Prognostic value of circulating proteins at diagnosis among patients with lung cancer: a comprehensive analysis by smoking status
Highlight box
Key findings
• The circulating proteins in non-small cell lung cancer (NSCLC) patients varied by stage, histology, and smoking history. Before taking these factors into account, more than 20 proteins showed associations with overall survival, but after adjustment for these confounders and correcting for multiple testing, only interleukin 8 (IL8) and hepatocyte growth factor (HGF) remained associated with overall survival among never smoking NSCLC patients, while none of the measured proteins remained associated with survival among NSCLC patients with smoking history.
What is known and what is new?
• Despite advancements in lung cancer diagnostics and therapeutics, its 5-year survival remains poor. Recently, efforts were made to find biomarkers that could identify patients at higher risk of lung cancer mortality. However, previous studies had several limitations with respect to sample size, follow-up time, and generalizability that hindered conclusive findings. Further, with the decline in smoking prevalence in high-income countries, the proportion of lung cancers diagnosed in patients without smoking history has been increasing in recent decades, and only few studies investigated biomarkers with prognostic values among never smoking lung cancer patients.
• In this study, we measured 91 circulating immune- and cancer-related proteins from almost 1,000 NSCLC patients, of whom 244 had never smoked. We identified two proteins that are associated with NSCLC survival only among never smoking patients. However, integrating proteins into the models with clinical risk factors did not improve the predictive performance of NSCLC prognosis.
What is the implication, and what should change now?
• Our study highlights the importance of considering smoking status and tumor stage in analysis of circulating proteins in relation survival among NSCLC patients. We encourage future well-powered studies that investigate a broader panel of circulating biomarkers in relation to cancer outcome.
Introduction
Lung cancer is the leading cause of cancer death worldwide. In 2022, an estimated 2.5 million people were diagnosed with lung cancer and 1.8 million died of this disease around the world (1). Smoking remains the most important risk factor for lung cancer, accounting for over 85% of lung cancer cases (2,3), but with the decline in smoking prevalence in many high-income countries, the proportion of lung cancers diagnosed in patients without smoking history has been increasing in recent decades (4,5). Lung cancer among never smokers has been documented to have distinct epidemiological, clinical, molecular and genetic features from lung cancer among smoker patients, yet it is less studied (3,6,7).
Some studies have suggested that circulating proteins, especially biomarkers related to the immune system, can improve prediction of lung cancer survival (8-11). Our team previously analysed pre-diagnostic level of 1,159 proteins in 708 cohort participants subsequently diagnosed with lung cancer, but we did not identify any robust associations with survival (12). However, our study used measurements of proteins taken up to 3 years before diagnosis, and like most other studies we only analysed lung cancer patients with a smoking history. Studies are lacking among lung cancer patients who have never smoked cigarettes, which is important since levels of immune and inflammation biomarkers could be altered by smoking (13-17). Consequently, it remains unclear whether levels of circulating proteins measured at the time of diagnosis, and their associations with lung cancer survival, are different across lung cancer patients with and without a smoking history.
We performed a proteomic analysis on plasma samples that were collected at diagnosis from more than 900 patients with non-small cell lung cancer (NSCLC) who were originally recruited to a large multi-centric prospective cohort study of lung cancer in central and eastern Europe. In this analysis, we aimed to explore the prognostic significance of circulating protein levels at the time of lung cancer diagnosis, among patients with and without smoking history. We present this article in accordance with the REMARK reporting checklist (available at https://tlcr.amegroups.com/article/view/10.21037/tlcr-24-242/rc).
Methods
Study population, recruitment, sample collection, and follow-up
Participants were originally recruited to a large prospective study to assess the survival of early-stage NSCLC in central and eastern Europe, which has been described previously (18). In brief, from April 2007 to July 2016, patients with newly diagnosed surgically resected stage I–IIIA NSCLC, were recruited from 9 sites in Russian Federation, Czechia, Romania, Serbia, and Poland. Upon recruitment, blood samples were collected from the participants before receiving any treatment for their disease, and were then stored at a temperature of −70 ℃. Ever smokers were defined as participants who reported to have smoked at least 100 cigarettes through their lifetime, otherwise, the participants were considered as never smokers. Patients were followed twice per year through an active and a passive process to determine vital status, disease progression, and treatments. At recruitment, all participants provided written informed consent to participate in this study and this study was approved by the ethics committee of the International Agency for Research on Cancer (No. 06-11-A1 and No. 12-26-A1). The study was conducted in accordance with the Declaration of Helsinki (as revised in 2013). All participating institutions were informed and agreed with the study.
Sample selection
Out of the original 2,052 patients, we excluded 384 participants who either did not have plasma samples (n=192), or were diagnosed with neuroendocrine tumors (n=192). We stratified the remaining 1,668 participants by smoking status. To ensure a sufficient sample size in each smoking-status group, we selected all 246 never smokers, and randomly selected 377 patients from the 493 former smokers, and a further 377 random patients from the 929 current smokers in our study population.
Proteomics assays
We used the Olink proteomics platform (https://www.olink.com/) in Uppsala, Sweden to measure the relative concentrations of circulating proteins. The platform is based on proximity extension assays (PEAs) that are highly sensitive, avoid cross-reactivity, and have high reproducibility (19). It provides high-throughput semi-quantitative concentration measurements of annotated proteins by quantitative polymerase chain reaction (qPCR), and has been applied widely for proteomic measurement in various studies (20). We measured circulating levels of 92 proteins using the “immuno-oncology” panel based on their biological function (available online: https://cdn.amegroups.cn/static/public/tlcr-24-242-1.xlsx), of which 44 are involved in the inflammatory response. Protein measurements were expressed as normalized protein expression (NPX) values for each protein per individual. NPX is Olink’s relative protein quantification unit on a log2 scale, and the detailed normalization procedure was described before (21). In brief, it’s comprised of three main steps: normalization to the extension control, log2 transformation, and level adjustment using the plate control. We replaced protein values that were below the lower limit of detection (LOD) with the LOD divided by the square root of 2 and rescaled each protein to have a mean of 0 and a standard deviation (SD) of one. We didn’t observe protein levels above upper LOD. We excluded one protein (IL33) which had no variance across the patients. Of the remaining 91 proteins, only 5 had missing values. These include IL4 which was missing for 47 participants (4.8%), and four other proteins (IL1-alpha, IL2, IL12RB1, and IL13) which were only missing for one random participant. Because of the randomness of the missing values that were due to failed datapoints (assay/chip failure) and the small number of individuals with missing data, we replaced the missing values for the five proteins with the mean values of the study population. Furthermore, of the selected 1,000 samples, 14 were excluded due to contamination (n=11), and protein measurement failure (n=3). Consequently, 91 proteins from a total of 986 patients were included in the current analysis (Figure 1).
Sample size
Given 453 (46%) all-cause deaths accumulated among 986 patients during 5 years of follow-up. Assuming a significant P value threshold of 0.0005, this sample size provides at least 80% power to identify protein markers with a hazard ratio (HR) (per 1-SD increment) above 1.23 (22).
Statistical analysis
To examine the relationships between levels of circulating proteins with smoking status (ever and never) and TNM stage (continuous, stage IA, IB, IIA, IIB, and IIIA) at diagnosis, we used survey-weighted linear regression model (“survey” package in R version 4.0.4) (23), with weighting for smoking prevalence in the original cohort, and levels of circulating proteins as outcome. The weighting values were applied for current, former, and never smoking individuals. We calculated the weighting values by using the prevalence of current, former, and never smoking status in the full cohort to divide the corresponding prevalence in the protein cohort. The model for smoking status was adjusted for age at diagnosis (per 1-year), sex, education level (elementary, high school, and university), alcohol intake (never and ever), histology (adenocarcinoma and squamous cell carcinoma), and stage (continuous, stage IA, IB, IIA, IIB, and IIIA), while the model for TNM stage was adjusted for age at diagnosis, sex, alcohol intake, histology, and smoking status (never and ever).
For survival analysis, the entry time was set as the date of NSCLC diagnosis, and the exit time was set at the date of death, last contact date, or at 5 years after diagnosis, whichever occurred first. We truncated follow-up at 5 years to reduce the heterogeneity in the length of follow-up time due to the long length of recruitment (2007 to 2016). We used weighted Kaplan-Meier analysis to estimate the probability of overall survival and its 95% confidence interval (CI) at 1, 3, and 5 years after diagnosis, to provide representative survival estimates across all smoking categories. We further performed a sensitivity analysis to assess the risk of disease progression (recurrence, metastasis, and death whichever occurred first) as an alternative endpoint. We used three survey-weighted Cox models to assess the relationship between overall survival and levels of proteins. Model 1 was adjusted for age at diagnosis (per 1-year) and sex, model 2 was additionally adjusted for education level (elementary, high school, and university), smoking status (never and ever), alcohol intake (never and ever), and histology (adenocarcinoma and squamous cell carcinoma), and model 3 further included stage (continuous, stage IA, IB, IIA, IIB, and IIIA). Further adjustment for treatment (chemotherapy and radiation therapy) did not affect the observed estimates. Therefore, we proceeded with the more parsimonious model for subsequent analyses. We added an interaction term between protein concentrations and smoking status (never and ever) in these models to assess whether there is any heterogeneity in the association between proteins and overall mortality by smoking status.
We defined the association with P value less than 0.05 as nominal significance, and we accounted for multiple testing based on the ‘effective-number-of-tests (ENT)’. The ENT method accounts for multiple testing by applying a Bonferroni correction using the number of independent tests as the number of principal components needed to explain 95% of the variance in protein abundance (24).
To investigate the potential predictive utility of proteins for survival among NSCLC patients, we fit an integrated (protein + clinical) model for all patients, and separately for ever and never smoking subgroups. The clinical variables included age at diagnosis, sex, smoking status (for overall model), alcohol intake, histology, and stage. Further, to avoid overfitting, we did not include education (which did not show significant effects) in the model for never smoking patients (86 deaths). We selected proteins by using the LASSO Cox proportional hazards models (“glmnet” package in R version 4.0.4) (25). We used 10-fold cross-validation to confirm a suitable shrinkage parameter (λ), and randomly generated 1,000 different datasets wherein 80% of patients were served as the training set. For each training set, we applied the LASSO Cox regression models. To determine the most relevant proteins for incorporation into our risk prediction models, we selected the top 3 proteins that were chosen across the 1,000 training sets. Subsequently, we fit three-protein based risk models in the training sets using Cox proportional regression models and validated their performance in the remaining 20% of patients. The evaluation was based on the median C-index value calculated across the 1,000 iterations. Finally, we estimated the difference in C-indices between integrated (protein + clinical) models and clinical-only models across the 1,000 iterations of the 20% patient subset.
All tests were two-sided, and analyses were performed using R software.
Results
Descriptive statistics
For the 986 patients, the mean age at diagnosis was 64.4 years (SD 8.84 years), and 66.6% of patients were male. All patients underwent surgical resection for their tumor, while 25.8% further received chemotherapy, and 17.3% received radiation therapy. Compared to ever smoking patients, those who never smoked were more likely to have a histology of adenocarcinoma (86.5% vs. 40.0%) and be diagnosed at stage I (stage I: 63.9% vs. 50.1%). Never smoking patients were also less likely to regularly drink alcohol (16.8% vs. 52.3%) (Table 1).
Table 1
Characteristics | Never smoking (N=244) | Ever smoking (N=742) | Total (N=986) |
---|---|---|---|
Age at diagnosis (years), mean (SD) | 65.1 (10.4) | 64.1 (8.27) | 64.4 (8.84) |
Sex, n (%) | |||
Female | 197 (80.7) | 132 (17.8) | 329 (33.4) |
Male | 47 (19.3) | 610 (82.2) | 657 (66.6) |
Centers†, n (%) | |||
Czechia | 3 (1.2) | 21 (2.8) | 24 (2.4) |
Poland | 24 (9.8) | 223 (30.1) | 247 (25.1) |
Romania | 4 (1.6) | 30 (4.0) | 34 (3.4) |
Russian Federation | 213 (87.3) | 379 (51.1) | 592 (60.0) |
Serbia | 0 (0.0) | 89 (12.0) | 89 (9.0) |
Year at diagnosis, n (%) | |||
2007–2010 | 70 (28.7) | 152 (20.5) | 222 (22.5) |
2011–2013 | 94 (38.5) | 421 (56.7) | 515 (52.2) |
2014–2016 | 80 (32.8) | 169 (22.8) | 249 (25.3) |
Education, n (%) | |||
Elementary | 47 (19.3) | 273 (36.8) | 320 (32.5) |
High school | 88 (36.1) | 288 (38.8) | 376 (38.2) |
University | 109 (44.7) | 179 (24.1) | 288 (29.3) |
Missing | 0 | 2 | 2 |
Regular alcohol intake, n (%) | |||
Never | 203 (83.2) | 354 (47.7) | 557 (56.5) |
Ever | 41 (16.8) | 388 (52.3) | 429 (43.5) |
BMI at diagnosis | |||
Mean (SD) | 28.0 (4.74) | 26.0 (4.33) | 26.5 (4.52) |
Missing | 0 | 1 | 1 |
Smoking pack-years | |||
Mean (SD) | NA | 43.2 (21.6) | 43.2 (21.6) |
Missing | NA | 3 | 3 |
Histology, n (%) | |||
Adenocarcinoma | 211 (86.5) | 297 (40.0) | 508 (51.5) |
Squamous cell carcinoma | 33 (13.5) | 445 (60.0) | 478 (48.5) |
Stage, n (%) | |||
IA | 90 (36.9) | 170 (22.9) | 260 (26.4) |
IB | 66 (27.0) | 202 (27.2) | 268 (27.2) |
IIA | 25 (10.2) | 122 (16.4) | 147 (14.9) |
IIB | 16 (6.6) | 77 (10.4) | 93 (9.4) |
IIIA | 47 (19.3) | 171 (23.0) | 218 (22.1) |
Chemotherapy, n (%) | 49 (20.1) | 205 (27.6) | 254 (25.8) |
Radiation therapy, n (%) | 36 (14.8) | 135 (18.2) | 171 (17.3) |
All cause death, n (%) | 86 (35.2) | 367 (49.5) | 453 (45.9) |
Lung cancer death, n (%) | 64 (26.2) | 216 (29.1) | 280 (28.4) |
Survival probability (%), OS (95% CI) | |||
1-year | 90.6 (87.0–94.3) | 82.6 (79.9–85.4) | 84.1 (81.6–86.6) |
3-year | 77.7 (72.7–83.2) | 59.6 (56.2–63.3) | 62.7 (59.5–66.0) |
5-year | 63.1 (57.1–69.7) | 46.6 (42.9–50.7) | 48.9 (45.5–52.6) |
†, patients were recruited from 2 sites in Russian Federation (departments of thoracic surgery in N.N. Blokhin National Medical Research Center of Oncology, and the City Clinical Oncological Hospital No. 1 in Moscow), 3 sites in Czech Republic (Motol University Hospital in Prague, Masaryk Memorial Cancer Institute in Brno and University Hospital Olomouc in Olomouc), 1 site in Romania (Marius Nasta Institute of Pneumology in Bucharest), 1 site in Serbia (Clinical Centre of Serbia in Belgrade), and 2 sites in Poland (Institute of Tuberculosis and Lung Diseases in Warsaw, and Military Medical Academy in Lodz). SD, standard deviation; OS, overall survival; CI, confidence interval; NA, no observation.
There were 453 deaths over 5 years. The overall survival of participants with NSCLC was 84.1% (95% CI: 81.6–86.6%) at 1 year, 62.7% (95% CI: 59.5–66.0%) at 3 years, and 48.9% (95% CI: 45.5–52.6%) at 5 years. Five-year survival was higher in never- than in ever-smokers (63.1% vs. 46.6%, P<0.001) (Figure S1).
Protein levels by smoking status and TNM stage
After adjustment for potential confounders, protein levels were nominally different (P<0.05) for 32% (29/91) of the measured proteins between never and ever smokers (Figure 2A, available online: https://cdn.amegroups.cn/static/public/tlcr-24-242-1.xlsx). After controlling for multiple testing, levels of 9 proteins remained different among ever and never smokers. Compared to never smokers, ever smoker patients had significantly higher levels of hepatocyte growth factor (HGF) (P=8×10−6), MMP12 (P=3×10−6), LAMP3 (P<0.001), ANGPT2 (P<0.001), IL8 (P<0.001), and IL6 (P<0.001), and lower levels of FASLC (P=1×10-6), ICOSLG (P=4×10−6), GAL1 (P<0.001), VEGFR2 (P<0.001), and TIE2 (P=0.002).
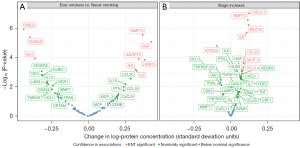
When comparing protein levels across TNM stages, 34 of 91 proteins showed nominally different levels with increasing TNM stage in the adjusted models (Figure 2B, available online: https://cdn.amegroups.cn/static/public/tlcr-24-242-1.xlsx). After correcting for multiple testing, patients with more advanced stages had significantly higher blood levels of CXCL13 (P=9×10−8), MMP12 (P=3×10−7), MUC16 (P=6×10−7), IL8 (P=2×10−6), CCL23 (P<0.001), MCP-3 (P<0.001), and CCL3 (P<0.001), but lower blood levels of ICOSLG (P=2×10−5).
After correction for multiple testing, three proteins (ICOSLG, MMP12, IL8) showed variation across both smoking status and stage at diagnosis in the adjusted models (Figure 2).
Protein levels and lung cancer survival
When analysing all patients regardless of smoking history, we found 23 proteins nominally associated with all-cause mortality after minimal adjustment for age and sex (P<0.05) (Figure 3A, available online: https://cdn.amegroups.cn/static/public/tlcr-24-242-1.xlsx). After accounting for multiple testing, five proteins remained associated with all-cause mortality, including MUC16 (HR per SD increase =1.26, 95% CI: 1.16–1.38, P=2×10−7), IL6 (HR per SD increase: 1.20, 95% CI: 1.09–1.31, P<0.001), CXCL13 (HR per SD increase: 1.19, 95% CI: 1.09–1.29, P<0.001), TRAIL (HR per SD increase: 0.83, 95% CI: 0.76–0.91, P=9×10−5), and ICOSLG (HR per SD increase: 0.81, 95% CI: 0.74–0.89, P<0.001). Further adjustment for education level, smoking status, alcohol intake, and histology did not influence these associations notably (Figure 3B). However, no protein remained associated with all-cause mortality after additionally accounting for tumour stage at diagnosis (all corrected P values >0.05) (Figure 3C). Similar results were obtained when we assessed disease progression as the outcome (Figure S2), and when we stratified the analysis by tumor stage as IA–IIA and IIB–IIIA subgroups (available online: https://cdn.amegroups.cn/static/public/tlcr-24-242-1.xlsx). However, among the proteins with nominally significant association with overall mortality in overall patients (Figure 3C). ICOSLG showed stronger inverse association in patients with stage IIB-IIIA than patients with stage IA–IIA [HR per SD increase: 0.76 (95% CI: 0.66–0.87) vs. 0.96 (95% CI: 0.84–1.10)], and MUC16 showed stronger positive association in patients with stage IA–IIA than patients with stage IIB–IIIA [HR per SD increase: 1.31 (95% CI: 1.15–1.49) vs. 1.08 (95% CI: 0.95–1.22)] (available online: https://cdn.amegroups.cn/static/public/tlcr-24-242-1.xlsx).
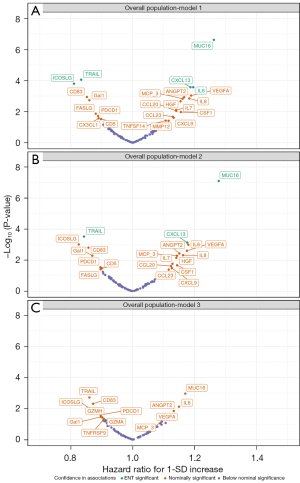
We subsequently carried out survival analysis stratified by smoking status (ever/never). Before adjusting for stage (models 1 and 2), MUC16 and TRAIL were associated with survival in ever smokers (P ENT-corrected <0.05), and MUC16, HGF, and IL8 were associated with survival among never smokers, showed ENT-significant association with survival (P ENT-corrected <0.05) (Figure 4A-4D). After adjustment for stage (model 3), no protein remained associated with survival in ever smokers (Figure 4E), but IL8 (HR per SD increase: 1.40, 95% CI: 1.18–1.65, P<0.001) and HGF (HR per SD increase: 1.45, 95% CI: 1.18–1.79, P<0.001) remained associated with survival in never smokers (Figure 4F, available online: https://cdn.amegroups.cn/static/public/tlcr-24-242-1.xlsx).
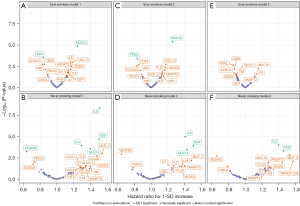
After comprehensive adjustments (model 3), 22 proteins showed nominally significant associations with survival either in the overall study population, or among never/ever smoking subgroups. Heterogeneity test showed that, seven out of 22 proteins were differently associated with survival across the smoking strata (P-heterogeneity<0.05). MCP-3, HGF, CCL20, and TNFRSF12A showed larger HRs in never smokers compared to patients who ever smoked, and VEGFR2 showed stronger inverse association with overall mortality in never smokers than ever smokers. The association between TNF levels and lung cancer survival was in different directions across the smoking strata (ever smokers: HR per SD increase: 0.90, 95% CI: 0.81 to 1.00; never smokers: HR per SD increase: 1.22, 95% CI: 0.89–1.65, P-heterogeneity=0.01) (Figure 5).
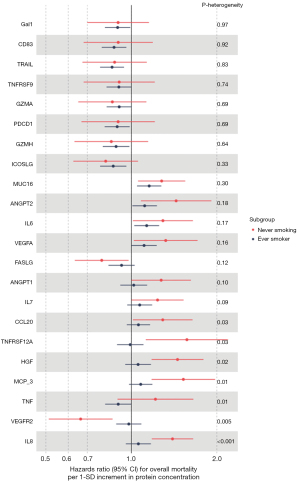
Assessment of predictive utility of proteins for lung cancer prognosis
We applied LASSO Cox regression for the 1,000 different training iterations, each time to select five proteins for prediction of mortality after NSCLC diagnosis. ICOSLG, MUC16, and ANGPT2 were the top 3 selected proteins across the 1,000 iterations for the overall patients, which were each selected in 75% of iterations (Figure S3). By smoking status, the top proteins were TRAIL, MUC16, and ANGPT2 for smoker patients (selected in at least 75% of iterations), and VEGFR2, HGF and TNFRSF12A for never smoker patients (selected in at least 50% of iterations) (Figure S4). When integrating selected proteins with clinical factors to predict the risk of overall mortality for patients (Table S1), among ever smoking patients, the C-index was 0.63 (95% CI: 0.57–0.68) with clinical factors only and 0.64 (95% CI: 0.58–0.70) when adding proteins (P=0.20 for improvement with proteins). Among never smoking patients, the C-index was 0.68 (95% CI: 0.56–0.80) with clinical factors only and 0.72 (95% CI: 0.59–0.82) when adding proteins (P=0.28 for improvement with proteins) (Table S2).
Protein levels and overall survival by histology
Stratifying the analysis by histology revealed no significant associations between proteins and overall mortality risk in patients with adenocarcinoma or squamous cell carcinoma after controlling for multiple testing. However, the protein profiles demonstrating nominally significant associations with overall mortality differed between the two patient groups (Figure S5).
Discussion
In this prospective study, we evaluated 91 circulating proteins in relation to lung cancer survival based on samples collected at the time of diagnosis in patients with stage I–IIIA NSCLC. We did not identify any protein strongly associated with lung cancer survival in ever smokers after taking clinical stage into account, but two proteins (HGF and IL8) were found associated with survival in never smokers. Integrating protein levels into a prognostic model did not improve prediction of lung cancer prognosis of NSCLC patients.
We recently published promising results indicating that pre-diagnostic levels of circulating proteins have strong potential in improving risk prediction of incident lung cancer (26,27). However, when we analysed the same data to evaluate the association between pre-diagnostic levels of proteins with lung cancer survival, we found that pre-diagnostic proteins did not improve prediction of prognosis after lung cancer diagnosis (12). Similar to our previous findings for protein levels before diagnosis, in the current study we found limited evidence for potential of circulating proteins in improving lung cancer prognosis when measured at the time of diagnosis in relation to NSCLC survival. Whereas as some proteins were found associated with survival in never smokers in this study, they did not improve prediction of survival beyond clinical factors, particularly tumor stage.
Proteins involved in inflammation and the immune system have been widely studied for the prognosis and treatment of NSCLC over the last decade (28,29). As an example, a proteomic signature that is linked to chronic inflammation, was able to predict NSCLC therapy response in a clinical trial and was subsequently proposed to have potential predictive value for NSCLC prognosis (10,30). In the current study, we focused on a group of 91 proteins measured on the Olink immuno-oncology panel, which comprise proteins associated with cancer development, inflammation, and immune function. We found 32% of the proteins nominally associated with survival of NSCLC patients in minimally adjusted models (age- and sex-adjustment). However, because most of these proteins were also associated with disease stage, and adjusting for tumour stage accounted for the survival association of each protein. This suggest that many of the studied proteins are proxies of disease severity and cancer stage and they do not add more prognostic and predictive values beyond those gained from clinical information.
It is challenging to identify robust prognostic markers for lung cancer survival, partly because lung cancer is one of the cancers with the highest frequency and number of mutations (31,32). Moreover, the incidence of mutations in lung cancer varies across histology, ethnicity, and smoking status (33,34). Most previous studies on circulating proteins and lung cancer survival had smaller sample size compared to this study which precluded accurate assessment by smoking status and tumor stage (8,9,35-37). Particularly, protein levels have been rarely studied in relation to lung cancer survival among never smokers. In this study we measured protein levels of NSCLC patients at diagnosis, and found not only that levels of many proteins differ by smoking status, but also a few of them showed stronger association with NSCLC survival in patients who never smoked, than ever smokers, which were not identified by previous studies including mostly ever smoking patients. After correction for multiple testing, IL8 and HGF remained significantly associated with overall mortality in NSCLC patients who never smoked. In previous studies, elevated IL8 was linked to an unfavorable tumor microenvironment and was shown to potentially serve as a therapeutic target for NSCLC (38). Similarly, HGF acts as a stromal cell-derived factor that strongly affects cancer cell invasiveness in the tumor microenvironment, that has also been targeted in anticancer drug discovery over the past decade (39).
Strengths of this study are its large sample size that allowed performing a comprehensive analysis across NSCLC patients with various stage, histology, and smoking status; inclusion of a large number of never smokers which allowed a detailed assessment of the values of proteins among this population that has been rarely investigated; the recruitment of patients from five countries which enhances the generalizability of the findings; and using stringent statistical approaches to account for potential biases and random findings. Our study lacked external validation for the prediction models but given that we did not identify improvements in prediction when using the proteins, we feel certain that this result would not be altered if an external validation sample were available. We did not consider sex and histology during the sample selection, which are differently distributed among never and ever smokers. However, we tried to address this limitation in the statistical analysis, by adjusting all models for sex and histology. An important limitation is that we assessed only one protein panel containing 92 pre-selected proteins, although we chose this panel because it contained the proteins that a-priori appeared most likely to be informative. However, we welcome future studies of similar design that assess a broader set of protein markers in relation to lung cancer survival.
Conclusions
In conclusion, analysis of 91 circulating immune- and cancer-related proteins in 986 patients with stage I-IIIA NSCLC did not provide strong evidence for an important potential in lung cancer prognostics. With the exception of HGF and IL8 which were only associated with NSCLC survival in never smokers, none of the proteins showed strong association with NSCLC survival in any of the study subgroups. Integration of the protein markers into a statistical model that contains demographics and clinical factors did not improve the prediction of NSCLC survival. While our study highlights the importance of considering smoking status and tumor stage in future analysis of circulating proteins in relation to cancer outcomes, well-powered future studies are needed to investigate a broader panel of the blood proteome and other biomarkers such as circulating tumor DNA (ctDNA) and circulating tumor cells (CTCs), in relation to survival in NSCLC patients.
Acknowledgments
Funding: None.
Footnote
Reporting Checklist: The authors have completed the REMARK reporting checklist. Available at https://tlcr.amegroups.com/article/view/10.21037/tlcr-24-242/rc
Data Sharing Statement: Available at https://tlcr.amegroups.com/article/view/10.21037/tlcr-24-242/dss
Peer Review File: Available at https://tlcr.amegroups.com/article/view/10.21037/tlcr-24-242/prf
Conflicts of Interest: All authors have completed the ICMJE uniform disclosure form (available at https://tlcr.amegroups.com/article/view/10.21037/tlcr-24-242/coif). The authors have no conflicts of interest to declare.
Ethical Statement: The authors are accountable for all aspects of the work in ensuring that questions related to the accuracy or integrity of any part of the work are appropriately investigated and resolved. All participants provided written informed consent to participate in this study and this study was approved by the ethics committee of the International Agency for Research on Cancer (No. 06-11-A1 and No. 12-26-A1). The study was conducted in accordance with the Declaration of Helsinki (as revised in 2013). All participating institutions were informed and agreed with the study.
Disclaimer: Where authors are identified as personnel of the International Agency for Research on Cancer/World Health Organization, the authors alone are responsible for the views expressed in this article and they do not necessarily represent the decisions, policy, or views of the International Agency for Research on Cancer/World Health Organization.
Open Access Statement: This is an Open Access article distributed in accordance with the Creative Commons Attribution-NonCommercial-NoDerivs 4.0 International License (CC BY-NC-ND 4.0), which permits the non-commercial replication and distribution of the article with the strict proviso that no changes or edits are made and the original work is properly cited (including links to both the formal publication through the relevant DOI and the license). See: https://creativecommons.org/licenses/by-nc-nd/4.0/.
References
- Bray F, Laversanne M, Sung H, et al. Global cancer statistics 2022: GLOBOCAN estimates of incidence and mortality worldwide for 36 cancers in 185 countries. CA Cancer J Clin 2024;74:229-63. [Crossref] [PubMed]
- Duma N, Santana-Davila R, Molina JR. Non-Small Cell Lung Cancer: Epidemiology, Screening, Diagnosis, and Treatment. Mayo Clin Proc 2019;94:1623-40. [Crossref] [PubMed]
- Carroll NM, Burnett-Hartman AN, Rendle KA, et al. Smoking status and the association between patient-level factors and survival among lung cancer patients. J Natl Cancer Inst 2023;115:937-48. [Crossref] [PubMed]
- Pelosof L, Ahn C, Gao A, et al. Proportion of Never-Smoker Non-Small Cell Lung Cancer Patients at Three Diverse Institutions. J Natl Cancer Inst 2017;109:djw295. [Crossref] [PubMed]
- Cufari ME, Proli C, De Sousa P, et al. Increasing frequency of non-smoking lung cancer: Presentation of patients with early disease to a tertiary institution in the UK. Eur J Cancer 2017;84:55-9. [Crossref] [PubMed]
- Sun S, Schiller JH, Gazdar AF. Lung cancer in never smokers--a different disease. Nat Rev Cancer 2007;7:778-90. [Crossref] [PubMed]
- Samet JM, Avila-Tang E, Boffetta P, et al. Lung cancer in never smokers: clinical epidemiology and environmental risk factors. Clin Cancer Res 2009;15:5626-45. [Crossref] [PubMed]
- Bodelon C, Polley MY, Kemp TJ, et al. Circulating levels of immune and inflammatory markers and long versus short survival in early-stage lung cancer. Ann Oncol 2013;24:2073-9. [Crossref] [PubMed]
- Meaney CL, Zingone A, Brown D, et al. Identification of serum inflammatory markers as classifiers of lung cancer mortality for stage I adenocarcinoma. Oncotarget 2017;8:40946-57. [Crossref] [PubMed]
- Gregorc V, Novello S, Lazzari C, et al. Predictive value of a proteomic signature in patients with non-small-cell lung cancer treated with second-line erlotinib or chemotherapy (PROSE): a biomarker-stratified, randomised phase 3 trial. Lancet Oncol 2014;15:713-21. [Crossref] [PubMed]
- Lai J, Yang S, Chu S, et al. Determination of a prediction model for therapeutic response and prognosis based on chemokine signaling-related genes in stage I-III lung squamous cell carcinoma. Front Genet 2022;13:921837. [Crossref] [PubMed]
- Feng X, Muller DC, Zahed H, et al. Evaluation of pre-diagnostic blood protein measurements for predicting survival after lung cancer diagnosis. EBioMedicine 2023;92:104623. [Crossref] [PubMed]
- Shiels MS, Katki HA, Freedman ND, et al. Cigarette smoking and variations in systemic immune and inflammation markers. J Natl Cancer Inst 2014;106:dju294. [Crossref] [PubMed]
- Tibuakuu M, Kamimura D, Kianoush S, et al. The association between cigarette smoking and inflammation: The Genetic Epidemiology Network of Arteriopathy (GENOA) study. PLoS One 2017;12:e0184914. [Crossref] [PubMed]
- Luetragoon T, Rutqvist LE, Tangvarasittichai O, et al. Interaction among smoking status, single nucleotide polymorphisms and markers of systemic inflammation in healthy individuals. Immunology 2018;154:98-103. [Crossref] [PubMed]
- Ugur MG, Kutlu R, Kilinc I. The effects of smoking on vascular endothelial growth factor and inflammation markers: A case-control study. Clin Respir J 2018;12:1912-8. [Crossref] [PubMed]
- Zahed H, Johansson M, Ueland PM, et al. Epidemiology of 40 blood biomarkers of one-carbon metabolism, vitamin status, inflammation, and renal and endothelial function among cancer-free older adults. Sci Rep 2021;11:13805. [Crossref] [PubMed]
- Sheikh M, Virani S, Robbins HA, et al. Survival and prognostic factors of early-stage non-small cell lung cancer in Central and Eastern Europe: A prospective cohort study. Cancer Med 2023;12:10563-74. [Crossref] [PubMed]
- Assarsson E, Lundberg M, Holmquist G, et al. Homogenous 96-plex PEA immunoassay exhibiting high sensitivity, specificity, and excellent scalability. PLoS One 2014;9:e95192. [Crossref] [PubMed]
- Eldjarn GH, Ferkingstad E, Lund SH, et al. Large-scale plasma proteomics comparisons through genetics and disease associations. Nature 2023;622:348-58. [Crossref] [PubMed]
- Wik L, Nordberg N, Broberg J, et al. Proximity Extension Assay in Combination with Next-Generation Sequencing for High-throughput Proteome-wide Analysis. Mol Cell Proteomics 2021;20:100168. [Crossref] [PubMed]
- Hsieh FY, Lavori PW. Sample-size calculations for the Cox proportional hazards regression model with nonbinary covariates. Control Clin Trials 2000;21:552-60. [Crossref] [PubMed]
- Lumley T. Analysis of Complex Survey Samples. Journal of Statistical Software 2004;9:1-19.
- Galwey NW. A new measure of the effective number of tests, a practical tool for comparing families of non-independent significance tests. Genet Epidemiol 2009;33:559-68. [Crossref] [PubMed]
- Friedman J, Hastie T, Tibshirani R. Regularization Paths for Generalized Linear Models via Coordinate Descent. J Stat Softw 2010;33:1-22.
- The blood proteome of imminent lung cancer diagnosis. Nat Commun 2023;14:3042. [Crossref] [PubMed]
- Feng X, Wu WY, Onwuka JU, et al. Lung cancer risk discrimination of prediagnostic proteomics measurements compared with existing prediction tools. J Natl Cancer Inst 2023;115:1050-9. [Crossref] [PubMed]
- Walker MJ, Zhou C, Backen A, et al. Discovery and Validation of Predictive Biomarkers of Survival for Non-small Cell Lung Cancer Patients Undergoing Radical Radiotherapy: Two Proteins With Predictive Value. EBioMedicine 2015;2:841-50. [Crossref] [PubMed]
- Suwinski R, Giglok M, Galwas-Kliber K, et al. Blood serum proteins as biomarkers for prediction of survival, locoregional control and distant metastasis rate in radiotherapy and radio-chemotherapy for non-small cell lung cancer. BMC Cancer 2019;19:427. [Crossref] [PubMed]
- Leal TA, Argento AC, Bhadra K, et al. Prognostic performance of proteomic testing in advanced non-small cell lung cancer: a systematic literature review and meta-analysis. Curr Med Res Opin 2020;36:1497-505. [Crossref] [PubMed]
- Kandoth C, McLellan MD, Vandin F, et al. Mutational landscape and significance across 12 major cancer types. Nature 2013;502:333-9. [Crossref] [PubMed]
- Mendiratta G, Ke E, Aziz M, et al. Cancer gene mutation frequencies for the U.S. population. Nat Commun 2021;12:5961. [Crossref] [PubMed]
- Dearden S, Stevens J, Wu YL, et al. Mutation incidence and coincidence in non small-cell lung cancer: meta-analyses by ethnicity and histology (mutMap). Ann Oncol 2013;24:2371-6. [Crossref] [PubMed]
- Kerr KM, Dafni U, Schulze K, et al. Prevalence and clinical association of gene mutations through multiplex mutation testing in patients with NSCLC: results from the ETOP Lungscape Project. Ann Oncol 2018;29:200-8. [Crossref] [PubMed]
- Liao C, Yu Z, Guo W, et al. Prognostic value of circulating inflammatory factors in non-small cell lung cancer: a systematic review and meta-analysis. Cancer Biomark 2014;14:469-81. [Crossref] [PubMed]
- Vaes RDW, Reynders K, Sprooten J, et al. Identification of Potential Prognostic and Predictive Immunological Biomarkers in Patients with Stage I and Stage III Non-Small Cell Lung Cancer (NSCLC): A Prospective Exploratory Study. Cancers (Basel) 2021;13:6259. [Crossref] [PubMed]
- Huang H, Yang Y, Zhu Y, et al. Blood protein biomarkers in lung cancer. Cancer Lett 2022;551:215886. [Crossref] [PubMed]
- Pasello G, Fabricio ASC, Del Bianco P, et al. Sex-related differences in serum biomarker levels predict the activity and efficacy of immune checkpoint inhibitors in advanced melanoma and non-small cell lung cancer patients. J Transl Med 2024;22:242. [Crossref] [PubMed]
- Matsumoto K, Umitsu M, De Silva DM, et al. Hepatocyte growth factor/MET in cancer progression and biomarker discovery. Cancer Sci 2017;108:296-307. [Crossref] [PubMed]