DeepGR: a deep-learning prognostic model based on glycolytic radiomics for non-small cell lung cancer
Highlight box
Key findings
• Elevated glycolysis levels are associated with poorer survival outcomes in non-small cell lung cancer (NSCLC) patients.
• This study identified 11 glycolysis-related radiomic features using the least absolute shrinkage and selection operator (LASSO) algorithm.
• A deep-learning prognostic model (the DeepGR model) that integrated computed tomography (CT) radiomics and clinical features demonstrated significant predictive accuracy with area under the curve values of 0.8760 and 0.8259 in different cohorts.
• High-risk DeepGR scores were linked to poor overall survival (OS), resting memory CD4+ T cells, and higher response rates to immunotherapy.
What is known, and what is new?
• Glycolysis has prognostic value in NSCLC, but associated genomic markers are costly and difficult to identify.
• This study identified glycolysis-related CT radiomic features and developed the DeepGR model, which effectively predicted NSCLC prognosis, and was correlated with the immune response and survival outcomes.
What is the implication, and what should change now?
• The DeepGR model provides a non-invasive, cost-effective method for predicting NSCLC prognosis and identifying patients likely to benefit from immunotherapy.
• The DeepGR model should be integrated routinely into clinical practice to improve the prognosis of and treatment planning for NSCLC patients. The DeepGR model could potentially guide personalized treatment decision making based on radiomic analysis.
Introduction
Lung cancer ranks among the most frequently diagnosed cancers and remains the primary cause of cancer-related mortality (1). Non-small cell lung cancer (NSCLC), the predominant histological subtype, constitutes 85% of all lung cancer cases (2). Lung cancer is also the most common cancer in China (3). The precise stratification of patients with NSCLC based on survival is crucial for effective treatment. Due to significant heterogeneity in survival rates among individuals (4), improving the overall clinical outcomes of patients is essential (5). Thus, there is an urgent need to develop effective prognostic models to predict overall survival and to guide clinical practice.
Targeting enhanced glycolysis in cancer cells increases susceptibility to various conventional treatment modalities, such as chemotherapy, radiotherapy, hormonal therapy, immunotherapy, and photodynamic therapy (6). Similarly, immune cells in the tumor microenvironment transition to a glycolytic metabolic profile, fostering competition between cancer cells and infiltrating cells for nutrients (7). Termed the “Warburg effect”, this phenomenon warrants a survival advantage to cancer cells, and fosters a tumor microenvironment towards cancer progression (8). After undergoing metabolic reprogramming, cancer cells switch to a “glycolysis-dominant” profile that promotes survival and meets energy and macromolecular needs. Moreover, the metabolic switch from oxidative phosphorylation to glycolysis regulates the invasion-metastasis cascade by promoting epithelial-mesenchymal transition, tumor angiogenesis and the metastatic colonization of distant organs. Metastases, which are often difficult to treat, are the leading cause of cancer-related mortality. Advanced NSCLC is particularly prone to metastasis, resulting in severe symptoms and reduced overall survival. The presence of distant metastases is a key indicator of a poor prognosis (9). Although studies have investigated the role of glycolysis in NSCLC development and clinical outcomes, comprehensive investigations in this area are still missing.
Deep-learning networks aim at discerning intricate relationships between prognostic clinical features and an individual’s risk of mortality, and thus could be used to make tailored recommendations based on risk assessments (10). A recent study employed computerized methods, including random forests, least absolute shrinkage and selection operator (LASSO) regression, and neural networks. An over 90% accuracy in predicting lung cancer stages was achieved (11). Another study developed a deep-learning network model that surpassed the traditional proportional hazard regression model in analyzing progression-free survival (12).
In the field of cancer diagnosis, the features obtained from imaging data can serve as crucial biomarkers, offering diagnostic, predictive, and prognostic insights through their correlations with pathological or molecular references, treatment responses, and survival outcomes (13). This approach, known as radiomics, involves extracting a range of features from target lesions. These features encompass: (I) first-order metrics such as energy, minimum, maximum, mean, median, interquartile range and standard deviation; (II) shape characteristics including volume, surface area, and sphericity; and (III) higher-order statistical texture measures, which involve analyses like Gray-Level Co-occurrence Matrix (GLCM), Gray-Level Run Length Matrix (GLRLM) and Neighboring-Gray Tone Difference Matrix (NGTDM). In this domain, extracted image features, such as lesion volume, shape, and texture descriptors, are harnessed as predictive tools (14). Conversely, “radiogenomics” explores the integration of imaging-derived parameters and genomic data to uncover clinically relevant associations (15). This methodology not only captures comprehensive information from entire tumor lesions and facilitates ongoing treatment monitoring at various intervals also. Moreover, it is particularly advantageous when biopsy is unfeasible or not available (16). Nonetheless, the application of deep learning to radiomic features for prognostic prediction is still in its early stages.
This aim of this study is to develop a deep-learning prognostic model based on glycolysis-related radiomic features. Additionally, the relationship between the model and the immune response was analyzed. The results showed that the glycolysis-related radiomic deep-learning model could predict the prognosis of NSCLC patients and thus could potentially guide immunotherapy strategies. We present this article in accordance with the TRIPOD reporting checklist (available at https://tlcr.amegroups.com/article/view/10.21037/tlcr-24-716/rc).
Methods
Study design
The study flowchart is shown in Figure 1. Radiomics analyses were conducted retrospectively on the following two cohorts: the Second Affiliated Hospital of Soochow University cohort (SZ cohort, n=64); and The Cancer Imaging Archive (TCIA) dataset comprising The Cancer Genome Atlas (TCGA)-NSCLC cohort (n=74) (https://www.cancerimagingarchive.net/access-data/) (17,18). The prognostic ability of the glycolysis genes was validated using cohorts from the Gene Expression Omnibus (GEO) datasets (GSE19188 and GSE87340, n=136). To be eligible for inclusion in the SZ cohort, the patients had to meet the following inclusion criteria: (I) have a diagnosis of lung squamous cell carcinoma or lung adenocarcinoma; (II) have undergone surgery or biopsy within four weeks of the computed tomography (CT) scan; and (III) have a lesion with a maximum diameter greater than 1 cm to minimize the partial volume effect. Patients were excluded from the SZ cohort if they met any of the following exclusion criteria: (I) had a history of other malignancies; (II) had incomplete case records; (III) had poor-quality images; (IV) had target lesions that were difficult to delineate on the CT scan, such as those overlapping with adjacent lesions or surrounding tissues, or diffuse lesions; and (V) were aged under 18 years. The study was conducted in accordance with the Declaration of Helsinki (as revised in 2013). The study was approved by The Medical Ethics Committee of The Second Affiliated Hospital of Soochow University (No. JD-HG-2023-63) and informed consent was taken from all the patients.
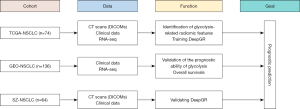
CT scan protocol
Patients in the SZ cohort underwent CT scanning using one of two multidetector CT scanners: the GE Revolution CT machine, or the Philips Brilliance iCT machine. All the scans were performed in the supine position during full inspiration. The scanning parameters were as follows: 150 kVp, 80–450 mA, detector collimation of either 64×0.625 mm or 128×0.625 mm, and a field of view of 350×350 mm. The obtained images represent a real clinical practice scenario.
CT segmentation and structuring
The CT data in the Digital Imaging and Communications in Medicine (DICOM) format of all the cohorts were imported into LIFEx software (19) (see Figure S1). Three radiologists, blinded to the patient data, outlined the primary tumor lesions on the lung window to create volumes of interest (VOIs). The VOIs were first re-sampled to 1×1×1 mm3 voxels, and then re-scaled from −1,000 to 3,000 Hounsfield units (HU) with a bin size of 10 HU. After these processes, 201 radiomic features were extracted, including the shape features, first-order statistics, and second-order statistics from four gray-level matrices. Manual image labeling reproducibility was assessed using the intraclass correlation coefficient (ICC) for intra-observer agreement. Radiomic features with an ICC value <0.75 were deemed poorly reproducible and excluded from the study. Ultimately, 155 radiomic features were included in the study.
Identification of glycolysis-related radiomic features in NSCLC
A single-sample gene set enrichment analysis (ssGSEA) was conducted to evaluate the glycolysis enrichment scores. The NSCLC patients were divided into low- and high-glycolysis groups using the optimal threshold determined by the Survminer package (https://rdocumentation.org/packages/survminer/versions/0.4.9). The glycolysis genes were obtained from the Molecular Signatures Database (MSigDB; https://www.gsea-msigdb.org/gsea/msigdb/; see Box S1). Kaplan-Meier curves and log-rank tests were used to compare OS between the glycolysis groups of NSCLC patients. The LASSO algorithm, which is known for its efficacy in handling high-dimensional collinear data, was used to extract predictive features after data splitting. A Python package was developed to implement the LASSO regression model for fitting and prediction (alpha =1.0, max_iter =1,000, seed =12,345). The diagnostic performance of the model was evaluated using receiver operating characteristic (ROC) curves that were generated with the ROCR 1.1.0 package in R.
Construction and validation of a deep-learning model based on glycolysis-related radiomics (DeepGR)
A deep neural network model with 155 glycolysis-related radiomics and clinical features was created. Notably, considering that the smoking status was not available in the TCGA dataset, this clinical feature was not included in the deep-learning model. The model had an architecture consisting of one input layer, three hidden layers, and one output layer. The model was trained using the TensorFlow framework (https://github.com/tensorflow/tensorflow) with a Tanh activation function and stochastic gradient descent optimizer. To prevent overfitting, a hybrid L1 and L2 regularization method, combined with dropout, was employed. Bayesian optimization was used to fine-tune the hyperparameters, resulting in a learning rate of 0.92, a decay rate of 0.999, and a dropout rate of 0.08. The model architecture is depicted in Figure 2. The prognostic performance of different models was validated using area under the curve (AUC) values. Risk estimates and confidence intervals (CIs) were corrected for regression dilution bias using a non-parametric method. P values for linear trends and interactions between the DeepGR scores and survival outcomes were tested. Interaction P values were derived using the Wald test.
Biological function analysis of the DeepGR model
The patients in TCGA cohort were divided into high- and low-risk subgroups based on the DeepGR coefficients. The restricted cubic spline function from Hmisc (https://cran.r-project.org/web/packages/Hmisc, version 5.1.0) was used to explore the nonlinear relationship between our predictor and clinical outcomes. The differentially expressed genes (DEGs) in these subgroups were identified using Deseq2 based on the following criteria: log fold change >2, and P<0.05. A biological analysis was performed using the Kyoto Encyclopedia of Genes and Genomes (KEGG) and Gene Ontology (GO) databases, and the results were visualized using the clusterProfiler (20) (version 4.0) and graph (version 2.1.0) packages. The protein-protein interaction network from the Search Tool for the Retrieval of Interacting Genes/Proteins (STRING) was analyzed and visualized using the network (version 1.18.2) and igraph (version 1.5.1) packages. The prognostic capabilities of the models were examined using Kaplan-Meier curves and log-rank tests.
Assessment of immune cells infiltration of DeepGR
The CIBERSORT algorithm was used to quantify the infiltration levels of the immune cells (22 classes) in both the high- and low-risk DeepGR groups. In this phase, normalized gene expression data were analyzed in conjunction with the LM22 signature and 1,000 permutations. A lollipop graph depicted the correlation between the immune cells and the DeepGR model. Box plots were created using immune scores from both DeepGR groups.
Prediction of the immunotherapy response
TCIA database has comprehensive immunogenomic analysis capabilities. Cancer immunogenicity was quantified on a scale of 0 to 10, known as the immunophenoscore (IPS). The response to immune checkpoint inhibitors (ICIs) in both the high- and low-risk DeepGR groups was assessed using the IPS.
Statistical analyses
GraphPad Prism (version 9.0), Python (version 3.7), Figdraw (www.figdraw.com) and R software (version 3.5.1) were used for the statistical analyses and visualizations. The survival (version 3.5) and survminer (version 0.4.9) packages of R software were used for the survival analysis and to plot the results. A significance level of P<0.05 was adopted.
Results
Figure 3 illustrates the study design, which involved 138 patients with CT data from two NSCLC cohorts (TCGA and SZ). After annotating regions of interest (ROIs), processing the data, and selecting features, glycolysis-related radiomic features were extracted and combined with clinical variables. This integration was used to develop a deep learning model, DeepGR, which aims to predict survival outcomes for NSCLC patients. Furthermore, the biological underpinnings of the model were elucidated through the integration of RNA-seq data.
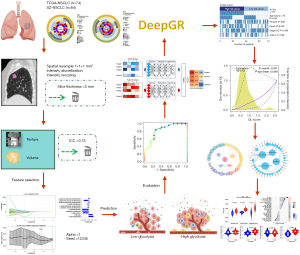
Baseline information
The characteristics of the enrolled patients are shown in Figure 4A,4B. Specifically, the SZ cohort had a slightly higher percentage of women (39/64), lung adenocarcinoma cases (41/64), and ever-smokers (44/64). While TCGA cohort had a significant number of elderly patients (age ≥70 years, 42/74), females (41/74), and lung squamous cell carcinoma cases (40/74).
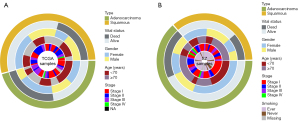
Survival analysis and cut-off calculation of glycolysis scores
A ssGSEA was conducted to calculate the glycolysis enrichment scores for samples from TCGA cohort and the two GEO cohorts based on the glycolysis gene sets. The optimal cut-off values for the glycolysis scores were determined to enhance the discriminative ability of predicting survivals, allowing the NSCLC patients from TCGA (cutoff =2), GSE19188 (cutoff =0.43), and GSE87340 (cutoff =2.46) datasets to be classified into low- and high-glycolysis groups (Figure 5A-5C). High-glycolysis levels were associated with poorer survival outcomes in all three cohorts (Figure 5D-5F), with P=0.04, P<0.001 and P<0.001, respectively.
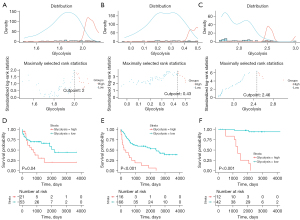
Glycolysis-related radiomic features
The LASSO regression model was used to select glycolysis-related radiomic features (see Figure 6A,6B). The following 11 radiomic features were selected to construct the radiomic signature: CentreOfMassShift, Uniformity, JointMaximum, AngularSecondMoment, LongRunsEmphasis, LongRunLowGreyLevelEmphasis, LongRunHighGreyLevelEmphasis, LargeZoneEmphasis, SmallZoneHighGreyLevelEmphasis, LargeZoneLowGreyLevelEmphasis, and LargeZoneHighGreyLevelEmphasis. The coefficient of each feature is shown in Figure 6C. The ROC curve showed good discriminative ability (see Figure 6D) with an AUC of 0.8442.
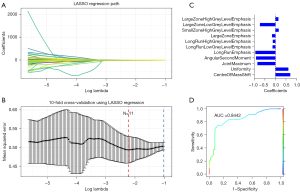
Evaluation of the DeepGR model
The performance of the DeepGR model was evaluated by plotting survival curves and AUCs (see Figure 7A,7B). The patients in the high-risk group exhibited poorer survival outcomes than those in the low-risk group (with both P<0.001). Additionally, the AUCs of the DeepGR model outperformed those of clinical features or radiomic features alone with values of 0.8760 (sensitivity: 0.7787, specificity: 0.7617, precision: 0.7570 and recall: 0.7787) and 0.8259 (sensitivity: 0.7131, specificity: 0.7446, precision: 0.7324 and recall: 0.7131) in the SZ and TCGA cohorts, respectively (see Figure 7C,7D). The risk scores, shown in Figure 7E using restricted cubic splines, exhibited a U-shaped association with survival risk, indicating that low and high-risk groups have different clinical outcomes. The calibration plot was drawn to provide a visual representation of how well the predicted probabilities align with the observed outcomes (see Figure S2).
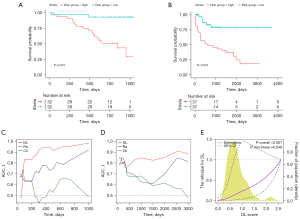
Relationship between DeepGR grouping and clinical features
Heatmaps were plotted to explore any differences in the clinical features between the high- and low-risk DeepGR groups (see Figure 8). There were significant differences observed between the two cohorts in terms of the deceased status (23 dead in 37 patients in the high-risk group, P=0.002 and 18 dead in 32 patients in the high-risk group, P<0.001), providing further evidence of the prognostic capabilities of the DeepGR model.
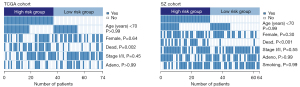
Biological function of the DeepGR
To enhance the interpretability of the DeepGR, we calculated the risk scores for TCGA samples and stratified the patients into high- and low-risk groups. We identified 829 DEGs (of which 374 were upregulated and 455 were downregulated) (see Figure 9A). The network analysis revealed 11 modules (see Figure 9B). The GO analysis primarily indicated enrichment of the glycolysis process, metabolic pathways, intermediate filament, and channel activity (see Figure 9C). The KEGG analysis demonstrated that the DEGs were primarily enriched in metabolism-related pathways, such as carbohydrate metabolism and lipid metabolism (see Figure 9D).
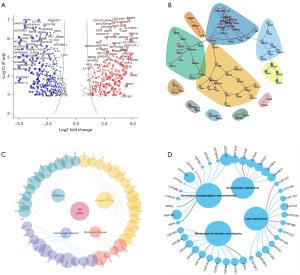
Immune heterogeneity of the DeepGR
The ESTIMATE score, immune score, and stromal score were positively correlated with an increasing DeepGR risk score (see Figure 10A). The analysis with the CIBERSORT algorithm revealed a positive association between resting memory CD4+ T cells, monocytes, and resting dendritic cells, and DeepGR. Conversely, M0 (resting) macrophages and T follicular helper cells revealed a negative association with DeepGR (see Figure 10B). Additionally, using the TCIA, we assessed patients’ susceptibility to immunotherapy. We observed that high-risk DeepGR patients had a higher IPS in programmed cell death protein 1 (PD-1) positive cases, implying their potential increased sensitivity to PD-1 targeted ICIs (see Figure 10C-10F).
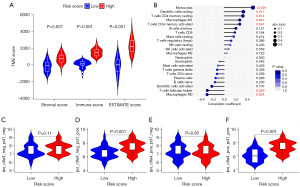
Discussion
Targeting glycolysis and cancer cell-specific biosynthetic pathways is a promising area of focus in lung cancer research (21). While the improved glycolytic dependency of neoplastic cells hints at the potential therapeutic efficacy of glycolytic inhibitors in lung cancer treatment, clinical glycolytic inhibition remains ineffective due to the absence of efficient glycolysis biomarkers to date (22). Therefore, the prompt detection of glycolysis status is pivotal for selecting appropriate glycolytic inhibitors in NSCLC patients. Traditional methods are invasive, and thus are biased by limitations such as sampling challenges, tissue availability constraints, and spatial tumor heterogeneity. Non-invasive radiomic features have been shown to be able to predict molecular characteristics potentially with an effective performance (23). This study introduced the DeepGR model, a deep-learning model designed to non-invasively predict the glycolytic status of NSCLC patients, which showed a superior efficiency with an AUC of 0.876.
Metabolic modulation has been shown to influence cancer survival by sensitizing cancer cells to chemotherapy and radiotherapy (24). Increased glycolysis, driven by heightened glucose uptake, serves as the primary energy source for cancer cells and provides essential macromolecules for cell proliferation and survival. Targeted glycolytic therapy may enhance the clinical treatment of tumor patients through its combination with other therapeutic approaches (25). A recent study explored the potential of targeting glycolysis, noting that enhanced glycolysis in cancer cells could improve their sensitivity to conventional treatments such as chemotherapy, radiotherapy, hormonal therapy, immunotherapy, and photodynamic therapy (6). Additionally, metabolic reprogramming in cancer cells is a key factor contributing to the diminished effectiveness of immunotherapy (26). Moreover, it has been suggested that metabolic interventions significantly enhance the effectiveness of immunotherapy. Thus, identifying glycolysis status could help predicting and enhancing the clinical outcomes of lung cancer patients.
Several studies have been tested successfully in predicting survival outcomes in different cancers, including pancreatic cancer (27), colon adenocarcinoma (28), bladder cancer (29), and laryngeal cancer (30), based on glycolysis genes. However, detecting genomic markers related to glycolysis is quite expensive and challenging. Thus, this study searched for characterized glycolysis markers to predict the survival outcomes of NSCLC patients using radiomic features. Previous studies have investigated predictive radiomic features for lung cancer. For example, Fave et al. used delta-radiomic features to predict outcomes in stage-III NSCLC patients undergoing radiation therapy (31). Another study positively identified clusters of radiomic features associated with lung cancer prognosis (32). Song et al. also described a correlation between CT radiomic features and OS in NSCLC patients (33). Researchers also demonstrated that radiomic features extracted from PET/CT images can effectively predict the risk of metastasis in NSCLC patients (34). One novel commentary discussed that navigating the prediction of disease remains a challenge at the intersection of radiomics and deep learning (35). A recent meta-analysis demonstrated that AI algorithms, particularly deep learning, using radiomics features can significantly enhance the prediction of EGFR mutation status in NSCLC (36).
Despite the promising therapeutic efficacy of glycolytic inhibitors in lung cancer treatment, no radiomic features are currently available for predicting glycolysis status. In the study, 11 selected glycolysis-related radiomic features capture various aspects of tumor heterogeneity and microenvironmental characteristics, which are closely linked to the biological behavior of NSCLC. For instance, CentreOfMassShift indicates asymmetry in tumor growth, reflecting uneven cellular proliferation. Uniformity and AngularSecondMoment are texture-based features that measure the homogeneity of pixel intensities, potentially correlating with the consistency of glycolytic activity across the tumor. LongRunsEmphasis and related features (e.g., LongRunLowGreyLevelEmphasis, LargeZoneHighGreyLevelEmphasis) capture the distribution of voxel intensities in specific patterns, which might reflect the metabolic state and structural complexity of the tumor. These features together provide a comprehensive radiomic signature that can offer insights into the underlying glycolytic processes and tumor aggressiveness, ultimately contributing to the prediction of patient outcomes. The connection between the selected radiomic features and immune responses is an area of growing interest, particularly in understanding their role in predicting sensitivity to immunotherapy. Features like CentreOfMassShift and Uniformity may be associated with the spatial distribution of immune cells within the tumor microenvironment, reflecting how immune infiltration varies across different tumor regions. Texture features such as AngularSecondMoment and LongRunsEmphasis might correlate with the density and arrangement of immune cells, potentially indicating areas of immune activity or evasion. Exploring these connections could provide valuable insights into how these radiomic signatures not only reflect tumor biology but also predict the tumor’s response to immunotherapy.
This study leveraged glycolysis-related radiomics to develop a deep neural network model called the DeepGR model. Subsequently, we identified the DEGs between the DeepGR-low and DeepGR-high groups. The DeepGR-high group showed a more favorable response to PD-1 immunotherapy, characterized by a significant infiltration of resting memory CD4+ T cells, monocytes, and dendritic resting cells, that had not previously been reported. Glycolysis may have distinct effects on immunotherapy response, and thus deserves further investigation. Additionally, the genomic network analysis and KEGG pathway enrichment analysis revealed the significant enrichment of metabolic functions in the DeepGR. In clinical practice, there will be a three-step workflow to facilitate its practical application: clinicians first gather clinical information and perform CT annotation; next, the model’s risk scores are computed using LifeX and our deep-learning framework; and finally, the model provides output on predicted survival outcomes. While the DeepGR model has shown strong performance in predicting prognosis in NSCLC patients, we acknowledge the importance of further external validation. In future work, we plan to expand validation with data from multiple centers to further strengthen the model’s generalizability.
This study had several limitations. First, variations between devices might have influenced the outcomes, even though we restricted image acquisition to two CT systems and preprocessed them before segmentation. Moreover, the process of semi-automatic tumor segmentation proved to be time-consuming for the operators. Besides, the restricted sample size emphasizes the necessity for additional prospective studies to support the generalizability and robustness of the constructed model. Finally, this study may not fully capture the heterogeneity of NSCLC across different demographics, clinical settings, or imaging platforms. Future work will aim to incorporate data from more diverse populations to enhance the model’s generalizability and to mitigate overfitting.
Conclusions
A novel approach to assess glycolysis status by integrating radiomics and deep-learning models is proposed. This approach could aid clinicians in the near future to optimize treatment strategies and to minimize invasive sample harvesting in NSCLC patients. The results are surprising but a more standardized procedure must be trained before promoting translation into routine clinical practice.
Acknowledgments
Funding: The study was funded by
Footnote
Reporting Checklist: The authors have completed the TRIPOD reporting checklist. Available at https://tlcr.amegroups.com/article/view/10.21037/tlcr-24-716/rc
Data Sharing Statement: Available at https://tlcr.amegroups.com/article/view/10.21037/tlcr-24-716/dss
Peer Review File: Available at https://tlcr.amegroups.com/article/view/10.21037/tlcr-24-716/prf
Conflicts of Interest: All authors have completed the ICMJE uniform disclosure form (available at https://tlcr.amegroups.com/article/view/10.21037/tlcr-24-716/coif). The authors have no conflicts of interest to declare.
Ethical Statement: The authors are accountable for all aspects of the work in ensuring that questions related to the accuracy or integrity of any part of the work are appropriately investigated and resolved. The study was conducted in accordance with the Declaration of Helsinki (as revised in 2013). The study was approved by the Medical Ethics Committee of the Second Affiliated Hospital of Soochow University (No. JD-HG-2023-63) and informed consent was taken from all the patients.
Open Access Statement: This is an Open Access article distributed in accordance with the Creative Commons Attribution-NonCommercial-NoDerivs 4.0 International License (CC BY-NC-ND 4.0), which permits the non-commercial replication and distribution of the article with the strict proviso that no changes or edits are made and the original work is properly cited (including links to both the formal publication through the relevant DOI and the license). See: https://creativecommons.org/licenses/by-nc-nd/4.0/.
References
- Dela Cruz CS, Tanoue LT, Matthay RA. Lung cancer: epidemiology, etiology, and prevention. Clin Chest Med 2011;32:605-44. [Crossref] [PubMed]
- Inamura K. Lung Cancer: Understanding Its Molecular Pathology and the 2015 WHO Classification. Front Oncol 2017;7:193. [Crossref] [PubMed]
- Chen P, Liu Y, Wen Y, et al. Non-small cell lung cancer in China. Cancer Commun (Lond) 2022;42:937-70. [Crossref] [PubMed]
- Casal-Mouriño A, Ruano-Ravina A, Lorenzo-González M, et al. Epidemiology of stage III lung cancer: frequency, diagnostic characteristics, and survival. Transl Lung Cancer Res 2021;10:506-18. [Crossref] [PubMed]
- Huang S, Yang J, Shen N, et al. Artificial intelligence in lung cancer diagnosis and prognosis: Current application and future perspective. Semin Cancer Biol 2023;89:30-7. [Crossref] [PubMed]
- Chelakkot C, Chelakkot VS, Shin Y, et al. Modulating Glycolysis to Improve Cancer Therapy. Int J Mol Sci 2023;24:2606. [Crossref] [PubMed]
- Vander Heiden MG, Cantley LC, Thompson CB. Understanding the Warburg effect: the metabolic requirements of cell proliferation. Science 2009;324:1029-33. [Crossref] [PubMed]
- Liberti MV, Locasale JW. The Warburg Effect: How Does it Benefit Cancer Cells? Trends Biochem Sci 2016;41:211-8. [Crossref] [PubMed]
- Popper HH. Progression and metastasis of lung cancer. Cancer Metastasis Rev 2016;35:75-91. [Crossref] [PubMed]
- Calderaro J, Seraphin TP, Luedde T, et al. Artificial intelligence for the prevention and clinical management of hepatocellular carcinoma. J Hepatol 2022;76:1348-61. [Crossref] [PubMed]
- Huang S, Yang J, Fong S, et al. Artificial intelligence in cancer diagnosis and prognosis: Opportunities and challenges. Cancer Lett 2020;471:61-71. [Crossref] [PubMed]
- Chang R, Qi S, Wu Y, et al. Nomograms integrating CT radiomic and deep learning signatures to predict overall survival and progression-free survival in NSCLC patients treated with chemotherapy. Cancer Imaging 2023;23:101. [Crossref] [PubMed]
- Bera K, Braman N, Gupta A, et al. Predicting cancer outcomes with radiomics and artificial intelligence in radiology. Nat Rev Clin Oncol 2022;19:132-46. [Crossref] [PubMed]
- Binczyk F, Prazuch W, Bozek P, et al. Radiomics and artificial intelligence in lung cancer screening. Transl Lung Cancer Res 2021;10:1186-99. [Crossref] [PubMed]
- Liu Z, Duan T, Zhang Y, et al. Radiogenomics: a key component of precision cancer medicine. Br J Cancer 2023;129:741-53. [Crossref] [PubMed]
- Hannequin P, Decroisette C, Kermanach P, et al. FDG PET and CT radiomics in diagnosis and prognosis of non-small-cell lung cancer. Transl Lung Cancer Res 2022;11:2051-63. [Crossref] [PubMed]
- Kirk S, Lee Y, Kumar P, et al. The Cancer Genome Atlas Lung Squamous Cell Carcinoma Collection (TCGA-LUSC) (Version 4). The Cancer Imaging Archive 2016. doi:
10.7937/K9/TCIA.2016.TYGKKFMQ . - Albertina B, Watson M, Holback C, et al. The Cancer Genome Atlas Lung Adenocarcinoma Collection (TCGA-LUAD) (Version 4). The Cancer Imaging Archive 2016. doi:
10.7937/K9/TCIA.2016.JGNIHEP5 . - Nioche C, Orlhac F, Boughdad S, et al. LIFEx: A Freeware for Radiomic Feature Calculation in Multimodality Imaging to Accelerate Advances in the Characterization of Tumor Heterogeneity. Cancer Res 2018;78:4786-9. [Crossref] [PubMed]
- Wu T, Hu E, Xu S, et al. clusterProfiler 4.0: A universal enrichment tool for interpreting omics data. Innovation (Camb) 2021;2:100141. [Crossref] [PubMed]
- Weiss JM. The promise and peril of targeting cell metabolism for cancer therapy. Cancer Immunol Immunother 2020;69:255-61. [Crossref] [PubMed]
- Feng Y, Xiong Y, Qiao T, et al. Lactate dehydrogenase A: A key player in carcinogenesis and potential target in cancer therapy. Cancer Med 2018;7:6124-36. [Crossref] [PubMed]
- Lambin P, Leijenaar RTH, Deist TM, et al. Radiomics: the bridge between medical imaging and personalized medicine. Nat Rev Clin Oncol 2017;14:749-62. [Crossref] [PubMed]
- Ganapathy-Kanniappan S, Geschwind JF. Tumor glycolysis as a target for cancer therapy: progress and prospects. Mol Cancer 2013;12:152. [Crossref] [PubMed]
- Zhou D, Duan Z, Li Z, et al. The significance of glycolysis in tumor progression and its relationship with the tumor microenvironment. Front Pharmacol 2022;13:1091779. [Crossref] [PubMed]
- Xia L, Oyang L, Lin J, et al. The cancer metabolic reprogramming and immune response. Mol Cancer 2021;20:28. [Crossref] [PubMed]
- Yan L, Raj P, Yao W, et al. Glucose Metabolism in Pancreatic Cancer. Cancers (Basel) 2019;11:1460. [Crossref] [PubMed]
- Liu G, Wu X, Chen J. Identification and validation of a glycolysis-related gene signature for depicting clinical characteristics and its relationship with tumor immunity in patients with colon cancer. Aging (Albany NY) 2022;14:8700-18. [Crossref] [PubMed]
- Lv J, Li K, Yu H, et al. HNRNPL induced circFAM13B increased bladder cancer immunotherapy sensitivity via inhibiting glycolysis through IGF2BP1/PKM2 pathway. J Exp Clin Cancer Res 2023;42:41. [Crossref] [PubMed]
- Ding Z, Yu D, Li H, et al. Prognostic Correlation of Glycolysis-Related Gene Signature in Patients with Laryngeal Cancer. Am J Med Sci 2021;362:161-72. [Crossref] [PubMed]
- Fave X, Zhang L, Yang J, et al. Delta-radiomics features for the prediction of patient outcomes in non-small cell lung cancer. Sci Rep 2017;7:588. [Crossref] [PubMed]
- Vallières M, Kay-Rivest E, Perrin LJ, et al. Radiomics strategies for risk assessment of tumour failure in head-and-neck cancer. Sci Rep 2017;7:10117. [Crossref] [PubMed]
- Song J, Shi J, Dong D, et al. A New Approach to Predict Progression-free Survival in Stage IV EGFR-mutant NSCLC Patients with EGFR-TKI Therapy. Clin Cancer Res 2018;24:3583-92. [Crossref] [PubMed]
- Wilk AM, Kozłowska E, Borys D, et al. Radiomic signature accurately predicts the risk of metastatic dissemination in late-stage non-small cell lung cancer. Transl Lung Cancer Res 2023;12:1372-83. [Crossref] [PubMed]
- Le NQK. Hematoma expansion prediction: still navigating the intersection of deep learning and radiomics. Eur Radiol 2024;34:2905-7. [Crossref] [PubMed]
- Nguyen HS, Ho DKN, Nguyen NN, et al. Predicting EGFR Mutation Status in Non-Small Cell Lung Cancer Using Artificial Intelligence: A Systematic Review and Meta-Analysis. Acad Radiol 2024;31:660-83. [Crossref] [PubMed]