A nomogram for predicting invasiveness of lung adenocarcinoma manifesting as ground-glass nodules based on follow-up CT imaging
Highlight box
Key findings
• The nomogram combining morphological characteristics and quantitative parameters derived from follow-up computed tomography (CT) imaging achieved good performance in predicting the invasiveness of lung adenocarcinoma manifesting as ground-glass nodules (GGNs).
What is known and what is new?
• Age, diameter, density, pleural indentation, vessel changes, mass, mean density, mass growth rate, relative mass growth rate, and volume growth rate were significantly correlated with the invasiveness of GGNs.
• Quantitative parameters derived from follow-up CT imaging can enhance model performance.
What is the implication, and what should change now?
• We developed and validated a novel nomogram combined morphological features and quantitative parameters from follow-up CT imaging for predicting the invasiveness of lung adenocarcinoma manifesting as GGNs. This nomogram might help clinicians to make individualized predictions of each patient and to guide GGNs management.
Introduction
According to data published by the National Cancer Center in 2020 (1), the incidence and mortality rates of lung cancer still rank first among all cancers in China, with lung adenocarcinoma being the most prevalent subtype. Ground-glass nodules (GGNs) have a higher risk of malignancy than solid nodules, while in lung adenocarcinoma, GGNs have a better prognosis than solid nodules (2,3). Malignant GGNs generally display a slower growth rate and lower metastatic potential (3-5), making it challenging to accurately assess their invasiveness. The current surgery strategy for different pathologic stages of lung adenocarcinoma tends to differ (6). Patients with adenocarcinoma in situ (AIS) or minimally invasive adenocarcinoma (MIA) are typically considered for sublobar resection, while invasive adenocarcinoma (IA) may be preferred for lobectomy (6,7). Until now, there has been no effective model to guide surgical management. In lung cancer screening, overdiagnosis and/or overtreatment may occur in patients with GGNs (2).
Various studies have developed radiomics or deep learning models to predict the invasiveness of lung adenocarcinoma (8,9). However, these models often lack interpretability and reproducibility due to their black-box nature. Numerous studies have explored qualitative or quantitative computed tomography (CT) features for predicting the invasiveness of lung adenocarcinoma (10-13). Some research has focused solely on features extracted from pre-surgical CT images to construct predictive models (13-15). Unlike previous studies, we incorporate the morphological characteristics obtained from pre-surgical CT scans and quantitative parameters derived from follow-up CT imaging of patients to investigate whether this approach contributes to the development of more efficient models.
The nomogram is a reliable tool to create a simple intuitive graph of a statistical predictive model that quantifies the risk of a clinical event. In this study, we aimed to establish a nomogram for predicting the invasiveness of lung adenocarcinoma manifesting as GGNs based on the morphological characteristics obtained from pre-surgical CT scans and quantitative parameters derived from follow-up CT imaging, in order to provide personalized medical care for patients. We present this article in accordance with the TRIPOD reporting checklist (available at https://tlcr.amegroups.com/article/view/10.21037/tlcr-24-492/rc).
Methods
The study was conducted in accordance with the Declaration of Helsinki (as revised in 2013). The study was approved by the Ethics Committee of Wuhan Union Hospital (Approval No. 0516). Informed consent was obtained from prospective patients, while the requirement for informed consent was waived for retrospective patients due to the retrospective nature of the analysis.
Patients
From June 14, 2017 to August 17, 2023, data of a total of 8,458 GGNs that underwent surgical resection at Wuhan Union Hospital were retrospectively reviewed. The inclusion criteria were as follows: (I) lesion presenting as GGN at CT scans; (II) pathologic diagnoses of GGNs determined to be atypical adenomatous hyperplasia (AAH), AIS, MIA, or IA; and (III) an interval between pre-surgical CT and surgery of less than one month. A total of 7,835 GGNs were excluded. Exclusion criteria were as follows: (I) availability of only one chest CT image in the picture archiving and communication system (PACS) (n=7,465); (II) prior administration of radiotherapy, chemotherapy, or neoadjuvant immunotherapy for GGNs, or for other tumors, these treatments administered within the past two years (n=331); (III) presence of motion artifacts on CT images that compromised accurate evaluation (n=36); and (IV) GGNs with a diameter larger than 30 mm (n=3). A total of 623 GGNs (601 patients), including 7 AAHs, 191 AISs, 212 MIAs, and 213 IAs, were ultimately included. The cohort was randomly divided into a training (n=436) and a testing set (n=187) at 7:3 ratio.
Additionally, between September 3, 2023, and April 20, 2024, a total of 1,783 GGNs underwent surgical resection. Of these, 1,696 were excluded due to the following reasons: (I) availability of only one chest CT image in the PACS (n=1,497); (II) prior administration of radiotherapy, chemotherapy, or neoadjuvant immunotherapy for GGNs, or for other tumors, these treatments administered within the past two years (n=188); (III) presence of motion artifacts on CT images compromising accurate evaluation (n=4); and (IV) GGNs with a diameter larger than 30 mm (n=7). Accordingly, a total of 87 GGNs (86 patients), including 16 AISs, 43 MIAs, and 28 IAs, formed the validation cohort.
The patient selection procedure is shown in Figure 1. For patients with multiple GGNs, we included them in the study and treated each GGN as a separate entity with similar clinical characteristics. We did not observe any newly appeared GGNs on the second CT scan that were confirmed pathologically as lung adenocarcinoma.
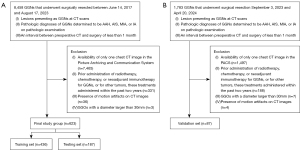
Histologic evaluation
The pathological findings for pulmonary adenocarcinoma were assessed according to the International Association for the Study of Lung Cancer/American Thoracic Society/European Respiratory classification. This classification system includes various subtypes such as AAH, AIS, MIA, lepidic-predominant adenocarcinoma (LPA), acinar-predominant adenocarcinoma (APA), papillary-predominant adenocarcinoma (PPA), micropapillary-predominant adenocarcinoma (MPA), solid-predominant adenocarcinoma (SPA) and others. In this study, GGNs classified as AAH, AIS, and MIA were considered Group A, while the remaining subtypes were categorized as Group B. The classification was based on the subtype with the highest proportion among the observed subtypes. Tumor staging was conducted following the tumor-node-metastasis classification, 8th edition (16). The presence of spread through air space, perineural invasion, pleural invasion, vascular invasion, lymphatic invasion, and surgical margin status was routinely evaluated for each tumor. Anaplastic lymphoma kinase (ALK) rearrangement and epidermal growth factor receptor (EGFR) status were evaluated based on clinical requirements.
CT image acquisition
All patients underwent unenhanced CT scans within one month prior to surgery. CT examinations were performed using SOMATOM Force scanner, SOMATOM Perspective scanner, SOMATOM Definition AS + scanner (Siemens Healthineers, Forchheim, Germany), Aquilion ONE Toshiba CT scanner (Toshiba Medical Systems Corp., Tochigi, Japan), or Discovery CT750 HD scanner (General Electric Inc., Milwaukee, USA). CT examinations were conducted from the chest inlet to the inferior level of the costophrenic angle. The scan parameters included detector collimation width of 64×0.6 mm or 128×0.6 mm, and tube voltage of 100, 110 or 120 kV. The tube current was regulated by an automatic exposure control system (CARE Dose 4D). CT images were acquired with slice thickness of either 1.5 or 2.0 mm, and corresponding reconstruction interval of 1.5 or 2.0 mm. Then, the reconstructed image was transmitted to the workstation and PACS for multiplanar reconstruction (MPR) postprocessing. The lung window was set at a level of −600 Hounsfield units (HU) with a width of 1,200 HU, while the mediastinal window was set at a level of 50 HU with a width was 1,200 HU.
CT image interpretation
Two radiologists (H.L., a thoracic radiologist with 2 years of experience, and H.S., a radiology fellow with 35 years of experience in interpreting CT images) evaluated all imaging data independently. Both radiologists reviewed the CT images without clinical and histologic information but knew the presence and locations of the tumors. When the interpretations of the two radiologists differed, the discrepancies were resolved by consensus.
The following morphologic features were evaluated on the pre-surgical CT images: nodule location, diameter (the longest diameter on axial CT images), density [pure ground-glass nodule (pGGN) or mixed ground-glass nodule (mGGN)], uniformity (homogeneous or heterogeneous), shape (round, oval, or irregular), margin (smooth or coarse), nodule-lung interface (well defined or ill defined), lobulation, spiculation, pleural indentation, air bronchogram, vascular convergence sign, vacuole sign, cavity, vessel changes, halo sign, pleural effusion, pleural thickening, calcification, and lymphadenopathy. The relationship between GGNs and vessels was categorized as type I (vessels passing by or through nodules) or type II (vessels distorted or dilated within nodules). mGGNs were divided into two subtypes, ground-glass opacities (GGOs)-dominant and solid-dominant mGGNs using a consolidation-to-tumor ratio (CTR) cutoff value of 0.5. CTR was defined as the ratio of the maximum size of consolidation to the maximum tumor size.
Quantitative parameters from follow-up CT imaging
The InferRead CT Lung (version R13.3; Infervision Medical Technology Co., Ltd., Beijing, China), an AI-based pulmonary nodule auxiliary diagnosis system, was used to measure the volume, mean density, solid component volume (SV), percentage of solid component (PSC), and mass of GGNs in each patient’s two CT images. The CT value threshold of −300 HU was used to distinguish the solid component from the ground glass component. Volume growth rate was calculated as the increase in volume from the baseline level to the pre-surgical level divided by time (Figures 2,3). Relative volume growth rate was defined as the relative net change of volume divided by time. The equation is described as:
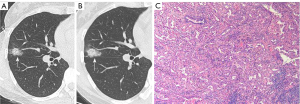
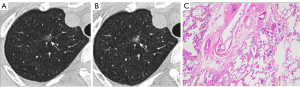
where Vpre is the volume on the pre-surgical CT, and Vbaselineis volume on the baseline CT. Similarly, other parameters were evaluated. The growth rates of GGNs from two follow-up CT scans mainly included parameters growth rate and relative parameter growth rate in this study.
Construction of the prediction model
Clinical characteristics, morphological features and quantitative parameters were analyzed using least absolute shrinkage and selection operator (LASSO) regression analysis in the training set. The selected variables were used to establish three multivariable logistic predictive models: model 1 utilized clinical characteristics and morphological features on pre-surgical CT, model 2 incorporated clinical characteristics, morphological features and quantitative parameters on pre-surgical CT, and model 3 incorporated clinical characteristics, morphological features, quantitative parameters from pre-surgical CT, and growth rates of GGNs.
The performance of the models included its discrimination and calibration was tested using the testing and validation data sets. Receiver operating characteristic (ROC) curves and the area under the ROC curve (AUC) were used to determine the discrimination of the models. The Hosmer-Lemeshow test and calibration curves were used to evaluate the calibration performance of the radiomic models. By quantifying the net benefits at different threshold probabilities, we conducted a decision curve analysis (DCA) to evaluate the clinical value of the nomogram.
Statistical analysis
Statistical analysis was conducted with R version 4.3.2 (R Foundation for Statistical Computing, Vienna, Austria) and the IBM SPSS software (version 26.0). P<0.05 was considered statistically significant.
SPSS was used for the following purposes: Distribution normality was evaluated with the Kolmogorov-Smirnov test. Data with a normally distributed were presented as the mean [standard deviation (SD)], while non-normally distributed data were presented as the median [interquartile range (IQR)]. Categorical variables were presented as the frequency (%). Unordered categorical variables were compared using the Pearson Chi-squared test or Fisher’s exact test. Non-normally distributed continuous variables and ordered categorical variables were compared between the groups using the Wilcoxon rank-sum test.
R language was utilized for the following purposes: For CT signs, interobserver agreement was assessed using the k coefficient. The DeLong test was used to compare AUCs between models. LASSO regression analysis was performed using “glmnet” package. The “rms” package was applied to analyze multivariate logistic regression. ROC analysis was carried out with the “pROC” package. The “rmda” package was used to perform DCA.
Results
Clinicopathologic characteristics
The clinicopathological characteristics of all patients on admission were summarized in Table 1. A total of 710 patients were included in this study, including 218 men and 492 women, with a median age of 55 years (range, 23 to 77 years). Of these, 92 patients (12.96%) had a smoking history, and 59 (8.31%) had a history of another malignant tumor, with thyroid cancer being the most common (36 patients, 61.02%).
Table 1
Factor | Total patients (N=710) | Group A (N=469) | Group B (N=241) | P value |
---|---|---|---|---|
Sex | 0.03* | |||
Female | 492 (69.30) | 338 (72.07) | 154 (63.90) | |
Male | 218 (30.70) | 131 (27.93) | 87 (36.10) | |
Age, years | 55.00 (46.00, 62.00) | 53.00 (43.00, 60.00) | 59.00 (53.00, 66.00) | <0.001* |
Smoking history | 92 (12.96) | 43 (9.17) | 49 (20.33) | <0.001* |
History of another malignant tumor | 59 (8.31) | 41 (8.74) | 18 (7.47) | 0.56 |
Pathological T staging† | <0.001* | |||
Tis | 207 (29.15) | 207 (44.14) | 0 (0.00) | |
T1a(mi) | 255 (35.92) | 255 (54.37) | 0 (0.00) | |
T1a | 78 (10.99) | 0 (0.00) | 78 (32.37) | |
T1b | 131 (18.45) | 0 (0.00) | 131 (54.36) | |
T1c | 26 (3.66) | 0 (0.00) | 26 (10.79) | |
T2a | 6 (0.85) | 0 (0.00) | 6 (2.49) | |
Histologic subtypes | <0.001* | |||
AHH | 7 (0.99) | 7 (1.49) | 0 (0.00) | |
AIS | 207 (29.15) | 207 (44.14) | 0 (0.00) | |
MIA | 255 (35.92) | 255 (54.37) | 0 (0.00) | |
LPA | 48 (6.76) | 0 (0.00) | 48 (19.92) | |
APA | 125 (17.61) | 0 (0.00) | 125 (51.87) | |
MPA | 2 (0.28) | 0 (0.00) | 2 (0.83) | |
PPA | 58 (8.17) | 0 (0.00) | 58 (24.07) | |
SPA | 4 (0.56) | 0 (0.00) | 4 (1.66) | |
MA | 4 (0.56) | 0 (0.00) | 4 (1.66) | |
Lymphatic invasion | 0 (0.00) | 0 (0.00) | 0 (0.00) | – |
Perineural invasion | 1 (0.14) | 0 (0.00) | 1 (0.41) | 0.34 |
Pleural invasion | 6 (0.85) | 0 (0.00) | 6 (2.49) | 0.001* |
Surgical margin | 1 (0.14) | 0 (0.00) | 1 (0.41) | 0.34 |
Spread through air space | 18 (2.54) | 0 (0.00) | 18 (7.47) | <0.001* |
Vascular invasion | 0 (0.00) | 0 (0.00) | 0 (0.00) | – |
ALK rearrangement | 9 (1.27) | 1 (0.21) | 8 (3.32) | 0.001* |
EGFR | 56 (7.89) | 12 (2.56) | 44 (18.26) | <0.001* |
Follow-up, months | 5.00 (3.00, 8.00) | 5.00 (3.00, 8.00) | 4.00 (3.00, 8.00) | 0.20 |
Data are presented as median (IQR) or n (%). Group A: atypical adenomatous hyperplasia, adenocarcinoma in situ, and minimally invasive adenocarcinoma; Group B: invasive adenocarcinoma. *, P values <0.05. †, pathological T staging was based on the IASLC 8th TNM Lung Cancer Staging System. AHH, atypical adenomatous hyperplasia; AIS, adenocarcinoma in situ; MIA, minimally invasive adenocarcinoma; LPA, lepidic-predominant adenocarcinoma; APA, acinar-predominant adenocarcinoma; MPA, micropapillary-predominant adenocarcinoma; PPA, papillary-predominant adenocarcinoma; SPA, solid-predominant adenocarcinoma; MA, mucinous adenocarcinoma; ALK, anaplastic lymphoma kinase; EGFR, epidermal growth factor receptor; IQR, interquartile range.
Compared to the Group A, Group B had a higher proportion of males (36.10% vs. 27.93%, P=0.03), older patients [59.00 (53.00, 66.00) vs. 53.00 (43.00, 60.00), P<0.001], and more patients with a history of smoking (20.33% vs. 9.17%, P<0.001). In our study, preoperative lymphatic and vascular invasion were not observed in any of the patients. Perineural invasion, pleural invasion, surgical margin, and spread through air space were exclusively identified in Group B, with frequencies of 0.41% (1/241, P=0.34), 2.49% (6/241, P=0.001), 0.41% (1/241, P=0.34), and 7.47% (18/241, P<0.001) respectively. ALK rearrangement was present in nine patients (1.27%) and EGFR mutation in 56 patients (7.89%). ALK rearrangement and EGFR mutation were more prevalent in Group B compared to Group A (P=0.001, P<0.001, respectively).
Interobserver agreement in CT interpretation
The intraclass correlation coefficient for tumor maximum diameter was 0.892 [95% confidence interval (CI): 0.844–0.926]. Regarding other CT features, the concordance between the two observers was good, with the k coefficients ranging between 0.713 and 1.000 (Table 2).
Table 2
CT features | N (% of concordance) | Kappa (95% CI) | Kappa interpretation |
---|---|---|---|
Density | 93/100 | 0.845 (0.735–0.956) | Almost perfect |
Shape | 91/100 | 0.796 (0.676–0.915) | Substantial |
Uniformity | 90/100 | 0.796 (0.676–0.915) | Substantial |
Margin | 94/100 | 0.840 (0.716–0.964) | Almost perfect |
Nodule lung interface | 89/100 | 0.753 (0.616–0.890) | Substantial |
Pleural indentation | 95/100 | 0.868 (0.756–0.981) | Almost perfect |
Lobulation | 88/100 | 0.754 (0.623–0.884) | Substantial |
Spiculation | 91/100 | 0.713 (0.538–0.889) | Substantial |
Air bronchogram | 96/100 | 0.757 (0.531–0.983) | Substantial |
Vascular convergence sign | 100/100 | 1.000 | Almost perfect |
Vacuole sign | 92/100 | 0.836 (0.727–0.945) | Almost perfect |
Cavity | 100/100 | 1.000 | Almost perfect |
Vessel changes | 94/100 | 0.735 (0.533–0.936) | Substantial |
Halo sign | 100/100 | 1.000 | Almost perfect |
Pleural effusion | 100/100 | 1.000 | Almost perfect |
Pleural thickening | 93/100 | 0.718 (0.522–0.914) | Substantial |
Calcification | 100/100 | 1.000 | Almost perfect |
Lymphadenopathy | 100/100 | 1.000 | Almost perfect |
CT, computed tomography; CI, confidence interval.
Morphological features and quantitative parameters from follow-up CT imaging
Table 3 show the comparison of morphological features and quantitative parameters between Group A and Group B. The analysis conducted in this study indicated that various morphological characteristics, including diameter (P<0.001), density (P<0.001), shape (P<0.001), uniformity (P<0.001), margin (P<0.001), pleural indentation (P<0.001), lobulation (P=0.002), spiculation (P<0.001), air bronchograms (P<0.001), vascular convergence sign (P=0.01), cavity (P<0.001), vessel changes (P<0.001), and pleural thickening (P=0.001) were significantly correlated with the invasiveness of GGNs. Clinicopathological and radiological characteristics of 710 patients in pGGN, GGO-dominant mixed GGO (mGGO), and solid-dominant mGGO groups were summarized in Table S1.
Table 3
Factor | Total patients (N=710) | Group A (N=469) | Group B (N=241) | P value |
---|---|---|---|---|
Location | 0.15 | |||
LUL | 199 (28.03) | 137 (29.21) | 62 (25.73) | |
LLL | 85 (11.97) | 56 (11.94) | 29 (12.03) | |
RUL | 259 (36.48) | 168 (35.82) | 91 (37.76) | |
RML | 47 (6.62) | 37 (7.89) | 10 (4.15) | |
RLL | 120 (16.90) | 71 (15.14) | 49 (20.33) | |
Density | <0.001* | |||
pGGN | 297 (41.83) | 260 (55.44) | 37 (15.35) | |
mGGN | 413 (58.17) | 209 (44.56) | 204 (84.65) | <0.001* |
GGO-dominant | 350 (84.75) | 200 (95.69) | 150 (73.53) | |
Solid-dominant | 63 (15.25) | 9 (4.31) | 54 (26.47) | |
Shape | <0.001* | |||
Round or oval | 440 (61.97) | 334 (71.22) | 106 (43.98) | |
Irregular | 270 (38.03) | 135 (28.78) | 135 (56.02) | |
Uniformity | <0.001* | |||
Homogeneous | 176 (24.79) | 158 (33.69) | 18 (7.47) | |
Heterogeneous | 534 (75.21) | 311 (66.31) | 223 (92.53) | |
Margin | <0.001* | |||
Smooth | 479 (67.46) | 367 (78.25) | 112 (46.47) | |
Coarse | 231 (32.54) | 102 (21.75) | 129 (53.53) | |
Nodule lung interface | 0.91 | |||
Well-defined | 510 (71.83) | 338 (72.07) | 172 (71.37) | |
Ill-defined | 200 (28.17) | 131 (27.93) | 69 (28.63) | |
Pleural indentation | 243 (34.23) | 115 (24.52) | 128 (53.11) | <0.001* |
Lobulation | 494 (69.58) | 304 (64.82) | 190 (78.84) | <0.001* |
Spiculation | 176 (24.79) | 66 (14.07) | 110 (45.64) | <0.001* |
Air bronchogram | 164 (23.10) | 82 (17.48) | 82 (34.02) | <0.001* |
Vascular convergence sign | 4 (0.56) | 0 (0.00) | 4 (1.66) | 0.01* |
Vacuole sign | 368 (51.83) | 236 (50.32) | 132 (54.77) | 0.30 |
Cavity | 21 (2.96) | 3 (0.64) | 18 (7.47) | <0.001* |
Vessel changes | <0.001* | |||
Type I | 584 (82.25) | 412 (87.85) | 172 (71.37) | |
Type II | 126 (17.75) | 57 (12.15) | 69 (28.63) | |
Halo sign | 2 (0.28) | 0 (0.00) | 2 (0.83) | 0.11 |
Pleural effusion | 2 (0.28) | 2 (0.43) | 0 (0.00) | 0.55 |
Pleural thickening | 96 (13.52) | 49 (10.45) | 47 (19.50) | 0.001* |
Calcification | 1 (0.14) | 0 (0.00) | 1 (0.41) | 0.34 |
Lymphadenopathy | 7 (0.99) | 3 (0.64) | 4 (1.66) | 0.24 |
Diameter, mm | 10.00 (8.00, 14.00) | 9.00 (8.00, 12.00) | 13.00 (11.00, 17.00) | <0.001* |
Diameter growth rate, mm/month | 0.00 (0.00, 0.25) | 0.00 (0.00, 0.20) | 0.17 (0.00, 0.40) | <0.001* |
Relative diameter growth rate, %/month | 0.00 (0.00, 1.92) | 0.00 (0.00, 1.52) | 0.87 (0.00, 2.38) | 0.001* |
Mass, mg | 171.65 (95.20, 463.73) | 118.74 (76.48, 217.95) | 541.42 (252.74, 1,163.56) | <0.001* |
Mass growth rate, mg/month | 2.09 (−0.61, 9.98) | 0.83 (−0.85, 4.40) | 11.49 (1.33, 35.07) | <0.001* |
Relative mass growth rate, %/month | 1.43 (−0.50, 4.42) | 0.89 (−0.95, 3.27) | 2.92 (0.41, 6.35) | <0.001* |
Mean density, HU | −550.50 (−632.75, −442.25) | −584.00 (−652.00, −508.00) | −457.00 (−560.00, −321.00) | <0.001* |
Mean density growth rate, HU/month | −1.15 (−7.66, 4.53) | −1.30 (−7.00, 3.91) | −0.83 (−9.00, 6.33) | 0.64 |
Relative mean density growth rate, %/month | 0.20 (−0.85, 1.49) | 0.24 (−0.68, 1.19) | 0.13 (−1.43, 2.29) | 0.73 |
PSC, % | 7.67 (1.87, 20.96) | 4.70 (1.10, 11.81) | 19.58 (9.14, 43.32) | <0.001* |
PSC growth rate, %/month | −0.04 (−0.61, 0.36) | −0.04 (−0.50, 0.19) | −0.03 (−0.97, 0.76) | 0.41 |
Relative PSC growth rate, %/month | −0.59 (−6.86, 4.83) | −0.87 (−7.69, 4.27) | −0.01 (−5.01, 5.44) | 0.054 |
SV, mm3 | 26.50 (6.00, 129.50) | 12.00 (2.00, 38.00) | 191.00 (62.00, 482.00) | <0.001* |
SV growth rate, mm3/month | 0.00 (−1.00, 3.30) | 0.00 (−1.00, 0.90) | 3.50 (−1.20, 19.33) | <0.001* |
Relative SV growth rate, %/month | 0.00 (−5.51, 8.33) | 0.00 (−7.41, 7.14) | 2.69 (−1.11, 9.70) | <0.001* |
Volume, mm3 | 322.00 (176.50, 1,110.25) | 232.00 (137.00, 424.00) | 1,220.00 (381.00, 2,076.00) | <0.001* |
Volume growth rate, mm3/month | 5.00 (−1.31, 25.58) | 2.50 (−1.50, 11.50) | 21.33 (1.50, 75.40) | <0.001* |
Relative volume growth rate, %/month | 1.82 (−0.57, 5.65) | 1.24 (−0.94, 4.50) | 3.18 (0.39, 7.83) | <0.001* |
Data are presented as median (IQR) or n (%). Group A: atypical adenomatous hyperplasia, adenocarcinoma in situ, and minimally invasive adenocarcinoma; Group B: invasive adenocarcinoma. *, P values <0.05. GGNs, ground-glass nodules; LUL, left upper lobe; LLL, left lower lobe; RUL, right upper lobe; RML, right middle lobe; RLL, right lower lobe; pGGN, pure ground-glass nodule; mGGN, mixed ground-glass nodule; GGO, ground-glass opacity; HU, Hounsfield unit; PSC, percentage of solid components; SV, solid component volume; IQR, interquartile range.
The median time interval between the two follow-up CT scans for patients was found to be 5 months (IQR, 3–8 months), with no statistically significant differences between groups. The quantitative parameters on pre-surgical CT were found to be significantly associated with invasiveness, as evidenced by statistically significant differences in mass, mean density, PSC, SV, and volume (all P<0.001). The growth rates of GGNs from two CT scans, including diameter growth rate (P<0.001), relative diameter growth rate (P=0.001), mass growth rate (P<0.001), relative mass growth rate (P<0.001), SV growth rate (P<0.001), relative SV growth rate (P<0.001), volume growth rate (P<0.001), and relative volume growth rate (P<0.001) were significantly associated with invasiveness.
Development and validation of the invasiveness prediction models
There were 436 patients in the training set, 187 patients in the testing set, and 87 patients in the validation set (Table 4). A total of 10 features exhibiting non-zero coefficients were identified using LASSO regression analysis (Figure 4), including age, diameter, density, pleural indentation, vessel changes, mass, mean density, mass growth rate, relative mass growth rate, and volume growth rate.
Table 4
Factor | Training set (N=436) | Testing set (N=187) | Validation set (N=87) | ||||||||
---|---|---|---|---|---|---|---|---|---|---|---|
Group A (N=293) | Group B (N=143) | P value | Group A (N=117) | Group B (N=70) | P value | Group A (N=59) | Group B (N=28) | P value | |||
Sex | 0.19 | 0.03* | 0.89 | ||||||||
Female | 208 (70.99) | 92 (64.34) | 86 (73.50) | 40 (57.14) | 44 (74.58) | 22 (78.57) | |||||
Male | 85 (29.01) | 51 (35.66) | 31 (26.50) | 30 (42.86) | 15 (25.42) | 6 (21.43) | |||||
Age, years | 53.00 (42.00, 60.00) |
58.00 (53.00, 64.50) |
<0.001* | 51.00 (40.00, 59.00) |
59.50 (54.00, 69.00) |
<0.001* | 54.00 (49.00,60.50) |
58.00 (51.75, 62.75) |
0.16 | ||
Smoking history | 29 (9.90) | 30 (20.98) | 0.002* | 8 (6.84) | 16 (22.86) | 0.003* | 6 (10.17) | 3 (10.71) | >0.99 | ||
History of another malignant tumor | 22 (7.51) | 7 (4.90) | 0.30 | 16 (13.68) | 8 (11.43) | 0.66 | 3 (5.08) | 3 (10.71) | 0.61 | ||
Pathological T staging† | <0.001* | <0.001* | <0.001* | ||||||||
Tis | 136 (46.42) | 0 (0.00) | 55 (47.01) | 0 (0.00) | 16 (27.12) | 0 (0.00) | |||||
T1a(mi) | 152 (51.88) | 0 (0.00) | 60 (51.28) | 0 (0.00) | 43 (72.88) | 0 (0.00) | |||||
T1a | 0 | 45 (31.47) | 0 | 18 (25.71) | 0 | 15 (53.57) | |||||
T1b | 0 | 78 (54.55) | 0 | 45 (64.29) | 0 | 12 (42.86) | |||||
T1c | 0 | 20 (13.99) | 0 | 7 (10.00) | 0 | 1 (3.57) | |||||
T2a | 0 | 6 (4.20) | 0 | 0 | 0 | 0 (0.00) | |||||
Histologic subtypes | <0.001* | <0.001* | <0.001* | ||||||||
AHH | 5 (1.71) | 0 (0.00) | 2 (1.71) | 0 (0.00) | 0 (0.00) | 0 | |||||
AIS | 136 (46.42) | 0 (0.00) | 55 (47.01) | 0 (0.00) | 16 (27.12) | 0 | |||||
MIA | 152 (51.88) | 0 (0.00) | 60 (51.28) | 0 (0.00) | 43 (72.88) | 0 | |||||
LPA | 0 | 26 (18.31) | 0 | 16 (22.86) | 0 | 5 (17.86) | |||||
APA | 0 | 79 (55.63) | 0 | 28 (40.00) | 0 | 18 (64.29) | |||||
MPA | 0 | 1 (0.70) | 0 | 1 (1.43) | 0 | 0 (0.00) | |||||
PPA | 0 | 30 (21.13) | 0 | 23 (32.86) | 0 | 5 (17.86) | |||||
SPA | 0 | 3 (2.11) | 0 | 1 (1.43) | 0 | 0 | |||||
MA | 0 | 3 (2.11) | 0 | 1 (1.43) | 0 | 0 | |||||
Lymphatic invasion | 0 | 0 (0.00) | – | 0 | 0 (0.00) | – | 0 | 0 | – | ||
Perineural invasion | 0 | 0 (0.00) | – | 0 | 1 (1.43) | 0.37 | 0 | 0 | – | ||
Pleural invasion | 0 | 6 (4.20) | 0.001* | 0 | 0 (0.00) | – | 0 | 0 | – | ||
Surgical margin | 0 | 0 (0.00) | – | 0 | 0 (0.00) | – | 0 | 1 (3.57) | 0.32 | ||
Spread through air space | 0 | 9 (6.29) | <0.001* | 0 | 6 (8.57) | 0.002* | 0 | 3 (10.71) | 0.03* | ||
Vascular invasion | 0 | 0 | – | 0 | 0 | – | 0 | 0 (0.00) | – | ||
ALK rearrangement | 1 (0.34) | 4 (2.80) | 0.04* | 0 | 3 (4.29) | 0.051 | 0 | 1 (3.57) | 0.32 | ||
EGFR | 8 (2.73) | 28 (19.58) | <0.001* | 4 (3.42) | 13 (18.57) | 0.001* | 0 | 3 (10.71) | 0.03* | ||
Follow-up, months | 6.00 (3.00, 9.00) |
5.00 (3.00, 8.00) |
0.11 | 4.00 (2.00, 8.00) |
4.50 (3.00, 9.00) |
0.42 | 5.00 (3.00, 8.00) |
4.00 (2.00, 6.00) |
0.19 | ||
Location | 0.74 | 0.07 | 0.90 | ||||||||
LUL | 86 (29.35) | 43 (30.07) | 40 (34.19) | 14 (20.00) | 11 (18.64) | 5 (17.86) | |||||
LLL | 38 (12.97) | 17 (11.89) | 9 (7.69) | 8 (11.43) | 9 (15.25) | 4 (14.29) | |||||
RUL | 103 (35.15) | 50 (34.97) | 45 (38.46) | 29 (41.43) | 20 (33.90) | 12 (42.86) | |||||
RML | 25 (8.53) | 8 (5.59) | 4 (3.42) | 0 | 8 (13.56) | 2 (7.14) | |||||
RLL | 41 (13.99) | 25 (17.48) | 19 (16.24) | 19 (27.14) | 11 (18.64) | 5 (17.86) | |||||
Density | <0.001* | <0.001* | 0.004* | ||||||||
pGGN | 175 (59.73) | 20 (13.99) | 64 (54.70) | 15 (21.43) | 21 (35.59) | 2 (7.14) | |||||
mGGN | 118 (40.27) | 123 (86.01) | <0.001* | 53 (45.30) | 55 (78.57) | 0.002* | 38 (64.41) | 26 (92.86) | 0.08 | ||
GGO-dominant | 111 (94.07) | 87 (70.73) | 53 (100.00) | 42 (76.36) | 36 (94.74) | 21 (80.77) | |||||
Solid-dominant | 7 (5.93) | 36 (29.27) | 0 | 13 (23.64) | 2 (5.26) | 5 (19.23) | |||||
Shape | <0.001* | <0.001* | 0.09 | ||||||||
Round or oval | 211 (72.01) | 66 (46.15) | 81 (69.23) | 26 (37.14) | 42 (71.19) | 14 (50.00) | |||||
Irregular | 82 (27.99) | 77 (53.85) | 36 (30.77) | 44 (62.86) | 17 (28.81) | 14 (50.00) | |||||
Uniformity | <0.001* | <0.001* | 0.009* | ||||||||
Homogeneous | 106 (36.18) | 11 (7.69) | 36 (30.77) | 6 (8.57) | 16 (27.12) | 1 (3.57) | |||||
Heterogeneous | 187 (63.82) | 132 (92.31) | 81 (69.23) | 64 (91.43) | 43 (72.88) | 27 (96.43) | |||||
Margin | <0.001* | <0.001* | 0.06 | ||||||||
Smooth | 228 (77.82) | 70 (48.95) | 94 (80.34) | 27 (38.57) | 45 (76.27) | 15 (53.57) | |||||
Coarse | 65 (22.18) | 73 (51.05) | 23 (19.66) | 43 (61.43) | 14 (23.73) | 13 (46.43) | |||||
Nodule lung interface | 0.85 | 0.42 | 0.54 | ||||||||
Well-defined | 209 (71.33) | 104 (72.73) | 80 (68.38) | 43 (61.43) | 49 (83.05) | 25 (89.29) | |||||
Ill-defined | 84 (28.67) | 39 (27.27) | 37 (31.62) | 27 (38.57) | 10 (16.95) | 3 (10.71) | |||||
Pleural indentation | 66 (22.53) | 85 (59.44) | <0.001* | 30 (25.64) | 31 (44.29) | 0.01* | 19 (32.20) | 12 (42.86) | 0.47 | ||
Lobulation | 194 (66.21) | 114 (79.72) | 0.005* | 82 (70.09) | 55 (78.57) | 0.27 | 28 (47.46) | 21 (75.00) | 0.03* | ||
Spiculation | 44 (15.02) | 70 (48.95) | <0.001* | 17 (14.53) | 32 (45.71) | <0.001* | 5 (8.47) | 8 (28.57) | 0.03* | ||
Air bronchogram | 51 (17.41) | 47 (32.87) | <0.001* | 19 (16.24) | 27 (38.57) | 0.001* | 12 (20.34) | 8 (28.57) | 0.56 | ||
Vascular convergence sign | 0 | 1 (0.70) | 0.33 | 0 | 3 (4.29) | 0.051 | 0 | 0 | – | ||
Vacuole sign | 156 (53.24) | 77 (53.85) | 0.99 | 52 (44.44) | 36 (51.43) | 0.44 | 28 (47.46) | 19 (67.86) | 0.12 | ||
Cavity | 2 (0.68) | 11 (7.69) | <0.001* | 1 (0.85) | 7 (10.00) | 0.005* | 0 | 0 | – | ||
Vessel changes | 0.004* | <0.001* | 0.003* | ||||||||
Type I | 258 (88.05) | 110 (76.92) | 105 (89.74) | 48 (68.57) | 49 (83.05) | 14 (50.00) | |||||
Type II | 35 (11.95) | 33 (23.08) | 12 (10.26) | 22 (31.43) | 10 (16.95) | 14 (50.00) | |||||
Halo sign | 0 | 1 (0.70) | 0.33 | 0 | 1 (1.43) | 0.37 | 0 | 0 | – | ||
Pleural effusion | 2 (0.68) | 0 | >0.99 | 0 | 0 | – | 0 | 0 | – | ||
Pleural thickening | 30 (10.24) | 34 (23.78) | <0.001* | 14 (11.97) | 10 (14.29) | 0.82 | 5 (8.47) | 3 (10.71) | 0.71 | ||
Calcification | 0 | 0 | – | 0 | 1 (1.43) | 0.37 | 0 | 0 | – | ||
Lymphadenopathy | 0 | 2 (1.40) | 0.107 | 3 (2.56) | 2 (2.86) | >0.99 | 0 | 0 | – | ||
Diameter, mm | 8.00 (8.00, 12.00) | 14.00 (10.00, 18.00) |
<0.001* | 9.00 (8.00, 11.00) |
13.00 (11.00, 17.00) |
<0.001* | 9.00 (7.00, 12.00) |
13.50 (9.75, 16.25) |
<0.001* | ||
Diameter growth rate, mm/month | 0.00 (0.00, 0.17) |
0.17 (0.00, 0.35) |
<0.001* | 0.00 (0.00, 0.25) |
0.11 (0.00, 0.44) |
0.04* | 0.00 (−0.15, 0.14) |
0.23 (0.00, 0.70) |
0.009* | ||
Relative diameter growth rate, %/month | 0.00 (0.00, 1.52) |
0.86 (0.00, 2.16) |
0.006* | 0.00 (0.00, 2.08) |
0.61 (0.00, 2.56) |
0.11 | 0.00 (−1.22, 1.12) |
1.24 (0.00, 4.13) |
0.02* | ||
Mass, mg | 117.77 (76.67, 220.55) |
636.21 (261.76, 1273.39) |
<0.001* | 117.51 (73.51, 194.65) |
532.47 (245.03, 1167.90) |
<0.001* | 143.32 (82.80, 247.27) |
426.73 (217.59, 737.60) |
<0.001* | ||
Mass growth rate, mg/month | 0.70 (−0.78, 3.92) |
11.22 (2.18, 35.68) |
<0.001* | 1.43 (−0.84, 5.72) |
11.01 (−0.96, 28.88) |
<0.001* | 0.28 (−1.35, 4.16) |
16.29 (4.02, 29.42) |
<0.001* | ||
Relative mass growth rate, %/month | 0.83 (−0.82, 2.98) |
2.92 (0.62, 5.95) |
<0.001* | 1.52 (−0.95, 4.84) |
2.54 (−0.34, 6.16) |
0.17 | 0.32 (−1.42, 3.03) |
3.44 (2.06, 7.72) |
<0.001* | ||
Mean density, HU | −591.00 (−656.00, −518.00) |
−470.00 (−545.00, −308.50) |
<0.001* | −602.00 (−668.00, −517.00) |
−473.50 (−587.25, −363.25) |
<0.001* | −529.00 (−630.50, −458.50) |
−442.50 (−523.50, −329.50) |
<0.001* | ||
Mean density growth rate, HU/month | −1.36 (−6.25, 3.67) |
−0.50 (−8.04, 6.54) |
0.31 | −1.00 (−7.60, 4.50) |
−2.55 (−13.38, 5.82) |
0.42 | −1.50 (−10.38, 3.58) |
−0.30 (−5.52, 6.44) |
0.79 | ||
Relative mean density growth rate, %/month | 0.28 (−0.64, 1.18) |
0.13 (−1.47, 1.88) |
0.41 | 0.16 (−0.72, 1.19) |
0.37 ( −1.04, 3.16) |
0.42 | 0.30 (−0.77, 1.77) |
0.07 (−1.75, 1.21) |
0.77 | ||
PSC, % | 4.57 (0.99, 10.17) |
18.50 (9.40, 45.46) |
<0.001* | 3.18 (0.91, 10.84) |
19.11 (6.98, 40.79) |
<0.001* | 9.75 (3.76, 19.25) |
24.09 (12.53, 39.24) |
<0.001* | ||
PSC growth rate, %/month |
−0.04 (−0.47, 0.14) |
0.00 (−0.91, 0.81) |
0.23 | −0.06 (−0.49, 0.22) |
−0.22 (−1.15, 0.67) |
0.54 | −0.03 (−1.12, 0.32) |
−0.09 (−0.89, 1.19) |
0.56 | ||
Relative PSC growth rate, %/month | −0.78 (−7.74, 4.27) |
0.00 (−3.97, 5.66) |
0.06 | −0.89 (−7.16, 6.46) |
−0.86 (−6.33, 4.10) |
0.82 | −1.61 (−7.43, 1.89) |
−0.36 (−5.79, 6.98) |
0.38 | ||
SV, mm3 | 11.00 (2.00, 35.00) |
216.00 (68.00, 486.50) |
<0.001* | 8.00 (2.00, 29.00) |
149.50 (59.00, 571.00) |
<0.001* | 23.00 (11.00, 58.00) |
160.50 (62.25, 293.75) |
<0.001* | ||
SV growth rate, mm3/month | 0.00 (−1.00, 0.92) | 4.00 (−0.42, 23.20) |
<0.001* | 0.00 (−1.00, 0.89) |
2.17 (−1.67, 16.58) |
0.01* | 0.00 (−3.25, 0.63) |
1.90 (−1.05, 12.88) |
0.01* | ||
Relative SV growth rate, %/month | 0.00 (−7.43, 7.14) |
3.28 (−0.75, 9.70) |
<0.001* | 0.00 (−7.16, 7.50) |
2.40 (−2.08, 8.33) |
0.10 | 0.00 (−6.05, 2.07) |
3.44 (−1.81, 11.28) |
0.04* | ||
Volume, mm3 | 229.00 (137.00, 429.00) |
1,340.00 (383.00, 2,428.50) |
<0.001* | 230.00 (141.00, 356.00) |
1,251.50 (399.75, 1,935.75) |
<0.001* | 266.00 (137.00, 554.50) |
815.50 (281.25, 1,784.50) |
<0.001* | ||
Volume growth rate, mm3/month | 2.73 (−1.67, 11.00) |
21.33 (2.76, 76.50) |
<0.001* | 1.86 (−1.13, 13.00) |
24.86 (0.80, 68.70) |
<0.001* | 1.27 (−2.29, 16.33) |
14.33 (2.05, 88.31) |
0.01* | ||
Relative volume growth rate, %/month | 1.38 (−0.92, 3.95) |
3.30 (0.40, 7.69) |
<0.001* | 0.95 (−1.08, 5.86) |
2.51 (0.13, 7.80) |
0.07 | 0.96 (−0.89, 6.27) |
4.40 (0.67, 9.18) |
0.11 |
Data are presented as median (IQR) or n (%). Group A: atypical adenomatous hyperplasia, adenocarcinoma in situ, and minimally invasive adenocarcinoma; Group B: invasive adenocarcinoma. *, P values <0.05. †, pathological T staging was based on the IASLC 8th TNM Lung Cancer Staging System. AHH, atypical adenomatous hyperplasia; AIS, adenocarcinoma in situ; MIA, minimally invasive adenocarcinoma; LPA, lepidic-predominant adenocarcinoma; APA, acinar-predominant adenocarcinoma; MPA, micropapillary-predominant adenocarcinoma; PPA, papillary-predominant adenocarcinoma; SPA, solid-predominant adenocarcinoma; MA, mucinous adenocarcinoma; ALK, anaplastic lymphoma kinase; EGFR, epidermal growth factor receptor; GGN, ground-glass nodule; pGGN, pure ground-glass nodule; mGGN, mixed ground-glass nodule; LUL, left upper lobe; LLL, left lower lobe; RUL, right upper lobe; RML, right middle lobe; RLL, right lower lobe; HU, Hounsfield unit; PSC, percentage of solid components; SV, solid component volume; IQR, interquartile range.
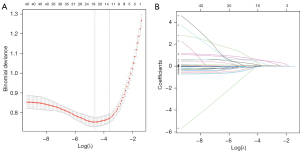
In the training set, the AUC for model 3 (0.911) was significantly higher than the AUC values of model 1 (0.855) and model 2 (0.895), which demonstrated statistical significance (model 1 vs. model 2, P<0.001; model 2 vs. model 3, P=0.02; model 1 vs. model 3, P<0.001) (Figure 5). The accuracy, sensitivity, specificity, positive predictive value (PPV) and negative predictive value (NPV) of model 3 were the highest, which were 84.40%, 87.41%, 82.94%, 71.43% and 93.10%, respectively (Table 5). In the testing set, the AUCs of model 1, 2, and 3 were 0.858, 0.891, and 0.893 respectively. Both model 3 and model 2 exhibited significantly higher AUC values compared to model 1 (model 1 vs. model 2, P=0.04; model 2 vs. model 3, P=0.51; model 1 vs. model 3, P=0.04) (Figure 5). Model 3 also showed the highest accuracy (83.96%), sensitivity (82.86%), specificity (84.62%), PPV (76.32%), and NPV (89.19%) (Table 5). In the validation set, model 3 (AUC 0.932) performed better than model 2 (AUC 0.903) and model 1 (AUC 0.816) (model 1 vs. model 2, P=0.02; model 2 vs. model 3, P=0.04; model 1 vs. model 3, P=0.004) (Figure 5), with the highest accuracy (86.21%), sensitivity (78.57%), specificity (89.83%), PPV (78.57%), and NPV (89.83%) (Table 5).
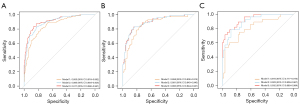
Table 5
Predicted results | TP | FP | FN | TN | ACC | SEN | SPE | PPV | NPV | Total patients |
---|---|---|---|---|---|---|---|---|---|---|
Training set | ||||||||||
Model 1 | 110 | 60 | 33 | 233 | 78.67% | 76.92% | 79.52% | 64.70% | 87.59% | 436 |
Model 2 | 120 | 53 | 23 | 240 | 82.57% | 83.92% | 81.91% | 69.36% | 91.25% | 436 |
Model 3 | 125 | 50 | 18 | 243 | 84.40% | 87.41% | 82.94% | 71.43% | 93.10% | 436 |
Testing set | ||||||||||
Model 1 | 59 | 28 | 11 | 89 | 79.14% | 84.29% | 76.07% | 67.82% | 89.00% | 187 |
Model 2 | 58 | 19 | 12 | 98 | 83.42% | 82.86% | 83.76% | 75.32% | 89.09% | 187 |
Model 3 | 58 | 18 | 12 | 99 | 83.96% | 82.86% | 84.62% | 76.32% | 89.19% | 187 |
Validation set | ||||||||||
Model 1 | 21 | 14 | 7 | 45 | 75.86% | 75.00% | 76.27% | 60.00% | 86.54% | 87 |
Model 2 | 22 | 7 | 6 | 52 | 85.06% | 78.57% | 88.14% | 75.86% | 89.66% | 87 |
Model 3 | 22 | 6 | 6 | 53 | 86.21% | 78.57% | 89.83% | 78.57% | 89.83% | 87 |
Model 1 utilized clinical characteristics and morphological features on pre-surgical CT, model 2 incorporated clinical characteristics, morphological features and quantitative parameters on pre-surgical CT, and model 3 incorporated clinical characteristics, morphological features and quantitative parameters on pre-surgical CT, and growth rates of GGNs. TP, true positive; FP, false positive; FN, false negative; TN, true negative; ACC, accuracy; SEN, sensitivity; SPE, specificity; PPV, positive predictive value; NPV, negative predictive value; CT, computed tomography.
The calibration curves of the models indicated good consistency (Figure 6, Figures S1,S2). The decision curves displayed the clinical usefulness of the three predictive models by comparing the net benefits at different threshold probabilities. The results demonstrated that the model 3 had a higher net benefit than model 1 and model 2 in the training and validation sets, and model 3 and model 2 had a higher net benefit than model 1 in the testing set (Figure 7). Model3 demonstrates superior performance and was used to establish a nomogram (Figure 8).
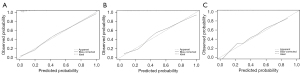
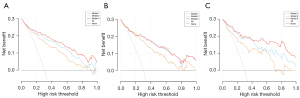
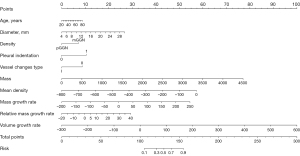
Discussion
With the application of low-dose computed tomography (LDCT), the incidence of lung adenocarcinoma presenting as GGN has increased (17,18). Precisely determining the invasiveness of GGNs is imperative for the advancement of personalized medicine. Thus, developing a model that accurately predicts the invasiveness of GGNs is essential. Unlike previous studies that primarily relied on pre-surgical CT scans, a nomogram model was developed utilizing morphological features and quantitative parameters from follow-up CT imaging in our study. The nomogram, including age, diameter, density, pleural indentation, vessel changes, mass, mean density, mass growth rate, relative mass growth rate, and volume growth rate, demonstrated good performance with AUC values of 0.893 in the testing set and 0.932 in the validation set.
Chu et al. (10) revealed that age, pleural indentation, and changes of internal vessels are important parameters for distinguishing between preinvasive and invasive GGNs. Tumor biology studies indicate that neoangiogenesis or vascular remodeling is a major tumor-initiating event (19,20). During lung adenocarcinoma progression, cancer cells release various pro-angiogenic factors, including vascular endothelial growth factor, which compensates for hypoxia by promoting neoangiogenesis or vasculature remodeling. Jin et al. (21) found that IAs tended to be significantly larger than MIAs, AISs, and AAHs. Zhu et al. (22) found that density was also correlated with the invasiveness of GGNs (odds ratio: 1.926). Su et al. (23) found that patients with clinical stage IA solid-dominant adenocarcinoma (C/T ratio >0.5) had a higher rate of recurrence after limited resection compared to lobectomy, indicating that limited resection should be performed cautiously in these cases. In our study, we observed that solid-dominant mGGNs were mostly IAs. However, the number of solid-dominant mGGNs in our study was small.
Quantifying GGN features using computer-aided measurement software ensures a degree of repeatability. With respect to CT attenuation, previous studies revealed that CT attenuation was an important factor in predicting invasiveness: higher CT attenuation was an indicator of GGNs with invasiveness (10,20). This could be due to the fact that the retained air space in non-invasive adenocarcinomas was larger than that in invasive adenocarcinomas. The reduced air space in invasive adenocarcinomas resulted from an increased tumor tissue component and thickening of the alveolar septa (24). Several studies have shown that mass is an important parameter for differentiating preinvasive nodules from invasive nodules (12,25). In our study, mass, and mean density were significantly greater in IAs compared to AHHs, AISs, or MIAs. This was mainly caused by an increased tumor tissue component and thickening of the alveolar septa in invasive adenocarcinomas (26).
Growth rate is known risk factors for lung cancer, and the growth is an important parameter that can predict the malignancy potential of GGNs and guide its management (2). Tao et al. have utilized volume doubling time to predict the invasiveness of pulmonary nodules (27). Our study incorporated mass growth rate, relative mass growth rate, and volume growth rate into the nomogram. Compared to model1 and model 2, the model 3 demonstrated superior discrimination capacity with higher AUC values in both the testing and validation sets. The nomogram showed sensitivity and specificity rates of 82.86% and 84.62% in the testing set, and 78.57% and 89.83% in the validation set, respectively. These results suggest that growth rates of GGNs derived from follow-up CT imaging can enhance model performance.
In our study, we observed that compared to the Group A, Group B had a higher proportion of patients with a history of smoking. This may be attributed to smoking being an important factor affecting tumorigenesis and development, closely associated with an immunosuppressive tumor microenvironment (28). Compared to Group A, Group B exhibited a higher incidence of pleural invasion and a spread through air space. This finding is attributed to the higher invasiveness of IA compared to AAH, AIS, and MIA. AAH denotes a localized proliferation of mildly to moderately atypical type II pneumocytes and/or Clara cells along alveolar walls and sometimes respiratory bronchioles, typically ≤0.5 cm in size. AIS is a small adenocarcinoma (≤3 cm) that remains confined to neoplastic cells lining preexisting alveolar structures (lepidic growth) without invasion into stromal, vascular, or pleural tissues. MIA refers to a solitary adenocarcinoma (≤3 cm) with a predominantly lepidic pattern, showing invasion of ≤5 mm in any one focus (23).
ALK rearrangement and EGFR mutation were more prevalent in Group B compared to Group A. EGFR is a transmembrane tyrosine kinase receptor playing crucial roles in cancer cell proliferation and growth. Kobayashi et al. revealed that EGFR mutations are significantly associated with tumor growth, and EGFR-mutated AAH develops a linear progression schema, whereby AAH progresses to AIS and is followed by IA (29). ALK gene rearrangements occur in approximately 5.6% of non-small cell lung cancer (30). In our study, patients had a slightly lower ALK rearrangement rate (1.27%), which may be due to ALK positivity being more frequently found in advanced lung adenocarcinoma. Han et al. (31) revealed that ALK positive status was related to advanced tumour stage. This is similar to our finding.
Our study had several limitations. First, it is a single-center study, and further multi-center studies are needed to validate our findings. Second, the majority of patients underwent two CT scans within a year, whose follow-up time was limited. Third, although internal validation showed that the model had optimal discrimination and excellent calibration, the generalizability of this nomogram still requires external validation using additional databases, particularly from other countries, considering the differences in epidemiology and clinical behavior among different ethnic groups. Forth, the included lesions are all early-stage lung adenocarcinomas, rendering the model less applicable to advanced-stage lung cancer.
Conclusions
In conclusion, we developed and validated a novel nomogram combined morphological features and quantitative parameters from follow-up CT imaging for predicting the invasiveness of lung adenocarcinoma manifesting as GGNs. The nomogram is easy to use, highly accurate, and exhibits excellent calibration. It could assist clinicians in making individualized predictions of each patient and guiding GGNs management.
Acknowledgments
We would like to thank all colleagues for helping us during the current study and all the selfless volunteers who participated in the study.
Funding: This work was supported by
Footnote
Reporting Checklist: The authors have completed the TRIPOD reporting checklist. Available at https://tlcr.amegroups.com/article/view/10.21037/tlcr-24-492/rc
Data Sharing Statement: Available at https://tlcr.amegroups.com/article/view/10.21037/tlcr-24-492/dss
Peer Review File: Available at https://tlcr.amegroups.com/article/view/10.21037/tlcr-24-492/prf
Conflicts of Interest: All authors have completed the ICMJE uniform disclosure form (available at https://tlcr.amegroups.com/article/view/10.21037/tlcr-24-492/coif). C.D. is an employee of Bayer Healthcare. The other authors have no conflicts of interest to declare.
Ethical Statement: The authors are accountable for all aspects of the work in ensuring that questions related to the accuracy or integrity of any part of the work are appropriately investigated and resolved. The study was conducted in accordance with the Declaration of Helsinki (as revised in 2013). The study was approved by the Ethics Committee of Wuhan Union Hospital (Approval No. 0516)
Open Access Statement: This is an Open Access article distributed in accordance with the Creative Commons Attribution-NonCommercial-NoDerivs 4.0 International License (CC BY-NC-ND 4.0), which permits the non-commercial replication and distribution of the article with the strict proviso that no changes or edits are made and the original work is properly cited (including links to both the formal publication through the relevant DOI and the license). See: https://creativecommons.org/licenses/by-nc-nd/4.0/.
References
- Gao S, Li N, Wang S, et al. Lung Cancer in People’s Republic of China. J Thorac Oncol 2020;15:1567-76. [Crossref] [PubMed]
- Ricciardi S, Booton R, Petersen RH, et al. Managing of screening-detected sub-solid nodules-a European perspective. Transl Lung Cancer Res 2021;10:2368-77. [Crossref] [PubMed]
- Schreuder A, Scholten ET, van Ginneken B, Jacobs C. Artificial intelligence for detection and characterization of pulmonary nodules in lung cancer CT screening: ready for practice? Transl Lung Cancer Res 2021;10:2378-88. [Crossref] [PubMed]
- Obayashi K, Shimizu K, Nakazawa S, et al. The impact of histology and ground-glass opacity component on volume doubling time in primary lung cancer. J Thorac Dis 2018;10:5428-34. [Crossref] [PubMed]
- Silva M, Prokop M, Jacobs C, et al. Long-Term Active Surveillance of Screening Detected Subsolid Nodules is a Safe Strategy to Reduce Overtreatment. J Thorac Oncol 2018;13:1454-63. [Crossref] [PubMed]
- Liu S, Wang R, Zhang Y, et al. Precise Diagnosis of Intraoperative Frozen Section Is an Effective Method to Guide Resection Strategy for Peripheral Small-Sized Lung Adenocarcinoma. J Clin Oncol 2016;34:307-13. [Crossref] [PubMed]
- Su H, Gu C, She Y, et al. Predictors of upstage and treatment strategies for stage IA lung cancers after sublobar resection for adenocarcinoma in situ and minimally invasive adenocarcinoma. Transl Lung Cancer Res 2021;10:32-44. [Crossref] [PubMed]
- Wu G, Woodruff HC, Shen J, et al. Diagnosis of Invasive Lung Adenocarcinoma Based on Chest CT Radiomic Features of Part-Solid Pulmonary Nodules: A Multicenter Study. Radiology 2020;297:451-8. Erratum in: Radiology 2020;297:E282. [Crossref] [PubMed]
- Liu RS, Ye J, Yu Y, et al. The predictive accuracy of CT radiomics combined with machine learning in predicting the invasiveness of small nodular lung adenocarcinoma. Transl Lung Cancer Res 2023;12:530-46. [Crossref] [PubMed]
- Chu ZG, Li WJ, Fu BJ, et al. CT Characteristics for Predicting Invasiveness in Pulmonary Pure Ground-Glass Nodules. AJR Am J Roentgenol 2020;215:351-8. [Crossref] [PubMed]
- Wang MM, Li JQ, Dou SH, et al. Lack of incremental value of three-dimensional measurement in assessing invasiveness for lung cancer. Eur J Cardiothorac Surg 2023;64:ezad373. [Crossref] [PubMed]
- Ko JP, Suh J, Ibidapo O, et al. Lung Adenocarcinoma: Correlation of Quantitative CT Findings with Pathologic Findings. Radiology 2016;280:931-9. [Crossref] [PubMed]
- Liu J, Xie C, Li Y, et al. The solid component within part-solid nodules: 3-dimensional quantification, correlation with the malignant grade of nonmucinous pulmonary adenocarcinomas, and comparisons with 2-dimentional measures and semantic features in low-dose computed tomography. Cancer Imaging 2023;23:65. [Crossref] [PubMed]
- Jin C, Cao J, Cai Y, et al. A nomogram for predicting the risk of invasive pulmonary adenocarcinoma for patients with solitary peripheral subsolid nodules. J Thorac Cardiovasc Surg 2017;153:462-469.e1. [Crossref] [PubMed]
- Qiu ZB, Zhang C, Chu XP, et al. Quantifying invasiveness of clinical stage IA lung adenocarcinoma with computed tomography texture features. J Thorac Cardiovasc Surg 2022;163:805-815.e3. [Crossref] [PubMed]
- Detterbeck FC, Boffa DJ, Kim AW, et al. The Eighth Edition Lung Cancer Stage Classification. Chest 2017;151:193-203.
- Li R, Li X, Xue R, et al. Early metastasis detected in patients with multifocal pulmonary ground-glass opacities (GGOs). Thorax 2018;73:290-2. [Crossref] [PubMed]
- Kim YW, Kwon BS, Lim SY, et al. Lung cancer probability and clinical outcomes of baseline and new subsolid nodules detected on low-dose CT screening. Thorax 2021;76:980-8. [Crossref] [PubMed]
- Gao F, Li M, Ge X, et al. Multi-detector spiral CT study of the relationships between pulmonary ground-glass nodules and blood vessels. Eur Radiol 2013;23:3271-7. [Crossref] [PubMed]
- Seaman S, Zhu Z, Saha S, et al. Eradication of Tumors through Simultaneous Ablation of CD276/B7-H3-Positive Tumor Cells and Tumor Vasculature. Cancer Cell 2017;31:501-515.e8. [Crossref] [PubMed]
- Jin X, Zhao SH, Gao J, et al. CT characteristics and pathological implications of early stage (T1N0M0) lung adenocarcinoma with pure ground-glass opacity. Eur Radiol 2015;25:2532-40. [Crossref] [PubMed]
- Zhu M, Yang Z, Wang M, et al. A computerized tomography-based radiomic model for assessing the invasiveness of lung adenocarcinoma manifesting as ground-glass opacity nodules. Respir Res 2022;23:96. [Crossref] [PubMed]
- Su H, Dai C, Xie H, et al. Risk Factors of Recurrence in Patients With Clinical Stage IA Adenocarcinoma Presented as Ground-Glass Nodule. Clin Lung Cancer 2018;19:e609-e617. [Crossref] [PubMed]
- Eguchi T, Kondo R, Kawakami S, et al. Computed tomography attenuation predicts the growth of pure ground-glass nodules. Lung Cancer 2014;84:242-7. [Crossref] [PubMed]
- Song Q, Song B, Li X, et al. A CT-based nomogram for predicting the risk of adenocarcinomas in patients with subsolid nodule according to the 2021 WHO classification. Cancer Imaging 2022;22:46. [Crossref] [PubMed]
- Yoshida M, Yuasa M, Ogawa H, et al. Can computed tomography differentiate adenocarcinoma in situ from minimally invasive adenocarcinoma? Thorac Cancer 2021;12:1023-32. [Crossref] [PubMed]
- Tao G, Shi D, Yu L, et al. Longitudinal prediction of lung nodule invasiveness by sequential modelling with common clinical computed tomography (CT) measurements: a prediction accuracy study. Transl Lung Cancer Res 2022;11:845-57. [Crossref] [PubMed]
- Sato K, Mimaki S, Yamashita R, et al. Association between the mutational smoking signature and the immune microenvironment in lung adenocarcinoma. Lung Cancer 2020;147:12-20. [Crossref] [PubMed]
- Kobayashi Y, Mitsudomi T, Sakao Y, et al. Genetic features of pulmonary adenocarcinoma presenting with ground-glass nodules: the differences between nodules with and without growth. Ann Oncol 2015;26:156-61. [Crossref] [PubMed]
- Li W, Zhang J, Wang Z, et al. Guidelines for clinical practice of ALK fusion detection in non-small-cell lung cancer: a proposal from the Chinese RATICAL study group. J Natl Cancer Cent 2021;1:123-31. [Crossref] [PubMed]
- Han X, Fan J, Gu J, et al. CT features associated with EGFR mutations and ALK positivity in patients with multiple primary lung adenocarcinomas. Cancer Imaging 2020;20:51. [Crossref] [PubMed]