of Cancer Among Twins in Nordic Countries. JAMA 2016;315:68-76. [Crossref] [PubMed]
- Lichtenstein P, Holm NV, Verkasalo PK, et al. Environmental and heritable factors in the causation of cancer--analyses of cohorts of twins from Sweden, Denmark, and Finland. N Engl J Med 2000;343:78-85. [Crossref] [PubMed]
- Liu Y, Lai H, Zhang R, et al. Causal relationship between gastro-esophageal reflux disease and risk of lung cancer: insights from multivariable Mendelian randomization and mediation analysis. Int J Epidemiol 2023;52:1435-47. [Crossref] [PubMed]
- Li L, Ren Q, Zheng Q, et al. Causal associations between gastroesophageal reflux disease and lung cancer risk: A Mendelian randomization study. Cancer Med 2023;12:7552-9. [Crossref] [PubMed]
- Yang J, Nie D, Chen Y, et al. The role of smoking and alcohol in mediating the effect of gastroesophageal reflux disease on lung cancer: A Mendelian randomization study. Front Genet 2022;13:1054132. [Crossref] [PubMed]
- Smith GD, Ebrahim S. 'Mendelian randomization': can genetic epidemiology contribute to understanding environmental determinants of disease? Int J Epidemiol 2003;32:1-22. [Crossref] [PubMed]
- Solovieff N, Cotsapas C, Lee PH, et al. Pleiotropy in complex traits: challenges and strategies. Nat Rev Genet 2013;14:483-95. [Crossref] [PubMed]
- Zhu Z, Lee PH, Chaffin MD, et al. A genome-wide cross-trait analysis from UK Biobank highlights the shared genetic architecture of asthma and allergic diseases. Nat Genet 2018;50:857-64. [Crossref] [PubMed]
- Little J, Higgins JP, Ioannidis JP, et al. STrengthening the REporting of Genetic Association studies (STREGA): an extension of the STROBE Statement. Ann Intern Med 2009;150:206-15. [Crossref] [PubMed]
- McKay JD, Hung RJ, Han Y, et al. Large-scale association analysis identifies new lung cancer susceptibility loci and heterogeneity in genetic susceptibility across histological subtypes. Nat Genet 2017;49:1126-32. [Crossref] [PubMed]
- Bulik-Sullivan B, Finucane HK, Anttila V, et al. An atlas of genetic correlations across human diseases and traits. Nat Genet 2015;47:1236-41. [Crossref] [PubMed]
- Zhang Y, Lu Q, Ye Y, et al. SUPERGNOVA: local genetic correlation analysis reveals heterogeneous etiologic sharing of complex traits. Genome Biol 2021;22:262. [Crossref] [PubMed]
- Finucane HK, Bulik-Sullivan B, Gusev A, et al. Partitioning heritability by functional annotation using genome-wide association summary statistics. Nat Genet 2015;47:1228-35. [Crossref] [PubMed]
- Bernstein BE, Stamatoyannopoulos JA, Costello JFThe NIH Roadmap Epigenomics Mapping Consortium, et al. Nat Biotechnol 2010;28:1045-8. [Crossref] [PubMed]
- Li X, Zhu X. Cross-Phenotype Association Analysis Using Summary Statistics from GWAS. Methods Mol Biol 2017;1666:455-67. [Crossref] [PubMed]
- Purcell S, Neale B, Todd-Brown K, et al. PLINK: a tool set for whole-genome association and population-based linkage analyses. Am J Hum Genet 2007;81:559-75. [Crossref] [PubMed]
- Qu Y, Xiao C, Wu X, et al. Genetic Correlation, Shared Loci, and Causal Association Between Sex Hormone-Binding Globulin and Bone Mineral Density: Insights From a Large-Scale Genomewide Cross-Trait Analysis. J Bone Miner Res 2023;38:1635-44. [Crossref] [PubMed]
- McLaren W, Gil L, Hunt SE, et al. The Ensembl Variant Effect Predictor. Genome Biol 2016;17:122. [Crossref] [PubMed]
- Schaid DJ, Chen W, Larson NB. From genome-wide associations to candidate causal variants by statistical fine-mapping. Nat Rev Genet 2018;19:491-504. [Crossref] [PubMed]
- Wu X, Xiao C, Han Z, et al. Investigating the shared genetic architecture of uterine leiomyoma and breast cancer: A genome-wide cross-trait analysis. Am J Hum Genet 2022;109:1272-85. [Crossref] [PubMed]
- Farh KK, Marson A, Zhu J, et al. Genetic and epigenetic fine mapping of causal autoimmune disease variants. Nature 2015;518:337-43. [Crossref] [PubMed]
- Giambartolomei C, Vukcevic D, Schadt EE, et al. Bayesian test for colocalisation between pairs of genetic association studies using summary statistics. PLoS Genet 2014;10:e1004383. [Crossref] [PubMed]
- Gusev A, Ko A, Shi H, et al. Integrative approaches for large-scale transcriptome-wide association studies. Nat Genet 2016;48:245-52. [Crossref] [PubMed]
- The Genotype-Tissue Expression (GTEx) project. Nat Genet 2013;45:580-5. [Crossref] [PubMed]
- Burgess S, Thompson SG. Avoiding bias from weak instruments in Mendelian randomization studies. Int J Epidemiol 2011;40:755-64. [Crossref] [PubMed]
- Brion MJ, Shakhbazov K, Visscher PM. Calculating statistical power in Mendelian randomization studies. Int J Epidemiol 2013;42:1497-501. [Crossref] [PubMed]
- Burgess S, Scott RA, Timpson NJ, et al. Using published data in Mendelian randomization: a blueprint for efficient identification of causal risk factors. Eur J Epidemiol 2015;30:543-52. [Crossref] [PubMed]
- Bowden J, Davey Smith G, Burgess S. Mendelian randomization with invalid instruments: effect estimation and bias detection through Egger regression. Int J Epidemiol 2015;44:512-25. [Crossref] [PubMed]
- Bowden J, Davey Smith G, Haycock PC, et al. Consistent Estimation in Mendelian Randomization with Some Invalid Instruments Using a Weighted Median Estimator. Genet Epidemiol 2016;40:304-14. [Crossref] [PubMed]
- Morrison J, Knoblauch N, Marcus JH, et al. Mendelian randomization accounting for correlated and uncorrelated pleiotropic effects using genome-wide summary statistics. Nat Genet 2020;52:740-7. [Crossref] [PubMed]
- Verbanck M, Chen CY, Neale B, et al. Detection of widespread horizontal pleiotropy in causal relationships inferred from Mendelian randomization between complex traits and diseases. Nat Genet 2018;50:693-8. [Crossref] [PubMed]
- Kamat MA, Blackshaw JA, Young R, et al. PhenoScanner V2: an expanded tool for searching human genotype-phenotype associations. Bioinformatics 2019;35:4851-3. [Crossref] [PubMed]
- Burgess S, Thompson SG. Multivariable Mendelian randomization: the use of pleiotropic genetic variants to estimate causal effects. Am J Epidemiol 2015;181:251-60. [Crossref] [PubMed]
- Pulit SL, Stoneman C, Morris AP, et al. Meta-analysis of genome-wide association studies for body fat distribution in 694 649 individuals of European ancestry. Hum Mol Genet 2019;28:166-74. [Crossref] [PubMed]
- Liu M, Jiang Y, Wedow R, et al. Association studies of up to 1.2 million individuals yield new insights into the genetic etiology of tobacco and alcohol use. Nat Genet 2019;51:237-44. [Crossref] [PubMed]
- Klimentidis YC, Raichlen DA, Bea J, et al. Genome-wide association study of habitual physical activity in over 377,000 UK Biobank participants identifies multiple variants including CADM2 and APOE. Int J Obes (Lond) 2018;42:1161-76. [Crossref] [PubMed]
- Dashti HS, Jones SE, Wood AR, et al. Genome-wide association study identifies genetic loci for self-reported habitual sleep duration supported by accelerometer-derived estimates. Nat Commun 2019;10:1100. [Crossref] [PubMed]
- Plowright L, Harrington KJ, Pandha HS, et al. HOX transcription factors are potential therapeutic targets in non-small-cell lung cancer (targeting HOX genes in lung cancer). Br J Cancer 2009;100:470-5. [Crossref] [PubMed]
- Li WF, Herkilini A, Tang Y, et al. The transcription factor PBX3 promotes tumor cell growth through transcriptional suppression of the tumor suppressor p53. Acta Pharmacol Sin 2021;42:1888-99. [Crossref] [PubMed]
- Ricklin D, Hajishengallis G, Yang K, et al. Complement: a key system for immune surveillance and homeostasis. Nat Immunol 2010;11:785-97. [Crossref] [PubMed]
- Babaie F, Hosseinzadeh R, Ebrazeh M, et al. The roles of ERAP1 and ERAP2 in autoimmunity and cancer immunity: New insights and perspective. Mol Immunol 2020;121:7-19. [Crossref] [PubMed]
- Debaize L, Troadec MB. The master regulator FUBP1: its emerging role in normal cell function and malignant development. Cell Mol Life Sci 2019;76:259-81. [Crossref] [PubMed]
- Byun J, Han Y, Li Y, et al. Cross-ancestry genome-wide meta-analysis of 61,047 cases and 947,237 controls identifies new susceptibility loci contributing to lung cancer. Nat Genet 2022;54:1167-77. [Crossref] [PubMed]
- Mang J, Korzeniewski N, Dietrich D, et al. Prognostic Significance and Functional Role of CEP57 in Prostate Cancer. Transl Oncol 2015;8:487-96. [Crossref] [PubMed]
- Snape K, Hanks S, Ruark E, et al. Mutations in CEP57 cause mosaic variegated aneuploidy syndrome. Nat Genet 2011;43:527-9. [Crossref] [PubMed]
- Li W, Tian W, Yuan G, et al. Molecular basis of nucleosomal H3K36 methylation by NSD methyltransferases. Nature 2021;590:498-503. [Crossref] [PubMed]
- Sengupta D, Zeng L, Li Y, et al. NSD2 dimethylation at H3K36 promotes lung adenocarcinoma pathogenesis. Mol Cell 2021;81:4481-4492.e9. [Crossref] [PubMed]
- Zhang HJ, Chang WJ, Jia CY, et al. Destrin Contributes to Lung Adenocarcinoma Progression by Activating Wnt/β-Catenin Signaling Pathway. Mol Cancer Res 2020;18:1789-802. [Crossref] [PubMed]
- Zhu Z, Anttila V, Smoller JW, et al. Statistical power and utility of meta-analysis methods for cross-phenotype genome-wide association studies. PLoS One 2018;13:e0193256. [Crossref] [PubMed]
- He C, Li Y, Zhang R, et al. Low CFB expression is independently associated with poor overall and disease-free survival in patients with lung adenocarcinoma. Oncol Lett 2021;21:478. [Crossref] [PubMed]
- Lee MJ, Na K, Jeong SK, et al. Identification of human complement factor B as a novel biomarker candidate for pancreatic ductal adenocarcinoma. J Proteome Res 2014;13:4878-88. [Crossref] [PubMed]
- Zhang ML, Ran LQ, Wu MJ, et al. NF-κB: A novel therapeutic pathway for gastroesophageal reflux disease? World J Clin Cases 2022;10:8436-42. [Crossref] [PubMed]
- Liu T, Zhang L, Joo D, et al. NF-κB signaling in inflammation. Signal Transduct Target Ther 2017;2:17023. [Crossref] [PubMed]
- Roesch A, Becker B, Meyer S, et al. Retinoblastoma-binding protein 2-homolog 1: a retinoblastoma-binding protein downregulated in malignant melanomas. Mod Pathol 2005;18:1249-57. [Crossref] [PubMed]
- Hodson DJ, Screen M, Turner M. RNA-binding proteins in hematopoiesis and hematological malignancy. Blood 2019;133:2365-73. [Crossref] [PubMed]
- Dang H, Pomyen Y, Martin SP, et al. NELFE-Dependent MYC Signature Identifies a Unique Cancer Subtype in Hepatocellular Carcinoma. Sci Rep 2019;9:3369. [Crossref] [PubMed]
- Han L, Zan Y, Huang C, et al. NELFE promoted pancreatic cancer metastasis and the epithelial‑to‑mesenchymal transition by decreasing the stabilization of NDRG2 mRNA. Int J Oncol 2019;55:1313-23. [Crossref] [PubMed]
- Renieri A, Mencarelli MA, Cetta F, et al. Oligogenic germline mutations identified in early non-smokers lung adenocarcinoma patients. Lung Cancer 2014;85:168-74. [Crossref] [PubMed]
- Amarnath S, Starr A, Chukkalore D, et al. The Association Between Gastroesophageal Reflux Disease and Non-Small Cell Lung Cancer: A Retrospective Case-Control Study. Gastroenterology Res 2022;15:173-9. [Crossref] [PubMed]
- Richter JE, Rubenstein JH. Presentation and Epidemiology of Gastroesophageal Reflux Disease. Gastroenterology 2018;154:267-76. [Crossref] [PubMed]