Stem-like exhausted CD8 T cells in pleural effusions predict improved survival in non-small cell lung cancer (NSCLC) and mesothelioma
Highlight box
Key findings
• Higher frequency of pleural effusion (PE) stem-like exhausted CD8 T cells (Texstem) is associated with improved survival in both non-small cell lung cancer (NSCLC) and mesothelioma whilst terminally exhausted CD8 T cells (Texterm) did not correlate with survival outcomes.
What is known and what is new?
• Exhausted CD8 T cells exhibit a gradient of phenotypic and functional states and are broadly comprised of functional ‘stem-like’ exhausted T cells that transition into terminally exhausted T cells that lose their cytotoxic and proliferative capacity. Little is yet known about the prognostic significance of these different exhausted T cell subsets.
• This study showed that malignant PEs can be used to examine the different exhausted CD8 T cell subsets and that Texstem are associated with favourable prognosis in both NSCLC and mesothelioma. Mass cytometry and single cell ribonucleic acid (RNA) and T cell receptor (TCR) sequencing of Texstem and Texterm allowed greater insight into their phenotypic heterogeneity, functional complexities and antigen specificity.
What is the implication, and what should change now?
• Our study suggests that Texstem may serve as a new prognostic biomarker and its utility should be examined in larger prospective studies.
Introduction
Cancers harbour somatic mutations that can be presented as novel antigens to the immune system and engage cytotoxic CD8 T cells (1). The number, location and functional status of intratumoral CD8 T cells has been shown to affect clinical outcomes in multiple solid tumors (2-5).
However, successful elimination of cancer cells by CD8 T cells is hampered by a broad set of immunosuppressive pathways in the tumor microenvironment (TME), one of which is T-cell exhaustion (6,7). Understanding the nature and degree of this exhaustion in tumor-associated T cells is crucial, as it could inform immunotherapy treatment regimens. It is well recognised that tumor infiltrating lymphocytes (TILs) exhibit an exhausted phenotype and impaired effector function, partly driven by long term T-cell receptor (TCR) engagement by cancer antigens (6-10). Based on work in chronic viral infections in mouse models, a nuanced view of T-cell exhaustion has emerged which recognises that T-cell exhaustion is a progressive state. Instead of being a homogenous population, exhausted CD8 T (Tex) cells exhibit a gradient of phenotypic and functional states with distinct transcriptional and epigenetic signatures (11-15). These cell states are delimited by progenitor or ’stem-like’ exhausted T (Texstem) cells which retain proliferation and cytotoxic abilities. Following chronic antigenic stimulation, Texstem cells gradually transition through a series of poorly defined intermediary states into terminally exhausted T (Texterm) cells that are functionally inefficient and have limited reinvigoration capacity.
Several studies have identified Texstem and Texterm cells within the TME (16-19), and in animal models, Texstem cells are associated with improved tumor control (14). Across a series of cancers, Texstem cells are associated with improved outcomes following immune checkpoint blockade (ICPB) (14,18,19), whilst there is evidence in vitro that Texterm cells can be associated with a poor prognosis (20). In a cohort of 14 non-small cell lung cancer (NSCLC) patients, single cell sequencing was used to demonstrate that a higher ratio of ‘pre-exhausted’ to exhausted T cells was associated with better prognosis (21).
One of the unique TME in advanced cancers, especially thoracic malignancies, is malignant pleural effusions (PE). Malignant PEs usually result from pleural dissemination of cancer cells, they are typically lymphocyte-rich (22), providing an interface between tumor cells and immune cells, and thus a ‘window’ on tumor-immune events. These samples also allow sequential investigation in some patients and have the benefit of requiring minimal laboratory manipulations prior to cell phenotyping. We therefore sought to determine if PE can be used to measure T-cell exhaustion and then to determine if the T-cell exhaustion status can potentially serve as a prognostic biomarker.
The field lacks a single, agreed-upon definition of Tex subsets. Researchers have distinguished Tex subsets phenotypically based on the expression levels of various combinations of inhibitory and costimulatory receptors and transcription factors (15,23-25). In this study, we chose to use the markers employed by Jansen and colleagues when sorting stem-like and terminally exhausted T cells from human tumors (23). Texstem cells are defined by intermediate expression of the inhibitory receptor programmed death 1 (PD1), undetectable expression of CD39 and positive expression of CD28, while high PD1 and CD39 co-expression indicated a Texterm phenotype. CD39 is an ectonucleotidase in the adenosine pathway and is marker of terminal T-cell exhaustion (26,27). In melanoma and kidney cancer, CD39 differentiates terminally exhausted TIM3 expressing cells from TIM3− cells (23,25). CD28 is a costimulatory molecule that is co-expressed with the transcription factor TCF1 in Texstem and is a critical marker of functional TCF1+ stem-like CD8 T cells in both chronic viral infection and cancer (12,23,28). Using these makers, Texstem and Texterm cells were defined in the malignant PE from NSCLC patients to assess their prognostic relevance and were again used in a validation study in a mesothelioma patient cohort. We also performed mass cytometry and deep single cell RNA and TCR sequencing on Texstem and Texterm cells isolated from the PE of NSCLC and mesothelioma patients to dissect the phenotypic and functional complexities of these cell subsets and examine their antigen specificity. We present this article in accordance with the STROBE reporting checklist (available at https://tlcr.amegroups.com/article/view/10.21037/tlcr-24-284/rc).
Methods
Patients and PE samples
To characterise Tex subsets and their prognostic significance, we accessed the National Centre for Asbestos Related Diseases Biobank which has collected consecutive PE samples from patients attending Sir Charles Gairdner Hospital, Perth, Australia since 2012. For CyTOF analysis, five mesothelioma and three lung cancer samples were selected based on sample availability of each tumor type. For scRNA-seq two samples were selected for each tumor type based on sample availability and adequate frequencies of Tex cell subsets for data analysis. PE samples were processed and cryopreserved in RPMI-1640 media, 50% newborn calf serum (NCS) and 10% dimethyl sulfoxide. For some samples, CD45+ cells were positively selected using EasySep Human CD45 Depletion Kit (Stemcell, cat17898) following the manufacturer’s instructions before cryopreservation. For the present study, all cases with a confirmed pathological diagnosis of NSCLC or mesothelioma and a malignant PE sample available within three months of diagnosis and prior to treatment were identified and analysed. No patients had prior pleurodesis. For a subset of cases, multiple PE samples were available.
Clinical data including age, gender, smoking history (ex/current or never), Eastern Cooperative Oncology Group (ECOG) performance status (0–1 or 2–4), Charlson Comorbidities Index (7–9 or 10–13), histological subtype [NSCLC (squamous or non-squamous); mesothelioma (epithelioid, sarcomatoid or biphasic)] and treatment [number of lines of systemic therapy received (0, 1 or ≥2) and treatment regimens] were collected from hospital records. For non-squamous NSCLC cases, epidermal growth factor receptor (EGFR), Kirsten rat sarcoma virus (KRAS), ALK, ROS1 mutation status and PD-L1 total proportion score (TPS) were recorded. The prognostic outcome measure was OS, calculated from the day of histological diagnosis until death or date of census. The study was conducted in accordance with the Declaration of Helsinki (as revised in 2013). All participants provided written informed consent. This study was approved by the Human Research Ethics Committees of Sir Charles Gairdner Osborne Park Health Care Group (RGS0000001516), the University of Western Australia and the Fred Hutchinson Cancer Centre Human Subjects Committee (IRB file/approval number 10422).
Flow cytometry
PE samples were thawed, washed, filtered using a 100 µm cell strainer to remove clumps and debris, and then resuspended in PBS 1 mM EDTA. Cells were stained with Fixable viability dye eFluor 780 (eBioscience, Carlsbad, USA) for 20 minutes at room temperature (RT) followed by cell surface staining. For cell surface staining, cells were stained with fluorochrome conjugated anti-CD3–BV510 (BioLegend, UCHT1, San Diego, USA), anti-CD4–BV711 (BD Biosciences, SK3, Milpitas, USA) or –BB515 (BD Biosciences, RPA-T4), anti-CD8–BB700 (BD Biosciences, RPA-T8), anti-PD1–PE-Cy7 (BD Biosciences, EH12.1), anti-CD39–PE (BD Biosciences, Tu66), anti-CD28–APC (BioLegend, CD28.2) antibodies prepared in Brilliant Stain Buffer (BD Biosciences, cat# 566349) for 30 minutes at 4 ℃. For intracellular staining, cells were fixed and permeabilised for 20 minutes using the Foxp3 Transcription Factor Staining Buffer Set (eBioscience), and then incubated with fluorochrome conjugated anti-TCF1 antibody–AF488 (Cell Signalling, C63D9, Danvers, USA) in 1x Permeabilization Buffer (eBioscience) for 30 minutes at RT. After washing twice, samples were collected and analysed using LSR Fortessa or FACS Melody. Data were analysed with FlowJo software version 10.6.
Percentages and ratios of Texstem (PD1mid CD39− CD28+) and Texterm (PD1hi CD39+) CD8 T cells were reported. All Tex subsets were gated on live CD3+ CD8+ CD4− T cells. Gating strategy is shown in Figure S1.
Serum mesothelin
Pre-treatment serum samples were available for 26 patients with epithelioid mesothelioma from the National Centre for Asbestos Related Diseases Biobank. Mesothelin concentrations were determined following the manufacturer’s instructions using the MESOMARK assay (Fujirebio Diagnostics, Malvern, USA).
Mass cytometry
Purified antibodies lacking carrier proteins were purchased as shown in Table S1. Antibody conjugation was performed according to the protocol provided by Fluidigm (Markham, Canada). PE samples were thawed, washed, and stained with cisplatin (5 mM) in PBS for 5 minutes on ice, and incubated with primary and secondary surface antibody cocktails for 20 minutes each on ice and fixed overnight in 2% paraformaldehyde. The next day, cells were washed and permeabilised using permeabilization buffer (eBioscience) and stained with intracellular antibodies for 30 minutes at RT followed by DNA staining (Cell-ID intercalator-Ir, Fluidigm) for 30 minutes at RT. Cells were lastly washed three times with water and run on CyTOF (Helios, Fluidigm). After CyTOF acquisition, any zero values were randomised using a uniform distribution of values between 0–1 using R and the signal of each parameter was normalised based on EQ bead values (Fluidigm). CD8 T cells were manually gated and subjected to UMAP analysis similar to that previously described using customised R scripts based on the ‘flowCore’ and ‘uwot’ R packages. Data were transformed using the logicleTransform function with parameters: w=0.25, t=16409, m=4.5, a=0 to match scaling historically used in Flowjo.
Single cell sequencing
We used scRNA-seq to profile four pre-treatment PE samples from two lung adenocarcinoma and two epithelioid mesothelioma patients (Table S1). PE samples were stained with TotalSeq-C antibody cocktails along with fluorescent antibody cocktails (Table S1). T cells were isolated by FACS sorting and loaded onto the 10x Chromium controller using Chromium Next GEM Single Cell 5’ Reagent Kits v2 (Dual Index) with Feature Barcoding technology for Cell-Surface Protein and Immune Receptor Mapping (10x Genomics) according to the manufacturer’s protocol. Quality of final libraries was assessed using Agilent 2200 TapeStation with High Sensitivity D5000 ScreenTape, quantified using a Qubit Fluorometer (ThermoFisher) and KAPA library quantification kit (Roche), and carried through to sequencing with Novaseq S1 on Illumina sequencer. Raw fastq files were pre-processed through CellRanger Pipeline (10x Genomics) and aligned to the reference genome (refdata-gex-GRCh38-2020-A). Seurat package in R library, was used to remove RNA features that appeared in less than 10 cells: VDJ and BCR genes and cells with less than 200 or more than 4,000 RNA features. Then RNA data was normalised using LogNormalize across features and the FindVariableFeatures function with 500 variables using variance-stabilizing transformation method (29). Antibody-derived tag (ADT) data was normalised using centred log ratio transformation across cells. To account for batch effect, Seurat’s data integration workflow was applied on RNA and ADT data separately (29). We perform weighted nearest neighbour analysis, which entails performing principal component analysis (PCA) on integrated RNA and integrated ADT datasets and using FindMultiModalNeighbors function to define and construct weighted nearest neighbour graphs (29). We used 30 principal components (PCs) from integrated RNA data and 18 PCs from integrated ADT data. Clustering was performed using SLM algorithm on shared nearest neighbour with resolution of 2.0.
Statistics
Descriptive summaries included frequency distributions and means, standard deviations or medians and ranges for categorical and continuous data, respectively. Associations between T-cell subsets and categorical patient or disease variables were analysed using Mann-Whitney U tests or Kruskall Wallis H tests as appropriate. Pearson’s correlation was used to calculate the correlation between T-cell subsets, age and mesothelin. Median OS was estimated using the Kaplan-Meier method and different groups compared via log-rank test. Cox regression analysis was performed with T-cell subset percentages as a continuous variable, as well as a dichotomous variable stratifying into ‘high’ or ‘low’ abundance according to the median value, respectively. Multivariate analyses of the Cox regression model were applied to estimate hazard ratios (HRs) and 95% confidence intervals (CIs). Regression coefficient analysis was performed to analyse the change in Tex over time. Statistical analyses were conducted with IBM SPSS Statistics version 28.0 and GraphPad Prism software version 9. All hypotheses were two-sided and P values <0.05 were considered statistically significant.
Results
NSCLC patient characteristics
Analysis was performed on a cohort of 43 NSCLC patients with histo- and/or cyto-logically confirmed malignant PE. The median age of the cohort was 73 and there were 22 females (51.2%). Most patients were former (55.8%) or current (30.2%) smokers. The majority of patients had non-squamous carcinomas (39 adenocarcinomas, one adenosquamous and one poorly differentiated) and two patients had squamous cell carcinomas. Of the 41 non-squamous carcinomas, molecular testing was performed for 39 patients and 11 (28.2%) had a KRAS mutation, seven (17.9%) had an EGFR mutation and one (2.6%) had a ROS1 fusion. Tumor PD-L1 tumor proportion score was available for 22 patients and was <1%, 1–49% and ≥50% in six (27.2%), eight (36.4%) and eight (36.4%) patients, respectively (Table 1). Twenty patients received at least one line of systemic therapy. The treatment regimens used are listed in Table 1. In the first line setting, 11 patients received platinum doublet chemotherapy with or without maintenance pemetrexed, only five patients received ICPB (four single agent and one chemoimmunotherapy) and four received an EGFR tyrosine kinase inhibitor. In subsequent line therapies, four patient received chemotherapy and five received single agent ICPB.
Table 1
Patient characteristic | Category | Data |
---|---|---|
Age (years) | 73 [47–95] | |
Sex | M | 21 (48.8) |
F | 22 (51.2) | |
Smoking | Former | 24 (55.8) |
Current | 13 (30.2) | |
Never | 6 (14.0) | |
ECOG | 0–1 | 25 (58.1) |
2–4 | 18 (41.9) | |
Charlson Comorbidities Index | 6–9 | 20 (46.5) |
10–13 | 23 (53.5) | |
Histology | Adenocarcinoma | 39 (90.7) |
Squamous | 2 (4.7) | |
Adenosquamous | 1 (2.3) | |
Poorly differentiated | 1 (2.3) | |
Mutation (non-squamous n=39)† |
KRAS | 11 (28.2) |
EGFR | 7 (17.9) | |
ROS1 | 1 (2.6) | |
Tumour PD-L1 TPS (n=22)‡ | <1% | 6 (27.2) |
1–49% | 8 (36.4) | |
≥50% | 8 (36.4) | |
Lines of therapy (n=41)§ | 0 | 21 (51.2) |
1 | 10 (24.4) | |
≥2 [2–5] | 10 (24.4) | |
Treatment regime | ||
First line | Chemotherapy | 11 (25.6) |
Single agent ICPB | 4 (9.3) | |
Chemoimmunotherapy | 1 (2.3) | |
EGFR TKI | 4 (9.3) | |
Subsequent line | Chemotherapy | 4 (9.3) |
Single agent ICPB | 5 (11.6) |
Data are presented as n (%) or median [range]. †, 2 patients not done; ‡, 21 patients not done; §, excludes patients having ongoing treatment at time of censoring. M, male; F, female; ECOG, Eastern Cooperative Oncology Group; KRAS, Kirsten rat sarcoma virus; EGFR, epidermal growth factor receptor; PD-L1, programmed death ligand 1; TPS, tumor proportion score; ICPB, immune checkpoint blockade; TKI, tyrosine kinase inhibitor.
Distinct T-cell exhaustion subsets can be identified in malignant PEs in NSCLC
In order to assess T-cell exhaustion, we focused on Texstem and Texterm cells defined here, and previously (23), as CD3+CD4−CD8+CD39−PD1midCD28+ and CD3+CD4−CD8+CD39+PD1hi T cells, respectively (Figure 1A and Figure S1). We explored the feasibility of using PEs to characterize the phenotype of exhausted T cells. To assess the short-term stability of Tex cell populations, we collected PE from four patients. Each patient underwent serial sampling, where 2–3 samples were collected independently across a period of 4–8 days. In these samples, CD4 and CD8, Texstem and Texterm cell subsets were consistent across the sampling period, indicating remarkable short-term stability of T cells within the PE (Figure 1B).
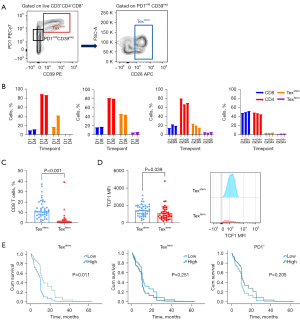
Next, as TCF1 is a key transcription factor for Texstem cells, we analyzed the TCF expression of Texstem and Texterm cells in these samples to confirm that they are representative of the stem-like and terminally exhausted populations, respectively. As expected, Texstem cells were enriched in TCF1 expression compared to Texterm in all patients (Figure S2).
We next sought to survey the Texstem and Texterm cells across the entire cohort of 43 NSCLC patients. Consistent with the short term data, Texstem cells were more prevalent than Texterm cells (mean 10.9% vs. 1.5%) (Figure 1C, Table 2). Again, in this larger cohort, TCF1 expression was significant higher in Texstem compared to Texterm (Figure 1D).
Table 2
Cell subset | NSCLC (%), median (range) |
Mesothelioma (%), median (range) |
---|---|---|
CD8a | 18.7 (3.6–69.4) | 18.7 (3.0–69.3) |
CD4a | 74.2 (24.4–95.2) | 75.5 (25.4–96.0) |
PD1+b | 25.7 (6.0–75.0) | 46.2 (8.3–84.5) |
Texstemb | 10.9 (1.6–37.1) | 14.9 (2.1–47.5) |
Textermb | 1.5 (0.3–39.2) | 3.5 (0.02–47.6) |
a, CD4 and CD8 T cells as a percentage of total CD3+ T cells; b, PD1+ and Tex cells as a percentage of CD3+CD8+CD4− T cells. NSCLC, non-small cell lung cancer; PD1, programmed death 1; Texstem, stem-like exhausted CD8 T cells; Texterm, terminally exhausted CD8 T cells.
Texstem but not Texterm T cells correlate with improved overall survival (OS)
Having determined that CD8 Texstem and Texterm cells were present and stable within PE of NSCLC patients, we next sought to determine if the prevalence of either population correlated with OS. In the NSCLC cohort, 41 of 43 patients had died by the date of census, all due to cancer progression. The median duration of follow-up was 55 months (range, 1–117 months). Univariate and multivariate analysis were performed using clinicopathological variables that can potentially influence clinical outcomes, including sex, age, smoking status, ECOG, comorbidities, number of lines of systemic therapy and ICPB treatment (Table 3). We were unable to adjust for histological subtype as there were only two patients with squamous carcinomas in our cohort. As well, we did not include PD-L1 expression levels in the multivariable analysis due to a significant number of missing data (only 22 patients had PD-L1 expression data). Multivariate analysis showed that patients with higher frequency (above median) of Texstem cells had significantly higher median OS than those with a lower frequency (below median) (median 9.9 vs. 3.4 months, HR 0.36, 95% CI: 0.16–0.79, P=0.01) (Figure 1E, Table 3). Among the non-squamous subgroup (n=41), we also adjusted for EGFR and KRAS mutation status and targeted therapy. An association between Texstem cells and OS was observed irrespective of these characteristics (Table S2, Figure S3). Higher Texterm cells was associated with worse OS in univariate but not multivariate analyses. Higher Texstem to Texterm cell ratios also predicted longer OS. Notably, PD1+ CD8 T cells as a broad population did not correlate with OS (Figure 1E, Table 3).
Table 3
Variable | Univariate | Multivariatea | |||||
---|---|---|---|---|---|---|---|
HR | 95% CI | P | HR | 95% CI | P | ||
Age (per unit decrease) | 1.01 | 0.98–1.03 | 0.62 | 0.93 | 0.89–0.98 | 0.005 | |
Sex (M vs. F) | 1.08 | 0.58–2.02 | 0.80 | 1.96 | 0.92–4.15 | 0.08 | |
Smoking (former/current vs. never) | 2.22 | 0.91–5.42 | 0.08 | 3.70 | 1.29–10.65 | 0.01 | |
ECOG (2–4 vs. 0–1) | 2.12 | 1.09–4.12 | 0.03 | 3.96 | 1.28–12.20 | 0.02 | |
Charlson Comorbidities Index (10–13 vs. 6–9) | 1.06 | 0.57–1.98 | 0.85 | 0.72 | 0.26–2.02 | 0.53 | |
Lines of therapy (vs. 0) | |||||||
1 | 0.48 | 0.22–1.06 | 0.07 | 0.64 | 0.19–2.15 | 0.47 | |
≥2 | 0.16 | 0.06–0.41 | <0.001 | 0.19 | 0.04–0.90 | 0.04 | |
ICPB treatment (yes vs. no) | 0.37 | 0.17–0.83 | 0.01 | 0.49 | 0.15–1.56 | 0.23 | |
Texstem (high vs. low) | 0.49 | 0.24–0.99 | 0.048 | 0.36 | 0.16–0.79 | 0.01 | |
Texterm (high vs. low) | 2.07 | 1.07–4.01 | 0.03 | 1.62 | 0.71–3.68 | 0.25 | |
Texstem:Texterm ratio (per unit increase) | 0.97 | 0.95–0.99 | 0.004 | 0.96 | 0.94–0.99 | 0.008 | |
PD1+ (high vs. low) | 0.66 | 0.33–1.29 | 0.22 | 0.62 | 0.29–1.30 | 0.20 |
a, multivariate cox regression analysis adjusted for age, sex, smoking, ECOG, Charlson Comorbidities Index, number of lines of systemic therapy and ICPB use. HR, hazard ratio; CI, confidence interval; M, male; F, female; ECOG, Eastern Cooperative Oncology Group; ICPB, immune checkpoint blockade; Texstem, stem-like exhausted CD8 T cells; Texterm, terminally exhausted CD8 T cells; PD1, programmed death 1.
The prognostic significance of Texstem cells is validated in mesothelioma
To validate our findings from NSCLC, we examined Texstem and Texterm cell frequencies in PE from a cohort of 49 mesothelioma patients (Table 4). The median age of the cohort was 70 and the majority (91.8%) were males. Nineteen (44.2%) and two (4.6%) patients were former and current smokers, respectively. Forty-one (83.6%) cases were mesothelioma of the epithelioid subtype and four (8.2%) cases were of the sarcomatoid subtype. Twenty-nine patients received at least one line of systemic therapy. In the first line setting, 21 patients received platinum doublet chemotherapy, five patients received chemoimmunotherapy and three patients received doublet ICPB with ipilimumab and nivolumab. Subsequent line therapies included chemotherapy (n=17), chemoimmunotherapy (n=1), doublet ICPB (n=3), single agent ICPB (n=4), FGFR inhibitor (n=2) and anti-CD80 antibody (n=1) (Table 4).
Table 4
Patient characteristic | Data |
---|---|
Age (years) | 70 [46–90] |
Sex | |
M | 45 (91.8) |
F | 4 (8.2) |
Smoking (n=43, 6 patients unknown) | |
Former | 19 (44.2) |
Current | 2 (4.6) |
Never | 22 (51.2) |
ECOG | |
0–1 | 39 (79.6) |
2–4 | 10 (20.4) |
Charlson Comorbidities Index | |
6–9 | 35 (71.4) |
10–13 | 14 (28.6) |
Histology | |
Epithelioid | 41 (83.6) |
Sarcomatoid | 4 (8.2) |
Biphasic | 4 (8.2) |
Lines of therapy (n=41)* | |
0 | 20 (48.8) |
1 | 5 (12.2) |
≥2 [2–5] | 16 (39.0) |
Treatment regimen | |
First line | |
Chemotherapy | 21a (42.8) |
Chemoimmunotherapy | 5 (10.2) |
Doublet ICPB | 3 (6.1) |
Subsequent line | |
Chemotherapy | 17 (34.7) |
Chemoimmunotherapy | 1 (2.0) |
Doublet ICPB | 3b (6.1) |
Single agent ICPB | 4c (8.2) |
FGFR inhibitor | 2 (4.1) |
Anti-CD80 antibody | 1 (2.0) |
Data are presented as n (%) or median [range]. a, two patients received chemotherapy +/- vascular endothelial growth factor inhibitor, one patient received chemotherapy plus pegylated arginine deiminase; b, one patient received doublet immunotherapy plus UV1 vaccine; c, one patient received single agent immunotherapy plus mesothelin antibody; *, excludes patients having ongoing treatment at time of censoring. M, male; F, female; ECOG, Eastern Cooperative Oncology Group; ICPB, immune checkpoint blockade; FGFR, fibroblast growth factor receptor.
A representative flow cytometry plot for mesothelioma is presented in Figure 2A. As seen in NSCLC, the percentage of Texstem cell was higher than Texterm cells in mesothelioma (mean 14.9% vs. 3.5%) (Figure 2B). The absolute percentages of Texstem and Texterm cells was greater in mesothelioma compared to NSCLC, however the proportion of Texstem and Texterm relative to total CD8 T cells were similar across both cancers (Table 2). Mesothelioma Texstem cells also had higher TCF1 MFI compared to Texterm cells (Figure 2C).
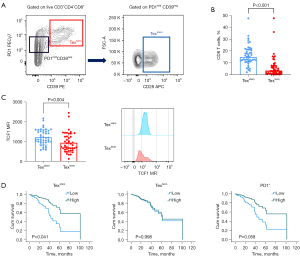
We next sought to determine if high Texstem cell proportion was also predictive of increased OS. From these 49 mesothelioma patients, 40 had died from cancer progression by the date of census and the median duration of follow-up was 51 months (6–122 months). In mesothelioma, as in NSCLC, increased Texstem cells was an independent predictor of improved OS irrespective of age, sex, histological subtype, smoking history, ECOG, number of lines of systemic therapy and ICPB treatment. Patients with a high abundance of Texstem cells had significantly longer OS compared to those with low abundance (median 32.1 vs. 19.8 months, HR 0.31, 95% CI: 0.10–0.96, P=0.04) (Figure 2D, Table 5). Higher Texstem to Texterm cell ratio also predicted longer OS. Increased Texterm cells was not associated with prognosis in univariate or multivariate analyses (Figure 2D, Table 5). As seen in NSCLC, PD1+ CD8 T cells as a broad population did not correlate with OS (Figure 2D, Table 5).
Table 5
Variable | Univariate | Multivariatea | |||||
---|---|---|---|---|---|---|---|
HR | 95% CI | P | HR | 95% CI | P | ||
Age (per unit decrease) | 1.04 | 1.01–1.08 | 0.02 | 0.99 | 0.92–1.07 | 0.86 | |
Sex (M vs. F) | 0.60 | 0.18–1.99 | 0.40 | 0.79 | 0.12–5.05 | 0.81 | |
Smoking (former/current vs. never) | 1.26 | 0.46–3.42 | 0.65 | 0.55 | 0.19–1.55 | 0.25 | |
ECOG (2–4 vs. 0–1) | 2.18 | 0.95–5.01 | 0.07 | 1.41 | 0.50–3.93 | 0.51 | |
Charlson Comorbidities Index (10–13 vs. 6–9) | 3.18 | 1.51–6.71 | 0.002 | 2.57 | 0.66–10.11 | 0.17 | |
Lines of therapy (vs. 0) | |||||||
1 | 1.11 | 0.40–3.04 | 0.84 | 1.23 | 0.33–4.60 | 0.76 | |
≥2 | 0.72 | 0.36–1.43 | 0.35 | 1.09 | 0.40–2.94 | 0.86 | |
Histology | |||||||
Epithelioid | |||||||
Sarcomatoid | 1.90 | 0.57–6.35 | 0.30 | 5.76 | 1.02–32.59 | 0.048 | |
Biphasic | 1.90 | 0.57–6.35 | 0.30 | 5.76 | 1.02–32.59 | 0.048 | |
ICPB treatment (yes vs. no) | 0.46 | 0.21–1.01 | 0.053 | 0.38 | 0.11–1.34 | 0.13 | |
Texstem (high vs. low) | 0.56 | 0.28–1.13 | 0.11 | 0.31 | 0.10–0.96 | 0.04 | |
Texterm (high vs. low) | 0.86 | 0.45–1.63 | 0.64 | 0.99 | 0.44–2.24 | >0.99 | |
Texstem:Texterm ratio (per unit increase) | 1.00 | 0.99–1.01 | 0.56 | 0.98 | 0.97–1.00 | 0.01 | |
PD1+ (high vs. low) | 0.46 | 0.24–0.87 | 0.02 | 0.38 | 0.14–1.03 | 0.06 |
a, multivariate cox regression analysis adjusted for age, sex, smoking, ECOG, Charlson Comorbidities Index, number of lines of systemic therapy, histology and ICPB treatment. HR, hazard ratio; CI, confidence interval; M, male; F, female; ECOG, Eastern Cooperative Oncology Group; ICPB, immune checkpoint blockade; Texstem, stem-like exhausted CD8 T cells; Texterm, terminally exhausted CD8 T cells; PD1, programmed death 1.
Correlation of exhausted CD8 T-cell subsets with clinical and pathological characteristics
The heterogeneous Tex cell landscape observed between patients indicates that there may be clinical or biological factors that influence the progression to terminal T-cell exhaustion. To explore the potential factors that may affect this pathway, we next asked whether the abundance of Tex cell subsets correlated with clinicopathological features.
In NSCLC, higher Texstem was observed in never smokers compared to former or current smokers (mean 20.2% vs. 11.9%, P=0.02) (Figure 3A). No difference in Texterm was observed between the two groups. Smoking pack years data were available for 28 of the 37 current or former smokers, but no correlation was observed between the number of pack years and Texstem cells. Increased Texterm cells were observed in EGFR wild type non-squamous carcinomas compared to EGFR mutant tumors (P=0.04) (Figure 3B). There was no difference in Texstem when stratified by EGFR mutation status. No associations were found between any of the Tex subsets and patient age, sex, or KRAS mutation status. In our cohort, there were only two patients with squamous cell carcinomas so we could not evaluate the correlation between Tex cell subsets with histological subtype. Furthermore, as tumor PD-L1 expression results were only available in 22 patients, we did not examine the correlation between PD-L1 expression and Tex subsets.
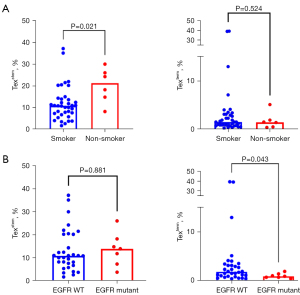
In mesothelioma, there were no associations between Tex cell subsets and patient age, sex, histological subtype. Smoking history was available for 43 patients. There was no association between smoking history and Tex cell subsets.
To investigate whether the degree of T-cell exhaustion correlated with tumor burden, we analysed pre-treatment serum mesothelin levels of 26 patients with epithelioid mesothelioma, as a surrogate marker for tumor burden (30). There was a statistically significant correlation between Texterm cells and serum mesothelin concentration (Pearson’s r=0.525, P=0.006), but this result was mainly driven by a single patient with the highest proportion of Texterm cells and mesothelin levels (data not shown). There was no correlation between mesothelin levels and Texstem cells.
No significant changes in Tex subset frequency in PEs during disease progression
In a smaller cohort of treatment-naïve patients we had access to samples over a 25-month period allowing us to perform an exploratory longitudinal analysis of T-cell exhaustion in the PE in the setting of cancer progression in the absence of any systemic therapy. Samples were collected at diagnosis and at a minimum of 1-month intervals, from eight patients with mesothelioma and two patients with NSCLC. Patient characteristics are described in Table S3. Two to six samples were available from each patient ranging from 1 to 22 months post diagnosis (Figure S4A). The median follow-up was 73.5 months (range, 31–110 months). At the time of data analysis, both NSCLC patients and six mesothelioma patients have died, with survival of 17 and 25 months for the two NSCLC patients and ranging from 18 to 102 months for those with mesothelioma.
Statistical power was limited in this small cohort, but a regression coefficient analysis showed that overall, there was no significant change in the frequencies of Texstem cells (Cohens d −0.49, P=0.16), Texterm cells (Cohens d 0.18, P=0.58) and the Texstem:Texterm cell ratio (Cohens d −0.31, P=0.35) over time (Figure S4B).
Texstem and Texterm cells display distinct phenotypes and intrinsic heterogeneity
To gain a comprehensive understanding of the immunological features of malignant PE, we performed high dimensional mass cytometry (a.k.a., cytometry by time-of-flight; CyTOF) profiling using 30 cellular markers dedicated to T cells of PE samples. We analysed a total of 16 PE samples from eight patients (five mesothelioma and three lung adenocarcinoma), collected at two time points per patient ranging from 1 to 8 months apart. Consistent with previous reports (31,32), our mass cytometry profiling revealed that malignant PE were enriched in T cells; predominantly CD4 T cells (Figure S5A,S5B). The distributions of key immune cell subsets in NSCLC and mesothelioma are presented in Figure S5C. Since we observed conserved immune cell distribution and T cell phenotypes across timepoints, only data from the initial time point was further presented (Figure 4).
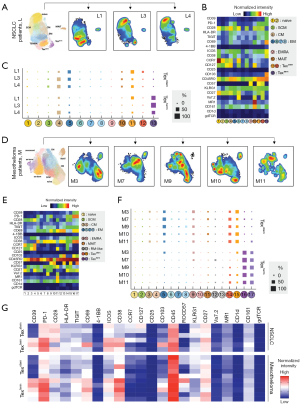
We then examined the detailed profiles of CD8 T cell populations present in malignant PE from NSCLC patients. To accomplish this, we manually gated CD8 T cells and employed Uniform Manifold Approximation and Projection (UMAP) dimensionality reduction, followed by clustering with the Louvain graph-based clustering algorithm (see Methods). This method allowed us to identify and resolve various cell types, including mucosal-associated invariant T (MAIT, MR-1+CD161+Va7.2+), exhausted (PD1+CD39+), and resident memory-like cells (CD103+) (Figure 4A,4B and Figure S6A).
Next, we examined the cellular phenotypes of the different Tex states by manually gating on Texstem (PD1midCD39–CD28+) and Texterm (PD1+CD39+) (Figure S6B). Texstem cells from NSCLC patients displayed a range of phenotypes, including: canonical Texstem (cluster 11, approximately 32.5%), central memory (CM)-like (cluster 4, approximately 23.8%), effector-memory (EM)-like (cluster 5, approximately 21.9%), MAIT-like (cluster 10, approximately 5.0%) and Va7.2+ Texstem (cluster 12, approximately 3.3%). In contrast, the majority of Texterm cells clustered into a single group (cluster 13, approximately 76.8%) and exhibited greater expression of immune inhibitory molecules including TIGIT and ICOS. Notably, the Texterm cell population, characterised by high levels of CD39, also co-expressed tissue resident markers CD103 and CD69 (33-35). Of note, a small fraction of Texstem and Texterm cells were MAIT cells (cluster 10) (Figure 4B,4C and Figure S6C), indicating that both conventional T cells and MAIT cells can exhibit markers of exhaustion, as previously shown in colorectal cancer and in lung cancer tumor infiltrates (36,37).
A similar analysis of malignant PE samples obtained from mesothelioma patients showed comparable findings, reinforcing the consistency of our findings (Figure S7). Texstem cells were associated with CD57+ Texstem (cluster 15, approximately 27.6%), CD57– Texstem (cluster 14, approximately 20.8%), EM-like (cluster 5, approximately 20.2%) and MAIT-like (cluster 11, approximately 3.8%). Meanwhile, Texterm cells exhibited greater expression of activation/exhaustion markers (e.g., CD38, HLA-DR, TIGIT and ICOS) and tissue resident markers (e.g., CD69 and CD103). Specifically, these Texterm cells were identified as CD57– Texterm (cluster 16, approximately 61.1%) and CD57+ Texterm (cluster 17, approximately 47.9%) (Figure 4D-4F). These consistent patterns indicate distinct phenotypic characteristics of Texstem and Texterm cells.
Interestingly, the phenotypic features of Tex cells varied between NSCLC and mesothelioma. Compared to their mesothelioma counterparts, NSCLC Texstem and Texterm cells displayed a more stem-like phenotype with enrichment of CCR7 chemokine receptor and CD127 (IL-7 receptor) (38), as well as displayed lower expression of the immune inhibitory/senescence marker CD57 (Figure 4G). Longitudinal profiling across the two time points showed that these phenotypic differences persisted consistently over an extended period (Figure S8).
Single cell multi-omics reveals phenotypic and clonotypic heterogeneity of Texstem and Texterm cells
To gain a more comprehensive understanding of phenotypic and clonotypic variation within Tex cells, we performed 10x Genomics Chromium single-cell multi-omics analysis on four patients (two mesothelioma and two NSCLC). By incorporating mRNA sequencing analysis, 50 oligo-tagged (CITE-seq) surface antibodies (39) and TCR sequences, this multimodal analysis facilitated the characterization of T-cell subsets based on gene expression, surface protein markers, and TCR diversity. We performed a weighted nearest neighbour analysis with UMAP to visualise the high-dimensional multi-omics (protein and gene expression) profiles in a lower-dimension embedding (Figure 5A,5B and Figure S9A-S9C) (29).
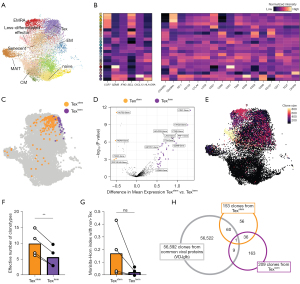
Expanding upon our findings from the CyTOF analysis, CD8 T cells infiltrating malignant PE were heterogeneous and Texstem and Texterm cells exhibited differential expression of proteins and genes (Figure 5C and Figure S9D,S9E). Differential expression analysis of Texterm cells in comparison to Texstem cells showed enrichment of genes and proteins related to immune inhibitory receptors (LAG3, ENTPD1, PDCD1, HAVCR2, TIGIT), and cytotoxicity (NKG7) but lower in pluripotent stemness (KLRG1, RORA, IL7R), indicating Texstem cells and Texterm cells are transcriptionally distinct (Figure 5D).
Given that Texstem cells are thought to act as progenitors for Texterm cells (40) and those cells are chronically exposed and proximal to the tumor site, we hypothesise that both Texstem cells and Texterm cells are enriched for cognate tumor-reactive TCRs. To test this hypothesis, we first analysed diversity of TCR clonotypic repertoires of Texstem and Texterm cells. In line with previous findings (23), Texstem cells exhibited more diverse TCR repertoires, as indicated by the clone size and effective number of clonotypes, whereas Texterm cells showed comparatively less TCR diversity (Figure 5E,5F). To understand the developmental relatedness between Texstem and Texterm cell states, we examined the extent of overlap in TCR repertoires between these cell populations using the Morisita-Horn index. Interestingly, though not reaching statistical significance, Texstem exhibited a higher degree of overlap with non-Tex cells compared to Texterm (Figure 5G). There was minimal overlap of TCR clonotypes between Texstem and Texterm cells, suggesting that only a small fraction of Tex cells transition between the Texstem and Texterm phenotypes (Figure S9F).
To extend these findings, we investigated whether TCR sequences from Tex cells exhibited any overlap with TCRs of known cancer-unrelated specificities (e.g., Epstein-Barr virus-, cytomegalovirus-, or influenza virus-specific T cells) in the VDJ database (41). Out of the 153 clones identified from Texstem, 61 TCRs were found within the database. In contrast, out of the 209 clones from Texterm, only 10 clones were detected in the database (Figure 5H). These results indicate that Texstem in the TME partially consist of cancer-unrelated antigen-specific bystander T cells, while this phenomenon is not observed to the same extent in the Texterm cell population.
Discussion
In this study, we demonstrate that an increase in the proportion of Texstem cells in PE is associated with improved OS and may present a useful prognostic biomarker. Importantly, our findings were demonstrated in two distinct tumor types, supporting the importance of Texstem cells in anti-tumor immunity irrespective of cancer type. In addition, we used mass cytometry and single-cell multi-omics data to explore the phenotype of T cells within the PE of NSCLC and mesothelioma patients in finer detail.
CD8 TILs have been associated with clinical outcomes in several cancers (5,42,43). However, there are limited studies that address the functional heterogeneity of the Tex cell population. In this study, higher frequency of PE Texstem CD8 T cells were independently associated with a favourable prognosis in both NSCLC and mesothelioma. The Texstem cell population was enriched for TCF1 expression compared to Texterm cell population, as well as compared to the non-naive PD1midCD39-CD28- T cell population (data not shown). These results are consistent with previous reports of transcriptomic analysis of TCGA lung adenocarcinoma and melanoma cohorts, where increased CD8 TIL expression of TCF7 (encoding TCF1) in combination with PDCD1 (encoding PD1) was associated with improved clinical outcomes (21,44). PD1 expression on TILs has been associated with poor patient survival across a number of different cancer types (45-50). Notably, in our study there was no significant association between PD1+ CD8 T cells and OS, demonstrating that the prognostic relevance was specifically associated with the Texstem cell subset. Our results are in agreement with studies in other cancer types (14, 18, 19) that demonstrate that Texstem can be associated with improved outcomes. Texstem, with lower inhibitory receptor expressions, are less differentiated and retain self-renewal capacity and effector function. As a result, the observed improved prognosis associated with an increased frequency of Texstem cells is likely be attributed to their ability to generate more effective anti-cancer immune response. These results support the clinical significance of this cell subset and highlight their role as a crucial component of the anti-tumor immune response.
On the contrary, the frequency of Texterm cells did not impact OS, highlighting Texstem and Texterm as unique Tex cell populations with distinct prognostic relevance. Texterm are known to lose their expansion capacity and are functionally less efficient (44). Therefore, Texterm cells may not have prognostic implications because they are functionally impaired and continually deleted from the TME. An additional finding from our study was that in NSCLC, an increased proportion of Texterm cells was observed in EGFR wild type adenocarcinoma compared to EGFR mutant tumors and an increased proportion of Texstem cells was observed in never smokers compared to current or former smokers. This suggests that lower levels of terminal exhaustion and the stem-like exhaustion state of CD8 T cells may be related to the lower tumor mutational burden associated with EGFR mutant and non-smoking related lung cancer (51,52). We did not have genomic sequencing data for our patient cohort and further studies are currently being undertaken to delineate this relationship.
Our longitudinal data demonstrate stability of the frequency of Tex subsets in PE over time, supporting the utility of PE as a sample site for biomarker studies. Our longer term longitudinal exploratory analysis showed no statistically significant difference in Tex frequencies over time but given the small sample size, no conclusions can be drawn from this analysis and further study in a larger population is required.
Within the individual subsets of Texstem and Texterm there is evidence of considerable heterogeneity in phenotype; and this is seen across cancer types and in chronic infection models (53,54). Here in a subset of patients we were able to use multi-dimensional CyTOF and single cell sequencing to interogate the phenotype of cells within the PE in fine detail. We noted considerable heterogeneity within the Texstem and Texterm populations and across cancer types. Texstem and Texterm cells from patients with NSCLC had greater expression of CCR7 and CD127, and lower expression of CD57 compared to those from patients with mesothelioma. A low frequency of circulating CCR7+ CD8 T cells is a significant risk factor for tumor recurrence in patients with head and neck cancer (55). CD127, also known as the IL-7α chain, forms part of the IL-7 receptor complex. IL-7 signalling promotes human CD8 T cell generation and cytolytic reactivity (38). Our findings suggest that exhausted T cells in NSCLC patients may have improved functionality compared to those present within mesothelioma patients. These differences may contribute to, or be indicative of the level of immune reactivity in the TME and the responsiveness to immunotherapy of these two cancer types.
High expression of CD39 and CD103 have been suggested as markers for tumor-specific T cells (34,56) within tumors. Previous studies have implicated that those markers are useful in isolating tumor-specific cells in malignant PE as well (33,35). These results suggest that tumor antigen specific CD8 T cells have a Texterm phenotype. Surprisingly, in spite of the likelihood that Texterm cells are tumor specific, increased Texterm cells were not associated with improved clinical outcomes, perhaps due to their hypo-functionality. Our results revealed that a significant proportion of Texstem cell population is composed of cancer-unrelated, virus-specific, bystander T cells. The role of bystander T cells in anti-tumor immunity is not yet well understood. However, evidence from viral infection models suggest that T cells lacking disease relevant specificities can also participate in the immune response, driven by proinflammatory cytokines in the immune environment (57-60). These T cells can adopt effector functions, for example through the production of interferon γ (IFNγ) and GZMB, without the need for antigen recognition (61). The abundance of bystander T cells in the TME may also be a proxy for the degree of immune reactivity and T cell recruitment. Lastly, it has been proposed that some bystander T cells also recognise tumor antigens through TCR cross reactivity (62-64), and hence an increased abundance of bystander T cells may lead to direct anti-tumor immunity. Our results however do not exclude the possibility that tumor-specific T cells are also present in the Texstem cell population. Our group and others have previously identified T cells directed against tumor neoantigens in PE by testing for IFNγ T-cell responses against bioinformatically predicted neoantigens, using methods such as IFNγ ELISpot assays (65,66) and neoantigen peptide-MHC tetramer staining (unpublished data). Further work is underway to delineate the exhaustion phenotype of these neoantigen-specific T cells.In advanced thoracic malignancies, patients often develop PE during the course of their disease (22,67). PE provides a valuable sample source for translational research because it is proximal to tumor cells and is relatively easy to access compared to tumor biopsies. In addition, some patients require routine drainage of PE which allow longitudinal analysis that could reveal dynamic changes without the need for serial biopsies. Our study provides a proof of concept that PE can be used for immune analysis and that the data generate can have clinical relevance. In addition, PE is an unique immune microenvironment and its immunological characteristics do not necessarily mirror those of tumor tissue or peripheral blood, allowing greater insight into the complex landscape of the anti-tumor immune response. For example, the expression of inhibitory molecules such as PD1, TIM3 and LAG3 are greater on PE T cells compared to peripheral blood, but lower compared to TILs (31,68-74). These data suggest that PE should not at this stage be relied upon as a surrogate for tumor or peripheral blood T-cell analysis but rather as an adjunct for studies into anti-tumor immunity.
Our study has several limitations. Our NSCLC cohort comprised almost entirely of non-squamous cell carcinomas so the results cannot be generalised to the squamous cell carcinoma subtype, in which precursor exhausted T cells have previously been reported to have no effect on prognosis (21). In addition, the retrospective nature of our study predisposes it to potential biases. However, we validated our findings in a separate patient cohort, strengthening the validity of our results. Our multi-dimensional studies, in particular, the single cell sequencing studies were performed on a limited subset of patients. However, these studies are hypothesis generating and allow more focused studies in the future to determine, for example, which subset of Texstem cells are most protective in NSCLC and mesothelioma.
Conclusions
Overall, in this study, we demonstrated that increased abundance of Texstem CD8 T cells is associated with significantly improved prognosis in NSCLC and mesothelioma. We also further characterised the T-cell exhaustion landscape in malignant PE of NSCLC and mesothelioma patients using mass cytometry and single cell RNA sequencing. Together, these findings suggest that Texstem cells are a key population for cancer control and regression.
Acknowledgments
We thank all other members of the Newell lab for their feedback and support for this project. We acknowledge the support of patients and the National Centre for Asbestos Related Diseases Biobank for providing the pleural effusion samples.
Funding: This work was supported by
Footnote
Reporting Checklist: The authors have completed the STROBE reporting checklist. Available at https://tlcr.amegroups.com/article/view/10.21037/tlcr-24-284/rc
Data Sharing Statement: Available at https://tlcr.amegroups.com/article/view/10.21037/tlcr-24-284/dss
Peer Review File: Available at https://tlcr.amegroups.com/article/view/10.21037/tlcr-24-284/prf
Conflicts of Interest: All authors have completed the ICMJE uniform disclosure form (available at https://tlcr.amegroups.com/article/view/10.21037/tlcr-24-284/coif). H.R. reports grants from IIRC post-doctoral fellowship (No. 224990-99, to H.R.) and post-doctoral training fellowship from the National Research Foundation of Korea (NRF-2020R1A6A3A03037852, to H.R.). T.F. reports serving as the board secretary of Mind the Change Inc. Y.C.G.L. is the current NHMRC Leadership Fellow and a recipient of the Investigator Grant (Leadership 2) (since July 2023), and the recipient of the Next Generation Clinical Research Practitioner Fellowship (from 2018 to June 2023). Rocket Med (UK) has provided free pleural fluid drainage kits for patients enrolled in randomized trials that Y.C.G.L. led. B.W.R. reports funding from the NHMRC of Australia, Insurance Commission of Western Australia, The US Department of Defense, Western Australian Department of Health, iCARE Foundation, Sir Charles Gairdner Research Advisory Committee, the Future Health Research and Innovation Fund scheme through the Biobank Interim Support Program 2021 and the Cancer Council of Western Australia. J.C. reports funding from the Australian National Health and Medical Research Council; The US Department of Defense; Insurance Commission of Western Australia; Western Australian Department of Health; iCARE Foundation; Sir Charles Gairdner Research Advisory Committee; Future Health Research and Innovation Fund scheme through the Biobank Interim Support Program 2021; Cancer Council of Western Australia. E.W.N. reports funding from the National Institutes of Health (NIH, Nos. R01CA264646 and R01AI176563 to E.W.N.), US Department of Defense, and Fred Hutchinson Cancer Center. And a cofounder, advisor and shareholder of ImmunoScape; an advisor and shareholder of Neogene Therapeutics; an advisor for NanoString Technologies and InduPro, and a shareholder in Trojan Bio. A.J.R. reports funding from The Australian National Health and Medical Research Council and The US Department of Defense. The other authors have no conflicts of interest to declare.
Ethical Statement: The authors are accountable for all aspects of the work in ensuring that questions related to the accuracy or integrity of any part of the work are appropriately investigated and resolved.
Open Access Statement: This is an Open Access article distributed in accordance with the Creative Commons Attribution-NonCommercial-NoDerivs 4.0 International License (CC BY-NC-ND 4.0), which permits the non-commercial replication and distribution of the article with the strict proviso that no changes or edits are made and the original work is properly cited (including links to both the formal publication through the relevant DOI and the license). See: https://creativecommons.org/licenses/by-nc-nd/4.0/.
References
- Schumacher TN, Hacohen N. Neoantigens encoded in the cancer genome. Curr Opin Immunol 2016;41:98-103. [Crossref] [PubMed]
- Koletsa T, Kotoula V, Koliou GA, et al. Prognostic impact of stromal and intratumoral CD3, CD8 and FOXP3 in adjuvantly treated breast cancer: do they add information over stromal tumor-infiltrating lymphocyte density? Cancer Immunol Immunother 2020;69:1549-64. [Crossref] [PubMed]
- Kahn RM, Matrai C, Quinn AS, et al. CD3+ and CD8+ tumor-infiltrating lymphocytes (TILs) as markers of improved prognosis in high-grade serous ovarian cancer. Gynecol Oncol 2019;154:68.
- Tokito T, Azuma K, Kawahara A, et al. Predictive relevance of PD-L1 expression combined with CD8+ TIL density in stage III non-small cell lung cancer patients receiving concurrent chemoradiotherapy. Eur J Cancer 2016;55:7-14. [Crossref] [PubMed]
- Borsetto D, Tomasoni M, Payne K, et al. Prognostic Significance of CD4+ and CD8+ Tumor-Infiltrating Lymphocytes in Head and Neck Squamous Cell Carcinoma: A Meta-Analysis. Cancers (Basel) 2021;13:781. [Crossref] [PubMed]
- Baitsch L, Baumgaertner P, Devêvre E, et al. Exhaustion of tumor-specific CD8+ T cells in metastases from melanoma patients. J Clin Invest 2011;121:2350-60. [Crossref] [PubMed]
- Thommen DS, Koelzer VH, Herzig P, et al. A transcriptionally and functionally distinct PD-1(+) CD8(+) T cell pool with predictive potential in non-small-cell lung cancer treated with PD-1 blockade. Nat Med 2018;24:994-1004. [Crossref] [PubMed]
- Wherry EJ. T cell exhaustion. Nat Immunol 2011;12:492-9. [Crossref] [PubMed]
- Ahmadzadeh M, Johnson LA, Heemskerk B, et al. Tumor antigen-specific CD8 T cells infiltrating the tumor express high levels of PD-1 and are functionally impaired. Blood 2009;114:1537-44. [Crossref] [PubMed]
- Zarour HM. Reversing T-cell Dysfunction and Exhaustion in Cancer. Clin Cancer Res 2016;22:1856-64. [Crossref] [PubMed]
- Blackburn SD, Shin H, Haining WN, et al. Coregulation of CD8+ T cell exhaustion by multiple inhibitory receptors during chronic viral infection. Nat Immunol 2009;10:29-37. [Crossref] [PubMed]
- Im SJ, Hashimoto M, Gerner MY, et al. Defining CD8+ T cells that provide the proliferative burst after PD-1 therapy. Nature 2016;537:417-21. [Crossref] [PubMed]
- Paley MA, Kroy DC, Odorizzi PM, et al. Progenitor and terminal subsets of CD8+ T cells cooperate to contain chronic viral infection. Science 2012;338:1220-5. [Crossref] [PubMed]
- Miller BC, Sen DR, Al Abosy R, et al. Subsets of exhausted CD8(+) T cells differentially mediate tumor control and respond to checkpoint blockade. Nat Immunol 2019;20:326-36. [Crossref] [PubMed]
- Blank CU, Haining WN, Held W, et al. Defining 'T cell exhaustion'. Nat Rev Immunol 2019;19:665-74. [Crossref] [PubMed]
- Peng Y, Xiao L, Rong H, et al. Single-cell profiling of tumor-infiltrating TCF1/TCF7(+) T cells reveals a T lymphocyte subset associated with tertiary lymphoid structures/organs and a superior prognosis in oral cancer. Oral Oncol 2021;119:105348. [Crossref] [PubMed]
- Wang D, Fang J, Wen S, et al. A comprehensive profile of TCF1(+) progenitor and TCF1(-) terminally exhausted PD-1(+)CD8(+) T cells in head and neck squamous cell carcinoma: implications for prognosis and immunotherapy. Int J Oral Sci 2022;14:8. [Crossref] [PubMed]
- Koh J, Kim S, Woo YD, et al. TCF1(+)PD-1(+) tumour-infiltrating lymphocytes predict a favorable response and prolonged survival after immune checkpoint inhibitor therapy for non-small-cell lung cancer. Eur J Cancer 2022;174:10-20. [Crossref] [PubMed]
- Ma L, Sun L, Zhao K, et al. The prognostic value of TCF1+CD8+T in primary small cell carcinoma of the esophagus. Cancer Sci 2021;112:4968-76. [Crossref] [PubMed]
- Chakravarti M, Dhar S, Bera S, et al. Terminally Exhausted CD8+ T Cells Resistant to PD-1 Blockade Promote Generation and Maintenance of Aggressive Cancer Stem Cells. Cancer Res 2023;83:1815-33. [Crossref] [PubMed]
- Guo X, Zhang Y, Zheng L, et al. Global characterization of T cells in non-small-cell lung cancer by single-cell sequencing. Nat Med 2018;24:978-85. [Crossref] [PubMed]
- Morgensztern D, Waqar S, Subramanian J, et al. Prognostic impact of malignant pleural effusion at presentation in patients with metastatic non-small-cell lung cancer. J Thorac Oncol 2012;7:1485-9. [Crossref] [PubMed]
- Jansen CS, Prokhnevska N, Master VA, et al. An intra-tumoral niche maintains and differentiates stem-like CD8 T cells. Nature 2019;576:465-70. [Crossref] [PubMed]
- Philip M, Schietinger A. CD8(+) T cell differentiation and dysfunction in cancer. Nat Rev Immunol 2022;22:209-23. [Crossref] [PubMed]
- Sade-Feldman M, Yizhak K, Bjorgaard SL, et al. Defining T Cell States Associated with Response to Checkpoint Immunotherapy in Melanoma. Cell 2018;175:998-1013.e20. [Crossref] [PubMed]
- Gupta PK, Godec J, Wolski D, et al. CD39 Expression Identifies Terminally Exhausted CD8+ T Cells. PLoS Pathog 2015;11:e1005177. [Crossref] [PubMed]
- Canale FP, Ramello MC, Núñez N, et al. CD39 Expression Defines Cell Exhaustion in Tumor-Infiltrating CD8(+) T Cells. Cancer Res 2018;78:115-28. [Crossref] [PubMed]
- Kim KH, Kim HK, Kim HD, et al. PD-1 blockade-unresponsive human tumor-infiltrating CD8(+) T cells are marked by loss of CD28 expression and rescued by IL-15. Cell Mol Immunol 2021;18:385-97. [Crossref] [PubMed]
- Hao Y, Hao S, Andersen-Nissen E, et al. Integrated analysis of multimodal single-cell data. Cell 2021;184:3573-3587.e29. [Crossref] [PubMed]
- Creaney J, Francis RJ, Dick IM, et al. Serum soluble mesothelin concentrations in malignant pleural mesothelioma: relationship to tumor volume, clinical stage and changes in tumor burden. Clin Cancer Res 2011;17:1181-9. [Crossref] [PubMed]
- Principe N, Kidman J, Lake RA, et al. Malignant Pleural Effusions-A Window Into Local Anti-Tumor T Cell Immunity? Front Oncol 2021;11:672747. [Crossref] [PubMed]
- Huang ZY, Shao MM, Zhang JC, et al. Single-cell analysis of diverse immune phenotypes in malignant pleural effusion. Nat Commun 2021;12:6690. [Crossref] [PubMed]
- Dhupar R, Okusanya OT, Eisenberg SH, et al. Characteristics of Malignant Pleural Effusion Resident CD8(+) T Cells from a Heterogeneous Collection of Tumors. Int J Mol Sci 2020;21:6178. [Crossref] [PubMed]
- Duhen T, Duhen R, Montler R, et al. Co-expression of CD39 and CD103 identifies tumor-reactive CD8 T cells in human solid tumors. Nat Commun 2018;9:2724. [Crossref] [PubMed]
- Zhang Y, Li W, Zhai J, et al. Phenotypic and functional characterizations of CD8(+) T cell populations in malignant pleural effusion. Exp Cell Res 2022;417:113212. [Crossref] [PubMed]
- Rodin W, Sundström P, Ahlmanner F, et al. Exhaustion in tumor-infiltrating Mucosal-Associated Invariant T (MAIT) cells from colon cancer patients. Cancer Immunol Immunother 2021;70:3461-75. [Crossref] [PubMed]
- Li S, Simoni Y, Becht E, et al. Human Tumor-Infiltrating MAIT Cells Display Hallmarks of Bacterial Antigen Recognition in Colorectal Cancer. Cell Rep Med 2020;1:100039. [Crossref] [PubMed]
- van der Leun AM, Thommen DS, Schumacher TN. CD8(+) T cell states in human cancer: insights from single-cell analysis. Nat Rev Cancer 2020;20:218-32. [Crossref] [PubMed]
- Stoeckius M, Hafemeister C, Stephenson W, et al. Simultaneous epitope and transcriptome measurement in single cells. Nat Methods 2017;14:865-68. [Crossref] [PubMed]
- Chen Z, Ji Z, Ngiow SF, et al. TCF-1-Centered Transcriptional Network Drives an Effector versus Exhausted CD8 T Cell-Fate Decision. Immunity 2019;51:840-855.e5. [Crossref] [PubMed]
- Shugay M, Bagaev DV, Zvyagin IV, et al. VDJdb: a curated database of T-cell receptor sequences with known antigen specificity. Nucleic Acids Res 2018;46:D419-27. [Crossref] [PubMed]
- Schalper KA, Brown J, Carvajal-Hausdorf D, et al. Objective measurement and clinical significance of TILs in non-small cell lung cancer. J Natl Cancer Inst 2015;107:dju435. [Crossref] [PubMed]
- Palomero J, Panisello C, Lozano-Rabella M, et al. Biomarkers of tumor-reactive CD4(+) and CD8(+) TILs associate with improved prognosis in endometrial cancer. J Immunother Cancer 2022;10:e005443. [Crossref] [PubMed]
- Siddiqui I, Schaeuble K, Chennupati V, et al. Intratumoral Tcf1(+)PD-1(+)CD8(+) T Cells with Stem-like Properties Promote Tumor Control in Response to Vaccination and Checkpoint Blockade Immunotherapy. Immunity 2019;50:195-211.e10. [Crossref] [PubMed]
- Mansfield AS, Roden AC, Peikert T, et al. B7-H1 expression in malignant pleural mesothelioma is associated with sarcomatoid histology and poor prognosis. J Thorac Oncol 2014;9:1036-40. [Crossref] [PubMed]
- Kansy BA, Concha-Benavente F, Srivastava RM, et al. PD-1 Status in CD8(+) T Cells Associates with Survival and Anti-PD-1 Therapeutic Outcomes in Head and Neck Cancer. Cancer Res 2017;77:6353-64. [Crossref] [PubMed]
- Thompson RH, Dong H, Lohse CM, et al. PD-1 is expressed by tumor-infiltrating immune cells and is associated with poor outcome for patients with renal cell carcinoma. Clin Cancer Res 2007;13:1757-61. [Crossref] [PubMed]
- Zhang Y, Kang S, Shen J, et al. Prognostic significance of programmed cell death 1 (PD-1) or PD-1 ligand 1 (PD-L1) Expression in epithelial-originated cancer: a meta-analysis. Medicine (Baltimore) 2015;94:e515. [Crossref] [PubMed]
- Muenst S, Soysal SD, Gao F, et al. The presence of programmed death 1 (PD-1)-positive tumor-infiltrating lymphocytes is associated with poor prognosis in human breast cancer. Breast Cancer Res Treat 2013;139:667-76. [Crossref] [PubMed]
- Sun S, Fei X, Mao Y, et al. PD-1(+) immune cell infiltration inversely correlates with survival of operable breast cancer patients. Cancer Immunol Immunother 2014;63:395-406. [Crossref] [PubMed]
- Offin M, Rizvi H, Tenet M, et al. Tumor Mutation Burden and Efficacy of EGFR-Tyrosine Kinase Inhibitors in Patients with EGFR-Mutant Lung Cancers. Clin Cancer Res 2019;25:1063-9. [Crossref] [PubMed]
- Lin C, Shi X, Zhao J, et al. Tumor Mutation Burden Correlates With Efficacy of Chemotherapy/Targeted Therapy in Advanced Non-Small Cell Lung Cancer. Front Oncol 2020;10:480. [Crossref] [PubMed]
- Tsui C, Kretschmer L, Rapelius S, et al. MYB orchestrates T cell exhaustion and response to checkpoint inhibition. Nature 2022;609:354-60. [Crossref] [PubMed]
- Beltra JC, Manne S, Abdel-Hakeem MS, et al. Developmental Relationships of Four Exhausted CD8(+) T Cell Subsets Reveals Underlying Transcriptional and Epigenetic Landscape Control Mechanisms. Immunity 2020;52:825-841.e8. [Crossref] [PubMed]
- Czystowska M, Gooding W, Szczepanski MJ, et al. The immune signature of CD8(+)CCR7(+) T cells in the peripheral circulation associates with disease recurrence in patients with HNSCC. Clin Cancer Res 2013;19:889-99. [Crossref] [PubMed]
- Simoni Y, Becht E, Fehlings M, et al. Bystander CD8(+) T cells are abundant and phenotypically distinct in human tumour infiltrates. Nature 2018;557:575-9. [Crossref] [PubMed]
- Meier SL, Satpathy AT, Wells DK. Bystander T cells in cancer immunology and therapy. Nat Cancer 2022;3:143-55. [Crossref] [PubMed]
- Maurice NJ, Taber AK, Prlic M. The Ugly Duckling Turned to Swan: A Change in Perception of Bystander-Activated Memory CD8 T Cells. J Immunol 2021;206:455-62. [Crossref] [PubMed]
- Tripp RA, Hou S, McMickle A, et al. Recruitment and proliferation of CD8+ T cells in respiratory virus infections. J Immunol 1995;154:6013-21.
- Freeman BE, Hammarlund E, Raué HP, et al. Regulation of innate CD8+ T-cell activation mediated by cytokines. Proc Natl Acad Sci U S A 2012;109:9971-6. [Crossref] [PubMed]
- Danahy DB, Berton RR, Badovinac VP. Cutting Edge: Antitumor Immunity by Pathogen-Specific CD8 T Cells in the Absence of Cognate Antigen Recognition. J Immunol 2020;204:1431-5. [Crossref] [PubMed]
- Loftus DJ, Castelli C, Clay TM, et al. Identification of epitope mimics recognized by CTL reactive to the melanoma/melanocyte-derived peptide MART-1(27-35). J Exp Med 1996;184:647-57. [Crossref] [PubMed]
- Vujanovic L, Shi J, Kirkwood JM, et al. Molecular mimicry of MAGE-A6 and Mycoplasma penetrans HF-2 epitopes in the induction of antitumor CD8(+) T-cell responses. Oncoimmunology 2014;3:e954501. [Crossref] [PubMed]
- Richman LP, Vonderheide RH, Rech AJ. Neoantigen Dissimilarity to the Self-Proteome Predicts Immunogenicity and Response to Immune Checkpoint Blockade. Cell Syst 2019;9:375-382.e4. [Crossref] [PubMed]
- Sneddon S, Rive CM, Ma S, et al. Identification of a CD8+ T-cell response to a predicted neoantigen in malignant mesothelioma. Oncoimmunology 2020;9:1684713. [Crossref] [PubMed]
- Koya T, Niida Y, Togi M, et al. The Detection of Immunity against WT1 and SMAD4P130L of EpCAM+ Cancer Cells in Malignant Pleural Effusion. Int J Mol Sci 2022;23:12177. [Crossref] [PubMed]
- Ryu JS, Ryu HJ, Lee SN, et al. Prognostic impact of minimal pleural effusion in non-small-cell lung cancer. J Clin Oncol 2014;32:960-7. [Crossref] [PubMed]
- Marcq E, Waele J, Audenaerde JV, et al. Abundant expression of TIM-3, LAG-3, PD-1 and PD-L1 as immunotherapy checkpoint targets in effusions of mesothelioma patients. Oncotarget 2017;8:89722-35. [Crossref] [PubMed]
- Chee J, Watson MW, Chopra A, et al. Tumour associated lymphocytes in the pleural effusions of patients with mesothelioma express high levels of inhibitory receptors. BMC Res Notes 2018;11:864. [Crossref] [PubMed]
- Kim HR, Park HJ, Son J, et al. Tumor microenvironment dictates regulatory T cell phenotype: Upregulated immune checkpoints reinforce suppressive function. J Immunother Cancer 2019;7:339. [Crossref] [PubMed]
- Li L, Yang L, Wang L, et al. Impaired T cell function in malignant pleural effusion is caused by TGF-β derived predominantly from macrophages. Int J Cancer 2016;139:2261-9. [Crossref] [PubMed]
- Prado-Garcia H, Romero-Garcia S, Puerto-Aquino A, Rumbo-Nava U. The PD-L1/PD-1 pathway promotes dysfunction, but not "exhaustion", in tumor-responding T cells from pleural effusions in lung cancer patients. Cancer Immunol Immunother 2017;66:765-76. [Crossref] [PubMed]
- Khanna S, Thomas A, Abate-Daga D, et al. Malignant Mesothelioma Effusions Are Infiltrated by CD3(+) T Cells Highly Expressing PD-L1 and the PD-L1(+) Tumor Cells within These Effusions Are Susceptible to ADCC by the Anti-PD-L1 Antibody Avelumab. J Thorac Oncol 2016;11:1993-2005. [Crossref] [PubMed]
- Hu CY, Zhang YH, Wang T, et al. Interleukin-2 reverses CD8(+) T cell exhaustion in clinical malignant pleural effusion of lung cancer. Clin Exp Immunol 2016;186:106-14. [Crossref] [PubMed]