Microbiota modulate immune repertories in lung adenocarcinoma via microbiota-immunity interactive network
Highlight box
Key findings
• We have discovered a novel microbiota-immunity interaction, elucidating its potential contribution to unfavorable overall survival.
What is known and what is new?
• Stage II–IV lung cancer patients can benefit from immunotherapy. Tumor microbiota has emerged as a regulatory mechanism for reshaping the immune environment, thereby influencing the efficacy of immune checkpoint inhibitors and patient survival.
• We identified a novel interaction between microbial biomarkers and immune cells in the poor prognostic molecular subtypes.
What is the implication, and what should change now?
• Our findings elucidate the potential contribution of the microbiota-immunity correlation network to poor overall survival and therapeutic resistance.
Introduction
The tumor resident-microbiota constitutes an intrinsic component within the tumor microenvironment, interacting with immune and epithelial cells across various types of cancer (1-4), suggesting that the intratumor microbiota can reside intracellularly (4-6). In vitro and preclinical animal models highlight the role of the intratumor microbiota in tumor progression (7-9), immunosurveillance (10) and chemoresistance (11,12).
The lower respiratory tract of healthy individuals harbor diverse bacterial communities (13). Accumulating research indicates significant disparities in lung microbiota between lung cancer patients and healthy subjects, including variations in bacterial species diversity as well as prevalence of specific bacterial groups (14,15). Jin et al. investigated the mechanisms underlying how lung microbiota promotes proliferation of lung cancer cells through inflammation augmentation and stimulation of γδT cell proliferation and activation (10). Their findings highlight the crucial role played by lung microbiota in orchestrating local inflammatory responses and promoting tumors.
Lung cancer is currently the leading cause of global cancer-related mortality, with lung adenocarcinoma (LUAD) being its most prevalent pathological type (16,17). The enrichment of Aspergillus sydowii fungi within tumors from patients with lung LUAD is associated with immunosuppression and disease progression (18). The interplay between tumor microbiota and microenvironment holds significant implications for tumor progression and response to immunotherapy treatment (19). Nevertheless, there remains a dearth of comprehension regarding the diverse molecular subtypes of tumors and how the microbiota interfaces with immune cell components, ultimately shaping the effectiveness of immunotherapy.
Herein focusing on LUAD specifically, we aim to delineate the complex network of interactions between microbiome markers and immune cells within the tumor microenvironment, via integrating microbiome, genomic, transcriptome, and clinical data. Our findings offer profound insights into the role of tumor resident microbiome in shaping molecular subtype immunoprofiles and patient survival outcomes. We present this article in accordance with the STROBE reporting checklist (available at https://tlcr.amegroups.com/article/view/10.21037/tlcr-24-393/rc).
Methods
Study design
We obtained the genome, transcriptome, microbiome and clinical data of LUAD patients from publicly available database, including The Cancer Genome Atlas (TCGA), cBioPortal database (20) and the publicly available dataset (ftp://ftp.microbio.me/pub/cancer_microbiome_analysis/) generated by Poore et al.
To ensure the accuracy of our comparative microbial analysis, we implemented strict inclusion criteria. Specifically, we only included LUAD patients with stage II–IV, which are the stages eligible for immunotherapy (21). Samples without microbial information were excluded from the analysis, as they had been removed from the microbiome quantification pipeline by the original authors (22). Furthermore, samples with driver mutations (including ROS1, ERBB2, MET, KRAS, ALK, RET, EGFR, BRAF) of non-small cell lung cancer (NSCLC) derived from NCCN (National Comprehensive Cancer Network) were also excluded. Following these criteria, a total of 220 eligible patients were included in the downstream analysis. The study was conducted in accordance with the Declaration of Helsinki (as revised in 2013).
Identification of molecular subtype in LUAD
The fragments per kilobase of transcript per million mapped reads (FPKM) metric was then used to standardize the read counts. Subsequently, unsupervised non-negative matrix factorization (NMF) clustering method was performed using NMF package on the metadata set (23). The values of k where the magnitude of the cophenetic correlation coefficient began to fall were chosen as the optimal number of clusters (24). We classified patients into the molecular subtype 1 (MS1) and molecular subtype 2 (MS2) according to the NMF clustering result.
Mutation and mutational signature analysis
The MAFtools was used to summarize somatic mutations (25). The deconstructSigs program (26) was used to disassemble mutational signatures using default settings. A collection of thirty cosmic signals were used for mutational signatures identification (27). Additionally, a cosine similarity analysis was performed to evaluate how similar different components and signatures were to one another. The similarity scores showed a shift from total dissimilarity to maximum similarity, with a range of 0 to 1.
Transcriptome and gene set enrichment analysis (GSEA)
We performed GSEA (28) utilizing the FPKM matrix via clusterProfiler (29). Furthermore, we used the matrix of signed multi-omic feature weights (Wsigned) to compute the normalized enrichment scores (NES) of signature gene sets (30).
Immune infiltration
To define the immunological landscape within the tumor microenvironment, we used ImmuCellAI (31) to examine 24 different immune cell types. ImmuCellAI is a tool to estimate the abundance of immune cells from RNA-Seq data, in which the 24 immune cells are comprised of 18 T-cell subtypes (including CD4_T, CD8_T, NKT, Gamma_delta, CD4_naive, Tr1, nTreg, iTreg, Th1, Th2, Th17, Tfh, CD8_naive, cytotoxic, exhausted, MAIT, Central_memory, Effector_memory) and 6 other immune cells: B cell, NK cell, monocyte cell, macrophage cell, neutrophil cell and DC cell.
Microbiota diversity
We obtained the microbe abundance data from the publicly accessible microbiota dataset, which was produced by Poore et al. (22). We imported the taxonomic data, sample metadata, and microbiota counts using the Microeco tools (32). We utilized stacked bar plots to show group averages in order to visually represent the abundant taxa. We used two important metrics, α-diversity and β-diversity, to quantify the diversity. Within a specific sample or group, α-diversity characterizes the observed quantity of distinct species (also known as richness) and their evenness. It sheds light on the evenness and microbiological richness of our LUAD samples. Conversely, β-diversity describes how different groups are from one another. It clarifies the ways in which the MS1 and MS2 groups’ microbial compositions vary from one another. To find group-specific microbial biomarkers that distinguish one group’s communities from others, we used the linear discriminant analysis (LDA) effect size (LEfSe) study. In addition to assisting us in identifying relevant indicator taxa whose abundances differ significantly across the MS1 and MS2 groups, our methodology facilitates high-dimensional comparisons (33). Lastly, we added these microbial indicators to the microbe-immune network analysis that followed.
Microbiota-immune network
To visualize the complex interactions between bacterial biomarkers at the genus level and hallmark gene sets, we utilized the Microeco toolkit (32) to evaluate the correlation matrix. To accurately capture these relationships, we employed the Spearman rank correlation coefficient. Significant microbiota-gene correlations were imported to construct a representative correlative network using Cytoscape (34). The size of each node in the visual element indicates its degree of importance. Positive and negative correlations are represented by red and blue lines in networks, respectively.
Drug sensitivity analysis
Drug sensitivity for molecular subtypes is predicted using oncoPredict (35). The half maximal inhibitory concentration (IC50), which represents the concentration of a compound or drug that can inhibit a biological process or activity by 50% under specific conditions (36), serves as an indicator of drug potency, with lower values indicating increased efficacy.
Statistical analysis
All statistical analyses were conducted utilizing the R program. To visualize and compare survival distributions between the MS1 and MS2 groups, Kaplan-Meier plots were employed, with statistical significance assessed using log-rank tests. In describing baseline characteristics, binary variables were summarized as frequencies and percentages, while numerical variables were reported as medians with their corresponding interquartile ranges. Appropriate statistical tests were applied to compare these variables between the MS1 and MS2 groups: the Pearson Chi-squared test for binary variables and the Wilcoxon rank sum test for numerical variables. Statistical significance was set at a two-sided P value threshold of <0.05.
Results
Systematic review and characteristics of the MS1 and MS2 samples
According to the schematic representation of our analysis pipeline (Figure 1A), 514 LUAD patients were evaluated for eligibility according to the selection criteria, and 220 patients in total were included. We categorized patients into two molecular subtypes via NMF method, with 132 in the MS1 group and 88 in the MS2 group (Figure 1B,1C, table available at https://cdn.amegroups.cn/static/public/tlcr-24-393-1.xlsx). Of all included patients, 152 (69%) were aged 60 years or older, 108 (49%) were female. Baseline characteristics were generally balanced between the groups (Table 1). After adjustment for covariates, the molecular subtype was an independently associated factor with higher hazards of death with median overall survival of 40.6 months [95% confidence interval (CI): 31.3–49.3] in the MS1 group (Figure 2A,2B) and 21.6 months (95% CI: 15.7–34.3) in the MS2 group [hazard ratio (HR): 1.74, 95% CI: 1.17–2.6; P=0.006] (Figure 2A,2B). The median progression-free survival was 22.6 months (95% CI: 19.0–35.8) in the MS1 group (Figure 2C,2D) compared with 22.8 months (95% CI: 12.8–35.5) in the MS2 group (HR: 1.33, 95% CI: 0.89–2.0; P=0.16) (Figure 2C,2D).
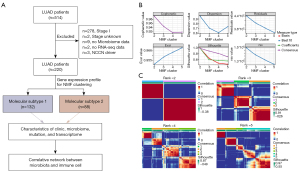
Table 1
Characteristic | Overall (N=220) | MS1 (N=132) | MS2 (N=88) | P value* |
---|---|---|---|---|
Age (years), n (%) | 0.40 | |||
<60 | 68 (31) | 38 (29) | 30 (34) | |
≥60 | 152 (69) | 94 (71) | 58 (66) | |
Sex, n (%) | <0.001 | |||
Female | 108 (49) | 79 (60) | 29 (33) | |
Male | 112 (51) | 53 (40) | 59 (67) | |
Race, n (%) | >0.9 | |||
American Indian or Alaska Native | 1 (0.5) | 1 (0.8) | 0 | |
Asian | 3 (1.4) | 2 (1.5) | 1 (1.1) | |
Black or African American | 25 (11) | 15 (11) | 10 (11) | |
Unknown | 26 (12) | 14 (11) | 12 (14) | |
White | 165 (75) | 100 (76) | 65 (74) | |
Stage, n (%) | 0.30 | |||
II | 114 (52) | 74 (56) | 40 (45) | |
III | 79 (36) | 42 (32) | 37 (42) | |
IV | 27 (12) | 16 (12) | 11 (13) | |
New tumor event, n (%) | 0.40 | |||
No | 101 (46) | 64 (48) | 37 (42) | |
Unknown | 34 (15) | 17 (13) | 17 (19) | |
Yes | 85 (39) | 51 (39) | 34 (39) | |
Radiation, n (%) | 0.053 | |||
No | 155 (70) | 101 (77) | 54 (61) | |
Unknown | 22 (10) | 10 (7.6) | 12 (14) | |
Yes | 43 (20) | 21 (16) | 22 (25) |
*, Pearson’s Chi-squared test. MS1, molecular subtype 1; MS2, molecular subtype 2.
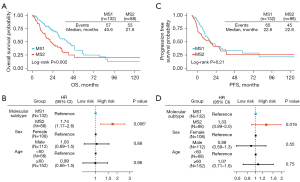
Immune infiltration atlas
We demonstrated that patients in the MS1 group had significantly higher stromal scores, immune scores, and estimate scores compared to those in the MS2 group (Figure 3A). Furthermore, analysis of immune cell composition revealed that the MS1 group exhibited a higher level of immune activity, which was significantly increased in CD4 T cells, CD8 T cells, NK cells, and macrophages (Figure 3B). Interestingly, we found a significant increase in neutrophils and γδT cells within the MS2 group (Figure 3B), indicating a heightened inflammatory response. The immune-cell association profiles are similar for both subtypes (Figure 3C,3D). Overall, our findings suggest that patients with MS2 exhibit a stronger inflammatory response.
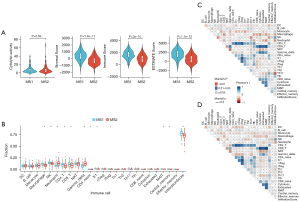
Gene mutational landscapes and transcriptomic profiles in molecular subtypes
To gain a more comprehensive understanding of the association between mutation repertoires and microbiota profiles, we examined the patterns of gene mutation and mutational signature. In the cohort of 220 subjects, we identified 77,797 single nucleotide variants (SNVs), 2,347 deletions, and 1,011 insertions. The top mutated genes were TP53 (49%), TTN (48%), MUC16 (38%), RYR2 (37%), and CSMD3 (36%) (Figure 4A). Furthermore, we investigated the transcriptomic gene sets between MS1 and MS2 groups comprehensively. Gene set enrichment analysis revealed that the top ten hallmark gene sets were significantly enriched in the MS1 and MS2 group (Figure 4B). Specifically related to immune response processes in oncology, these gene sets highlighted their significance in shaping molecular subtype patterns.
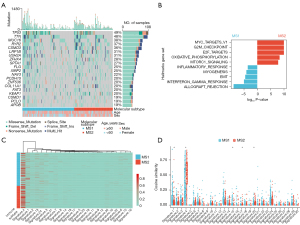
To explore potential carcinogenic factors associated with mutational spectrum involvement in molecular subtypes, we conducted an extensive analysis of mutational signatures. Signature 4 was found to be predominant in both MS1 and MS2 patients (Figure 4C,4D); however, it exhibited a significant increase specifically within the MS2 subgroup. This suggests that smoking-related mutagenesis signature 4 is a risk factor for MS2 subgroup with poor survival outcomes.
Microbiota biomarkers were associated with molecular subtype
We investigated the relative abundances in each group at various taxonomic levels (Figure S1). At the phylum level (Figure 5A), Proteobacteria (MS1: 47.59%, MS2: 45.64%), Actinobacteria (MS1: 26.59%, MS2: 27.22%), Bacteroidetes (MS1: 15.18%, MS2: 15.81%), Firmicutes (MS1: 7.60%, MS2: 8.04%), and Chlamydiae (MS1: 2.16%, MS2: 2.27%) were predominant. At the genus level (Figure 5B), Terrabacter (MS1: 22.74%, MS2: 23.32%), Neisseria (MS1: 16.17%, MS2: 12.07%), Bacteroides (MS1: 12.08%, MS2: 12.85%), Proteus (MS1: 5.61%, MS2: 6.25%), and Listeria (MS1: 4.58%, MS2: 4.99%) were predominant in our included samples.
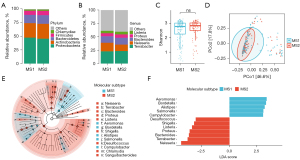
In order to investigate the impact of molecular subtype on microbiota diversity, we evaluated its effect on α-diversity using Shannon’s index (Figure 5C) as well as Fisher’s index, Simpson’s index, InvSimpson’s index (Figure S1). However, no statistically significant differences were observed between the MS1 and MS2 groups based on these indices (P>0.05). Similarly, β-diversity analysis based on Bray-Curtis distances also did not reveal any notable dissimilarities (P=0.58) (Figure 5D). The microbiota spectrum were similar between the MS1 and MS2 groups. To identify high-dimensional biomarkers, we utilized the LEfSe software to discover predominant bacterial taxa associated with different clinical characteristics. We observed significant differences in five genera, namely Aeromonas, Bordetella, Alistipes, Salmonella, and Campylobacter, which exhibited higher abundance in the MS1 group compared to the MS2 group (Figure 5E,5F; Figure S2). Conversely, Desulfococcus, Terrabacter, Bacteroides, Shigella, Listeria, Proteus, and Neisseria showed significantly higher abundance in the MS2 group than in the MS1 group (Figure 5E,5F).
The γδT cells were correlated with microbiota biomarkers for suppressing immunity
In order to better characterize host-microbe interactions within the immune system context, we assessed the correlative matrix between microbiota biomarkers and immune profiles as represented by a refined network for MS1 (Figure 6A) and a closely connected network for MS2 (Figure 6B). These variations in microbiome and immune repertoires provide valuable insights for studying correlations between bacterial profiles and immune profiles in LUAD. Within this analysis of networks, we showed eight microbiota biomarkers, including Aeromonas, Bordetella, Salmonella, Desulfococcus, Terrabacter, Bacteroides, Proteus, and Neisseria, that correlated with γδT cells specifically within the context of the MS2 network (Figure 6B). Notably, the refined network for MS1 (Figure 6A) demonstrated closer correlations among Treg cells, CD4 cells, and B cells when compared to those observed within the MS2 network.
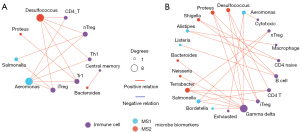
Diverse drug sensitivity may serve as novel approach for underpinning cancer immunotherapy
To gain a comprehensive understanding of potential disparities in treatment options, we conducted an investigation into the drug sensitivity across molecular subtypes (table available at https://cdn.amegroups.cn/static/public/tlcr-24-393-2.xlsx). Notably, there were no significant variations observed in the sensitivity to cisplatin, paclitaxel, docetaxel, gemcitabine, and common targeted drugs between the two molecular subtypes (Figure 7A-7L). However, it was found that MS2 exhibited heightened sensitivity towards savolitinib (Figure 7M), and venetoclax (Figure 7N)—a BCL-2 protein binder that displaces pro-apoptotic proteins—resulting in mitochondrial outer membrane permeability and caspase activation to restore apoptotic processes (37). Additionally, MS2 demonstrated increased susceptibility to MK-8766 (38), an agent targeting cell cycle regulation (Figure 7O). Conversely, MS1 displayed greater responsiveness to ERK-2440 (Figure 7P) which modulates the ERK/MAPK pathway. These findings on drug sensitivity suggest a close association between MS2 and cell cycle regulation while highlighting potential avenues for targeted drug development.
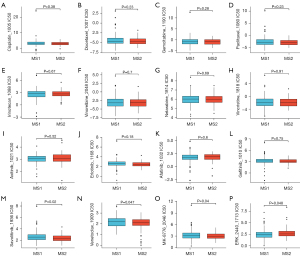
Discussion
There is abundant evidence that the microbiota affects the immune response to cancer (39). A deeper understanding of the intratumor microbiota’s impact on the tumor microenvironment (TME) holds immense potential for advancing tumor immunotherapy or microbiota-targeted therapies. Our findings uncover a previously unappreciated role of immune landscape in the interaction between immune cells and microbiota, promoting the prognosis of LUAD from an anti-tumor immunity state to progression. It is worth noting that microbiota biomarkers strongly correlate to γδT and neutrophil cells in the molecular subtype subject poor prognosis. Current pre-clinical studies and clinical trials with immunotherapy most are in the setting of gut microbiome, where the gut-lung axis plays a remote regulatory role, promoting antitumor immune responses (40,41), but also inducing pro-tumorigenic effects (42). However, the microbiota-immunity microenvironment in lung is very different from that of the intestinal system. More importantly, we demonstrated the molecular subtype with microbiota characteristics being different in the anti-tumor drugs sensitivity. Our observations warrant further investigation towards rationally harnessing the microbiota tumor heterogeneity profiles in the immunotherapy (43).
Recent studies have linked commensal microbiota to activation of oncogenic signaling pathways (44,45), or promotion on tumor progression (46). How the microbiota and TME act together to regulate the balance between tumor-promoting inflammation and anti-tumor immunity remains unclear. Our results displayed that tumor micro-environment landscapes were highly correlated with bacterial biomarker, indicating a relatively more significant contribution of the microbiota in lung cancer immune remodeling. We demonstrated that MS2 resulted in a worse survival prognosis. And there were significant differences in the composition of immune cells between the two molecular subtypes. The Neutrophils and γδT cells in MS2 were increased, and the macrophages, NK cells, CD4 T cells and CD8 T cells in MS1 were increased. This difference in immune cell composition may contribute to the varying survival outcomes observed between the two subtypes.
Evidence that commensal bacteria stimulated Myd88-dependent IL-1β and IL-23 production from myeloid cells, inducing proliferation and activation of Vγ6+Vδ1+ γδT cells that produced IL-17 and other effector molecules to promote inflammation and lung cancer cell proliferation has well been documented (10). This study also confirmed MS2 with a inflammatory profile and presented a strong association with microbiota biomarkers (Figure 6A,6B). For example, in the MS2 group, γδT cells were associated with eight biomarkers. Our results further reveal that intratumoral microbial markers can modulate gamma-delta T cell activity, leading to poorer tumor prognosis.
It has been documented that the abundance of Aeromonas is increased in tumor tissues such as thyroid cancer (47). These results strongly suggest the importance of lung microbiota in driving local inflammation and tumor promotion. In mouse studies, it has proved to be technically challenging to selectively manipulate the airway microbiota. Although the low biomass of microbial cells in the lung also makes the characterization of lung microbiota difficult (13,48), future studies will focus on defining the role of specific composition of the lung microbiota in tumor microenvironment.
Increasing evidence suggests that dysbiosis of intratumoral microbiome (49,50), serving as hallmarks of cancer, exert profound influences on tumor progression and responses to anticancer therapies (51-53). The Desulfococcus, Terrabacter, Bacteroides, Shigella, Listeria, Proteus, and Neisseria were significantly more abundant in MS2 than MS1 group (Figure 5A,5B). Specifically, the Desulfococcus, Terrabacter, Bacteroides, Proteus, and Neisseria were correlated with γδT cell, suggesting a strongly microbiota-immune cell interactive network.
Recent studies have report that diverse biomarkers, especially Bacteroides, Proteus, and Neisseria, may affect on tumor progression and prognosis, as well as the responses to ICI therapy. Recent studies have report that diverse biomarkers, including Bacteroides, Proteus, and Neisseria, may affect on tumor progression and prognosis (54-56), as well as the responses to ICI therapy (57).
It is probable that the microbiota effect is not solely reliant on a single biomarker, but rather a consequence of diverse microbiota repertories, contributing to the immunomodulatory reprogramme (39). Our findings further provide the evidence of the critical role of microbiota profiles in immunomodulatory. Furthermore, the sensitivity of tumor to chemotherapy is potentially regulated by the intratumoral flora.
In order to escape the apoptosis caused by chemotherapy, such as gemcitabine and cisplatin, the intratumoral bacteria can enhance tumor resistance by metabolizing drugs into compounds that have no therapeutic effect (12). A more thorough analysis of metabolites revealed that the drug-resistant group exhibited elevated levels of compounds like acetylcholine (58) and carnitine, which can promote tumor progression (58,59). However, there was no noteworthy variation in sensitivity towards commonly used chemotherapeutic agents between the two immunosubtypes (Figure 7). Intriguingly, MS2 exhibits greater sensitivity to venetoclax, a drug that binds to the BCL-2 protein, thereby suppressing pro-apoptotic proteins. This action leads to increased mitochondrial outer membrane permeability and the activation of caspases, ultimately restoring apoptosis.
Due to the limitations of the current experimental system for studying intratumoral flora, previous studies have not been able to accurately determine the influence of intratumoral microbiota on the efficacy of immune checkpoint inhibitors. The identification of the pivotal roles played by microbiota and immune cell composition holds immense significance for the development of novel clinical drugs specifically targeting the microbiota.
Limitations
It is an important issue of tumor microbiota immunity to analyze whether the disorder of tumor microbiota can trigger innate immunity and how to induce tumor-specific immune tolerance to drive the process of tumor metastasis. The interplay between smoking, lung microbiota, and immune infiltration necessitates further preclinical modeling for confirmation. However, unfortunately, we are yet to be able to elucidate the mechanisms by which the bacteria modulate the transcripts repertoire in different tissues. Ultimately, low levels of the bacterial contamination were not able to be excluded perfectly, further studies are needed to verify microbe-host interactions.
Conclusions
In conclusion, our results revealed distinct profiles of microbiome and transcriptome residing within LUAD. Furthermore, the microbiota biomarkers were correlated with cell composition with the increased of neutrophils and γδT cells, which in turn intensifies tumor immune infiltration and cell cycle, ultimately promoting tumor development. The findings of this study hold significant implications for the development of personalized immunotherapy strategies for LUAD.
Acknowledgments
We acknowledge TCGA and cBioportal databases for providing their platforms and contributors for uploading their meaningful datasets.
Funding: This study was supported by
Footnote
Reporting Checklist: The authors have completed the STROBE reporting checklist. Available at https://tlcr.amegroups.com/article/view/10.21037/tlcr-24-393/rc
Peer Review File: Available at https://tlcr.amegroups.com/article/view/10.21037/tlcr-24-393/prf
Conflicts of Interest: All authors have completed the ICMJE uniform disclosure form (available at https://tlcr.amegroups.com/article/view/10.21037/tlcr-24-393/coif). W.L. serves as an unpaid Associate Editor-in-Chief of Translational Lung Cancer Research from May 2024 to April 2025. The other authors have no conflicts of interest to declare.
Ethical Statement: The authors are accountable for all aspects of the work in ensuring that questions related to the accuracy or integrity of any part of the work are appropriately investigated and resolved. The study was conducted in accordance with the Declaration of Helsinki (as revised in 2013).
Open Access Statement: This is an Open Access article distributed in accordance with the Creative Commons Attribution-NonCommercial-NoDerivs 4.0 International License (CC BY-NC-ND 4.0), which permits the non-commercial replication and distribution of the article with the strict proviso that no changes or edits are made and the original work is properly cited (including links to both the formal publication through the relevant DOI and the license). See: https://creativecommons.org/licenses/by-nc-nd/4.0/.
References
- Sepich-Poore GD, Zitvogel L, Straussman R, et al. The microbiome and human cancer. Science 2021;371:eabc4552. [Crossref] [PubMed]
- Nejman D, Livyatan I, Fuks G, et al. The human tumor microbiome is composed of tumor type-specific intracellular bacteria. Science 2020;368:973-80. [Crossref] [PubMed]
- Riquelme E, Zhang Y, Zhang L, et al. Tumor Microbiome Diversity and Composition Influence Pancreatic Cancer Outcomes. Cell 2019;178:795-806.e12. [Crossref] [PubMed]
- Kalaora S, Nagler A, Nejman D, et al. Identification of bacteria-derived HLA-bound peptides in melanoma. Nature 2021;592:138-43. [Crossref] [PubMed]
- Bullman S, Pedamallu CS, Sicinska E, et al. Analysis of Fusobacterium persistence and antibiotic response in colorectal cancer. Science 2017;358:1443-8. [Crossref] [PubMed]
- Mouradov D, Greenfield P, Li S, et al. Oncomicrobial Community Profiling Identifies Clinicomolecular and Prognostic Subtypes of Colorectal Cancer. Gastroenterology 2023;165:104-20. [Crossref] [PubMed]
- Parhi L, Alon-Maimon T, Sol A, et al. Breast cancer colonization by Fusobacterium nucleatum accelerates tumor growth and metastatic progression. Nat Commun 2020;11:3259. [Crossref] [PubMed]
- Fu A, Yao B, Dong T, et al. Tumor-resident intracellular microbiota promotes metastatic colonization in breast cancer. Cell 2022;185:1356-1372.e26. [Crossref] [PubMed]
- Battaglia TW, Mimpen IL, Traets JJH, et al. A pan-cancer analysis of the microbiome in metastatic cancer. Cell 2024;187:2324-2335.e19. [Crossref] [PubMed]
- Jin C, Lagoudas GK, Zhao C, et al. Commensal Microbiota Promote Lung Cancer Development via γδ T Cells. Cell 2019;176:998-1013.e16. [Crossref] [PubMed]
- Yu T, Guo F, Yu Y, et al. Fusobacterium nucleatum Promotes Chemoresistance to Colorectal Cancer by Modulating Autophagy. Cell 2017;170:548-563.e16. [Crossref] [PubMed]
- Geller LT, Barzily-Rokni M, Danino T, et al. Potential role of intratumor bacteria in mediating tumor resistance to the chemotherapeutic drug gemcitabine. Science 2017;357:1156-60. [Crossref] [PubMed]
- Dickson RP, Erb-Downward JR, Martinez FJ, et al. The Microbiome and the Respiratory Tract. Annu Rev Physiol 2016;78:481-504. [Crossref] [PubMed]
- Kelly BJ, Imai I, Bittinger K, et al. Composition and dynamics of the respiratory tract microbiome in intubated patients. Microbiome 2016;4:7. [Crossref] [PubMed]
- Zakharkina T, Martin-Loeches I, Matamoros S, et al. The dynamics of the pulmonary microbiome during mechanical ventilation in the intensive care unit and the association with occurrence of pneumonia. Thorax 2017;72:803-10. [Crossref] [PubMed]
- Chen Z, Fillmore CM, Hammerman PS, et al. Non-small-cell lung cancers: a heterogeneous set of diseases. Nat Rev Cancer 2014;14:535-46. [Crossref] [PubMed]
- Sung H, Ferlay J, Siegel RL, et al. Global Cancer Statistics 2020: GLOBOCAN Estimates of Incidence and Mortality Worldwide for 36 Cancers in 185 Countries. CA Cancer J Clin 2021;71:209-49. [Crossref] [PubMed]
- Liu NN, Yi CX, Wei LQ, et al. The intratumor mycobiome promotes lung cancer progression via myeloid-derived suppressor cells. Cancer Cell 2023;41:1927-1944.e9. [Crossref] [PubMed]
- Helmink BA, Khan MAW, Hermann A, et al. The microbiome, cancer, and cancer therapy. Nat Med 2019;25:377-88. [Crossref] [PubMed]
- Cerami E, Gao J, Dogrusoz U, et al. The cBio cancer genomics portal: an open platform for exploring multidimensional cancer genomics data. Cancer Discov 2012;2:401-4. [Crossref] [PubMed]
- Wakelee H, Liberman M, Kato T, et al. Perioperative Pembrolizumab for Early-Stage Non-Small-Cell Lung Cancer. N Engl J Med 2023;389:491-503. [Crossref] [PubMed]
- Poore GD, Kopylova E, Zhu Q, et al. Microbiome analyses of blood and tissues suggest cancer diagnostic approach. Nature 2020;579:567-74. [Crossref] [PubMed]
- Gaujoux R, Seoighe C. A flexible R package for nonnegative matrix factorization. BMC Bioinformatics 2010;11:367. [Crossref] [PubMed]
- Brunet JP, Tamayo P, Golub TR, et al. Metagenes and molecular pattern discovery using matrix factorization. Proc Natl Acad Sci U S A 2004;101:4164-9. [Crossref] [PubMed]
- Mayakonda A, Lin DC, Assenov Y, et al. Maftools: efficient and comprehensive analysis of somatic variants in cancer. Genome Res 2018;28:1747-56. [Crossref] [PubMed]
- Rosenthal R, McGranahan N, Herrero J, et al. DeconstructSigs: delineating mutational processes in single tumors distinguishes DNA repair deficiencies and patterns of carcinoma evolution. Genome Biol 2016;17:31. [Crossref] [PubMed]
- Alexandrov LB, Nik-Zainal S, Wedge DC, et al. Signatures of mutational processes in human cancer. Nature 2013;500:415-21. [Crossref] [PubMed]
- Subramanian A, Tamayo P, Mootha VK, et al. Gene set enrichment analysis: a knowledge-based approach for interpreting genome-wide expression profiles. Proc Natl Acad Sci U S A 2005;102:15545-50. [Crossref] [PubMed]
- Yu G, Wang LG, Han Y, et al. clusterProfiler: an R package for comparing biological themes among gene clusters. OMICS 2012;16:284-7. [Crossref] [PubMed]
- Liberzon A, Birger C, Thorvaldsdóttir H, et al. The Molecular Signatures Database (MSigDB) hallmark gene set collection. Cell Syst 2015;1:417-25. [Crossref] [PubMed]
- Miao YR, Zhang Q, Lei Q, et al. ImmuCellAI: A Unique Method for Comprehensive T-Cell Subsets Abundance Prediction and its Application in Cancer Immunotherapy. Adv Sci (Weinh) 2020;7:1902880. [Crossref] [PubMed]
- Liu C, Cui Y, Li X, et al. microeco: an R package for data mining in microbial community ecology. FEMS Microbiol Ecol 2021;97:fiaa255. [Crossref] [PubMed]
- Segata N, Izard J, Waldron L, et al. Metagenomic biomarker discovery and explanation. Genome Biol 2011;12:R60. [Crossref] [PubMed]
- Shannon P, Markiel A, Ozier O, et al. Cytoscape: a software environment for integrated models of biomolecular interaction networks. Genome Res 2003;13:2498-504. [Crossref] [PubMed]
- Maeser D, Gruener RF, Huang RS. oncoPredict: an R package for predicting in vivo or cancer patient drug response and biomarkers from cell line screening data. Brief Bioinform 2021;22:bbab260. [Crossref] [PubMed]
- Iorio F, Knijnenburg TA, Vis DJ, et al. A Landscape of Pharmacogenomic Interactions in Cancer. Cell 2016;166:740-54. [Crossref] [PubMed]
- Pan G, Zhong M, Yao J, et al. Orelabrutinib and venetoclax synergistically induce cell death in double-hit lymphoma by interfering with the crosstalk between the PI3K/AKT and p38/MAPK signaling. J Cancer Res Clin Oncol 2023;149:5513-29. [Crossref] [PubMed]
- Rudd MT, Manley PJ, Hanney B, et al. Discovery of MK-8768, a Potent and Selective mGluR2 Negative Allosteric Modulator. ACS Med Chem Lett 2023;14:1088-94. [Crossref] [PubMed]
- Lam KC, Araya RE, Huang A, et al. Microbiota triggers STING-type I IFN-dependent monocyte reprogramming of the tumor microenvironment. Cell 2021;184:5338-5356.e21. [Crossref] [PubMed]
- Li Z, Zhang Y, Hong W, et al. Gut microbiota modulate radiotherapy-associated antitumor immune responses against hepatocellular carcinoma Via STING signaling. Gut Microbes 2022;14:2119055. [Crossref] [PubMed]
- Routy B, Le Chatelier E, Derosa L, et al. Gut microbiome influences efficacy of PD-1-based immunotherapy against epithelial tumors. Science 2018;359:91-7. [Crossref] [PubMed]
- Cui W, Guo M, Liu D, et al. Gut microbial metabolite facilitates colorectal cancer development via ferroptosis inhibition. Nat Cell Biol 2024;26:124-37. [Crossref] [PubMed]
- Galeano Niño JL, Wu H, LaCourse KD, et al. Effect of the intratumoral microbiota on spatial and cellular heterogeneity in cancer. Nature 2022;611:810-7. [Crossref] [PubMed]
- Ramírez-Labrada AG, Isla D, Artal A, et al. The Influence of Lung Microbiota on Lung Carcinogenesis, Immunity, and Immunotherapy. Trends Cancer 2020;6:86-97. [Crossref] [PubMed]
- Garrett WS. Cancer and the microbiota. Science 2015;348:80-6. [Crossref] [PubMed]
- Belkaid Y, Hand TW. Role of the microbiota in immunity and inflammation. Cell 2014;157:121-41. [Crossref] [PubMed]
- Dai D, Yang Y, Yang Y, et al. Alterations of thyroid microbiota across different thyroid microhabitats in patients with thyroid carcinoma. J Transl Med 2021;19:488. [Crossref] [PubMed]
- Lloyd CM, Marsland BJ. Lung Homeostasis: Influence of Age, Microbes, and the Immune System. Immunity 2017;46:549-61. [Crossref] [PubMed]
- Dohlman AB, Klug J, Mesko M, et al. A pan-cancer mycobiome analysis reveals fungal involvement in gastrointestinal and lung tumors. Cell 2022;185:3807-3822.e12. [Crossref] [PubMed]
- Narunsky-Haziza L, Sepich-Poore GD, Livyatan I, et al. Pan-cancer analyses reveal cancer-type-specific fungal ecologies and bacteriome interactions. Cell 2022;185:3789-3806.e17. [Crossref] [PubMed]
- Zitvogel L, Ma Y, Raoult D, et al. The microbiome in cancer immunotherapy: Diagnostic tools and therapeutic strategies. Science 2018;359:1366-70. [Crossref] [PubMed]
- Tsay JJ, Wu BG, Badri MH, et al. Airway Microbiota Is Associated with Upregulation of the PI3K Pathway in Lung Cancer. Am J Respir Crit Care Med 2018;198:1188-98. [Crossref] [PubMed]
- Patnaik SK, Cortes EG, Kannisto ED, et al. Lower airway bacterial microbiome may influence recurrence after resection of early-stage non-small cell lung cancer. J Thorac Cardiovasc Surg 2021;161:419-429.e16. [Crossref] [PubMed]
- Abate M, Vos E, Gonen M, et al. A Novel Microbiome Signature in Gastric Cancer: A Two Independent Cohort Retrospective Analysis. Ann Surg 2022;276:605-15. [Crossref] [PubMed]
- Liu W, Zhang X, Xu H, et al. Microbial Community Heterogeneity Within Colorectal Neoplasia and its Correlation With Colorectal Carcinogenesis. Gastroenterology 2021;160:2395-408. [Crossref] [PubMed]
- Huang Y, Zhu N, Zheng X, et al. Intratumor Microbiome Analysis Identifies Positive Association Between Megasphaera and Survival of Chinese Patients With Pancreatic Ductal Adenocarcinomas. Front Immunol 2022;13:785422. [Crossref] [PubMed]
- Vétizou M, Pitt JM, Daillère R, et al. Anticancer immunotherapy by CTLA-4 blockade relies on the gut microbiota. Science 2015;350:1079-84. [Crossref] [PubMed]
- Nguyen PH, Touchefeu Y, Durand T, et al. Acetylcholine induces stem cell properties of gastric cancer cells of diffuse type. Tumour Biol 2018;40:1010428318799028. [Crossref] [PubMed]
- Kawai A, Matsumoto H, Endou Y, et al. Repeated Combined Chemotherapy with Cisplatin Lowers Carnitine Levels in Gastric Cancer Patients. Ann Nutr Metab 2017;71:261-5. [Crossref] [PubMed]