Establishment of a survival predictive model for patients with two synchronous multiple primary lung cancers: a multicenter cohort analysis
Highlight box
Key findings
• This study proposed a new scoring system for synchronous multiple primary lung cancer (SMPLC) patients to predict survival, which can provide more powerful prognostic implications than the Tumor Node Metastasis (TNM) categories.
What is known and what is new?
• Multiple ground-glass nodules (GG/L) and SMPLC are two distinct groups of simultaneous multiple primary lung cancers with very different survival prognoses and pathologic features.
• For multiple primary lung cancers in which the primary lesion is a solid nodule, the presence of a ground-glass component in the secondary lesion is an important risk factor for prognosis. The new prognostic scoring system in this study can better reflect the prognosis of multiple primary lung cancers than the existing classification system.
What is the implication, and what should change now?
• The new prognostic scoring system enables further risk stratification of multiple primary lung cancers based on TNM staging, which helps in the selection of surgical strategies for multiple primary lung cancers. For concurrent multiple primary lung cancers, prognosis should be evaluated by combining multiple risk factors in order to select a surgical strategy with high patient benefit.
Introduction
Among all the malignant tumors worldwide, lung cancer is the leading cause of cancer mortality. According to the global cancer statistics for 2022, lung cancer has the highest mortality rate among all sexes and poses a great threat to the life and health of patients (1). Recently, with the convenience of computed tomography (CT) examination, more multifocal pulmonary nodules have been detected (2,3). These nodules belong to a complex type of lung cancer, which is called synchronous multiple primary lung cancer (SMPLC). The SMPLC is broadly defined as the simultaneous discovery of at least two or more primary tumors in the ipsilateral or bilateral lungs. Due to the inconsistent inclusion criteria of studies involving SMPLC patients, there is significant heterogeneity in survival and recurrence rates across studies. Therefore, the use of the traditional Tumor Node Metastasis (TNM) staging system to predict the survival of patients with SMPLC may be inadequate or unreliable. With the advancement of clinical research and the continuous updating of diagnostic criteria, it is still an important issue to explore the factors affecting the prognosis of SMPLC.
According to 8th edition of the lung cancer staging criteria in 2016, SMPLC in our research is defined as two or more distinct masses with imaging characteristics of lung cancer. Multifocal ground-glass/lepidic (GG/L) nodules should be distinguished from SMPLC (4). Multifocal GG/L nodules are classified separately as a type of SMPLC. In recent years, several retrospective studies have shown that the postoperative survival rate of SMPLC patients varies greatly. A previous meta-analysis of 26 studies demonstrated that the 5-year overall survival (OS) rate for real SMPLC patients was 45%, distinctly different from the 5-year OS (93%) rate for patients with multifocal GG/L nodules (5). However, the included studies did not use uniform categorization, which would lead to inaccurate reclassification in meta-analysis (6). As reported in recent literature, multiple clinical characteristics are prognostic factors for SMPLC. In a summary of 52 clinical studies, age, sex, tumor size, lymph node (LN) status, smoking status and forced expiratory volume in 1 second (FEV1) were found to be significant prognostic factors with SMPLC patients after surgery (7,8). In contrast, recent studies by Huo et al. showed that age, sex, and smoking history did not significantly affect the prognosis of patients with SMPLC (9), a result corroborated by previous studies by Hattori et al. and Shimada et al. (10,11). Although the results of the meta-analysis were more comprehensive, they were susceptible to publication bias, and the included studies used various diagnostic criteria and tumor staging guidelines. Given the lack of strict identification of SMPLC and multifocal GG/L in most previous studies, the risk factors for the prognosis of SMPLC in different studies are necessarily different. Therefore, the combined nature of SMPLC complexity and data inequality produces a high risk of bias, which may lead to inaccurate forecast of postoperative survival rate of SMPLC patients. The International Association for the Study of Lung Cancer (IASLC) has proposed that patients with multifocal GG/L nodules have a 5-year OS rate of up to 90% (3). At the same time, these patients also have a low rate of recurrence and LN involvement. In contrast, patients with SMPLC generally have a worse prognosis. Considering that differences in tumour histological types add to the complexity of SMPLC, the IASLC recommends further classification of SMPLC. Consequently, a more effective and precise prognostic categorization model is required to individually predict long-term survival outcomes after surgery for SMPLC.
In this research, we summarized clinicopathological data from five centers and re-staged 831 patients with resected SMPLC. We aimed to investigate the prognostic factors of patients using multicenter data with unified diagnostic criteria and to establish an accurate nomogram model for resected SMPLC. Another multicentric cohort was used to independently validate the model. We hope to provide a new method to assess the prognosis of patients with SMPLC more accurately in clinical settings. We present this article in accordance with the TRIPOD reporting checklist (available at https://tlcr.amegroups.com/article/view/10.21037/tlcr-24-252/rc).
Methods
Patient cohort for developing and validating the prognosis model
This retrospective study collected the clinical data from a multi-institutional registry. It included 831 SMPLC patients who had surgery from January 2004 to January 2018 at five institutions. In this study, the criteria for diagnosis of SMPLC were based on the 8th edition lung cancer staging criteria of the IASLC: (I) primary lung cancer; (II) pathological report showing that the number of lung tumors was more than 2; (III) preoperative chest CT showing two different lung tumors; (IV) two tumors from the same patient that had different histological types or morphological characteristics according to comprehensive histologic assessment (CHA) (12); and (V) two tumors from the same patient that had same histological types and no LN metastases, SMPLC was judged on the basis of molecular analysis or CHA. The exclusion criteria included induction therapy, adjuvant therapies before surgery, other lung cancer diagnoses in the past, lesions showing a ground-glass opacity (GGO) component in all tumors, and inadequate pathological or radiological information available for review.
In total, 499 patients from the Cancer Hospital, Chinese Academy of Medical Sciences, and Beijing Chao-Yang Hospital, Capital Medical University, met the inclusion criteria, which served as the training set (Figure S1). A total of 332 patients from The Third Xiangya Hospital of Central South University, the First Affiliated Hospital of University of Science and Technology of China, and Beijing Liangxiang Hospital met the inclusion criteria, which served as the external validation set (Figure S2). The Institutional Review Board of the Cancer Hospital, Chinese Academy of Medical Sciences has approved this study (No. 22/366-3568). The other participating institutions/hospitals were informed and agreed with the study. Since this is a retrospective study, the requirement for informed consent was waived. The study was conducted in accordance with the Declaration of Helsinki (as revised in 2013).
Radiological and histological evaluation
In this study, radiographic assessments were performed by three radiologists (L.Q., H.X. and Y.T.) and five thoracic surgeons (B.Q., Y.J., W.F., L.Y. and S.A.). We reviewed the radiological features of preoperative CT scans for all patients in detail. According to the latest staging criteria, the primary lesions of SMPLC were all pure-solid tumors. According to whether the secondary tumors had a GGO component, we categorize patients into two subgroups. We evaluated the existence of a GGO component in the lung window, with window width setting from 1,000 to 2,000 HU; and window level setting from −500 to −700 HU. Radiological grouping was as follows: all pure-solid tumors (APS) were defined as all tumors showing radiologic pure-solid mass (Figure 1A), whereas non-APS was defined as a pure-solid tumor accompanied by tumors with additional GGO [consolidation-to-tumor ratio (CTR), 0.25 to <1] (Figure 1B).
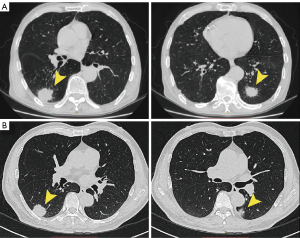
Experienced pathologists performed pathological assessments at individual centers. Postoperative pathological diagnosis was made according to the 2021 World Health Organization (WHO) classification of lung cancer. When the histopathological diagnosis of SMPLC was controversial, the consensus of a multidisciplinary team (MDT) was used to establish a definitive diagnosis and determine whether this study should include this case.
Surgical procedure
All the patients included in our cohort underwent thoracic surgery, which consisted of lobectomy, sublobar anatomic, non-anatomic sub-lobar and pneumonectomy. Among these, both sublobar anatomic and non-anatomic sub-lobar are sublobar resections. In the proposed study, we classified the patients into four subgroups according to the surgical approach of the primary lesion and the secondary lesions: all lobectomy (L&L, lobectomy was performed for both tumors), lobectomy with sublobar resection (L&S, lobectomy was performed for the primary tumor, and other tumors performed with sublobar resection), all sublobar resection (S&S, all tumors performed with sublobar resection), and pneumonectomy (PN, patients underwent pneumonectomy).
Statistical analysis
For the descriptive analysis of prognostic factors, data were reported as mean ± standard deviation (SD) for continuous factors and frequency statistics for discrete factors. OS calculated the time from tumor resection to death, regardless of the cause of death. For model development, we estimated the prognostic factors and predicted the survival rate of patients using a Cox proportional hazards model. Since most covariates are ordered categorical variables, a dummy transform technique should be used. For example, age has three levels, and thus, two dummy variables (S1,S2) were needed. When age was <60 years, then (S1,S2) = (0,0); when age is ≥70 years, then (S1,S2) = (1,0); and when age is between 60 and 70 years, (S1,S2) = (0,1). Other categorical variables were constructed in a similar manner.
Univariate variable analysis was first performed on all clinical data in the training set to analyze the influence of prognostic factors on the patients’ OS rate. Variables with P<0.05 were retained in the subsequent analysis. In the multivariate analysis, the Akaike Information Criterion (AIC) method was applied for further variable screening. Thereafter, screened variables were included in the nomogram model to construct a nomogram score for each patient. The Kaplan-Meier (K-M) method was used to plot the 5-year OS survival curves. Additionally, OS for patients with different TNM stages was also estimated with K-M approach and compared using log-rank tests. Finally, to evaluate the predictive performance of the nomogram model, the following metrics were used. The C-index, net reclassification improvement (NRI), and integrated discrimination improvement (IDI) metrics were used to evaluate the model performance for predicting outcomes. The R statistical language was adopted for all of the calculations (version 4.3.1). The statistically significant level (i.e., P values <0.05) was considered.
Results
Clinicopathological characteristics and prognostic factors
A total of 831 patients with confirmed SMPLC were included in this study. Table 1 presents the patients’ clinicopathological characteristics in both the validation and training sets. For the 499 patients in the training set, the 5-year OS rate was 68.8%, and 67.4% for the 332 patients in the validation set. In the subgroup analyses, SMPLC patients were divided into APS and non-APS subgroups according to imaging characteristics. Importantly, compared with the APS subgroup, the 5-year OS rate of the non-APS subgroup was significantly better (Figure S3) (training set: 80.9% vs. 53.1%, P<0.05; validation set: 83.5% vs. 44.8%, P<0.05). Therefore, the APS subgroup was considered to consist of more invasive tumors. Patients with both adenocarcinomas accounted for 75.2% and 76.5% of patients in the training and validation sets, respectively. In addition, 60 and 43 patients exhibited LN involvement in the training (12%) and validation sets (12.9%), respectively. Table 1 also presents the 5-year OS and 95% confidence intervals for all the patients.
Table 1
Variables | Training set (N=499) | Validation set (N=332) | |||||||
---|---|---|---|---|---|---|---|---|---|
No. of patients | % | 5-yr OS, % | 95% CI, % | No. of patients | % | 5-yr OS, % | 95% CI, % | ||
Gender | |||||||||
Male | 234 | 46.9 | 55.0 | 48.3 to 62.5 | 143 | 43.1 | 58.0 | 49.2 to 68.3 | |
Female | 265 | 53.1 | 81.8 | 76.5 to 87.5 | 189 | 56.9 | 74.9 | 68.1 to 82.4 | |
Age (years) | |||||||||
<60 | 164 | 32.9 | 79.2 | 72.3 to 86.8 | 110 | 33.1 | 78.2 | 69.7 to 87.9 | |
60–69 | 181 | 36.3 | 73.0 | 66.2 to 80.5 | 120 | 36.1 | 75.1 | 66.7 to 84.5 | |
≥70 | 154 | 30.9 | 54.5 | 46.4 to 64.1 | 102 | 30.7 | 48.5 | 38.5 to 61.0 | |
BMI type | |||||||||
Underweight | 15 | 3.0 | 76.2 | 55.6 to 100.0 | 3 | 0.9 | |||
Normal | 197 | 39.5 | 74.0 | 67.2 to 81.5 | 122 | 36.7 | 70.6 | 61.9 to 80.4 | |
Overweight | 164 | 32.9 | 63.6 | 55.5 to 72.9 | 138 | 41.6 | 70.2 | 61.9 to 79.6 | |
Obesity | 123 | 24.6 | 66.0 | 57.5 to 75.6 | 69 | 20.8 | 58.0 | 45.8 to 73.6 | |
Lung disease | |||||||||
No | 366 | 73.3 | 73.5 | 68.4 to 79.1 | 246 | 74.1 | 71.3 | 64.8 to 78.5 | |
Yes | 133 | 26.7 | 57.5 | 49.3 to 67.0 | 86 | 25.9 | 58.8 | 48.8 to 70.9 | |
Smoke | |||||||||
Never | 233 | 46.7 | 82.2 | 76.2 to 88.7 | 163 | 49.1 | 78.2 | 70.8 to 86.4 | |
Ever | 266 | 53.3 | 58.1 | 52.0 to 64.8 | 169 | 50.9 | 58.3 | 50.5 to 67.2 | |
Family history of tumor | |||||||||
No | 284 | 56.9 | 69.0 | 63.1 to 75.4 | 206 | 62.0 | 70.7 | 63.8 to 78.3 | |
Yes | 215 | 43.1 | 68.5 | 61.7 to 76.0 | 126 | 38.0 | 61.7 | 52.4 to 72.7 | |
Surgical approach | |||||||||
L&L | 204 | 40.9 | 77.3 | 71.2 to 84.0 | 144 | 43.4 | 74.1 | 66.5 to 82.6 | |
S&S | 50 | 10.0 | 56.6 | 42.8 to 74.8 | 34 | 10.2 | 49.0 | 31.6 to 76.1 | |
L&S | 226 | 45.3 | 64.1 | 57.1 to 72.0 | 144 | 43.4 | 67.6 | 59.1 to 77.3 | |
PN | 19 | 3.8 | 59.2 | 39.3 to 89.2 | 10 | 3.0 | 22.5 | 4.7 to 100.0 | |
Distribution of tumors | |||||||||
Same lobe | 89 | 17.8 | 55.2 | 41.6 to 73.2 | 53 | 16.0 | 71.2 | 57.9 to 87.5 | |
Ipsilateral different lobe | 208 | 41.7 | 66.4 | 59.3 to 74.3 | 148 | 44.6 | 60.7 | 51.8 to 71.1 | |
Contralateral lobe | 202 | 40.5 | 73.9 | 67.7 to 80.7 | 131 | 39.5 | 72.9 | 64.7 to 82.2 | |
Number of tumors | |||||||||
2 | 325 | 65.1 | 67.1 | 61.7 to 72.9 | 207 | 62.3 | 65.8 | 58.8 to 73.7 | |
3 | 92 | 18.4 | 64.8 | 54.4 to 77.3 | 70 | 21.1 | 71.7 | 60.4 to 85.2 | |
>3 | 82 | 16.4 | 77.7 | 64.9 to 93.1 | 55 | 16.6 | 60.5 | 42.2 to 86.7 | |
Pathological type | |||||||||
SC + SC | 44 | 8.8 | 54.2 | 40.4 to 72.7 | 23 | 6.9 | 43.9 | 25.1 to 76.7 | |
ADC + ADC | 375 | 75.2 | 72.1 | 66.9 to 77.6 | 254 | 76.5 | 75.7 | 69.9 to 81.9 | |
SC + ADC | 53 | 10.6 | 58.8 | 46.2 to 74.7 | 40 | 12.0 | 35.5 | 22.1 to 57.0 | |
SC + others† | 5 | 1.0 | NA§ | 2 | 0.6 | NA | |||
ADC + others | 22 | 4.4 | 76.4 | 60.2 to 96.8 | 13 | 3.9 | 41.0 | 9.98 to 100.0 | |
Radiologic grouping | |||||||||
APS | 209 | 41.9 | 53.1 | 46.2 to 61.0 | 131 | 39.5 | 44.8 | 36.0 to 55.8 | |
Non-APS | 290 | 58.1 | 80.9 | 75.6 to 86.6 | 201 | 60.5 | 83.5 | 77.8 to 89.7 | |
Pathology T stage‡ (primary tumor) | |||||||||
T1a + T1b | 223 | 44.7 | 82.7 | 76.9 to 88.9 | 155 | 46.7 | 77.3 | 69.8 to 85.6 | |
T1c | 117 | 23.4 | 69.4 | 60.4 to 79.6 | 72 | 21.7 | 60.3 | 47.5 to 76.7 | |
T2a | 125 | 25.1 | 51.6 | 42.9 to 62.1 | 80 | 24.1 | 56.1 | 45.3 to 69.5 | |
T2b | 16 | 3.2 | 46.3 | 26.2 to 81.9 | 13 | 3.9 | 61.5 | 40.0 to 94.6 | |
≥ T3 | 18 | 3.6 | 24.1 | 8.26 to 70.5 | 12 | 3.6 | 48.9 | 20.4 to 100.0 | |
Pathology T stage (secondary tumor) | |||||||||
T1a | 312 | 62.5 | 74.1 | 68.5 to 80.0 | 217 | 65.4 | 66.9 | 59.7 to 75.1 | |
T1b | 162 | 32.5 | 60.6 | 53.0 to 69.4 | 97 | 29.2 | 67.0 | 57.3 to 78.4 | |
T1c | 19 | 3.8 | 56.5 | 30.0 to 100.0 | 11 | 3.3 | NA | ||
T2a | 6 | 1.2 | NA | 7 | 2.1 | NA | |||
T2b | 0 | NA | NA | 0 | 0.0 | NA | |||
≥ T3 | 0 | NA | NA | 0 | 0.0 | NA | |||
Pathologic N stage | |||||||||
N0 | 439 | 88.0 | 71.1 | 66.3 to 76.1 | 289 | 87.0 | 70.0 | 64.1 to 76.5 | |
N1 | 41 | 8.2 | 54.6 | 39.2 to 76.0 | 34 | 10.2 | 48.0 | 30.6 to 75.4 | |
N2 | 19 | 3.8 | 45.1 | 26.7 to 76.1 | 9 | 2.7 | NA | ||
No. of cleaned LN stations¶ | |||||||||
<3 | 94 | 18.8 | 43.5 | 32.4 to 58.4 | 71 | 21.4 | 51.2 | 39.1 to 67.0 | |
3–5 | 212 | 42.5 | 64.9 | 57.4 to 73.4 | 162 | 48.8 | 63.3 | 54.2 to 73.9 | |
≥6 | 193 | 38.7 | 82.7 | 77.1 to 88.7 | 99 | 29.8 | 83.9 | 76.2 to 92.3 |
†, other tumors include small cell lung cancer, large cell carcinoma and carcinoid; ‡, each tumor was retrospectively re-staged according to the eighth edition of the TNM staging system; §, subgroups with fewer than 15 patients were omitted from statistical analysis; ¶, if patients underwent two separate thoracic surgeries, the number of cleaned LN stations was calculated as the sum of the number of the cleaned LN stations in two operations. OS, overall survival; CI, confidence interval; yr, year; BMI, body mass index; L&L, lobectomy was performed for both tumors; L&S, lobectomy was performed for the primary tumor and sublobar resection were performed for other tumors; S&S, sublobar resection were performed for all tumors; PN, pneumonectomy; SC, squamous cell carcinoma; ADC, adenocarcinoma; APS, all pure-solid tumors; non-APS, pure-solid tumor accompanied with tumors with additional GGO (CTR, 0.25 to <1); LN, lymph node; NA, not available; GGO, ground-glass opacity; CTR, consolidation-to-tumor ratio.
Table 2 presents the results of the multivariate analysis and AIC screening. Variables including age, smoking history, surgical approach, radiological grouping, pathological T stage (primary tumor), pathologic N stage, and the number of cleaned LN stations were retained. They were further treated as the independent prognostic factors for predicting the OS of patients with resected SMPLC. The hazard ratios and 95% confidence intervals are shown in Table 2.
Table 2
Variables | Univariable analysis | Multivariable analysis | AIC selected factors for building the model | ||||||
---|---|---|---|---|---|---|---|---|---|
P value | Hazard ratio | 95% CI | P value | Hazard ratio | 95% CI | P value | |||
Gender | <0.001 | ||||||||
Male | Reference | – | – | – | |||||
Female | 0.95 | 0.64–1.43 | 0.81 | – | – | – | |||
Age (years) | <0.001 | ||||||||
<60 | Reference | Reference | |||||||
60–69 | 1.28 | 0.81–2.03 | 0.29 | 1.30 | 0.82–2.06 | 0.26 | |||
≥70 | 2.52 | 1.64–3.89 | <0.001 | 2.47 | 1.61–3.79 | <0.001 | |||
Lung disease | <0.001 | ||||||||
No | Reference | – | – | – | |||||
Yes | 1.18 | 0.85–1.64 | 0.33 | – | – | – | |||
Smoke | <0.001 | ||||||||
Never | Reference | Reference | |||||||
Ever | 1.73 | 1.11–2.71 | 0.02 | 1.75 | 1.20–2.56 | <0.001 | |||
Surgical approach | 0.03 | ||||||||
L&L | Reference | Reference | |||||||
S&S | 1.87 | 1.08–3.23 | 0.02 | 1.93 | 1.14–3.29 | 0.01 | |||
L&S | 1.46 | 1.03–2.07 | 0.03 | 1.51 | 1.07–2.12 | 0.02 | |||
PN | 1.72 | 0.80–3.73 | 0.17 | 1.86 | 0.87–3.94 | 0.11 | |||
Number of tumors | 0.01 | ||||||||
2 | Reference | – | – | – | |||||
3 | 1.09 | 0.72–1.65 | 0.68 | – | – | – | |||
>3 | 0.56 | 0.30–1.04 | 0.07 | – | – | – | |||
Pathological type | <0.001 | ||||||||
SC + SC | Reference | – | – | – | |||||
ADC + ADC | 1.17 | 0.70–1.96 | 0.55 | – | – | – | |||
SC + ADC | 0.96 | 0.52–1.77 | 0.89 | – | – | – | |||
SC + others‡ | 0.99 | 0.27–3.65 | 0.98 | – | – | – | |||
ADC + others | 1.02 | 0.44–2.37 | 0.97 | – | – | – | |||
Radiologic grouping | <0.001 | ||||||||
APS | Reference | Reference | |||||||
Non-APS | 0.42 | 0.29–0.60 | <0.001 | 0.43 | 0.30–0.61 | <0.001 | |||
Pathology T stage§ (primary tumor) | <0.001 | ||||||||
T1a + T1b | Reference | Reference | |||||||
T1c | 1.39 | 0.89–2.19 | 0.15 | 1.37 | 0.88–2.14 | 0.17 | |||
T2a | 2.56 | 1.73–3.78 | <0.001 | 2.68 | 1.82–3.93 | <0.001 | |||
T2b | 3.34 | 1.53–7.29 | <0.001 | 3.04 | 1.43–6.44 | <0.001 | |||
≥ T3 | 4.45 | 2.29–8.67 | <0.001 | 4.15 | 2.19–7.89 | <0.001 | |||
Pathology T stage (secondary tumor) | <0.001 | ||||||||
T1a | Reference | – | – | – | |||||
T1b | 1.17 | 0.84–1.62 | 0.36 | – | – | – | |||
T1c | 0.53 | 0.21–1.33 | 0.17 | – | – | – | |||
T2a | 2.37 | 0.82–6.81 | 0.11 | – | – | – | |||
T2b | NA | – | – | – | |||||
≥ T3 | NA | – | – | – | |||||
No. of cleaned LN stations¶ | <0.001 | ||||||||
<3 | Reference | Reference | |||||||
3–5 | 0.49 | 0.31–0.79 | <0.001 | 0.43 | 0.28–0.64 | <0.001 | |||
≥6 | 0.25 | 0.15–0.41 | <0.001 | 0.23 | 0.15–0.35 | <0.001 | |||
Pathologic N stage | <0.001 | ||||||||
N0 | Reference | Reference | |||||||
N1 | 1.65 | 0.93–2.92 | 0.09 | 1.54 | 0.28–0.64 | <0.001 | |||
N2 | 3.20 | 1.59–6.44 | <0.001 | 3.27 | 0.15–0.35 | <0.001 |
‡, other tumors include small cell lung cancer, large cell carcinoma and carcinoid; §, each tumor was retrospectively re-staged according to the eighth edition of the TNM staging system; ¶, if patients underwent two separate thoracic surgeries, the number of cleaned LN stations was calculated as the sum of the number of the cleaned LN stations in two operations. AIC, Akaike Information Criterion; CI, confidence interval; L&L, lobectomy was performed for both tumors; L&S, lobectomy was performed for the primary tumor and sublobar resection were performed for other tumors; S&S, sublobar resection were performed for all tumors; PN, pneumonectomy; SC, squamous cell carcinoma; ADC, adenocarcinoma; APS, all pure-solid tumors; non-APS, pure-solid tumor accompanied with tumors with additional GGO (CTR, 0.25 to <1); LN, lymph node; GGO, ground-glass opacity; CTR, consolidation-to-tumor ratio.
Establishment, calibration, and validation of the nomogram
We developed a nomogram model to predict the OS of patients with resected SMPLC. Seven variables were included in the final model. The number of cleaned LN stations and pathological T stage (primary tumor) had the greatest impact on the prognosis, followed by age and radiological grouping (Figure 2A). Compared with model based on the highest tumor stage, the C-index value of the nomogram model was significantly higher (nomogram vs. TNM: internal validation 0.827 vs. 0.688, P<0.01; external validation 0.784 vs. 0.602, P<0.01). Calibration curves showed that the prediction of survival rates for 1-, 3-, and 5-year were consistent with the actual observations of the training and validation sets (Figure 2B,2C).
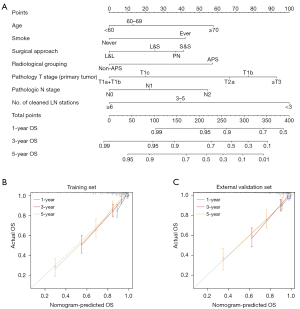
At the same time, the NRI index of the nomogram model was 0.33 and the IDI index was 0.21. These results indicate that compared with TNM staging system, the model improves the prediction ability of patients’ prognosis.
Nomogram model performance
According to the cutoff value of the total score of patients in the training group, we categorized the patients into three different groups: low-risk, medium-risk, and high-risk (total score: 0–97, 98–204, >204) (Figure 3A). We also developed a user-friendly access website for SMPLC survival probability calculation using the proposed model (https://survivalprediction.shinyapps.io/rssp/). A snapshot of the online calculator is shown in Figure 3B.
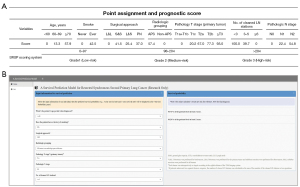
For each risk subgroups based on the SMPLC resection survival prediction scoring system [SMPLC resection prediction (SRSP) scoring system], the K-M survival curves in each TN category can be distinguished significantly (Figure S4). The patients in the validation set showed the same trend. According to the highest tumor TN stage only, the K-M survival curves showed poor discrimination between the groups in the validation set (Figure 4A). However, stratification into different risk subgroups according to the SRSP scoring system allowed for significant distinction between the K-M survival curves in the external validation set (Figure 4B). Even in each TN category (highest tumor stage), the difference in survival curves between the three risk subgroups was highly significant (Figure 4C-4H). Conversely, in each risk subgroup according to the SRSP scoring system, no significant differences were found in survival between different TN categories (Figure 4I-4K).

Discussion
In this research, we used a large retrospective multicenter cohort to construct a clinical tool for studying the OS trend in patients with resected SMPLC. The new risk stratification method based on our model can provide more powerful prognostic implications than the TNM categories. A user-friendly interface has also been provided to facilitate the survival prediction of SMPLC and further help thoracic surgeons in decision-making.
After rigorous case screening in this study, we found the pooled 5-year OS rate of SMPLC patients to be 68.7%, which is higher than that of the latest meta-analysis by Nie et al. (5) (45%). There are several possible reasons for this disparity. Aside from treatment variation and differences in inclusion criteria, the most likely reason may be selection bias from different studies. Ye et al. reported that the clinicopathological features of lung adenocarcinoma with partial solid nodules were significantly different from those of pure solid lung adenocarcinoma (13). Inspired by their study, we classified the imaging features of patients with SMPLC into two subgroups: APS and non-APS (see the Methods section and Figure 1). Survival analysis revealed a significant difference in OS between the two subgroups (Figure S3). Interestingly, the 5-year OS of SMPLC reported by most European-American researchers seems to be similar to the 5-year OS rate of our APS subgroup (14,15), whereas the 5-year OS rate of SMPLC reported by some Asian scholars is similar to the 5-year OS rate of the non-APS subgroup in our study (16-20). The discrepancy between these results can be explained by the GGO component in the secondary lung tumors. It is well known that subsolid nodules are considerably more common than solid nodules in Asian populations (21). Based on the obvious differences in survival patterns, our MDT suggests that SMPLC should be further subdivided to refine TNM classification. The presentation of secondary lung tumors may serve as an important reference for defining heterogeneous groups of patients with SMPLC.
Considering that the outcome of SMPLC patients with APS type is worse, the distinction between true SMPLC and intrapulmonary metastasis (IPM) is more important in these patients. Previously, the 5-year survival rate of IPM was found to be approximately 34% in the same lobe and only 11% in a different lobe (22). The SMPLC patients presenting as APS type in our study showed a significantly better prognosis than patients with IPM, despite the radiologic solid appearance. This indicates that patients with APS require more comprehensive and in-depth analyses than patients with the non-APS type before and after surgery. Moreover, despite the significant association of the GGO component in secondary lung tumors with SMPLC survival, the pathologic T stage of secondary lung tumors was not significant in the multivariate analysis. Even in the APS subgroup, the pathological T staging of the secondary lung tumor was not a significant prognostic factor for OS (Table S1). This important finding should be paid attention to because it suggests that primary lung tumors of SMPLC should be treated radically and aggressively.
One of the most important issues regarding SMPLC is selection of the optimal surgical approach. Current evidence regarding the extent of resection in SMPLC is limited and controversial. A recent meta-analysis suggested that anatomic lobectomy may not be applied because sublobar resection for all lesions have similar prognosis (23). However, most previous studies did not consider potential multifocal GG/L which thus heavily influenced the results of the analysis. In 2020, Hattori et al. demonstrated that for solid dominant (CTR, 0.5 to ≤1) tumors, it is necessary to implement lobectomy with complete mediastinal LN dissection. In addition, our results support lobectomy as the optimal surgery for primary lung tumors whenever possible. Another question was about the optimal strategy for LN dissection in patients with SMPLC. The assessment of LN in patients with SMPLC has rarely been alluded to in published work. Our results revealed that the number of examined LN stations was significantly associated with OS. In 2022, Jeon et al. demonstrated that the complete LN assessment had an impact on the survival rate (24). The relationship between more LN assessments and better survival was also supported by various relevant studies (25,26). Although these studies were based on solitary lung cancer, the results suggest an important role for complete LN sampling in precise staging. This again emphasizes the importance of radical lobectomy for primary lung tumors in patients, and further highlights the importance of operative evaluation for nodal staging. Since more patients with pure solid lesions were included in this study, there may be some bias in the selection of surgical methods. Although the results of our paper favor lobectomy of the primary tumor in patients with SMPLC, the appropriate surgical approach should be selected after discussion of the patient’s nodule using MDT in the clinic.
Based on the above-mentioned identified risk factors, we established a nomogram with good predictability in the survival prediction of patients with resected SMPLC. In addition, we developed a SRSP scoring system, which could separate patients with distinct survival outcomes (Figure 4). Through hierarchical comparison with TNM staging based on primary lung tumors, we found that our SRSP scoring system could more accurately predict the postoperative survival of patients. Currently, as far as we know, no reliable model exists for predicting the survival of patients with resected SMPLC and differentiating their prognosis. Further, we developed an easy-to-use online calculator based on our data and nomogram model. In addition, our SRSP scoring system can serve as a significant supplement to the existing staging systems. This system can not only help clinicians select patients who remain controversial in additional therapy, but also provide a reference for patient stratification when designing clinical studies.
This study still needs the following improvements in the future. First, as this was a retrospective study confined to the Chinese population, future studies should test whether the model is generalizable to more ethnically diverse populations. Second, since the retrospective nature of the data, some important molecular factors (e.g., EGFR mutations) were not taken into account. Third, as this was a retrospective study and not all samples had genomic and mutational data, we cannot completely ensure that all IPMs were excluded. This may also have biased the survival of patients with SMPLC, although the proportion is likely to be small. Nevertheless, this study was based on the current maximum sample size of carefully diagnosed SMPLC, providing more complete data for future exploration of prognostic factors and prediction of survival in patients with SMPLC.
Conclusions
In summary, we developed and validated a survival predictive nomogram model for patients with resected SMPLC. Based on this new model, we proposed an SRSP scoring system for risk-stratifying patients with heterogeneous SMPLC. Our results can provide more accurate prognostic information and assist in stratifying patients for further interventions. In addition, the risk factors and models have the potential to be used for stratification of patients with SMPLC in future trials. We hope that these data will help to better inform clinical considerations surrounding risk stratification.
Acknowledgments
Funding: This work was supported by
Footnote
Reporting Checklist: The authors have completed the TRIPOD reporting checklist. Available at https://tlcr.amegroups.com/article/view/10.21037/tlcr-24-252/rc
Data Sharing Statement: Available at https://tlcr.amegroups.com/article/view/10.21037/tlcr-24-252/dss
Peer Review File: Available at https://tlcr.amegroups.com/article/view/10.21037/tlcr-24-252/prf
Conflicts of Interest: All authors have completed the ICMJE uniform disclosure form (available at https://tlcr.amegroups.com/article/view/10.21037/tlcr-24-252/coif). The authors have no conflicts of interest to declare.
Ethical Statement: The authors are accountable for all aspects of the work in ensuring that questions related to the accuracy or integrity of any part of the work are appropriately investigated and resolved. The Institutional Review Board of the Cancer Hospital, Chinese Academy of Medical Sciences has approved this study (No. 22/366-3568). The other participating institutions/hospitals were informed and agreed with the study. Since this is a retrospective study, the requirement for informed consent was waived. The study was conducted in accordance with the Declaration of Helsinki (as revised in 2013).
Open Access Statement: This is an Open Access article distributed in accordance with the Creative Commons Attribution-NonCommercial-NoDerivs 4.0 International License (CC BY-NC-ND 4.0), which permits the non-commercial replication and distribution of the article with the strict proviso that no changes or edits are made and the original work is properly cited (including links to both the formal publication through the relevant DOI and the license). See: https://creativecommons.org/licenses/by-nc-nd/4.0/.
References
- Siegel RL, Miller KD, Fuchs HE, et al. Cancer statistics, 2022. CA Cancer J Clin 2022;72:7-33. [Crossref] [PubMed]
- Li J, Yang X, Xia T, et al. Stage I synchronous multiple primary non-small cell lung cancer: CT findings and the effect of TNM staging with the 7th and 8th editions on prognosis. J Thorac Dis 2017;9:5335-44.
- Detterbeck FC, Marom EM, Arenberg DA, et al. The IASLC Lung Cancer Staging Project: Background Data and Proposals for the Application of TNM Staging Rules to Lung Cancer Presenting as Multiple Nodules with Ground Glass or Lepidic Features or a Pneumonic Type of Involvement in the Forthcoming Eighth Edition of the TNM Classification. J Thorac Oncol 2016;11:666-80.
- Detterbeck FC, Bolejack V, Arenberg DA, et al. The IASLC Lung Cancer Staging Project: Background Data and Proposals for the Classification of Lung Cancer with Separate Tumor Nodules in the Forthcoming Eighth Edition of the TNM Classification for Lung Cancer. J Thorac Oncol 2016;11:681-92.
- Nie Y, Wang X, Yang F, et al. Surgical Prognosis of Synchronous Multiple Primary Lung Cancer: Systematic Review and Meta-Analysis. Clin Lung Cancer 2021;22:341-350.e3. [Crossref] [PubMed]
- Samadzadeh Tabrizi N, Gallant B, Harris E, et al. Contemporary Incidence of Synchronous Multiple Primary Lung Cancers and Survival in the Era of Lung Cancer Screening. Innovations (Phila) 2024;19:23-9. [Crossref] [PubMed]
- Tie H, Luo J, Shi R, et al. Characteristics and prognosis of synchronous multiple primary lung cancer after surgical treatment: A systematic review and meta-analysis of current evidence. Cancer Med 2021;10:507-20. [Crossref] [PubMed]
- Ma H, Li S, Zhu Y, et al. A Novel Prognostic Score Based on Multiple Quantitative Parameters of Chest CT for Patients with Synchronous Multiple Primary Lung Cancer: Is Solid Component Size a Better Prognostic Indicator? Ann Surg Oncol 2023;30:3769-78. [Crossref] [PubMed]
- Huo JW, Luo TY, He XQ, et al. Radiological classification, gene-mutation status, and surgical prognosis of synchronous multiple primary lung cancer. Eur Radiol 2022;32:4264-74. [Crossref] [PubMed]
- Hattori A, Takamochi K, Oh S, et al. Prognostic Classification of Multiple Primary Lung Cancers Based on a Ground-Glass Opacity Component. Ann Thorac Surg 2020;109:420-7. [Crossref] [PubMed]
- Shimada Y, Saji H, Otani K, et al. Survival of a surgical series of lung cancer patients with synchronous multiple ground-glass opacities, and the management of their residual lesions. Lung Cancer 2015;88:174-80. [Crossref] [PubMed]
- Girard N, Deshpande C, Lau C, et al. Comprehensive histologic assessment helps to differentiate multiple lung primary nonsmall cell carcinomas from metastases. Am J Surg Pathol 2009;33:1752-64. [Crossref] [PubMed]
- Ye T, Deng L, Wang S, et al. Lung Adenocarcinomas Manifesting as Radiological Part-Solid Nodules Define a Special Clinical Subtype. J Thorac Oncol 2019;14:617-27. [Crossref] [PubMed]
- Finley DJ, Yoshizawa A, Travis W, et al. Predictors of outcomes after surgical treatment of synchronous primary lung cancers. J Thorac Oncol 2010;5:197-205. [Crossref] [PubMed]
- Fabian T, Bryant AS, Mouhlas AL, et al. Survival after resection of synchronous non-small cell lung cancer. J Thorac Cardiovasc Surg 2011;142:547-53. [Crossref] [PubMed]
- Ishikawa Y, Nakayama H, Ito H, et al. Surgical treatment for synchronous primary lung adenocarcinomas. Ann Thorac Surg 2014;98:1983-8. [Crossref] [PubMed]
- Yu YC, Hsu PK, Yeh YC, et al. Surgical results of synchronous multiple primary lung cancers: similar to the stage-matched solitary primary lung cancers? Ann Thorac Surg 2013;96:1966-74. [Crossref] [PubMed]
- Cheng H, Lei BF, Peng PJ, et al. Histologic lung cancer subtype differentiates synchronous multiple primary lung adenocarcinomas from intrapulmonary metastases. J Surg Res 2017;211:215-22. [Crossref] [PubMed]
- Chen K, Chen W, Cai J, et al. Favorable prognosis and high discrepancy of genetic features in surgical patients with multiple primary lung cancers. J Thorac Cardiovasc Surg 2018;155:371-379.e1. [Crossref] [PubMed]
- Zhang Z, Gao S, Mao Y, et al. Surgical Outcomes of Synchronous Multiple Primary Non-Small Cell Lung Cancers. Sci Rep 2016;6:23252. [Crossref] [PubMed]
- Oudkerk M, Liu S, Heuvelmans MA, et al. Lung cancer LDCT screening and mortality reduction - evidence, pitfalls and future perspectives. Nat Rev Clin Oncol 2021;18:135-51. [Crossref] [PubMed]
- Okumura T, Asamura H, Suzuki K, et al. Intrapulmonary metastasis of non-small cell lung cancer: a prognostic assessment. J Thorac Cardiovasc Surg 2001;122:24-8. [Crossref] [PubMed]
- Chen TF, Xie CY, Rao BY, et al. Surgical treatment to multiple primary lung cancer patients: a systematic review and meta-analysis. BMC Surg 2019;19:185. [Crossref] [PubMed]
- Jeon YJ, Lee J, Shin S, et al. Prognostic impact of micropapillary and solid histological subtype on patients undergoing curative resection for stage I lung adenocarcinoma according to the extent of pulmonary resection and lymph node assessment. Lung Cancer 2022;168:21-9. [Crossref] [PubMed]
- Gajra A, Newman N, Gamble GP, et al. Effect of number of lymph nodes sampled on outcome in patients with stage I non-small-cell lung cancer. J Clin Oncol 2003;21:1029-34. [Crossref] [PubMed]
- Osarogiagbon RU, Ogbata O, Yu X. Number of lymph nodes associated with maximal reduction of long-term mortality risk in pathologic node-negative non-small cell lung cancer. Ann Thorac Surg 2014;97:385-93. [Crossref] [PubMed]