Molecular subtypes and prognostic factors of lung large cell neuroendocrine carcinoma
Highlight box
Key findings
• Molecular subtypes by immunohistochemistry (IHC) staining were validated, co-expression was found among different subtypes, with no prognostic value.
• Blood lymphocyte-to-monocyte ratio (LMR) was an independent prognostic predictor of disease-free survival (DFS) and overall survival (OS), high blood LMR might partly reflect a hot tumor tissue immune microenvironment (high intra-tumoral CD3+, CD8+, and CD68+ immune cell infiltration).
What is known and what is new?
• Previously studies have reported the molecular subtypes defined by IHC, co-expression was found among different subtypes.
• It was the first time to confirm that there is no prognosis effect of molecular subtypes in lung large cell neuroendocrine carcinoma (LCNEC). However, high LMR was associated with a favorable prognosis in lung LCNEC patients, which was correlated with higher intra-tumoral CD3+, CD8+, and CD68+ immune cell infiltration.
What is the implication and what should change now?
• No prognosis difference among different molecular subtypes was observed, it is necessary to explore other prognostic markers in lung LCNEC.
• High blood LMR was a favorable prognostic indicator for DFS and OS in lung LCNEC, which might partly reflect a hot tumor immune microenvironment, suggesting that LMR might be a new candidate to predict prognosis and indicate tumor immune status in lung LCNEC.
Introduction
Lung large cell neuroendocrine carcinoma (LCNEC) is a rare and aggressive malignant tumor that accounts for ~3% of lung cancer. Additionally, it is classified as high-grade neuroendocrine (NE) carcinoma and characterized by a close association with smoking and with high metastasis potential (1-3). The diagnosis of lung LCNEC is based on NE morphology, cytological features of non-small cell lung cancer (NSCLC), high proliferation rate, and NE marker expression (1,4). The prognosis of lung LCNEC is poor, and therapeutic means are currently limited (3,5-8). Therefore, identifying new promising biomarkers involved in the prognosis of lung LCENC is necessary, as they may contribute to identifying high-risk populations and therapeutic interventions.
Lung LCNEC patients were categorized into two types: type I showing overlapping key genes with lung adenocarcinoma or squamous cell carcinoma in genome analysis but with a high NE expression in transcriptome analysis (9). In contrast, type II showed overlapping key genes with small cell lung cancer (SCLC) but with a low NE expression in transcriptome analysis (9). This reminds us that genome-based typing of lung LCNEC may not be consistent with its histological features of NE differentiation. Another study suggested that different histological types of lung cancer (including lung LCNEC) are associated with specific transcriptome characteristics rather than genome characteristics, highlighting the importance of exploring the molecular biological features at the gene expression level in lung cancer (10).
SCLC subtypes could be defined by the expression of ASCL1, NEUROD1, POU2F3, and YAP1 transcription factors using transcriptome data (11), which has been verified in clinical specimens at the protein level (12-14). The expression of ASCL1, NEUROD1, POU2F3, and YAP1 are likely associated with the prognosis of SCLC (15-18). In lung LCNEC, relatively few studies have systematically analyzed the four transcription factors (19,20), the prognostic differences among different molecular subtypes were unclear. Thus, we comprehensively evaluated the expression patterns and prognosis of ASCL1, NEUROD1, POU2F3, and YAP1 in lung LCNEC in the present study.
Systemic inflammation has been shown to correlate with pathogenesis, disease progression, and prognosis across various tumors (21,22). Peripheral blood lymphocyte-to-monocyte ratio (LMR), neutrophil-to-lymphocyte ratio (NLR), platelet-to-lymphocyte ratio (PLR) and systemic immune-inflammation index (SII) were widely used as systemic inflammatory indicators (23,24). Tumor-infiltrating lymphocytes (TILs) and macrophages (TIMs) are essential components of the tumor immune microenvironment (TIME), which is formed by peripheral blood lymphocytes and monocytes infiltrating into tumor tissue through systemic circulation and exerting anti- and pro-tumor effects (25,26). In lung LCNEC, evidence on the relationship between these inflammatory indicators and prognosis was limited (27-29).
This study’s objectives were: (I) to investigate the protein expression patterns of ASCL1, NEUROD1, POU2F3, and YAP1 and explore their prognosis; (II) to evaluate the prognostic value of inflammatory indicators and their relationship with tissue TIL/TIM status. We present this article in accordance with the STROBE reporting checklist (available at https://tlcr.amegroups.com/article/view/10.21037/tlcr-24-292/rc).
Methods
Study population
From November 2007 to January 2021, patients diagnosed with lung LCNEC at Sun Yat-sen University Cancer Center (SYSUCC) were all identified in this study. Mixed lung LCNEC was defined as lung LCNEC mixed with NSCLC (adenocarcinoma/squamous cell carcinoma) or SCLC (4). The patients’ tissue specimens were collected if available. The inclusion criteria included age >18 years, no previous treatment for lung LCNEC, and complete clinical information. Exclusion criteria included multiple primary cancers (≥1 primary cancer of other organs except for lung) within 5 years, incomplete clinical information, and lack of baseline blood routine and biochemical test. The study was approved by the Institutional Review Board of SYSUCC (ID: B2021-030-01), and written informed consent was obtained from each participant. The study was conducted in accordance with the Declaration of Helsinki (as revised in 2013).
Information extraction and follow-up
Demographic and clinical information were collected from electronic medical records, incorporating age, sex, smoking status, Eastern Cooperative Oncology Group performance status (ECOG-PS), tumor stage, treatment means, and body mass index (BMI). Lymphocyte count, monocyte count, neutrophil count, platelet count, C-reactive protein (CRP), lactate dehydrogenase (LDH), and albumin (ALB) values were collected from blood samples and biochemical reports within 3 days before initial therapy. LMR was calculated as the lymphocyte count divided by monocyte count. NLR was calculated as the neutrophil count divided by lymphocyte count. PLR was calculated as the platelet count divided by lymphocyte count. SII was calculated by multiplying the platelet count with neutrophil count and dividing it by lymphocyte count (30). Individuals who had smoked ≥100 cigarettes in their lifetime were classified as current or ever smokers. Furthermore, tumor staging followed the American Joint Committee on Cancer (AJCC) eighth edition staging manual (31).
Telephone follow-up was conducted every 6 to 12 months until death or loss of follow-up. Tumor response assessment was performed every 2 cycles of chemotherapy or at every 3 months interval following radical surgery by computed tomography (CT), according to Response Evaluation Criteria in Solid Tumor (RECIST) 1.1 (32,33).
Immunohistochemistry (IHC) staining
Tissue sections were deparaffinized with xylene, hydrated with ethyl alcohol, and washed in phosphate-buffered saline (PBS), followed by antigen repair. Sections were treated with 3% H2O2 for 10 minutes to quench endogenous peroxidase activity. Primary antibodies of ASCL1, NEUROD1, POU2F3, YAP1, CD3, CD8, CD20, CD68, CD163, CD56, Syn, and CgA (source, clone and working dilution detailed in Table S1) were incubated 1 h at 37 ℃, washed in PBS twice for 3 minutes. Secondary antibodies were incubated for 45 minutes for ASCL1, NEUROD1, POU2F3, and YAP1, and were incubated for 20 minutes with CD3, CD8, CD20, CD68, CD163, CD56, Syn, and CgA at room temperature, washed in PBS and stained by 3,3'-diaminobenzidine (DAB).
Images of all stained slides were acquired using the digital pathology slide scanner (KFBIO, Ningbo Jiangfeng Biological Information Technology Co., Ltd., Ningbo, China) at 40× resolution and analyzed by K-viewer software (version 1.5.3.1; KFBIO). Two pathologists completed an independent evaluation of IHC staining results. CD56, Syn, and CgA were considered positive when there was ≥1% membrane staining of tumor cells. Histological score (H-score) was measured to evaluate ASCL1, NEUROD1, POU2F3, and YAP1 expression. H-score was derived by multiplying nuclear staining intensity (0, negative; 1, weak; 2, moderate; 3, strong; 4, very strong) by percentage of positive nuclei (1–100%). Additionally, the density of immune cell infiltration was evaluated via QuPath (version 0.3.2) software. Intra-tumoral immune cell infiltration was considered immune cells directly contacting tumor cells. In contrast, infiltration of stromal immune cells was considered immune cells that did not directly contact tumor cells. Positive immune cell density was counted in the average value of five randomly chosen intra-tumoral or stromal regions (0.1 mm2/per region).
Statistical analysis
Differences in clinicopathological features of different groups were compared using Chi-squared tests, continuity corrections, or Fisher’s exact test for categorical variables. Two independent sample t-test/one-way analysis of variance (ANOVA) test/Mann-Whitney test/Kruskal-Wallis test were used for continuity variables. Disease-free survival (DFS) was defined as the time from lung resection until the first disease progression or death of any cause or loss to follow-up. Overall survival (OS) was defined as the time from pathological diagnosis until the death of any cause or loss to follow-up. The Kaplan-Meier method performed DFS and OS survival curves with the log-rank test. Univariate and multivariate analyses of DFS and OS were analyzed using the Cox proportional hazards model. A two-sides of P<0.05 was considered significant. All the above statistical analyses were conducted using GraphPad Prism (version 8.0).
Results
Clinicopathological characteristics
A total of 151 lung LCNEC patients at SYSUCC were enrolled, of whom 41 had incomplete clinical information, 3 had multiple primary cancers within 5 years, and 4 did not have available blood routine and biochemistry test within 3 days before initial treatment. Finally, 103 lung LCNEC patients were included in this study (Figure S1). Tumor tissue specimens were available from 64 patients, and all specimens were surgical resected primary tumor lesions. The median age of the whole cohort was 60 years (range, 37–80 years). Sixty-eight patients (66.0%) were diagnosed as pure LCNEC, and 35 patients (34.0%) were diagnosed with mixed LCNEC, including 25 (24.3%) mixed with NSCLC and 10 (9.7%) mixed with SCLC. The study population was 87.4% (90/103) male, 74.8% (77/103) current or ever smokers, and all had a favorable ECOG-PS of 0–1. The stage at initial diagnosis was stage I in 35, stage II in 26, stage III in 32, and stage IV in 10 cases. Of the entire cohort, 92 patients (89.3%) underwent lung cancer resection, and 52 patients (50.4%) were treated with platinum-based chemotherapy, including paclitaxel/etoposide/pemetrexed plus platinum regimens (Table S2).
Expression of ASCL1, NEUROD1, YAP1 and POU2F3
Using a cutoff H-score of 0, positivity for ASCL1, NEUROD1, and YAP1 were detected in 53 (82.8%), 32 (50.0%) and 18 (28.1%) patients, respectively (Figure 1A,1B). No POU2F3 positive staining was detected in our study. The average H-score for ASCL1 was 145 (range, 10–360), NEUROD1 was 113 (range, 10–320), and YAP1 was 74 (range, 5–180) (Figure 1B).
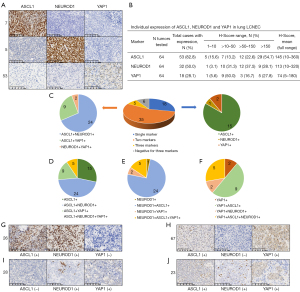
The co-expression pattern of ASCL1, NEUROD1, and YAP1 is shown in Figure 1C-1F. Six out of 64 patients (9.4%) detected negative expression of all four molecules. Additionally, 18/64 (28.1%) patients expressed single molecules, and 5/64 (7.8%) patients co-expressed three molecules. Thirty-five out of 64 patients (54.7%) co-expressed two molecules, including 24 co-expressed ASCL1 and NEUROD1, 9 co-expressed ASCL1 and YAP1, and 2 co-expressed NEUROD1 and YAP1. Figure 1G-1J shows the representative IHC images for ASCL1 and NEUROD1 co-expression, ASCL1 and YAP1 co-expression, NEUROD1 and YAP1 co-expression, and ASCL1, NEUROD1 and YAP1 co-expression, respectively.
ASCL1/NEUROD1-defined groups and NE markers
Due to no POU2F3 positive cases being detected in our study, and since YAP1 was widely co-expressed with ASCL1/NEUROD1 (Figure 1F), we applied the molecular subtyping approach as reported previously (14). Thus, a subsequent analysis was carried out based on this.
The molecular subtyping was based on the relative dominant expression of ASCL1 (H-score of ASCL1-NEUROD1 >0) or NEUROD1 (H-score of NEUROD1-ASCL1 >0), negative expression (H-score =0) of both ASCL1 and NEUROD1 was classified as a double negative group (14). According to the approach, the distribution of groups was as follows: ASCL1 dominant group, N=41 (64.1%); NEUROD1 dominant group, N=15 (23.4%); and double negative group, N=8 (12.5%) (Figure 2A).
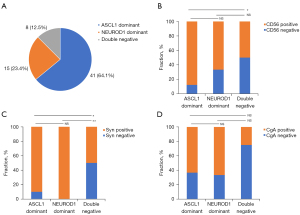
We performed IHC staining for three NE markers typically used in clinical practice: CD56, Syn, and CgA. The positive expression rates of CD56 in the ASCL1 dominant group were higher than those in the double negative group (87.8% vs. 50.0%, P=0.03, Figure 2B). Syn positive rates were also higher in ASCL1 dominant (90.2% vs. 50.0%, P=0.02) or NEUROD1 dominant (100.0% vs. 50.0%, P=0.008) groups versus those in the double negative group (Figure 2C). No significant difference in CgA positive rate was seen among different groups (P=0.12, Figure 2D). Furthermore, age, sex, smoking status, tumor stage, pathological classification, and chemotherapy regimens were balanced among different groups (Table S3).
Peripheral blood inflammation indicators and clinicopathological features
Baseline LMR, NLR, PLR, and SII values were available for all patients in the cohort. The optimal cutoff value was 3.3 for LMR, 2.9 for NLR, 95.4 for PLR, and 530.8 for SII, which was determined according to OS by X-tile software (34). Compared with patients with low LMR (≤3.3), patients with high LMR (>3.3) had lower CRP levels [mean ± standard deviation (SD), 8.1±14.6 vs. 25.4±40.3, P=0.004] and higher ALB levels (mean ± SD, 43.0±3.1 vs. 40.0±5.2, P=0.005). Patients with SII >530.8 had higher CRP level (mean ± SD, 18.2±32.1 vs. 5.4±8.8, P=0.02) than those with SII ≤530.8. Other clinicopathological parameters did not differ between groups stratified by LMR, NLR, PLR or SII (Table S4).
Figure 3A shows the representative IHC staining image for intra-tumoral and stromal TIL (marked by CD3, CD8, or CD20) and TIM (marked by CD68 or CD163). See Table S5 for detailed expression levels (mean ± SD and range). Compared to patients with low LMR (N=21), patients with high LMR (N=43) had significantly more intra-tumoral CD3+ (641±685 vs. 1,508±1,672 cells/mm2, P=0.004), CD8+ (484±618 vs. 1,096±1,342 cells/mm2, P=0.01), and CD68+ (398±153 vs. 667±373 cells/mm2, P<0.001) immune cell infiltration (Figure 3B, Table S6). The intra-tumoral and stromal TIL/TIM status were balanced between groups stratified by NLR, PLR or SII (Table S6). The LMR level was not differ among ASCL1 dominant, NEUROD1 dominant, and double negative groups (P=0.30), as well as NLR, PLR, SII level and intra-tumoral or stromal TIL/TIM status (Table S7).
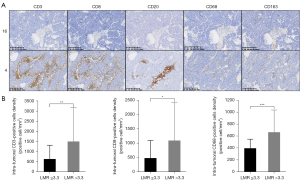
Prognostic indicators for DFS and OS
At the last follow-up date of January 30, 2023, the median follow-up time of the whole cohort was 37.4 months (range, 3.0–159.1 months). The median DFS and OS were 23.5 months (range, 0.6–125.5 months) and 25.6 months (range, 1.1–159.1 months), respectively. Kaplan-Meier survival curves showed that the median DFS (P=0.002, Figure 4A) and OS (P=0.001, Figure 4B) were longer in patients with high LMR than those with low LMR. No significant differences were observed in DFS (P=0.44, Figure 4C) and OS (P=0.54, Figure 4D) among ASCL1 dominant, NEUROD1 dominant, and double negative groups. No statistically substantial differences in DFS or OS were observed between groups stratified by NLR, PLR or SII (Figure S2).
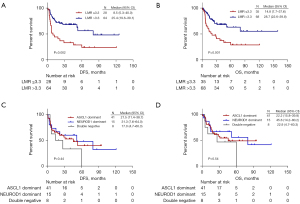
We performed the univariate and multivariate Cox regression analysis to investigate the independent prognostic indicators of DFS and OS in lung LCNEC. For DFS, high LMR (P=0.003) and early tumor stage (P=0.006) were associated with increased DFS in univariate analyses. From multivariate analyses, patients with high LMR (P=0.04) and early tumor stage (P=0.02) were independent predictors of favorable prognosis of DFS in lung LCNEC patients (Table 1). The univariate favorable prognostic factors for OS were high LMR (P=0.002) and early tumor stage (P=0.002). Furthermore, multivariate analyses indicated that high LMR (P=0.003) and low tumor stage (P=0.03) were independent predictors of favorable prognosis of OS in lung LCNEC patients (Table 2).
Table 1
Variables | Subtypes | Univariate analysis | Multivariate analysis | |||||
---|---|---|---|---|---|---|---|---|
HR | 95% CI | P value | HR | 95% CI | P value | |||
Age, years | ≤60 | 0.923 | 0.510–1.670 | 0.79 | 0.719 | 0.310–1.666 | 0.44 | |
>60 | 1 (Referent) | – | 1 (Referent) | – | ||||
Sex | Male | 2.315 | 0.715–7.501 | 0.16 | 1.458 | 0.302–7.035 | 0.64 | |
Female | 1 (Referent) | – | 1 (Referent) | – | ||||
Smoking status | Current/ever smokers | 1.017 | 0.509–2.031 | 0.96 | 0.910 | 0.304–2.727 | 0.87 | |
Never smokers | 1 (Referent) | – | 1 (Referent) | – | ||||
Tumor stage | I | 0.147 | 0.049–0.441 | 0.006* | 0.131 | 0.031–0.561 | 0.02* | |
II | 0.374 | 0.134–1.042 | 0.417 | 0.115–1.514 | ||||
III | 0.366 | 0.130–1.029 | 0.452 | 0.114–1.794 | ||||
IV | 1 (Referent) | – | 1 (Referent) | – | ||||
Molecular subtypes | ASCL1 dominant | 0.589 | 0.236–1.470 | 0.45 | 0.724 | 0.241–2.176 | 0.63 | |
NEUROD1 dominant | 0.522 | 0.178–1.530 | 0.551 | 0.161–1.887 | ||||
Double negative | 1 (Referent) | – | 1 (Referent) | – | ||||
LMR | >3.3 | 0.409 | 0.225–0.744 | 0.003* | 0.391 | 0.161–0.948 | 0.04* | |
≤3.3 | 1 (Referent) | – | 1 (Referent) | – | ||||
NLR | >2.9 | 1.029 | 0.545–1.944 | 0.93 | 0.376 | 0.137–1.032 | 0.06 | |
≤2.9 | 1 (Referent) | – | 1 (Referent) | – | ||||
PLR | >95.4 | 1.439 | 0.640–3.234 | 0.38 | 1.453 | 0.373–5.661 | 0.59 | |
≤95.4 | 1 (Referent) | – | 1 (Referent) | – | ||||
SII | >530.8 | 1.260 | 0.675–2.352 | 0.47 | 2.136 | 0.746–6.115 | 0.16 | |
≤530.8 | 1 (Referent) | – | 1 (Referent) | – |
*, statistically significance (P<0.05). DFS, disease-free survival; LCNEC, large cell neuroendocrine carcinoma; HR, hazard ration; CI, confidential interval; LMR, lymphocyte and monocyte ratio; NLR, neutrophil-to-lymphocyte ratio; PLR, platelet-to-lymphocyte ratio; SII, systemic immune-inflammation index.
Table 2
Variables | Subtypes | Univariate analysis | Multivariate analysis | |||||
---|---|---|---|---|---|---|---|---|
HR | 95% CI | P value | HR | 95% CI | P value | |||
Age, years | ≤60 | 0.904 | 0.496–1.646 | 0.74 | 0.704 | 0.282–1.754 | 0.45 | |
>60 | 1 (Referent) | – | 1 (Referent) | – | ||||
Sex | Male | 1.856 | 0.572–6.017 | 0.30 | 0.667 | 0.129–3.448 | 0.63 | |
Female | 1 (Referent) | – | 1 (Referent) | – | ||||
Smoking status | Current/ever smokers | 1.175 | 0.578–2.389 | 0.66 | 1.468 | 0.469–4.588 | 0.51 | |
Never smokers | 1 (Referent) | – | 1 (Referent) | – | ||||
Tumor stage | I | 0.164 | 0.064–0.421 | 0.002* | 0.122 | 0.027–0.554 | 0.03* | |
II | 0.368 | 0.156–0.870 | 0.439 | 0.121–1.593 | ||||
III | 0.318 | 0.132–0.765 | 0.467 | 0.118–1.852 | ||||
IV | 1 (Referent) | – | 1 (Referent) | – | ||||
Molecular subtypes | ASCL1 dominant | 0.634 | 0.235–1.711 | 0.55 | 0.760 | 0.227–2.540 | 0.66 | |
NEUROD1 dominant | 0.528 | 0.165–1.697 | 0.553 | 0.146–2.094 | ||||
Double negative | 1 (Referent) | – | 1 (Referent) | – | ||||
LMR | >3.3 | 0.386 | 0.212–0.703 | 0.002* | 0.201 | 0.071–0.574 | 0.003* | |
≤3.3 | 1 (Referent) | – | 1 (Referent) | – | ||||
NLR | >2.9 | 1.336 | 0.718–2.485 | 0.36 | 0.426 | 0.134–1.349 | 0.15 | |
≤2.9 | 1 (Referent) | – | 1 (Referent) | – | ||||
PLR | >95.4 | 2.070 | 0.813–5.271 | 0.13 | 1.465 | 0.334–6.420 | 0.61 | |
≤95.4 | 1 (Referent) | – | 1 (Referent) | – | ||||
SII | >530.8 | 1.519 | 0.779–2.960 | 0.19 | 1.208 | 0.400–3.645 | 0.74 | |
≤530.8 | 1 (Referent) | – | 1 (Referent) | – |
*, statistically significance (P<0.05). OS, overall survival; LCNEC, large cell neuroendocrine carcinoma; HR, hazard ration; CI, confidential interval; LMR, lymphocyte and monocyte ratio; NLR, neutrophil-to-lymphocyte ratio; PLR, platelet-to-lymphocyte ratio; SII, systemic immune-inflammation index.
Discussion
In this study, we explored molecular subtypes and immune microenvironment in lung LCNEC and assessed their correlation with clinical outcomes. Our study demonstrated the feasibility of defining molecular subtypes of lung LCNEC patients by IHC, and found ASCL1, NEUROD1, and YAP1 were co-expressed in different degrees, with no prognostic effect. Moreover, peripheral blood LMR was a strong independent predictor of outcomes for DFS and OS, and significantly correlated with tissue intra-tumoral CD3+, CD8+, and CD68+ immune cell infiltration.
Molecular subtypes defined by ASCL1, NEUROD1, POU2F3, and YAP1 were initially proposed for SCLC, called SCLC-A, SCLC-N, SCLC-P and SCLC-Y, respectively (11). In general, SCLC-A and SCLC-N demonstrated higher proportions, while SCLC-P and SCLC-Y showed lower proportions (16,35). Our study found that ASCL1 had the highest positive expression rate, followed by NEUROD1, and YAP1 was the lowest, consistent with the SCLC study (14). POU2F3+ tumor originated from a rare tuft cell lineage different from ASCL1+ and NEUROD1+. Although POU2F3 was detectable in lung LCNEC by RNA sequencing (20) and IHC (19,36) methods, its positive expression rate was low (about 10% to 15.9%). No POU2F3+ cases were detected in our study, which may be due to the sample size restriction.
ASCL1, NEUROD1, POU2F3, and YAP1 were disproportionately co-expressed in one sample in previous studies of SCLC (35,37) and lung LCNEC (19,20). A similar phenomenon was observed in our study, in which most lung LCNEC patients co-expressed ASCL1/NEUROD1/YAP1 to different degrees, suggesting inter- and intra-tumoral heterogeneities. Notably, preclinical studies demonstrated remarkable heterogeneity and plasticity within SCLC, with the capacity to subtypes switching: MYC drive the ASCL1+ to NEUROD1+ to YAP1+ phenotypic transition in vitro and in vivo, and KDM6A inactivation induced plasticity from ASCL1+ to NEUROD1+ state (35,38-41). A possible explanation for tumor heterogeneity of lung LCNEC is the time evolution process among ASCL1, NEUROD1, and YAP1. This dynamic subtype transformation may be the reason why there is no difference in prognosis among different subtypes observed in the present study.
NE differentiation is an essential feature of lung LCNEC (5). It is reported that the biological function of ASCL1 and NEUROD1 is to promote NE differentiation (11,42-45). Compared with patients in the double negative group, the ASCL1 dominant group had significantly higher CD56 positive expression rates. The NEUROD1 dominant group patients had significantly higher CD56 and Syn positive expression rates. This indirectly demonstrates the role of ASCL1 and NEUROD1 in promoting NE differentiation, which aligns with the observation in the SCLC study (14). Our findings validated the correlation between ASCL1/NEUROD1 expression and NE differentiation in lung LCNEC.
Our study was the first to explore the prognostic value of LMR in lung LCNEC and demonstrated that peripheral blood LMR was prognostic indicator for DFS and OS in lung LCNEC, and its prognostic value was independent of the tumor stage, suggesting that the blood LMR and tumor stage provide complementary prognostic information in lung LCNEC. This result was consistent with studies of lung cancer: high LMR level was significantly associated with improved OS and DFS (46,47).
We observed no prognostic effect of SII, NLR and PLR in our study. This is the first time to assess the prognostic value of SII in lung LCNEC. SII was reported to be a new prognostic systemic inflammation indicator, patients with high SII score had a worse prognosis in NSCLC (48,49). However, no correlation between SII and prognosis was found in lung LCNEC in our study. Previous two studies indicated that baseline high NLR or PLR was associated with poor prognosis in lung LCNEC patients (27-29), which is differ from our results. The differences may be related to different baseline characteristics of study population, small sample size and retrospective study design of these studies. Findings of the prognostic value of these inflammation indicators were needed to be interpreted with caution and validated in larger prospective cohorts.
In tumor tissue, high blood LMR was significantly associated with higher intra-tumoral CD3+ TIL, CD8+ TIL, and CD68+ TIM versus low LMR. CD3+ TIL contains all T cells infiltrated in tumor tissue, including cytotoxic T lymphocytes (CTLs), T helper (Th) cells, and Treg cells, which kill tumor cells through the direct killing effect of CTL and pose an indirect effect via cytokines secretion (50,51). Moreover, CD8+ cytotoxic T cells exert an antitumor effect by binding to MHC-I molecules on the surface of antigen-presenting cells (52). CD68 is a common marker of pan-macrophages in tumor tissue (53), which plays a dual role in antitumor immunity, tumor suppression, and promotion of tumor cell proliferation and invasion (54,55). Macrophages are classified into M1 and M2 macrophages, M1 macrophage can recruit an antitumor TIL response to prolong the survival of lung cancer patients (56,57). High CD3+ TIL, CD8+ TIL, and CD68+ TIM were associated with good prognosis in cancers (58-62), and high immune cell infiltration with anti-tumor effect in tumor tissue has been regarded as a hot tumor microenvironment (63,64). Thus, we speculate that the good prognosis of high LMR may be closely related to the hot tumor microenvironment as reflected by high CD3+, CD8+ TIL and CD68+ macrophages infiltration, which needs to be explored in further studies.
Our study has several limitations. First, this is a single-center retrospective study with a small sample size. However, given the low incidence rate of lung LCENC, the sample size is relatively large. Second, we explored the immune microenvironment of lung LCNEC using IHC which were not comprehensive enough. Thus, we are currently reaching out to conduct RNA sequencing for further investigation. Third, the result that peripheral blood LMR was an independent prognostic factor in lung LCNEC requires further validation in prospective studies with larger sample sizes.
Conclusions
Our study validated the molecular subtypes by IHC in lung LCNEC, and co-expression was found between different subtypes with no prognostic effect. High blood LMR was a favorable prognostic indicator for DFS and OS in lung LCNEC, which may be partly related to a hot TIME. Our findings may benefit clinical practice while prospective studies with larger sample size are warranted.
Acknowledgments
The authors thank AiMi Academic Services (www.aimieditor.com) for English language editing and review services.
Funding: None.
Footnote
Reporting Checklist: The authors have completed the STROBE reporting checklist. Available at https://tlcr.amegroups.com/article/view/10.21037/tlcr-24-292/rc
Data Sharing Statement: Available at https://tlcr.amegroups.com/article/view/10.21037/tlcr-24-292/dss
Peer Review File: Available at https://tlcr.amegroups.com/article/view/10.21037/tlcr-24-292/prf
Conflicts of Interest: All authors have completed the ICMJE uniform disclosure form (available at https://tlcr.amegroups.com/article/view/10.21037/tlcr-24-292/coif). The authors have no conflicts of interest to declare.
Ethical Statement: The authors are accountable for all aspects of the work in ensuring that questions related to the accuracy or integrity of any part of the work are appropriately investigated and resolved. The study was conducted in accordance with the Declaration of Helsinki (as revised in 2013). The study was approved by the Institutional Review Board of SYSUCC (ID: B2021-030-01), and written informed consent was obtained from each participant.
Open Access Statement: This is an Open Access article distributed in accordance with the Creative Commons Attribution-NonCommercial-NoDerivs 4.0 International License (CC BY-NC-ND 4.0), which permits the non-commercial replication and distribution of the article with the strict proviso that no changes or edits are made and the original work is properly cited (including links to both the formal publication through the relevant DOI and the license). See: https://creativecommons.org/licenses/by-nc-nd/4.0/.
References
- Nicholson AG, Tsao MS, Beasley MB, et al. The 2021 WHO Classification of Lung Tumors: Impact of Advances Since 2015. J Thorac Oncol 2022;17:362-87. [Crossref] [PubMed]
- Soldath P, Petersen RH. The Surgical Management of Lung Neuroendocrine Neoplasms. Cancers (Basel) 2023;15:1695. [Crossref] [PubMed]
- Kawasaki K, Rekhtman N, Quintanal-Villalonga Á, et al. Neuroendocrine neoplasms of the lung and gastrointestinal system: convergent biology and a path to better therapies. Nat Rev Clin Oncol 2023;20:16-32. [Crossref] [PubMed]
- WHO Classification of Tumours Editorial Board. Thoracic Tumours. 5th ed. Lyon (France): International Agency for Research on Cancer; 2021.
- Rekhtman N. Lung neuroendocrine neoplasms: recent progress and persistent challenges. Mod Pathol 2022;35:36-50. [Crossref] [PubMed]
- Raman V, Jawitz OK, Yang CJ, et al. Outcomes for Surgery in Large Cell Lung Neuroendocrine Cancer. J Thorac Oncol 2019;14:2143-51. [Crossref] [PubMed]
- Derks JL, Leblay N, Thunnissen E, et al. Molecular Subtypes of Pulmonary Large-cell Neuroendocrine Carcinoma Predict Chemotherapy Treatment Outcome. Clin Cancer Res 2018;24:33-42. [Crossref] [PubMed]
- Derks J, van Suylen RJ, Thunnissen E, et al. Why we should improve current practice of diagnosing and treating pulmonary large cell neuroendocrine carcinomas in patients with advanced disease. Eur Respir J 2017;50:1701658. [Crossref] [PubMed]
- George J, Walter V, Peifer M, et al. Integrative genomic profiling of large-cell neuroendocrine carcinomas reveals distinct subtypes of high-grade neuroendocrine lung tumors. Nat Commun 2018;9:1048. [Crossref] [PubMed]
- Tang M, Abbas HA, Negrao MV, et al. The histologic phenotype of lung cancers is associated with transcriptomic features rather than genomic characteristics. Nat Commun 2021;12:7081. [Crossref] [PubMed]
- Rudin CM, Poirier JT, Byers LA, et al. Molecular subtypes of small cell lung cancer: a synthesis of human and mouse model data. Nat Rev Cancer 2019;19:289-97. [Crossref] [PubMed]
- Qu S, Fetsch P, Thomas A, et al. Molecular Subtypes of Primary SCLC Tumors and Their Associations With Neuroendocrine and Therapeutic Markers. J Thorac Oncol 2022;17:141-53. [Crossref] [PubMed]
- Hwang S, Hong TH, Kim HK, et al. Whole-Section Landscape Analysis of Molecular Subtypes in Curatively Resected Small Cell Lung Cancer: Clinicopathologic Features and Prognostic Significance. Mod Pathol 2023;36:100184. [Crossref] [PubMed]
- Baine MK, Hsieh MS, Lai WV, et al. SCLC Subtypes Defined by ASCL1, NEUROD1, POU2F3, and YAP1: A Comprehensive Immunohistochemical and Histopathologic Characterization. J Thorac Oncol 2020;15:1823-35. [Crossref] [PubMed]
- Qi J, Zhang J, Liu N, et al. Prognostic Implications of Molecular Subtypes in Primary Small Cell Lung Cancer and Their Correlation With Cancer Immunity. Front Oncol 2022;12:779276. [Crossref] [PubMed]
- Megyesfalvi Z, Barany N, Lantos A, et al. Expression patterns and prognostic relevance of subtype-specific transcription factors in surgically resected small-cell lung cancer: an international multicenter study. J Pathol 2022;257:674-86. [Crossref] [PubMed]
- Chen P, Sun C, Wang H, et al. YAP1 expression is associated with survival and immunosuppression in small cell lung cancer. Cell Death Dis 2023;14:636. [Crossref] [PubMed]
- Park S, Hong TH, Hwang S, et al. Comprehensive analysis of transcription factor-based molecular subtypes and their correlation to clinical outcomes in small-cell lung cancer. EBioMedicine 2024;102:105062. [Crossref] [PubMed]
- Popper H, Brcic L, Eidenhammer S. Does subtyping of high-grade pulmonary neuroendocrine carcinomas have an impact on therapy selection? Transl Lung Cancer Res 2023;12:2412-26. [Crossref] [PubMed]
- Metovic J, La Salvia A, Rapa I, et al. Molecular Subtypes of Extra-pulmonary Neuroendocrine Carcinomas Identified by the Expression of Neuroendocrine Lineage-Specific Transcription Factors. Endocr Pathol 2022;33:388-99. [Crossref] [PubMed]
- Zhao H, Wu L, Yan G, et al. Inflammation and tumor progression: signaling pathways and targeted intervention. Signal Transduct Target Ther 2021;6:263. [Crossref] [PubMed]
- Rodriguez-Meira A, Norfo R, Wen S, et al. Single-cell multi-omics identifies chronic inflammation as a driver of TP53-mutant leukemic evolution. Nat Genet 2023;55:1531-41. [Crossref] [PubMed]
- Yang X, Zhao S, Wang S, et al. Systemic inflammation indicators and risk of incident arrhythmias in 478,524 individuals: evidence from the UK Biobank cohort. BMC Med 2023;21:76. [Crossref] [PubMed]
- Chen L, Xia S, Zuo Y, et al. Systemic immune inflammation index and peripheral blood carbon dioxide concentration at admission predict poor prognosis in patients with severe traumatic brain injury. Front Immunol 2022;13:1034916. [Crossref] [PubMed]
- Monberg TJ, Borch TH, Svane IM, et al. TIL Therapy: Facts and Hopes. Clin Cancer Res 2023;29:3275-83. [Crossref] [PubMed]
- Blériot C, Dunsmore G, Alonso-Curbelo D, et al. A temporal perspective for tumor-associated macrophage identities and functions. Cancer Cell 2024;42:747-58. [Crossref] [PubMed]
- Shirasawa M, Yoshida T, Horinouchi H, et al. Prognostic impact of peripheral blood neutrophil to lymphocyte ratio in advanced-stage pulmonary large cell neuroendocrine carcinoma and its association with the immune-related tumour microenvironment. Br J Cancer 2021;124:925-32. [Crossref] [PubMed]
- Shi M, Zhao W, Zhou F, et al. Neutrophil or platelet-to-lymphocyte ratios in blood are associated with poor prognosis of pulmonary large cell neuroendocrine carcinoma. Transl Lung Cancer Res 2020;9:45-54. [Crossref] [PubMed]
- Okui M, Yamamichi T, Asakawa A, et al. Prognostic significance of neutrophil-lymphocyte ratios in large cell neuroendocrine carcinoma. Gen Thorac Cardiovasc Surg 2017;65:633-9. [Crossref] [PubMed]
- He M, Chen ZF, Zhang L, et al. Associations of subcutaneous fat area and Systemic Immune-inflammation Index with survival in patients with advanced gastric cancer receiving dual PD-1 and HER2 blockade. J Immunother Cancer 2023;11:e007054. [Crossref] [PubMed]
- Rami-Porta R, Asamura H, Travis WD, et al. Lung cancer - major changes in the American Joint Committee on Cancer eighth edition cancer staging manual. CA Cancer J Clin 2017;67:138-55.
- Schwartz LH, Seymour L, Litière S, et al. RECIST 1.1 - Standardisation and disease-specific adaptations: Perspectives from the RECIST Working Group. Eur J Cancer 2016;62:138-45. [Crossref] [PubMed]
- Schwartz LH, Litière S, de Vries E, et al. RECIST 1.1-Update and clarification: From the RECIST committee. Eur J Cancer 2016;62:132-7. [Crossref] [PubMed]
- Camp RL, Dolled-Filhart M, Rimm DL. X-tile: a new bio-informatics tool for biomarker assessment and outcome-based cut-point optimization. Clin Cancer Res 2004;10:7252-9. [Crossref] [PubMed]
- Gay CM, Stewart CA, Park EM, et al. Patterns of transcription factor programs and immune pathway activation define four major subtypes of SCLC with distinct therapeutic vulnerabilities. Cancer Cell 2021;39:346-360.e7. [Crossref] [PubMed]
- Yamada Y, Belharazem-Vitacolonnna D, Bohnenberger H, et al. Pulmonary cancers across different histotypes share hybrid tuft cell/ionocyte-like molecular features and potentially druggable vulnerabilities. Cell Death Dis 2022;13:979. [Crossref] [PubMed]
- Wooten DJ, Groves SM, Tyson DR, et al. Systems-level network modeling of Small Cell Lung Cancer subtypes identifies master regulators and destabilizers. PLoS Comput Biol 2019;15:e1007343. [Crossref] [PubMed]
- Ireland AS, Micinski AM, Kastner DW, et al. MYC Drives Temporal Evolution of Small Cell Lung Cancer Subtypes by Reprogramming Neuroendocrine Fate. Cancer Cell 2020;38:60-78.e12. [Crossref] [PubMed]
- Wollenzien H, Afeworki Tecleab Y, Szczepaniak-Sloane R, et al. Single-Cell Evolutionary Analysis Reveals Drivers of Plasticity and Mediators of Chemoresistance in Small Cell Lung Cancer. Mol Cancer Res 2023;21:892-907. [Crossref] [PubMed]
- Duplaquet L, Li Y, Booker MA, et al. KDM6A epigenetically regulates subtype plasticity in small cell lung cancer. Nat Cell Biol 2023;25:1346-58. [Crossref] [PubMed]
- Pearsall SM, Williamson SC, Humphrey S, et al. Lineage Plasticity in SCLC Generates Non-Neuroendocrine Cells Primed for Vasculogenic Mimicry. J Thorac Oncol 2023;18:1362-85. [Crossref] [PubMed]
- Borges M, Linnoila RI, van de Velde HJ, et al. An achaete-scute homologue essential for neuroendocrine differentiation in the lung. Nature 1997;386:852-5. [Crossref] [PubMed]
- Zhang W, Girard L, Zhang YA, et al. Small cell lung cancer tumors and preclinical models display heterogeneity of neuroendocrine phenotypes. Transl Lung Cancer Res 2018;7:32-49. [Crossref] [PubMed]
- Ito T, Kudoh S, Fujino K, et al. Pulmonary Neuroendocrine Cells and Small Cell Lung Carcinoma: Immunohistochemical Study Focusing on Mechanisms of Neuroendocrine Differentiation. Acta Histochem Cytochem 2022;55:75-83. [Crossref] [PubMed]
- Lissa D, Takahashi N, Desai P, et al. Heterogeneity of neuroendocrine transcriptional states in metastatic small cell lung cancers and patient-derived models. Nat Commun 2022;13:2023. [Crossref] [PubMed]
- Lang C, Egger F, Alireza Hoda M, et al. Lymphocyte-to-monocyte ratio is an independent prognostic factor in surgically treated small cell lung cancer: An international multicenter analysis. Lung Cancer 2022;169:40-6. [Crossref] [PubMed]
- Park SY, Cho DG, Shim BY, et al. Relationship between Systemic Inflammatory Markers, GLUT1 Expression, and Maximum 18F-Fluorodeoxyglucose Uptake in Non-Small Cell Lung Carcinoma and Their Prognostic Significance. Diagnostics (Basel) 2023;13:1013. [Crossref] [PubMed]
- Fan R, Chen Y, Xu G, et al. Combined systemic immune-inflammatory index and prognostic nutritional index predict outcomes in advanced non-small cell lung cancer patients receiving platinum-doublet chemotherapy. Front Oncol 2023;13:996312. [Crossref] [PubMed]
- Chen WH, Shao JJ, Yang Y, et al. Prognostic significance of systemic immune inflammatory index in NSCLC: a meta-analysis. Lung Cancer Manag 2024;13:LMT67. [Crossref] [PubMed]
- Mellman I, Chen DS, Powles T, et al. The cancer-immunity cycle: Indication, genotype, and immunotype. Immunity 2023;56:2188-205. [Crossref] [PubMed]
- Falkowski L, Buddenkotte J, Datsi A. Epigenetics in T-cell driven inflammation and cancer. Semin Cell Dev Biol 2024;154:250-60. [Crossref] [PubMed]
- Giles JR, Globig AM, Kaech SM, et al. CD8(+) T cells in the cancer-immunity cycle. Immunity 2023;56:2231-53. [Crossref] [PubMed]
- Sylvestre M, Crane CA, Pun SH. Progress on Modulating Tumor-Associated Macrophages with Biomaterials. Adv Mater 2020;32:e1902007. [Crossref] [PubMed]
- Kloosterman DJ, Akkari L. Macrophages at the interface of the co-evolving cancer ecosystem. Cell 2023;186:1627-51. [Crossref] [PubMed]
- Cassetta L, Pollard JW. A timeline of tumour-associated macrophage biology. Nat Rev Cancer 2023;23:238-57. [Crossref] [PubMed]
- Garrido-Martin EM, Mellows TWP, Clarke J, et al. M1(hot) tumor-associated macrophages boost tissue-resident memory T cells infiltration and survival in human lung cancer. J Immunother Cancer 2020;8:e000778. [Crossref] [PubMed]
- Backman M, Strell C, Lindberg A, et al. Spatial immunophenotyping of the tumour microenvironment in non-small cell lung cancer. Eur J Cancer 2023;185:40-52. [Crossref] [PubMed]
- Viveiros N, Flores BC, Lobo J, et al. Detailed bladder cancer immunoprofiling reveals new clues for immunotherapeutic strategies. Clin Transl Immunology 2022;11:e1402. [Crossref] [PubMed]
- Yang F, Zeng Z, Li Y, et al. The prognostic value of a 4-factor neoimmunologic score system in non-small cell lung cancer. J Leukoc Biol 2022;112:1605-19. [Crossref] [PubMed]
- Noma T, Makino T, Ohshima K, et al. Immunoscore Signatures in Surgical Specimens and Tumor-Infiltrating Lymphocytes in Pretreatment Biopsy Predict Treatment Efficacy and Survival in Esophageal Cancer. Ann Surg 2023;277:e528-37. [Crossref] [PubMed]
- Yi B, Cheng Y, Chang R, et al. Prognostic significance of tumor-associated macrophages polarization markers in lung cancer: a pooled analysis of 5105 patients. Biosci Rep 2023;43:BSR20221659. [Crossref] [PubMed]
- Wattenberg MM, Colby S, Garrido-Laguna I, et al. Intratumoral Cell Neighborhoods Coordinate Outcomes in Pancreatic Ductal Adenocarcinoma. Gastroenterology 2024;166:1114-29. [Crossref] [PubMed]
- Zhang J, Huang D, Saw PE, et al. Turning cold tumors hot: from molecular mechanisms to clinical applications. Trends Immunol 2022;43:523-45. [Crossref] [PubMed]
- Galon J, Bruni D. Approaches to treat immune hot, altered and cold tumours with combination immunotherapies. Nat Rev Drug Discov 2019;18:197-218. [Crossref] [PubMed]