Serum tumor markers and outcomes in lung cancer patients with brain metastases: a retrospective longitudinal cohort study
Highlight box
Key findings
• Baseline serum tumor markers (STMs) can predict the prognosis of lung cancer patients with brain metastases (BM).
What is known and what is new?
• Baseline STMs are recommended for cancer diagnosis and surveillance.
• The relationship between baseline levels and dynamic changes of STMs in lung cancer patients with BM and prognosis for the first time was reported.
What is the implication, and what should change now?
• Baseline cytokeratin-19 fragment, carcinoembryonic antigen and neuron-specific enolase levels, as well as status of key driver genes are recommended to predict the prognosis of BM patients. Dynamic changes of STMs during disease course were not significantly associated with the final outcome of BM patients.
Introduction
Lung cancer is the primary cause of cancer-related mortality globally (1-3). Over 50% of lung cancers are diagnosed as unresectable or at an advanced stage, and 15–20% of lung cancer patients have been found to have brain metastases (BM) at the time of diagnosis (4,5). With the development of treatment options, different approaches such as tyrosine kinase inhibitors (TKIs) and immune checkpoint therapy have emerged alongside traditional cytotoxic chemotherapy, improving the long-term survival rate of patients with BM, highlighting the importance of ongoing disease surveillance. In terms of disease monitoring in clinical practice, serum tumor markers (STMs) are widely used in lung cancer (6,7), with carcinoembryonic antigen (CEA), cytokeratin-19 fragment (CYFRA21-1), neuron-specific enolase (NSE), squamous cell carcinoma antigen (SCC-Ag), cancer antigen 125 (CA125) and carbohydrate antigen 199 (CA199), being most sensitive. However, their role in BM remains unclear, lacking clinical evidence.
Previous studies have also emphasized the utilization of STMs alone or in combination to monitor treatment effectiveness and prognosis (8,9). Typically, both CEA and other STMs have been reported as predictive factors at baseline or prognostic factors for disease recurrence, progression-free survival (PFS), or overall survival (OS) (6,10). Moreover, a few studies have suggested that the trajectory of STMs in colorectal cancer can reflect dynamic patterns of changes during the disease progression, providing more information on the relationship between STMs and outcomes (11,12). However, due to the limited number of measurements, the relationship between STMs and the prognosis of patients with BM is still uncertain, and the longitudinal trajectory of STMs is often overlooked. Specifically, there is a lack of understanding regarding the trajectory of STMs in lung cancer patients with BM, and its association with the OS of these patients.
Therefore, we employed a latent class growth mixture model using a large-sample retrospective longitudinal cohort to define the dynamic pattern of STMs, and simultaneously analyzed the role of baseline levels and dynamic changes in STMs in predicting prognosis of lung cancer patients with BM. We present this article in accordance with the STROBE reporting checklist (available at https://tlcr.amegroups.com/article/view/10.21037/tlcr-24-404/rc).
Methods
Ethics
This retrospective study was approved by the Ethics Committee of West China Hospital (No. 2022127). The study was conducted according to the guidelines of the Declaration of Helsinki (as revised in 2013). Individual consent for this analysis was waived due to retrospective nature.
Patients
Referring to our previous literature (13), we obtained the clinicopathological and follow-up data of lung cancer patients with BM from the linked electronic medical care records at West China Hospital during the period from December 1, 2013 to September 30, 2020. Lung cancer patients with BM were verified by pathology reports and brain imaging. The exclusion criteria for this study included: (I) patients aged below 18 years or above 80 years, (II) incomplete STMs determinations, (III) lack of survival information, and (IV) less than 3 visits. BM patients with STMs records from the first admission and follow-up were included for STMs trajectory analysis. Participants who met the inclusion criteria for all STMs trajectory analyses simultaneously were subjected to joint analysis. The treatment regimen (radiotherapy, chemotherapy, immunotherapy, and targeted therapy) for all our included patients was used after diagnosis of BM. Targeted therapy includes anti-angiogenesis treatment and small molecule targeted therapy.
STMs measurement
Serum CYFRA21-1, CEA and NSE were selected in this study. A total of 3 mL of peripheral venous blood was withdrawn, serum was separated at 4,000 r/min and stored at −80 ℃ for measurement. An electrochemiluminescence immunoassay (Roche Cobas e601) was used to evaluate the three STMs. Normal reference values of CEA, CYFRA21-1 and NSE are <3.4, <3.0, and <15.0 ng/mL, respectively. Each patient underwent at least three follow-up measurements before being included in the analysis.
Surveillance outcome
The primary outcome was to evaluate the prognostic roles of STMs in the OS of lung cancer patients with BM. Kaplan-Meier analysis, Cox proportional hazards model, and log-rank test were performed.
Statistical analysis
The relationship between the baseline levels of CEA, CYFRA21-1, as well as NSE and BM outcomes was evaluated by Kaplan-Meier analysis and Cox proportional hazard models, which included age, gender, smoking, status of key driver genes, Graded Prognostic Assessment (GPA) score of BM and treatment regimen (radiotherapy, chemotherapy, immunotherapy, and targeted therapy). Kaplan-Meier analysis and cox proportional hazard models were performed via the “survival” package in R (version 4.2.1).
We utilized longitudinal mode to analyze the patterns and trajectories of STMs in lung cancer patients with BM. The logarithms of CEA, CYFRA21-1, and NSE values were then used for trajectory fitting due to their non-normal distribution. A latent class growth mixed model (LCGMM) was utilized to identify different trajectory patterns, considering the heterogeneity of the population. This model included longitudinal measurements as linear or nonlinear functions of time, with 2–5 potential groups considered. The optimal number of groups and the best fitting shape were determined based on the Bayesian information criterion, ensuring a representative proportion in each group. LCGMM analysis was carried out using the “lcmm” package in R (version 4.2.1). The relationship between trajectory groups and BM outcomes was evaluated by Kaplan-Meier analysis and Cox proportional hazard models with age, sex, and baseline levels adjusted.
Results
Participant characteristics
During the period from December 2013 and September 2020, a total of 1,169 lung cancer patients with BM were included in this study. Of these, 702 were males and 467 were females. The age distribution was as follows: 323 patients were under 50 years old, 419 were aged 50–60 years old, and 427 were over 60 years old. Among the participants, 591 were never-smokers, 180 were current smokers, and 398 were former smokers. According to the World Health Organization/International Association for the Study of Lung Cancer (WHO/IASLC) classification criteria for lung tumors, SCC accounted for 10.9%, adenocarcinoma (AD) for 66.9%, small cell lung cancer (SCLC) for 14.5%, and not otherwise specified (NOS) for the remaining 7.4%. In terms of treatment, 485 cases (41.5%) underwent targeted therapy, 39 cases (3.3%) underwent immunotherapy, 627 cases (53.6%) underwent radiotherapy and 954 cases (81.6%) underwent chemotherapy. The baseline characteristics of patients are shown in Table 1.
Table 1
Features | Level | Total | CYFRA21-1, ng/mL | NSE, ng/mL | CEA, ng/mL | ||||||||
---|---|---|---|---|---|---|---|---|---|---|---|---|---|
≥4.66 | <4.66 | P | ≥15.5 | <15.5 | P | ≥9.35 | <9.35 | P | |||||
n | 1,169 | 585 | 584 | 585 | 584 | 585 | 584 | ||||||
Histology | AD | 782 (66.9) | 407 (69.6) | 375 (64.2) | <0.001 | 351 (60.0) | 431 (73.8) | <0.001 | 449 (76.8) | 333 (57.0) | <0.001 | ||
AD_SCC | 3 (0.3) | 2 (0.3) | 1 (0.2) | 1 (0.2) | 2 (0.3) | 2 (0.3) | 1 (0.2) | ||||||
SAR | 1 (0.1) | 1 (0.2) | 0 (0.0) | 0 (0.0) | 1 (0.2) | 0 (0.0) | 1 (0.2) | ||||||
SCC | 127 (10.9) | 81 (13.8) | 46 (7.9) | 61 (10.4) | 66 (11.3) | 39 (6.7) | 88 (15.1) | ||||||
SCLC | 169 (14.5) | 45 (7.7) | 124 (21.2) | 121 (20.7) | 48 (8.2) | 54 (9.2) | 115 (19.7) | ||||||
NOS | 87 (7.3) | 49 (8.4) | 38 (6.5) | 51 (8.7) | 36 (6.2) | 41 (7.0) | 46 (7.9) | ||||||
Age (years) | <50 | 323 (27.6) | 144 (24.6) | 179 (30.7) | 0.03 | 149 (25.5) | 174 (29.8) | 0.25 | 154 (26.3) | 169 (28.9) | 0.047 | ||
>60 | 427 (36.6) | 232 (39.7) | 195 (33.4) | 222 (37.9) | 205 (35.1) | 234 (40.0) | 193 (33.0) | ||||||
50–60 | 419 (35.8) | 209 (35.7) | 210 (36.0) | 214 (36.6) | 205 (35.1) | 197 (33.7) | 222 (38.0) | ||||||
Gender | Male | 702 (60.1) | 370 (63.2) | 332 (56.8) | 0.03 | 371 (63.4) | 331 (56.7) | 0.02 | 330 (56.4) | 372 (63.7) | 0.01 | ||
Female | 467 (39.9) | 215 (36.8) | 252 (43.2) | 214 (36.6) | 253 (43.3) | 255 (43.6) | 212 (36.3) | ||||||
Smoking | No | 591 (76.7) | 280 (73.5) | 311 (79.7) | 0.049 | 269 (72.9) | 322 (80.1) | 0.02 | 322 (78.2) | 269 (74.9) | 0.33 | ||
Yes | 180 (23.3) | 101 (26.5) | 79 (20.3) | 100 (27.1) | 80 (19.9) | 90 (21.8) | 90 (25.1) | ||||||
KPS | <70 | 78 (6.7) | 37 (6.3) | 41 (7.0) | 0.76 | 39 (6.7) | 39 (6.7) | 0.67 | 31 (5.3) | 47 (8.0) | 0.17 | ||
>90 | 33 (2.8) | 15 (2.6) | 18 (3.1) | 14 (2.4) | 19 (3.3) | 17 (2.9) | 16 (2.7) | ||||||
70–90 | 1,058 (90.5) | 533 (91.1) | 525 (89.9) | 532 (90.9) | 526 (90.1) | 537 (91.8) | 521 (89.2) | ||||||
EM | No | 212 (18.1) | 75 (12.8) | 137 (23.5) | <0.001 | 79 (13.5) | 133 (22.8) | <0.001 | 66 (11.3) | 146 (25.0) | <0.001 | ||
Yes | 957 (81.9) | 510 (87.2) | 447 (76.5) | 506 (86.5) | 451 (77.2) | 519 (88.7) | 438 (75.0) | ||||||
MT | >3 | 560 (47.9) | 297 (50.8) | 263 (45.0) | 0.02 | 301 (51.5) | 259 (44.3) | 0.052 | 308 (52.6) | 252 (43.2) | 0.004 | ||
1 | 441 (37.7) | 197 (33.7) | 244 (41.8) | 205 (35.0) | 236 (40.4) | 197 (33.7) | 244 (41.8) | ||||||
2–3 | 168 (14.4) | 91 (15.6) | 77 (13.2) | 79 (13.5) | 89 (15.2) | 80 (13.7) | 88 (15.1) | ||||||
BMI (kg/m2) | 22.32 [20.34, 24.45] |
22.03 [20.20, 24.06] |
22.66 [20.70, 24.77] |
<0.001 | 22.04 [20.05, 24.24] |
22.60 [20.62, 24.58] |
0.01 | 22.31 [20.42, 24.43] |
22.40 [20.31, 24.47] |
0.65 | |||
EGFR | Negative | 238 (20.4) | 126 (21.5) | 112 (19.2) | 0.40 | 109 (18.6) | 129 (22.1) | 0.002 | 119 (20.3) | 119 (20.4) | 0.01 | ||
Positive | 265 (22.6) | 137 (23.4) | 128 (21.9) | 113 (19.3) | 152 (26.0) | 153 (26.2) | 112 (19.2) | ||||||
Unknown | 666 (57.0) | 322 (55.0) | 344 (58.9) | 363 (62.1) | 303 (51.9) | 313 (53.5) | 353 (60.4) | ||||||
ALK | Negative | 369 (31.6) | 199 (34.0) | 170 (29.1) | 0.11 | 169 (28.9) | 200 (34.2) | 0.04 | 186 (31.8) | 183 (31.3) | 0.99 | ||
Positive | 60 (5.1) | 25 (4.3) | 35 (6.0) | 25 (4.3) | 35 (6.0) | 30 (5.1) | 30 (5.1) | ||||||
Unknown | 740 (63.3) | 361 (61.7) | 379 (64.9) | 391 (66.8) | 349 (59.8) | 369 (63.1) | 371 (63.5) | ||||||
ROS | Negative | 311 (26.6) | 165 (28.2) | 146 (25.0) | 0.42 | 143 (24.4) | 168 (28.8) | 0.12 | 149 (25.5) | 162 (27.7) | 0.61 | ||
Positive | 14 (1.2) | 6 (1.0) | 8 (1.4) | 5 (0.9) | 9 (1.5) | 8 (1.4) | 6 (1.0) | ||||||
Unknown | 844 (72.2) | 414 (70.8) | 430 (73.6) | 437 (74.7) | 407 (69.7) | 428 (73.2) | 416 (71.2) | ||||||
Immunotherapy | No | 1,130 (96.7) | 562 (96.1) | 568 (97.3) | 0.33 | 565 (96.6) | 565 (96.7) | >0.99 | 575 (98.3) | 555 (95.0) | 0.003 | ||
Yes | 39 (3.3) | 23 (3.9) | 16 (2.7) | 20 (3.4) | 19 (3.3) | 10 (1.7) | 29 (5.0) | ||||||
Targeted therapy |
No | 684 (58.5) | 325 (55.6) | 359 (61.5) | 0.046 | 363 (62.1) | 321 (55.0) | 0.02 | 304 (52.0) | 380 (65.1) | <0.001 | ||
Yes | 485 (41.5) | 260 (44.4) | 225 (38.5) | 222 (37.9) | 263 (45.0) | 281 (48.0) | 204 (34.9) | ||||||
Radiotherapy | No | 542 (46.4) | 295 (50.4) | 247 (42.3) | 0.006 | 284 (48.5) | 258 (44.2) | 0.15 | 300 (51.3) | 242 (41.4) | 0.001 | ||
Yes | 627 (53.6) | 290 (49.6) | 337 (57.7) | 301 (51.5) | 326 (55.8) | 285 (48.7) | 342 (58.6) | ||||||
Chemotherapy | No | 215 (18.4) | 122 (20.9) | 93 (15.9) | 0.04 | 115 (19.7) | 100 (17.1) | 0.30 | 125 (21.4) | 90 (15.4) | 0.01 | ||
Yes | 954 (81.6) | 463 (79.1) | 491 (84.1) | 470 (80.3) | 484 (82.9) | 460 (78.6) | 494 (84.6) | ||||||
GPA | 0–1.0 | 413 (35.3) | 235 (40.2) | 178 (30.5) | <0.001 | 238 (40.7) | 175 (30.0) | <0.001 | 242 (41.4) | 171 (29.3) | <0.001 | ||
1.5–2.0 | 518 (44.3) | 263 (45.0) | 255 (43.7) | 249 (42.6) | 269 (46.0) | 249 (42.6) | 269 (46.1) | ||||||
2.5–3.0 | 217 (18.6) | 83 (14.2) | 134 (22.9) | 92 (15.7) | 125 (21.4) | 90 (15.4) | 127 (21.7) | ||||||
3.5–4.0 | 21 (1.8) | 4 (0.6) | 17 (2.9) | 6 (1.0) | 15 (2.6) | 4 (0.6) | 17 (2.9) |
Data are presented as n (%) or median [IQR]. CYFRA21-1, cytokeratin-19 fragment; NSE, neuron-specific enolase; CEA, carcinoembryonic antigen; AD, adenocarcinoma; SCC, squamous cell carcinoma; SAR, sarcomatoid; SCLC, small cell lung cancer; NOS, not otherwise specified; KPS, Karnofsky Performance Status; EM, extracranial metastases; MT, brain metastasis; BMI, body mass index; EGFR, epidermal growth factor receptor; ALK, anaplastic lymphoma kinase; ROS, reactive oxygen species; GPA, Graded Prognostic Assessment; IQR, interquartile range.
Association of single STMs at baseline with BM survival
In order to investigate the clinical significance of individual STMs (CEA, CYFRA21-1, and NSE) baseline levels, we collected baseline STM data and utilized the median as the cutoff value to classify patients into high- and low-level groups. The baseline characteristics of patients were further analyzed in detail (Table 1). In the high NSE levels group (≥15.5 ng/mL), there was a higher proportion of male individuals (63.4% vs. 56.7%), a lower body mass index (BMI), a higher prevalence of SCLC pathology type (20.7% vs. 8.2%), a higher prevalence of smoking habits (27.1% vs. 19.9%), and a lower GPA score (40.7% vs. 30%). Similarly, in the high CYFRA21-1 levels group (≥4.66 ng/mL), there was a higher prevalence of male individuals (63.2% vs. 56.8%), a lower BMI, or a higher prevalence of SCC pathology types (13.8% vs. 7.9%), had more number of brain metastatic lesions (50.8% vs. 45%), and a lower GPA score (40.2% vs. 30.5%). Conversely, the high CEA levels group (≥9.35 ng/mL) shows a tendency towards female gender (43.6% vs. 36.3%), age over 60 years (40% vs. 33%), presence of AD pathology (76.8% vs. 57%), more number of brain metastatic lesions (52.6% vs. 43.2%), a higher prevalence of positive epidermal growth factor receptor (EGFR) (26.2% vs. 19.2%), and a lower GPA score (41.4% vs. 29.3%). Furthermore, we observed that a significant proportion of BM patients with AD exhibit elevated levels of CEA (76.8%), CYFRA21-1 (69.6%) or NSE (60%).
In terms of survival, Kaplan-Meier survival analysis revealed that all STMs (CEA, CYFRA21-1, and NSE) were negatively correlated with survival outcomes (Figure 1A-1C). Patients with low serum NSE levels (<15.5 ng/mL) exhibited a significantly extended median survival time (MST) (P<0.001) compared to those with high NSE levels (≥15.5 ng/mL) [29 months (95% CI: 27–31) vs. 19 months (95% CI: 18–21)], indicating a survival benefit (P<0.001) for patients with low baseline serum NSE levels. Similarly, patients with low baseline serum CYFRA21-1 levels (<4.66 ng/mL) had a longer MST of 27 months (95% CI: 24–29) in contrast to patients with high CYFRA21-1 levels (≥4.66 ng/mL) with an MST of 21 months (95% CI: 19–23). Additionally, patients with low baseline serum CEA levels (<9.35 ng/mL) displayed a prolonged MST (P=0.01) of 24 months (95% CI: 22–27) compared to patients with high CEA levels (≥9.35 ng/mL), who had an MST of 23 months (95% CI: 21–25).
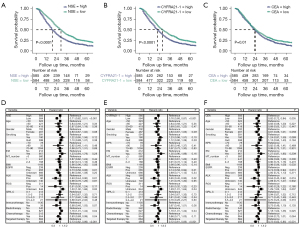
Multivariate Cox regression analysis to identify poor prognostic factors at baseline levels
We conducted a multivariate Cox regression analysis and observed a significant correlation between OS and CYFRA21-1 (P<0.001), CEA (P=0.005) as well as NSE (P<0.001), serving as independent prognostic factors (Figure 1D-1F). When all three were included in the Cox model as continuous variables, only CYFRA21-1 (P<0.001) as well as NSE (P=0.03) remained statistically different (Figure S1). In either scenario, the mutation status of anaplastic lymphoma kinase (ALK) (P<0.05) and EGFR (P<0.05) as well as receiving radiotherapy and targeted therapy were independent prognostic factors for patients with BM (Figure 1D-1F). Further analysis indicated that baseline CYFRA21-1, CEA, NSE as well as status of key driver genes were independent prognostic factors in non-small cell lung cancer (NSCLC) patients with BM, while for SCLC patients with BM, baseline NSE and receiving chemotherapy show independent correlations with survival (Figures S2-S4).
Net reclassification index (NRI) for individual STMs baseline levels
When each STM was individually integrated into the NRI clinical model, as compared to traditional GPA scores, each STM demonstrated an enhancement in discriminatory ability (Table 2). Among these, NSE exhibited the most substantial enhancement in NRI. The inclusion of NSE [NRI (categorical) 0.11, 95% CI: 0.04–0.2; NRI (continuous) 0.27, 95% CI: 0.1–0.38], CYFRA21-1 [NRI (categorical) 0.10, 95% CI: 0.03–0.2; NRI (continuous) 0.25, 95% CI: 0.09–0.38], or CEA [NRI (categorical) 0.08, 95% CI: 0.018–0.18; NRI (continuous) 0.21, 95% CI: 0.07–0.33] led to an increase in the accurate reclassification rate for both positive and negative outcomes.
Table 2
Basic model | NRI (categorical) (95% CI) | NRI (continuous) (95% CI) |
---|---|---|
+NSE | 0.11109563 (0.04212546–0.2003059) | 0.27211232 (0.09736432–0.3777031) |
+CYFRA21-1 | 0.09746602 (0.030884354–0.1973936) | 0.24931891 (0.09230719–0.3756895) |
+CEA | 0.07822513 (0.01807649–0.18462074) | 0.20980775 (0.06645839–0.3290698) |
NRI, net reclassification improvement; STMs, serum tumor markers; GPA, Graded Prognostic Assessment; CI, confidence interval; NSE, neuron-specific enolase; CYFRA21-1, cytokeratin-19 fragment; CEA, carcinoembryonic antigen.
Clinical value of STMs trajectory modeling
We conducted a longitudinal analysis to analyze the trajectories of STMs and understand how they evolve over time in the progression and treatment process of BM patients. Table 3 shows the baseline characteristics of participants in the trajectory groups of STMs. Based on LCGMM, the trajectories of STMs were classified by three classes. The distribution of the 1,169 patients with NSE across the three classes was 48 (class 1, persistently-rising trajectory), 1,014 (class 2, normal-level trajectory), and 107 (class 3, persistently-descending); the distribution of CYFRA21-1 was 933 (class 1, normal-level trajectory), 62 (class 2, early-rising trajectory), and 174 (class 3, later-rising trajectory); and the distribution of CEA was 914 (class 1, normal-level trajectory), 67 (class 2, baseline-high trajectory) and 188 (class 3, persistently-rising trajectory) (Figure 2A-2C).
Table 3
Features | Level | Total | CYFRA21-1 | NSE | CEA | |||||||||||
---|---|---|---|---|---|---|---|---|---|---|---|---|---|---|---|---|
1 | 2 | 3 | P | 1 | 2 | 3 | P | 1 | 2 | 3 | P | |||||
n | 1,169 | 933 | 62 | 174 | 48 | 1,014 | 107 | 914 | 67 | 188 | ||||||
Histology | AD | 782 (66.9) | 602 (64.5) | 39 (62.9) | 141 (81.0) | <0.001 | 5 (10.4) | 735 (72.5) | 42 (39.3) | <0.001 | 567 (62.0) | 57 (85.1) | 158 (84.0) | <0.001 | ||
AD_SCC | 3 (0.3) | 3 (0.3) | 0 (0.0) | 0 (0.0) | 0 (0.0) | 3 (0.3) | 0 (0.0) | 2 (0.2) | 0 (0.0) | 1 (0.5) | ||||||
SAR | 1 (0.1) | 1 (0.1) | 0 (0.0) | 0 (0.0) | 0 (0.0) | 1 (0.1) | 0 (0.0) | 1 (0.1) | 0 (0.0) | 0 (0.0) | ||||||
SCC | 127 (10.9) | 92 (9.9) | 17 (27.4) | 18 (10.3) | 2 (4.2) | 114 (11.2) | 11 (10.3) | 118 (12.9) | 2 (3.0) | 7 (3.7) | ||||||
SCLC | 169 (14.5) | 164 (17.6) | 1 (1.6) | 4 (2.3) | 34 (70.8) | 88 (8.7) | 47 (43.9) | 154 (16.8) | 2 (3.0) | 13 (6.9) | ||||||
NOS | 87 (7.3) | 71 (7.6) | 5 (8.1) | 11 (6.3) | 7 (14.6) | 73 (7.2) | 7 (6.5) | 72 (7.9) | 6 (9.0) | 9 (4.8) | ||||||
Age (years) | <50 | 323 (27.6) | 258 (27.7) | 20 (32.3) | 45 (25.9) | 0.75 | 8 (16.7) | 286 (28.2) | 29 (27.1) | 0.29 | 246 (26.9) | 14 (20.9) | 63 (33.5) | 0.03 | ||
>60 | 427 (36.6) | 341 (36.5) | 24 (38.7) | 62 (35.6) | 21 (43.8) | 361 (35.6) | 45 (42.1) | 323 (35.3) | 31 (46.3) | 73 (38.8) | ||||||
50–60 | 419 (35.8) | 334 (35.8) | 18 (29.0) | 67 (38.5) | 19 (39.6) | 367 (36.2) | 33 (30.8) | 345 (37.7) | 22 (32.8) | 52 (27.7) | ||||||
Gender | Male | 702 (60.1) | 545 (58.4) | 51 (82.3) | 106 (60.9) | 0.001 | 42 (87.5) | 577 (56.9) | 83 (77.6) | <0.001 | 559 (61.2) | 41 (61.2) | 102 (54.3) | 0.21 | ||
Female | 467 (39.9) | 388 (41.6) | 11 (17.7) | 68 (39.1) | 6 (12.5) | 437 (43.1) | 24 (22.4) | 355 (38.8) | 26 (38.8) | 86 (45.7) | ||||||
Smoking | No | 591 (76.7) | 487 (78.5) | 21 (56.8) | 83 (72.8) | 0.006 | 12 (50.0) | 549 (79.2) | 30 (55.6) | <0.001 | 441 (75.6) | 35 (76.1) | 115 (81.0) | 0.40 | ||
Yes | 180 (23.3) | 133 (21.5) | 16 (43.2) | 31 (27.2) | 12 (50.0) | 144 (20.8) | 24 (44.4) | 142 (24.4) | 11 (23.9) | 27 (19.0) | ||||||
KPS | <70 | 78 (6.7) | 58 (6.2) | 5 (8.1) | 15 (8.6) | 0.49 | 2 (4.2) | 73 (7.2) | 3 (2.8) | 0.28 | 68 (7.4) | 4 (6.0) | 6 (3.2) | 0.24 | ||
>90 | 33 (2.8) | 28 (3.0) | 0 (0.0) | 5 (2.9) | 0 (0.0) | 30 (3.0) | 3 (2.8) | 25 (2.7) | 1 (1.5) | 7 (3.7) | ||||||
70–90 | 1,058 (90.5) | 847 (90.8) | 57 (91.9) | 154 (88.5) | 46 (95.8) | 911 (89.8) | 101 (94.4) | 821 (89.8) | 62 (92.5) | 175 (93.1) | ||||||
EM | No | 212 (18.1) | 191 (20.5) | 4 (6.5) | 17 (9.8) | <0.001 | 6 (12.5) | 195 (19.2) | 11 (10.3) | 0.043 | 191 (20.9) | 8 (11.9) | 13 (6.9) | <0.001 | ||
Yes | 957 (81.9) | 742 (79.5) | 58 (93.5) | 157 (90.2) | 42 (87.5) | 819 (80.8) | 96 (89.7) | 723 (79.1) | 59 (88.1) | 175 (93.1) | ||||||
MT | >3 | 560 (47.9) | 439 (47.1) | 30 (48.4) | 91 (52.3) | 0.08 | 22 (45.8) | 478 (47.1) | 60 (56.1) | 0.42 | 428 (46.8) | 31 (46.3) | 101 (53.7) | 0.17 | ||
1 | 441 (37.7) | 369 (39.5) | 21 (33.9) | 51 (29.3) | 20 (41.7) | 385 (38.0) | 36 (33.6) | 359 (39.3) | 22 (32.8) | 60 (31.9) | ||||||
2–3 | 168 (14.4) | 125 (13.4) | 11 (17.7) | 32 (18.4) | 6 (12.5) | 151 (14.9) | 11 (10.3) | 127 (13.9) | 14 (20.9) | 27 (14.4) | ||||||
BMI | 22.32 [20.34, 24.45] |
22.46 [20.50, 24.49] |
21.63 [19.48, 23.24] |
21.97 [19.84, 24.13] |
0.02 | 21.76 [18.93, 25.09] |
22.41 [20.40, 24.44] |
21.83 [20.54, 24.78] |
0.78 | 22.36 [20.39, 24.44] |
21.62 [19.53, 24.52] |
22.45 [20.54, 24.48] |
0.33 | |||
EGFR | Negative | 238 (20.4) | 161 (17.3) | 22 (35.5) | 55 (31.6) | <0.001 | 2 (4.2) | 212 (20.9) | 24 (22.4) | <0.001 | 186 (20.4) | 16 (23.9) | 36 (19.1) | 0.001 | ||
Positive | 265 (22.6) | 206 (22.1) | 12 (19.4) | 47 (27.0) | 2 (4.2) | 253 (25.0) | 10 (9.3) | 183 (20.0) | 22 (32.8) | 60 (31.9) | ||||||
Unknown | 666 (57.0) | 566 (60.7) | 28 (45.2) | 72 (41.4) | 44 (91.7) | 549 (54.1) | 73 (68.2) | 545 (59.6) | 29 (43.3) | 92 (48.9) | ||||||
ALK | Negative | 369 (31.6) | 269 (28.8) | 25 (40.3) | 75 (43.1) | 0.001 | 3 (6.2) | 341 (33.6) | 25 (23.4) | <0.001 | 284 (31.1) | 24 (35.8) | 61 (32.4) | 0.65 | ||
Positive | 60 (5.1) | 46 (4.9) | 3 (4.8) | 11 (6.3) | 0 (0.0) | 54 (5.3) | 6 (5.6) | 48 (5.3) | 5 (7.5) | 7 (3.7) | ||||||
Unknown | 740 (63.3) | 618 (66.2) | 34 (54.8) | 88 (50.6) | 45 (93.8) | 619 (61.0) | 76 (71.0) | 582 (63.7) | 38 (56.7) | 120 (63.8) | ||||||
ROS | Negative | 311 (26.6) | 224 (24.0) | 23 (37.1) | 64 (36.8) | 0.002 | 3 (6.2) | 287 (28.3) | 21 (19.6) | 0.004 | 246 (26.9) | 16 (23.9) | 49 (26.1) | 0.67 | ||
Positive | 14 (1.2) | 11 (1.2) | 0 (0.0) | 3 (1.7) | 0 (0.0) | 13 (1.3) | 1 (0.9) | 13 (1.4) | 0 (0.0) | 1 (0.5) | ||||||
Unknown | 844 (72.2) | 698 (74.8) | 39 (62.9) | 107 (61.5) | 45 (93.8) | 714 (70.4) | 85 (79.4) | 655 (71.7) | 51 (76.1) | 138 (73.4) | ||||||
GPA | 0–1 | 413 (35.3) | 318 (34.1) | 24 (38.7) | 71 (40.8) | 0.008 | 24 (50.0) | 345 (34.0) | 44 (41.1) | 0.043 | 308 (33.7) | 29 (43.3) | 76 (40.4) | 0.16 | ||
1.5–2 | 518 (44.3) | 403 (43.2) | 31 (50.0) | 84 (48.3) | 12 (25.0) | 456 (45.0) | 50 (46.7) | 410 (44.9) | 28 (41.8) | 80 (42.6) | ||||||
2.5–3.0 | 217 (18.6) | 192 (20.6) | 6 (9.7) | 19 (10.9) | 11 (22.9) | 194 (19.1) | 12 (11.2) | 176 (19.3) | 9 (13.4) | 32 (17.0) | ||||||
3.5–4.0 | 21 (1.8) | 20 (2.1) | 1 (1.6) | 0 (0.0) | 1 (2.1) | 19 (1.9) | 1 (0.9) | 20 (2.2) | 1 (1.5) | 0 (0.0) |
Data are presented as n (%) or median [IQR]. CYFRA21-1: class 1, normal-level trajectory; class 2, early-rising trajectory; class 3, later-rising trajectory. CEA: class 1, normal-level trajectory; class 2, baseline-high trajectory; class 3, persistently-rising trajectory. NSE: class 1, persistently-rising trajectory; class 2, normal-level trajectory; class 3, persistently-descending. STMs, serum tumor markers; CYFRA21-1, cytokeratin-19 fragment; NSE, neuron-specific enolase; CEA, carcinoembryonic antigen; AD, adenocarcinoma; SCC, squamous cell carcinoma; SAR, sarcomatoid; SCLC, small cell lung cancer; NOS, not otherwise specified; KPS, Karnofsky Performance Status; EM, extracranial metastases; MT, brain metastasis; BMI, body mass index; EGFR, epidermal growth factor receptor; ALK, anaplastic lymphoma kinase; ROS, reactive oxygen species; GPA, Graded Prognostic Assessment; IQR, interquartile range.
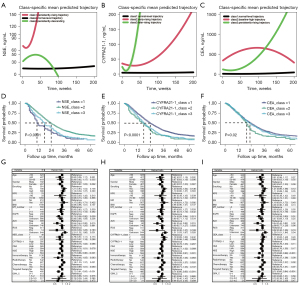
Relationship between BM survival and STM trajectories
Kaplan-Meier survival analyses showed that dynamic changes in all STMs (CEA, CYFRA21-1 and NSE) correlated with prognosis. In terms of NSE, class 1 (persistently-rising trajectory) had the worst prognosis, with a median time of 11 months (95% CI: 10–19). In contrast, the class 2 (normal-level trajectory) had the best prognosis, with an MST of 25 months (95% CI: 23–27), and the class 3 (persistently-descending) had a more intermediate median time of 18 months (95% CI: 15–23). In the dynamic analysis of serum CYFRA21-1 levels, the class 1 (normal-level trajectory) group had the best prognosis (P<0.001), with an MST of 25 months (95% CI: 23–27), and the class 2 (early-rising trajectory) and 3 (later-rising trajectory) groups all had a worse prognosis, with an MST of 17 months (95% CI: 14–23) and 19.5 months (95% CI: 17–23), respectively. In dynamic analysis of serum CEA levels, class 1 (normal-level trajectory) had the best prognosis, with a MST of 23 months (95% CI: 22–25); class 2 (baseline-high trajectory) had the worst prognosis, with a MST of 20 months (95% CI: 17–23); and class 3 (persistently-rising trajectory) had an intermediate prognosis, with a median time of 23 months (95% CI: 20–26) (Figure 2D-2F).
Multivariate Cox regression analysis and subgroup analysis
After excluding confounding factors using multivariate Cox regression analysis, dynamic changes of STMs were not significantly correlated with survival. In either scenario, status of driver genes (the mutation status of ALK and EGFR), receiving targeted therapy, baseline NSE level were independent prognostic factors for patients with BM (Figure 2G-2I). The joining of STM analysis showed that for both NSCLC and SCLC, there was no statistically significant difference in the dynamic trajectory of STMs (CEA, CYFRA21-1 and NSE), while NSCLC patients with positive driver genes (P<0.05) have better survival, and SCLC patients with chemotherapy (P<0.001) have better survival (Figure 3). Further analysis indicated that among BM patients lacking key driver genes, NSE trajectory(P<0.05), CYFRA21-1 trajectory (P<0.05) and receiving chemotherapy (P<0.001) were independent prognostic factors (Figure S5).
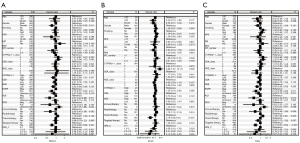
Discussion
We report for the first time the relationship between baseline levels and dynamic changes of STMs (CEA, CYFRA21-1 and NSE) in lung cancer patients with BM and prognosis. We found that serum CEA, CYFRA21-1 as well as NSE at baseline levels were correlated with OS of patients, serving as independent prognostic factors. Moreover, the performance of the GPA model was significantly improved with the addition of STMs at baseline levels. More specifically, we delineated the dynamic evolution trajectories of STMs. Each type of STMs can be divided into three different types based on changes in disease course. However, the dynamic alterations of STMs levels lacked prognostic significance. For patients with non-driver gene, the dynamic trajectory of CYFRA21-1 and NSE, as well as receiving chemotherapy were independent prognostic factors.
Several studies have shown that STMs are biomarkers and associated with BM development in lung cancer (14,15). After reviewing data from over 1,000 patients with BM from lung cancer from December 2013 and September 2020, we found that, the baseline levels of serum CEA (cut-off: 9.35 ng/mL), CYFRA21-1 (cut-off: 4.66 ng/mL) and NSE (cut-off: 15.5 ng/mL) were independent prognostic factors related to the outcomes of BM, representing a previously unexplored discovery. Normal reference values of CEA, CYFRA21-1 and NSE are <3.4, <3.0, and <15.0 ng/mL, respectively. In our study, the cutoff values of CYFRA21-1 and NSE did not differ much from previous normal reference ranges. However, the situation is different for CEA (which is elevated). This also explains why, after including STMs as continuous variables in Cox regression, only CEA did not show statistical significance, which suggesting that the baseline level of CEA should have a higher value when used as a prognostic indicator. Furthermore, the status of key driver gene mutations (EGFR and ALK) was considered an independent prognostic indicator for patients with BM, consistent with previous reports (16,17). Individuals with positive mutation of driver gene benefit from personalized targeted therapy, which has the characteristics of low molecular weight and effective penetration of the blood-brain barrier, thereby enhancing therapeutic efficacy in brain tissue (18,19). Moreover, this analysis showed that GPA scores did not demonstrate predictive significance. GPA was first published in 2008 (20), including age, Karnofsky Performance Status (KPS), number of BM, and extracranial metastases, based on data from 1,960 patients from five randomly assigned Radiation Therapy Oncology Group (RTOG) trials. However, we collected data from patients with BM after 2010, with an increasing proportion receiving targeted therapy, radiotherapy and immunotherapy. The advancements in targeted therapy and immunotherapy have improved patient outcomes, necessitating further updates and research into prognostic models for BM.
A LCGMM was used for the first time to determine the dynamic trajectory of STMs of lung cancer patients with BM using a large sample of cohort. Indeed, we found that after for lung cancer patients with BM receiving treatments, serum STM level showed dynamic changes. And we identified for the first time the distinct dynamic trajectories of CEA, CYFRA21-1, and NSE in lung cancer patients with BM, and analyzed their relationship with prognosis. Li et al. reported that the simultaneously dynamic measurements of CEA, CA199, and CA125 can be as independent prognostic factors of colorectal cancer patients (11), indicating the importance of dynamic monitoring of STMs. Interestingly, for patients with BM, no matter whether CYFRA21-1 rises dynamically earlier or later, patients with high baseline levels have poor prognosis, which is consistent with the close relationship between baseline levels of CYFRA21-1 and prognosis. After excluding confounding factors, dynamic changes of STMs during disease course were not significantly associated with the final outcome of BM patients, while status of driver gene and receiving targeted therapy is an absolute prognostic factor for BM patients. The mechanisms of these interactions identified are not yet clear and need further exploration in future studies. For patients with lung cancer BM, the combined use of anti-angiogenic targeted drugs has shown promising anti-tumor activity and tolerable safety (21). Of course, with the widespread adoption of next-generation sequencing (NGS) over the past decade, a positive driver gene status means the accessibility to targeted therapy and better control of BM disease progression (22-24). This is consistent with the findings of our study, emphasizing the importance of driver gene testing and targeted therapy in the management of BM patients, as well as the need for future research to delve into exploring their interaction mechanisms. In subgroup analysis, we observed that the dynamic trajectory of CYFRA21-1 and NSE as well as receiving chemotherapy were closely related to prognosis in driver gene-negative patients. Due to the limitation of the number of research subjects, this conclusion still needs further verification in future studies.
Nevertheless, there are several limitations in this study. The data set evaluated was a heterogeneous cohort of patients with BM. We collected the survival data of patients with BM from different treatment models and histology, and we did not study the prognosis of patients with different treatment models separately. There would be selection bias in the final results.
Conclusions
Baseline levels of serum CEA, CYFRA21-1 and NSE are recommended for evaluating the prognosis of lung cancer patients with BM. Dynamic changes of STMs during disease course were not significantly associated with the final outcome of BM patients. More attention to driver gene testing and targeted therapy may be needed in the management of BM patients.
Acknowledgments
Funding: The work was supported by
Footnote
Reporting Checklist: The authors have completed the STROBE reporting checklist. Available at https://tlcr.amegroups.com/article/view/10.21037/tlcr-24-404/rc
Data Sharing Statement: Available at https://tlcr.amegroups.com/article/view/10.21037/tlcr-24-404/dss
Peer Review File: Available at https://tlcr.amegroups.com/article/view/10.21037/tlcr-24-404/prf
Conflicts of Interest: All authors have completed the ICMJE uniform disclosure form (available at https://tlcr.amegroups.com/article/view/10.21037/tlcr-24-404/coif). All authors report funding from the National Key Research and Development Program of China (2021YFE0206600), the International Science and Technology Cooperation Program of Chengdu Science and Technology Bureau (2024-YF06-00011-HZ and 2022-GH03-00004-HZ), the Strategic Cooperation Special Fund of Sichuan University-Dazhou Municipal People’s Government (2021CDDZ-25), the Science and Technology Cooperation Special Fund of Sichuan University-Zigong (2021CDZG-24), and the Health Research Project of Chengdu Eastern New Area Management Committee (202304), Talent Excellence Development Project of West China Hospital (ZYYC23006), the Achievement Transformation Fund of West China Hospital (CGZH19002), Science and Technology Project of Sichuan Provincial Health Commission (Clinical Research Special Project JH2023082), Clinical Research Incubation Project of West China Hospital (23HXFH001), the National Natural Sciences Foundation of China (82172842), Sichuan Provincial Science and Technology Department Key Research and Development Program (2022YFSY0012), the Ministry of Education University-Industry Collaborative Education Program (230720523707281), and Miaozi Project in Science and Technology Innovation Program of Sichuan Province (23-YCG034). The funders had no role in study design, data collection and analysis, decision to publish, or preparation of the manuscript. The authors have no other conflicts of interest to declare.
Ethical Statement:
Open Access Statement: This is an Open Access article distributed in accordance with the Creative Commons Attribution-NonCommercial-NoDerivs 4.0 International License (CC BY-NC-ND 4.0), which permits the non-commercial replication and distribution of the article with the strict proviso that no changes or edits are made and the original work is properly cited (including links to both the formal publication through the relevant DOI and the license). See: https://creativecommons.org/licenses/by-nc-nd/4.0/.
References
- Ganti AK, Klein AB, Cotarla I, et al. Update of Incidence, Prevalence, Survival, and Initial Treatment in Patients With Non-Small Cell Lung Cancer in the US. JAMA Oncol 2021;7:1824-32. [Crossref] [PubMed]
- Lu T, Yang X, Huang Y, et al. Trends in the incidence, treatment, and survival of patients with lung cancer in the last four decades. Cancer Manag Res 2019;11:943-53. [Crossref] [PubMed]
- Christopoulos P. Unravelling the puzzle of immunotherapeutic efficacy in lung cancer. Transl Lung Cancer Res 2024;13:1173-6. [Crossref] [PubMed]
- Jiang K, Parker M, Materi J, et al. Epidemiology and survival outcomes of synchronous and metachronous brain metastases: a retrospective population-based study. Neurosurg Focus 2023;55:E3. [Crossref] [PubMed]
- Gillespie CS, Mustafa MA, Richardson GE, et al. Genomic Alterations and the Incidence of Brain Metastases in Advanced and Metastatic NSCLC: A Systematic Review and Meta-Analysis. J Thorac Oncol 2023;18:1703-13. [Crossref] [PubMed]
- Jiang C, Zhao M, Hou S, et al. The Indicative Value of Serum Tumor Markers for Metastasis and Stage of Non-Small Cell Lung Cancer. Cancers (Basel) 2022;14:5064. [Crossref] [PubMed]
- Zhang Z, Yuan F, Chen R, et al. Dynamics of Serum Tumor Markers Can Serve as a Prognostic Biomarker for Chinese Advanced Non-small Cell Lung Cancer Patients Treated With Immune Checkpoint Inhibitors. Front Immunol 2020;11:1173. [Crossref] [PubMed]
- Dal Bello MG, Filiberti RA, Alama A, et al. The role of CEA, CYFRA21-1 and NSE in monitoring tumor response to Nivolumab in advanced non-small cell lung cancer (NSCLC) patients. J Transl Med 2019;17:74. [Crossref] [PubMed]
- Li Q, Sang S. Diagnostic Value and Clinical Significance of Combined Detection of Serum Markers CYFRA21-1, SCC Ag, NSE, CEA and ProGRP in Non-Small Cell Lung Carcinoma. Clin Lab 2020;
- van den Heuvel M, Holdenrieder S, Schuurbiers M, et al. Serum tumor markers for response prediction and monitoring of advanced lung cancer: A review focusing on immunotherapy and targeted therapies. Tumour Biol 2024;46:S233-68. [Crossref] [PubMed]
- Li C, Zhang D, Pang X, et al. Trajectories of Perioperative Serum Tumor Markers and Colorectal Cancer Outcomes: A Retrospective, Multicenter Longitudinal Cohort Study. EBioMedicine 2021;74:103706. [Crossref] [PubMed]
- Limonte CP, Gao X, Bebu I, et al. Longitudinal Trajectories of Biomarkers of Kidney Tubular Function in Type 1 Diabetes. Kidney Int Rep 2024;9:1406-18. [Crossref] [PubMed]
- Yu J, Zhang Y, Liu Z, et al. Association of smoking with the survival of patients with brain metastasis of lung cancer. Front Neurol 2023;14:1036387. [Crossref] [PubMed]
- Arrieta O, Saavedra-Perez D, Kuri R, et al. Brain metastasis development and poor survival associated with carcinoembryonic antigen (CEA) level in advanced non-small cell lung cancer: a prospective analysis. BMC Cancer 2009;9:119. [Crossref] [PubMed]
- Guo D, Jing W, Zhu H, et al. Clinical value of carcinoembryonic antigen for predicting the incidence of brain metastases and survival in small cell lung cancer patients treated with prophylactic cranial irradiation. Cancer Manag Res 2018;10:3199-205. [Crossref] [PubMed]
- Berghoff AS, Bartsch R, Wöhrer A, et al. Predictive molecular markers in metastases to the central nervous system: recent advances and future avenues. Acta Neuropathol 2014;128:879-91. [Crossref] [PubMed]
- Shen E, Van Swearingen AED, Price MJ, et al. A Need for More Molecular Profiling in Brain Metastases. Front Oncol 2021;11:785064. [Crossref] [PubMed]
- Popat S, Ahn MJ, Ekman S, et al. Osimertinib for EGFR-Mutant Non-Small-Cell Lung Cancer Central Nervous System Metastases: Current Evidence and Future Perspectives on Therapeutic Strategies. Target Oncol 2023;18:9-24. [Crossref] [PubMed]
- Rangachari D, Yamaguchi N, VanderLaan PA, et al. Brain metastases in patients with EGFR-mutated or ALK-rearranged non-small-cell lung cancers. Lung Cancer 2015;88:108-11. [Crossref] [PubMed]
- Sperduto PW, Berkey B, Gaspar LE, et al. A new prognostic index and comparison to three other indices for patients with brain metastases: an analysis of 1,960 patients in the RTOG database. Int J Radiat Oncol Biol Phys 2008;70:510-4. [Crossref] [PubMed]
- Fang L, Zhao W, Ye B, et al. Combination of Immune Checkpoint Inhibitors and Anti-Angiogenic Agents in Brain Metastases From Non-Small Cell Lung Cancer. Front Oncol 2021;11:670313. [Crossref] [PubMed]
- Nishino M, Soejima K, Mitsudomi T. Brain metastases in oncogene-driven non-small cell lung cancer. Transl Lung Cancer Res 2019;8:S298-307. [Crossref] [PubMed]
- Pan K, Concannon K, Li J, et al. Emerging therapeutics and evolving assessment criteria for intracranial metastases in patients with oncogene-driven non-small-cell lung cancer. Nat Rev Clin Oncol 2023;20:716-32. [Crossref] [PubMed]
- Nelson TA, Wang N. Targeting lung cancer brain metastases: a narrative review of emerging insights for anaplastic lymphoma kinase (ALK)-positive disease. Transl Lung Cancer Res 2023;12:379-92. [Crossref] [PubMed]