Predicting prognosis in patients with stage IA lung adenocarcinoma with a micropapillary component using a nomogram based on computed tomography radiomics and clinicopathologic factors: a retrospective analysis
Highlight box
Key findings
• We identified clinicopathological factors and radiomics features that can predict prognosis in patients with stage IA lung adenocarcinoma containing a micropapillary (MIP) component.
What is known and what is new?
• While the high-risk factors for poor prognosis in patients with MIP-positive lung adenocarcinoma have been analyzed, whether stage IA lung adenocarcinoma has a higher risk of recurrence after surgery and the need for postoperative adjuvant chemotherapy remains unresolved.
• T1c stage, an MIP percentage ≥1%, spread through air space, a preoperative carcinoembryonic antigen level >5 µg/L, and radiomics score were independent predictors of disease-free survival and overall survival in patients with stage IA lung adenocarcinoma containing an MIP component.
What is the implication, and what should change now?
• We established and validated a nomogram based on clinicopathologic factors and radiomics features, which can be used as a powerful tool for predicting postoperative recurrence and survival in patients with stage IA lung adenocarcinoma containing an MIP component.
Introduction
Background
Globally, lung cancer is the primary cause of cancer-related deaths, and adenocarcinoma (ADC) is the most common histological type of lung cancer (1). Patients with early-stage lung ADC still have a high probability of recurrence and metastasis after surgical resection. The study by Ito et al. assessed the 10-year overall survival (OS) rate after lobectomy in patients with clinical T1 N0 lung cancer to be 80.4% and the 10-year recurrence-free survival rate to be 77.1% (2). Nomori et al. followed up patients with early-stage postoperative lung cancer and found that the 10-year probability of recurrence-free survival was 97% for T1a, 69% for T1b, and 69% for T1c (3). Patients with early-stage lung ADC have a higher risk of lymph node metastasis after surgical resection (approximately 20–40%) (4,5). Therefore, to improve the postoperative survival of patients with early-stage lung ADC, the International Association for the Study of Lung Cancer (IASLC)/American Thoracic Society (ATS)/European Respiratory Society (ERS) proposed a new classification system in 2011 to categorize invasive lung ADC into the following five subtypes: lepidic, acinar, papillary, micropapillary (MIP), and solid predominant (6).
Rationale and knowledge gap
The MIP subtype is characterized by a poor prognosis and high aggressiveness and is usually accompanied by extensive lymphovascular invasion and lymph node metastases (7-9). Even with early surgical resection, a high risk of lymph node metastasis remains (10-12). However, the decision on whether to perform postoperative adjuvant chemotherapy (ACT) in patients with stage IA lung ADC with an MIP component remains inconclusive. The 2023 National Comprehensive Cancer Guidelines only recommend postoperative ACT in patients with stage IB lung ADC with high-risk factors, and the guidelines on the need for ACT in those with an MIP component in stage IA are not mentioned (13). However, the results of a retrospective analysis by Wang et al. showed that postoperative ACT was a favorable prognostic factor in patients with stage IA lung ADC with an MIP component and that they could benefit from ACT (9). Therefore, there is a need for a predictive model that can better identify patients at higher risk of recurrence based on clinicopathological factors, such as an MIP component, and administer postoperative ACT to reduce the risk of recurrence and improve survival. Radiomics is a quantitative analysis tool that is promising for cancer diagnosis, lymph node metastasis prediction, and prognosis. It works by high-throughput extraction of quantitative features from medical images to create mineable data (14-16). Studies have been conducted to analyze the high-risk factors for poor prognosis in patients with MIP-positive lung ADC, including T-stage and preoperative carcinoembryonic antigen (CEA) levels (17-20).
Objective
The question of whether patients with MIP-containing stage IA lung ADC have a higher risk of recurrence after surgery and whether postoperative ACT should be given remains unresolved. Therefore, this study aimed to comprehensively analyze radiomics features and clinicopathological factors to identify predictive factors affecting the prognosis of patients with stage IA lung ADC containing an MIP component and those patients with a high risk of recurrence in order to guide clinical treatment and improve prognosis. We present this article in accordance with the TRIPOD reporting checklist (available at https://tlcr.amegroups.com/article/view/10.21037/tlcr-24-544/rc).
Methods
Patient selection
The study was conducted in accordance with the Declaration of Helsinki (as revised in 2013). The study was approved by Institutional Ethics Committee of Cancer Hospital Affiliated to Shandong First Medical University (No. SDTHEC2023011030) and individual consent for this retrospective analysis was waived. Patients with stage IA lung ADC who underwent radical lung cancer resection and whose pathological results showed an MIP component from January 2012 to December 2018 at the Shandong Cancer Hospital and Institute were retrospectively analyzed in this study. The inclusion criteria were as follows: (I) patients with pathologically confirmed lung ADC containing an MIP component and negative margins; (II) pathological stage pT1a–1cN0M0; (III) patients without significant cardiopulmonary abnormalities and specific postoperative complications; and (IV) patients who did not receive any adjuvant therapy postoperatively. Exclusion criteria included: (I) no record of CT examination at our study institution prior to surgical treatment; (II) pathological confirmation of the presence of a solid subtype; (III) death from non-cancer causes; and (IV) incomplete documentation of imaging information or clinicopathologic information and loss of visits. Ultimately, 254 patients with stage IA lung ADC with an MIP component were eligible for this study and were randomly assigned to the training (n=169) and validation (n=85) cohorts in a 7:3 ratio using the “complete_ra” function of the “randomizr” package in R software version 4.3.2 (Figure 1). The Ethics Committee of the Shandong Cancer Hospital and Institute approved this study, within which the International Union Against Cancer/American Joint Committee on Cancer 8th edition tumor-node-metastasis (TNM) staging system was used (21).
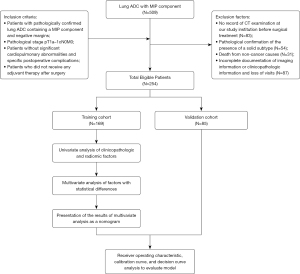
Surgical treatment
All patients underwent a multidisciplinary consultation to assess the disease and select an appropriate treatment plan before starting treatment, and they were clinically evaluated as having resectable non-small-cell lung cancer (NSCLC). The surgical modalities included video-assisted thoracoscopic surgery or thoracotomy. Lung resection methods included lobectomy, sleeve resection, and segmentectomy. All patients were returned to the thoracic surgery department postoperatively, encouraged to cough and expectorate sputum to promote drainage and lung re-expansion, and instructed to perform activities soon after surgery.
Study endpoints and follow-up
The study endpoints in this study were OS and disease-free survival (DFS), with OS defined as the span between radical lung cancer resection and death due to cancer or the last follow-up. DFS was defined as the time interval between radical resection of lung cancer and the first recorded recurrence, death due to a cancer-related cause, or the last follow-up visit. All enrolled patients received regular outpatient reviews and telephone follow-up after admission, with regular physical examination and chest-enhancing computed tomography (CT) during follow-up, as well as positron emission tomography (PET)-CT, ultrasound, endoscopy, magnetic resonance imaging, or whole-body skeletal imaging when necessary. For patients whose last case record in the case system was recorded more than 1 month before the cut-off time of this study, telephone follow-up was used to complete the collection of patients’ clinical data and establish a database for statistical analysis, and they were asked for details of their disease progression and survival. Follow-up ended on July 31, 2023, and the median duration of follow-up for all patients was 73 months (range, 17–133 months).
CT image acquisition
All patients underwent enhanced CT scanning of the chest. Enhanced scanning was performed by injecting the iodine contrast agent iohexol (350 mg I/mL) through the anterior vein of the elbow using a high-pressure syringe at an injection rate of 3.0 mL/s with a dose of 60–100 mL of iodine contrast. CT-enhanced images of the arterial and venous phases were acquired 20 and 40 s after the iodine contrast injection, respectively. The scanning parameters used were as follows: voltage 120 kV, tube current 200 mA, reconstruction layer thickness 5 mm, reconstruction interval 5 mm, and matrix 512×512. CT images obtained from the scan were uploaded to the Picture Archiving and Communication System, and the enhanced CT images were exported in DICOM format. Scanning area: scanning layer by layer from top to bottom, starting from the thyroid cartilage and ending at the lower edge of the liver.
Radiomics
CT scans obtained before radical lung cancer resection in patients with stage IA lung ADC containing an MIP component were analyzed in this study. If multiple CT scans were available, the most recent CT scan preoperatively was used. All tumor target areas were manually depicted layer by layer by an oncologist using the medical image processing and navigation software 3D Slicer (version 5.2.1). The regions of interest were subsequently confirmed by a radiologist with advanced experience in chest CT analysis. All data were Z-score normalized to reduce the variation between images from different patients. To reduce any bias or overfitting caused by many features, feature selection was conducted through a two-step process as follows: first, features with high repeatability and stability were screened by calculating the intra-class correlation coefficient (ICC) index, and features were screened with the criterion of an ICC index >0.9. Features with high repeatability were screened; subsequently, these features were further downscaled and screened for key features using the least absolute shrinkage and selection operator (LASSO) regression (22,23). Finally, a radiomics score (Rad score) was calculated for each patient by weighting the selected features according to their respective coefficients (24).
Model construction and evaluation
Based on previous studies on the prognosis of patients with MIP-containing lung ADC, we retrospectively analyzed clinicopathological information, such as spread through air space (STAS), the proportion of MIP components, and radiomics information (7-10). First, a univariate Cox proportional hazard model was used in the training cohort to evaluate the predictive ability of clinicopathological and radiomics features for OS and DFS. Next, factors with P<0.05 in univariate analyses were assessed in multivariate analyses. Finally, a nomogram was constructed using factors with significant predictive value in multivariate Cox regression analyses, and 3-, 5-, and 7-year risk values for death or progression were calculated for each patient in the training and validation cohorts. Subsequently, receiver operating characteristic (ROC) curves, calibration curves, and decision curve analysis (DCA) were used in the training and validation cohorts to evaluate the predictive performance of the nomogram for OS and DFS at 3, 5, and 7 years. We used X-tile software (version 3.6.1) to determine the optimal cut-off value for the risk value in the nomogram and classified the population into low- and high-risk groups for death or disease progression based on this cut-off value. OS and DFS survival analyses of patients in these two risk groups were performed in the training cohort and validation cohort to assess the prognosis of patients with different risks.
Statistical analysis
The independent samples t-test or rank-sum test was used to compare continuous variables; the Chi-squared or Fisher’s exact test was used to compare categorical variables. Univariate and multivariate analyses were performed to identify independent prognostic factors for OS and DFS using Cox proportional risk models. Patients’ DFS and OS were assessed and compared using the Kaplan-Meier method and log-rank test, respectively. All tests were two-sided, and statistical significance was set at P<0.05. All data analyses and graphing were performed using R software version 4.3.2.
Results
Patient characteristics
Overall, 254 patients with stage IA lung ADC with an MIP component were enrolled in this study; 112 (44.1%) and 142 (55.9%) patients were males and females, respectively. As of the end of follow-up, a total of 109 patients (42.9%) had progressed, of which 45 patients (41.3%) had local recurrence (the recurrence within the lung on the side where the primary tumor was located or local lymph node recurrence) and 64 patients (58.7%) had distant metastasis (nonregional lymph node metastasis, systemic metastasis, or malignant pleural effusion); a total of 92 patients (36.2%) died. Additionally, 124 (48.8%) and 130 (51.2%) patients were aged <60 and ≥60 years, respectively; 225 (88.6%) patients received lobectomy, and the remaining 29 (11.4%) underwent non-lobectomy. Of them, 169 and 85 patients were included in the training and validation cohorts, respectively, with death occurring in 64 (37.9%) and 28 (32.9%) and progression occurring in 71 (42.0%) and 38 (44.7%), respectively. Table 1 summarizes the baseline characteristics of the training and validation cohorts, with each clinical factor satisfying P>0.05.
Table 1
Characteristics | Training (n=169) | Validation (n=85) | P |
---|---|---|---|
Sex, n (%) | |||
Female | 89 (52.7) | 53 (62.4) | 0.18 |
Male | 80 (47.3) | 32 (37.6) | |
Age (years), n (%) | |||
<60 | 75 (44.4) | 49 (57.6) | 0.13 |
≥60 | 94 (55.6) | 36 (42.4) | |
Smoking, n (%) | |||
Yes | 46 (27.2) | 22 (25.9) | 0.94 |
No | 123 (72.8) | 63 (74.1) | |
Drinking, n (%) | |||
Yes | 34 (20.1) | 21 (24.7) | 0.50 |
No | 135 (79.9) | 64 (75.3) | |
Family history, n (%) | |||
Yes | 37 (21.9) | 20 (23.5) | 0.89 |
No | 132 (78.1) | 65 (76.5) | |
MIP proportion, n (%) | |||
<1% | 78 (46.2) | 47 (55.3) | 0.39 |
1–5% | 49 (29.0) | 20 (23.5) | |
>5% | 42 (24.9) | 18 (21.2) | |
ECOG, n (%) | |||
0 | 10 (5.9) | 7 (8.2) | 0.67 |
1 | 159 (94.1) | 78 (91.8) | |
FEV1, n (%) | |||
≤70% | 23 (13.6) | 7 (8.2) | 0.30 |
>70% | 146 (86.4) | 78 (91.8) | |
T-stage, n (%) | |||
T1a–1b | 138 (81.7) | 69 (81.2) | >0.99 |
T1c | 31 (18.3) | 16 (18.8) | |
Surgical excision method, n (%) | |||
Lobectomy | 149 (88.2) | 76 (89.4) | 0.93 |
Non-lobectomy | 20 (11.8) | 9 (10.6) | |
Location, n (%) | |||
Lower lobe | 60 (35.5) | 31 (36.5) | 0.81 |
Middle lobe | 16 (9.5) | 10 (11.8) | |
Upper lobe | 93 (55.0) | 44 (51.8) | |
CEA level, n (%) | |||
≤5 μg/L | 128 (75.7) | 69 (81.2) | 0.41 |
>5 μg/L | 41 (24.3) | 16 (18.8) | |
STAS, n (%) | |||
Yes | 21 (12.4) | 9 (10.6) | 0.82 |
No | 148 (87.6) | 76 (89.4) | |
Length of surgery (minutes)† | 115.57±33.73 | 118.21±36.82 | 0.57 |
Amount of intraoperative bleeding (mL)† | 95.21±63.71 | 93.76±40.68 | 0.85 |
Rad score† | −1.99±2.22 | −1.79±1.87 | 0.47 |
†, data are presented as mean ± standard deviation. MIP, micropapillary; ECOG, Eastern Cooperative Oncology Group; FEV1, forced expiratory volume in one second; CEA, carcinoembryonic antigen; STAS, spread through air space; Rad score, radiomics score.
Univariate and multivariate analyses of prognosis in the training cohort
In the univariate analysis of patients’ clinicopathological factors, we found that the percentage of the MIP component (OS P<0.001, DFS P<0.001), STAS (OS P<0.001, DFS P<0.001), T-stage (OS P=0.009, DFS P=0.007), and CEA levels (OS P<0.001, DFS P<0.001) were significantly correlated with patients’ OS and DFS (Tables 2,3). After screening using the ICC index and LASSO regression, a total of 11 radiomics features most valuable for predicting OS were selected, and the calculated Rad score was −2.37±1.19 vs. −0.47±0.82 (P<0.001) in patients with and without death, respectively. The 12 radiomics features most valuable for predicting DFS were selected, and the Rad score was −1.58±2.56 vs. −2.07±2.10 (P=0.047) in patients with and without progression, respectively (Figure S1).
Table 2
Characteristics | Univariate analysis | Multivariate analysis | |||||
---|---|---|---|---|---|---|---|
Death (n=64) | No (n=105) | P value | Regression coefficient | HR (95% CI) | P value | ||
Sex, n (%) | |||||||
Female | 35 (54.7) | 54 (51.4) | 0.62 | ||||
Male | 29 (45.3) | 51 (48.6) | |||||
Age (years), n (%) | |||||||
<60 | 25 (39.1) | 49 (46.7) | 0.11 | ||||
≥60 | 39 (60.9) | 56 (53.3) | |||||
Smoking, n (%) | |||||||
Yes | 19 (29.7) | 27 (25.7) | 0.85 | ||||
No | 45 (70.3) | 78 (74.3) | |||||
Drinking, n (%) | |||||||
Yes | 15 (23.4) | 19 (18.1) | 0.55 | ||||
No | 49 (76.6) | 86 (81.9) | |||||
Family history, n (%) | |||||||
Yes | 10 (15.6) | 27 (25.7) | 0.28 | ||||
No | 54 (84.4) | 78 (74.3) | |||||
MIP proportion, n (%) | |||||||
<1% | 11 (17.2) | 67 (63.8) | <0.001 | Ref | Ref | ||
1–5% (ref = “<1%”) | 27 (42.2) | 22 (21.0) | 1.243 | 3.467 (1.635, 7.347) | 0.001 | ||
>5% (ref = “<1%”) | 26 (40.6) | 16 (15.2) | 0.830 | 2.294 (1.096, 4.805) | 0.03 | ||
>5% (ref = “1–5%”) | 0.27 | 0.413 | 1.511 (0.860, 2.655) | 0.15 | |||
FEV1, n (%) | |||||||
≤70% | 10 (15.6) | 13 (12.4) | 0.33 | ||||
>70% | 54 (84.4) | 92 (87.6) | |||||
T-stage, n (%) | |||||||
T1a–1b | 49 (76.6) | 89 (84.8) | 0.009 | Ref | Ref | ||
T1c | 15 (23.4) | 16 (15.2) | 1.329 | 3.779 (1.791, 7.972) | <0.001 | ||
Location, n (%) | |||||||
Lower lobe | 25 (39.1) | 35 (33.3) | 0.84 | ||||
Middle lobe | 6 (9.4) | 10 (9.5) | |||||
Upper lobe | 33 (51.6) | 60 (57.1) | |||||
CEA level, n (%) | |||||||
≤5 μg/L | 33 (51.6) | 95 (90.5) | <0.001 | Ref | Ref | ||
>5 μg/L | 31 (48.4) | 10 (9.5) | 0.892 | 2.410 (1.237, 3.711) | 0.001 | ||
STAS, n (%) | |||||||
Yes | 13 (20.3) | 8 (7.6) | <0.001 | 1.600 | 4.954 (2.252, 10.902) | <0.001 | |
No | 51 (79.7) | 97 (92.4) | Ref | Ref | |||
Surgical excision method, n (%) | |||||||
Lung lobectomy | 56 (87.5) | 93 (88.6) | 0.65 | ||||
Non-lobectomy | 8 (12.5) | 12 (11.4) | |||||
Length of surgery (minutes)† | 113.25±34.69 | 116.98±33.21 | 0.72 | ||||
Amount of intraoperative bleeding (mL)† | 86.41±37.35 | 100.57±75.06 | 0.28 | ||||
Rad score† | −2.37±1.19 | −0.47±0.82 | <0.001 | 0.797 | 2.219 (1.685, 2.923) | <0.001 |
†, data are presented as mean ± standard deviation. OS, overall survival; HR, hazard ratio; 95% CI, 95% confidence interval; MIP, micropapillary; FEV1, forced expiratory volume in one second; CEA, carcinoembryonic antigen; STAS, spread through air space; Rad score, radiomics score.
Table 3
Characteristics | Univariate analysis | Multivariate analysis | |||||
---|---|---|---|---|---|---|---|
Progression (n=71) | No (n=98) | P value | Regression coefficient | HR (95% CI) | P value | ||
Sex, n (%) | |||||||
Female | 38 (53.5) | 51 (52.0) | 0.89 | ||||
Male | 33 (46.5) | 47 (48.0) | |||||
Age (years), n (%) | |||||||
<60 | 26 (36.6) | 48 (49.0) | 0.10 | ||||
≥60 | 45 (63.4) | 50 (51.0) | |||||
Smoking, n (%) | |||||||
Yes | 23 (32.4) | 23 (23.5) | 0.37 | ||||
No | 48 (67.6) | 75 (76.5) | |||||
Drinking, n (%) | |||||||
Yes | 18 (25.4) | 16 (16.3) | 0.35 | ||||
No | 53 (74.6) | 82 (83.7) | |||||
Family history, n (%) | |||||||
Yes | 10 (14.1) | 27 (27.6) | 0.08 | ||||
No | 61 (85.9) | 71 (72.4) | |||||
MIP proportion, n (%) | |||||||
<1% | 17 (23.9) | 61 (62.2) | <0.001 | Ref | Ref | ||
1–5% (ref = “<1%”) | 29 (40.8) | 20 (20.4) | 1.335 | 3.800 (2.012, 7.178) | <0.001 | ||
>5% (ref = “<1%”) | 25 (35.2) | 17 (17.3) | 1.170 | 3.221 (1.634, 6.349) | <0.001 | ||
>5% (ref = “1–5%”) | 0.87 | −0.165 | 0.848 (0.474, 1.517) | 0.58 | |||
FEV1, n (%) | |||||||
≤70% | 10 (14.1) | 13 (13.3) | 0.69 | ||||
>70% | 61 (85.9) | 85 (86.7) | |||||
T-stage, n (%) | |||||||
T1a–1b | 54 (76.1) | 84 (85.7) | 0.007 | Ref | Ref | ||
T1c | 17 (23.9) | 14 (14.3) | 0.736 | 2.089 (1.157, 3.772) | 0.02 | ||
Location, n (%) | |||||||
Lower lobe | 27 (38.0) | 33 (33.7) | 0.82 | ||||
Middle lobe | 8 (11.3) | 8 (8.2) | |||||
Upper lobe | 36 (50.7) | 57 (58.2) | |||||
CEA level, n (%) | |||||||
≤5 μg/L | 39 (54.9) | 89 (90.8) | <0.001 | Ref | Ref | ||
>5 μg/L | 32 (45.1) | 9 (9.2) | 1.408 | 1.245 (1.144, 2.416) | <0.001 | ||
STAS, n (%) | |||||||
Yes | 14 (19.7) | 7 (7.1) | <0.001 | 1.055 | 2.872 (1.517, 5.436) | 0.001 | |
No | 57 (80.3) | 91 (92.9) | Ref | Ref | |||
Surgical excision method, n (%) | |||||||
Lung lobectomy | 63 (88.7) | 86 (87.8) | 0.93 | ||||
Non-lobectomy | 8 (11.3) | 12 (12.2) | |||||
Length of surgery (minutes)† | 113.30±33.52 | 117.21±33.95 | 0.31 | ||||
Amount of intraoperative bleeding (mL)† | 84.37±36.63 | 103.06±76.89 | 0.06 | ||||
Rad score† | −1.58±2.56 | −2.07±2.10 | 0.047 | 0.132 | 1.141 (1.016, 1.282) | 0.03 |
†, data are presented as mean ± standard deviation. DFS, disease-free survival; HR, hazard ratio; 95% CI, 95% confidence interval; MIP, micropapillary; FEV1, forced expiratory volume in one second; CEA, carcinoembryonic antigen; STAS, spread through air space; Rad score, radiomics score.
Multivariate Cox regression analysis was subjected to clinicopathological and imaging characteristics with P<0.05 in univariate analysis. In multivariate Cox regression analysis of OS, MIP percentages of 1–5% and >5% [hazard ratio (HR): 3.467, 95% confidence interval (CI): 1.635–7.347, P=0.001 and HR: 2.294, 95% CI: 1.096–4.805, P=0.03, respectively], STAS (HR: 4.954, 95% CI: 2.252–10.902, P<0.001), the T1c stage (HR: 3.779, 95% CI: 1.791–7.972, P<0.001), CEA level >5 µg/L (HR: 2.410, 95% CI: 1.237–3.711, P=0.001), and Rad score (HR: 2.219, 95% CI: 1.685–2.923, P<0.001) were five independent prognostic factors for OS. In multivariate Cox regression analysis of DFS, MIP percentages of 1–5% and >5% (HR: 3.800, 95% CI: 2.012–7.178, P<0.001 and HR: 3.221, 95% CI: 1.634–6.349, P<0.001, respectively), STAS (HR: 2.872, 95% CI: 1.517–5.436, P=0.001), T1c stage (HR: 2.089, 95% CI: 1.157–3.772, P=0.02), CEA >5 µg/L (HR: 1.245, 95% CI: 1.144–2.416, P<0.001), and Rad score (HR: 1.141, 95% CI: 1.016–1.282, P=0.03) were five independent prognostic factors for DFS.
Establishment and evaluation of the nomogram
Based on the multivariate Cox regression analysis, the predictive model was visualized in the form of a nomogram (Figure 2). A ROC was created for the model to assess its predictive efficacy (Figure 3). In the training cohort, the area under the curve (AUC) values of the nomogram predicting 3-, 5-, and 7-year OS were 0.910 (95% CI: 0.787–0.933), 0.914 (95% CI: 0.835–0.973), and 0.904 (95% CI: 0.845–0.962), respectively; the AUC values of the nomogram predicting 3-, 5-, and 7-year DFS were 0.868 (95% CI: 0.761–0.976), 0.838 (95% CI: 0.761–0.912), and 0.848 (95% CI: 0.775–0.921), respectively (Tables S1,S2). In the validation cohort, the AUC values of the nomogram predicting 3-, 5-, and 7-year OS were 0.879 (95% CI: 0.776–0.982), 0.895 (95% CI: 0.815–0.975), and 0.899 (95% CI: 0.822–0.977), respectively; the AUC values of the nomogram predicting 3-, 5-, and 7-year DFS were 0.817 (95% CI: 0.697–0.936), 0.805 (95% CI: 0.694–0.917), and 0.811 (95% CI: 0.699–0.922), respectively. Additionally, the calibration curves of the training and validation cohorts of the two predictive models showed good agreement between the predicted OS and DFS and the actual observations (Figures S2,S3), and the DCA demonstrated a satisfactory net benefit for the two predictive models in most of the threshold probabilities, suggesting that the models have a favorable potential clinical outcome (Figures S4,S5).
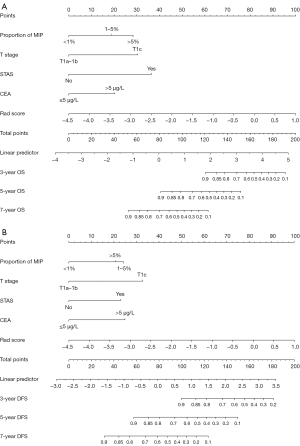
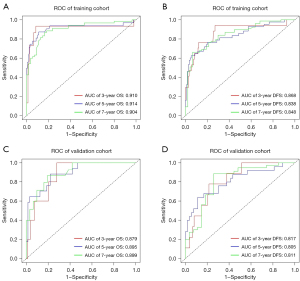
Development of a prediction stratification model
Based on the individual total scores calculated from the nomograms predicting OS and DFS, cut-off values were calculated in the total population using the X-tile procedure, which classified the total population into two risk groups: low risk (n=217, total point ≤111.9; n=173, total point ≤97.2) and high risk (n=37, total point >111.9; n=81, total point >97.2). Subsequent Kaplan-Meier survival analyses of the two risk groups in the total population based on the above cut-off values showed good differentiation, with significant differences in OS at 3, 5, and 7 years (3-year OS: 97.7% vs. 56.9%; 5-year OS: 90.6% vs. 15.1%; and 7-year OS: 75.3% vs. 3.4%; P<0.001) and the same significant differences in DFS at 3, 5, and 7 years (3-year DFS: 96.0% vs. 70.3%; 5-year DFS: 86.5% vs. 34.0%; and 7-year DFS: 75.8% vs. 0.0%; P<0.001) (Figure 4).
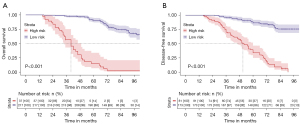
Discussion
Surgery is the most effective treatment for patients with early-stage lung cancer; however, the prognosis of patients varies considerably, and there is still a risk of tumor recurrence and metastasis postoperatively. The results of a meta-analysis in 1995 showed that patients with early-stage lung cancer who received postoperative platinum-based ACT had an approximately 5% increase in 5-year survival (25). This meta-analysis was followed by three randomized trials evaluating postoperative chemotherapy, including The Adjuvant Lung Project Italy study (26), The Big Lung Trial (27), and The Cancer and Leukemia Group B 9633 Trials (28), which demonstrated that patients with early-stage lung cancer after surgery did not benefit from ACT. In 2011, IASLC/ATS/ERS proposed a new classification system for lung ADC, which classified MIP involvement for the first time as a new histological subtype. It has been demonstrated that the MIP subtype is more prone to lymph nodes and distant metastases and has a higher risk of recurrence postoperatively (8,29-32). Although the current guidelines do not list the MIP component levels as a high-risk pathological factor for postoperative ACT (13) and do not recommend postoperative ACT for patients with the MIP subtype in early stages, an increasing number of studies have indicated that those with early-stage lung ADC with an MIP component benefit from postoperative ACT (9,33-35). In this study, we constructed and validated a clinical prediction model for predicting patients’ OS and DFS based on preoperative CT imaging and clinicopathological factors in individuals with stage IA lung ADC, achieving an effective prediction of those with early-stage lung ADC with MIP components who have a poor prognosis for clinical development of treatment strategies.
Key findings
In this study, using multifactorial analysis, we found that MIP component percentage, T-stage, STAS, CEA levels, and Rad score were independent high-risk pathological factors for poor prognosis in patients with early lung ADC containing an MIP component. In the training cohort, the AUC values of the nomogram for predicting 3-, 5-, and 7-year OS and DFS were 0.910, 0.914, and 0.904 and 0.868, 0.838, and 0.848, respectively; in the validation cohort, it was 0.879, 0.895, and 0.899 and 0.817, 0.805, and 0.811, respectively. The AUC values of the nomogram were significantly higher than those of each factor in both the training and validation cohorts, which demonstrated the high predictive value of the nomogram. Subsequently, we used the X-tile program to calculate the cut-off value of the nomogram individual total score in the total population and divided the people in the total population into two risk groups. When comparing the survival risk of the two risk groups, we found significant differences in both DFS and OS between patients in the low- and high-risk groups.
Comparison with similar researches
Several previous studies have explored CT radiomics as a predictor of treatment response and prognosis (16,36,37). For example, Perez-Johnston et al. retrospectively analyzed 219 preoperative CT images of patients with completely surgically resected stage I lung ADC, and CT-based imaging consensus clustering identified individual patients at high risk of recurrence or metastasis in those with lung ADC (36). Chang et al.’s study based on PET/CT parameter prediction found that patients with an MIP component had a higher risk of lymph node metastasis regardless of whether it was the major component or not (38). Therefore, it is feasible to predict recurrent metastasis in lung ADC patients with MIP components at stage IA by CT imaging histology, as in our study. We retrospectively examined 254 patients with stage IA pulmonary ADC containing an MIP component, analyzing their preoperative CT images and incorporating clinicopathological factors. In contrast, our study focused more on the effect of one pathological subtype, the MIP subtype, on recurrent metastasis in patients with stage IA lung ADC; therefore, we excluded patients with solid subtype, a high-risk pathology, to avoid bias in our results and included other clinicopathological factors, such as STAS. Our model will be beneficial in providing a predictive tool for clinicians for the purpose of individualized treatment, allowing them to identify which patients would most benefit from ACT.
In our analysis of postoperative pathological characteristics, we found that patient prognosis was significantly correlated with MIP percentage, T-stage, STAS, and CEA levels, which is consistent with previous findings. Lee et al. found that even the presence of only a small percentage of MIP components (<5%) significantly affected OS (20). Their survival analyses showed significantly better OS in patients without an MIP component (<1%) than in those with MIP percentages of 1–5% or >5%; however, no significant difference was found between patients with MIP percentages of 1–5% and >5%, which is consistent with our results. Regarding DFS, patients with MIP percentages of <1% and 1–5% had significantly better survival than those with MIP percentages >5%; however, no significant difference was observed between patients with MIP percentages of 1–5% and <1%, which contradicts our results. In our study, patients with percentages of MIP 1–5% and >5% had significantly worse OS and DFS than those with MIP percentages of <1%; however, no significant difference was found between patients with MIP percentages of 1–5% and >5%. The risk was higher for patients with MIP percentages of >5% and 1–5% in the prediction models predicting OS and DFS, respectively; however, the difference was not statistically significant. We believe that the lack of a significant difference between individuals with MIP percentages of 1–5% and >5% in our study may be because we included patients at the earliest stage of lung cancer, i.e., stage IA. Additionally, because of the high malignancy and rapid growth of the MIP component, even if the MIP accounts for only 1–5%, there is a high likelihood that the MIP will become a major component of the tumor during tumor progression in patients with lung ADC at stage IA, thereby affecting the prognosis. Furthermore, we excluded patients with lymph node metastasis, which is increased by the MIP component; the higher the percentage of MIP components, the greater the risk of lymph node metastasis in patients (39).
Previous research has shown that tumor size has an important prognostic value in early-stage lung cancer; studies by Hattori et al. and Hung et al. indicated that tumor size significantly affected the 5-year OS of patients (40,41). Additionally, Takenaka et al. concluded that data on tumor diameter are more readily available and can directly reflect the extent of lesion progression. This study analyzed the impact of maximum diameter, total volume, and consolidation tumor ratio (CTR) on patient prognosis in stage IA NSCLC (42). Data from this study showed that 5-year DFS was better in patients who had tumors with diameters ≤20 mm than in those with tumor diameters >20 mm. The study by Huang et al. predicted the risk of recurrence of stage IA lung ADC based on the percentage of MIP and solid components, and T-stage was a high-risk factor in their study (43). Consistent with our findings, patients with stage T1c cancer had a significantly worse prognosis than those with stage T1a–1b. The study by Wang et al. yielded the same results, where univariate and multivariate analyses of the T-stage revealed that it was a significant predictor of OS and PFS (9). With regard to the effectiveness of ACT in patients with stage T1c cancer, the results of Tsutani et al. demonstrated that the RPF and OS of T1c-stage patients who received ACT were significantly longer than those who did not receive ACT (44).
Through in-depth research in pathology, a new mode of metastasis, STAS, in addition to the common metastatic modes of lymphatic, hematogenous, and local implantation metastasis, has been identified. The World Health Organization defines STAS as airspace dissemination in the form of MIP clusters, solid nests, or single cancer cells in the peripheral lung parenchyma beyond the margins of the main tumor nests (45). In lung ADC, STAS is present in approximately 14.8–60.5% of cases and frequently in aggressive histologic types, such as the MIP or solid subtypes. The presence of high-level histologic subtypes is an independent predictor of STAS (6,18,46,47). Many studies have confirmed that the presence of STAS is one of the causes of shortened recurrence-free survival time and OS in patients postoperatively (41,47-49). Our study similarly demonstrated that STAS is a pathological factor affecting patient prognosis. Ding et al. determined, through a detailed examination of pathological serial sections, that clusters in the complex structure of the MIP appear to extend into the air (50). The lungs contain sufficient air, and the MIP component cells may have acquired anoikis resistance, enabling them to remain viable even after detachment. Thus, the cells constituting the MIP component, accompanied by air, can easily and extensively extend beyond the main tumor; through this mechanism, STAS occurs (51). In addition to biological factors, physical factors, including detachment of tumor cells caused by extrusion pressure during surgery and contamination of the scalpel blade with tumor cells, are also major factors affecting the presence of STAS (47,52). Therefore, further studies on the mechanism of STAS are needed to inform clinicians’ medical decisions. STAS is a common biological phenomenon that can seriously interfere with the effectiveness of surgical treatment for early-stage lung cancer and even affect the treatment strategy.
Serum CEA is crucial in the diagnosis and prognostic assessment of lung cancer. Patients who have lung cancers with positive CEA indexes are mostly clinically progressive or advanced; moreover, elevated CEA levels can be used as an indicator of lung cancer metastasis (53-56). In our study, the prognosis of patients with preoperative CEA >5 µg/L was significantly worse than that of those with CEA ≤5 µg/L, which is consistent with the findings of Huang et al. (43). Therefore, it is recommended that clinicians monitor the CEA levels of patients with early-stage lung ADC preoperatively to guide their diagnosis and treatment postoperatively.
In addition, the current study calculated risk scores for each patient by nomogram, and cut-off values for different risks in the nomogram were obtained using X-tile software. In the survival analysis between different risk subgroups, we found that the higher the risk, i.e., the higher the score, the higher the probability of postoperative progression of the patient and the worse the patient’s prognosis. Thus, for the patients who were judged to be at high risk by the prediction model used in this study, we recommend postoperative adjuvant therapy to improve the patient’s prognosis. For low-risk patients, although we do not recommend postoperative adjuvant therapy, we should conduct regular reviews and pay close attention to the changes in each risk factor.
Strengths and limitations
This study has some limitations. First, due to the nature of the retrospective analysis in this study, it is difficult to avoid selection bias; therefore, we recommend more prospective clinical studies on adjuvant therapy for stage IA lung ADC containing an MIP component. Second, this was a single-center study, and although internal validation indicates that the current prediction model has a superior AUC regarding OS and DFS, the results would be more convincing with external validation. Third, the sample size of this study was relatively small.
Implications and actions needed
Thus, future studies should include data from various research centers and larger sample sizes to enhance the reliability and generalizability of the findings. Nevertheless, our study provided important insight into the clinical management of high- and low-risk patients with stage IA lung ADC containing an MIP component.
Conclusions
This study found that the T1c stage, an MIP percentage ≥1%, STAS, a CEA level >5 µg/L, and Rad score were independent predictors of DFS and OS in patients with stage IA lung ADC containing an MIP component. We established and validated a nomogram based on clinicopathologic factors and radiomics features, which can be used as a powerful tool for predicting postoperative recurrence and survival in patients with stage IA lung ADC containing an MIP component.
Acknowledgments
We thank each patient for allowing the use of their clinicopathological data for this study.
Funding: This work was supported by
Footnote
Reporting Checklist: The authors have completed the TRIPOD reporting checklist. Available at https://tlcr.amegroups.com/article/view/10.21037/tlcr-24-544/rc
Data Sharing Statement: Available at https://tlcr.amegroups.com/article/view/10.21037/tlcr-24-544/dss
Peer Review File: Available at https://tlcr.amegroups.com/article/view/10.21037/tlcr-24-544/prf
Conflicts of Interest: All authors have completed the ICMJE uniform disclosure form (available at https://tlcr.amegroups.com/article/view/10.21037/tlcr-24-544/coif). The authors have no conflicts of interest to declare.
Ethical Statement: The authors are accountable for all aspects of the work in ensuring that questions related to the accuracy or integrity of any part of the work are appropriately investigated and resolved. The study was conducted in accordance with the Declaration of Helsinki (as revised in 2013). The study was approved by Institutional Ethics Committee of Cancer Hospital Affiliated to Shandong First Medical University (No. SDTHEC2023011030) and individual consent for this retrospective analysis was waived.
Open Access Statement: This is an Open Access article distributed in accordance with the Creative Commons Attribution-NonCommercial-NoDerivs 4.0 International License (CC BY-NC-ND 4.0), which permits the non-commercial replication and distribution of the article with the strict proviso that no changes or edits are made and the original work is properly cited (including links to both the formal publication through the relevant DOI and the license). See: https://creativecommons.org/licenses/by-nc-nd/4.0/.
References
- Kuhn E, Morbini P, Cancellieri A, et al. Adenocarcinoma classification: patterns and prognosis. Pathologica 2018;110:5-11.
- Ito H, Suzuki K, Mizutani T, et al. Long-term survival outcome after lobectomy in patients with clinical T1 N0 lung cancer. J Thorac Cardiovasc Surg 2020; Epub ahead of print. [Crossref]
- Nomori H, Mori T, Shiraishi A, et al. Long-Term Prognosis After Segmentectomy for cT1 N0 M0 Non-Small Cell Lung Cancer. Ann Thorac Surg 2019;107:1500-6. [Crossref] [PubMed]
- Lou F, Sima CS, Rusch VW, et al. Differences in patterns of recurrence in early-stage versus locally advanced non-small cell lung cancer. Ann Thorac Surg 2014;98:1755-60; discussion 1760-1. [Crossref] [PubMed]
- Uramoto H, Tanaka F. Recurrence after surgery in patients with NSCLC. Transl Lung Cancer Res 2014;3:242-9. [Crossref] [PubMed]
- Travis WD, Brambilla E, Noguchi M, et al. International association for the study of lung cancer/american thoracic society/european respiratory society international multidisciplinary classification of lung adenocarcinoma. J Thorac Oncol 2011;6:244-85. [Crossref] [PubMed]
- Amin MB, Tamboli P, Merchant SH, et al. Micropapillary component in lung adenocarcinoma: a distinctive histologic feature with possible prognostic significance. Am J Surg Pathol 2002;26:358-64. [Crossref] [PubMed]
- Tsubokawa N, Mimae T, Sasada S, et al. Negative prognostic influence of micropapillary pattern in stage IA lung adenocarcinoma. Eur J Cardiothorac Surg 2016;49:293-9. [Crossref] [PubMed]
- Wang C, Yang J, Lu M. Micropapillary Predominant Lung Adenocarcinoma in Stage IA Benefits from Adjuvant Chemotherapy. Ann Surg Oncol 2020;27:2051-60. [Crossref] [PubMed]
- Dai C, Xie H, Kadeer X, et al. Relationship of Lymph Node Micrometastasis and Micropapillary Component and Their Joint Influence on Prognosis of Patients With Stage I Lung Adenocarcinoma. Am J Surg Pathol 2017;41:1212-20. [Crossref] [PubMed]
- Martin LW, D'Cunha J, Wang X, et al. Detection of Occult Micrometastases in Patients With Clinical Stage I Non-Small-Cell Lung Cancer: A Prospective Analysis of Mature Results of CALGB 9761 (Alliance). J Clin Oncol 2016;34:1484-91. [Crossref] [PubMed]
- Rusch VW, Hawes D, Decker PA, et al. Occult metastases in lymph nodes predict survival in resectable non-small-cell lung cancer: report of the ACOSOG Z0040 trial. J Clin Oncol 2011;29:4313-9. [Crossref] [PubMed]
- Ettinger DS, Wood DE, Aisner DL, et al. NCCN Guidelines® Insights: Non-Small Cell Lung Cancer, Version 2.2023. J Natl Compr Canc Netw 2023;21:340-50. [Crossref] [PubMed]
- Braman N, Prasanna P, Whitney J, et al. Association of Peritumoral Radiomics With Tumor Biology and Pathologic Response to Preoperative Targeted Therapy for HER2 (ERBB2)-Positive Breast Cancer. JAMA Netw Open 2019;2:e192561. [Crossref] [PubMed]
- Coroller TP, Agrawal V, Huynh E, et al. Radiomic-Based Pathological Response Prediction from Primary Tumors and Lymph Nodes in NSCLC. J Thorac Oncol 2017;12:467-76. [Crossref] [PubMed]
- Wang X, Zhao X, Li Q, et al. Can peritumoral radiomics increase the efficiency of the prediction for lymph node metastasis in clinical stage T1 lung adenocarcinoma on CT? Eur Radiol 2019;29:6049-58. [Crossref] [PubMed]
- Kadota K, Nitadori JI, Sima CS, et al. Tumor Spread through Air Spaces is an Important Pattern of Invasion and Impacts the Frequency and Location of Recurrences after Limited Resection for Small Stage I Lung Adenocarcinomas. J Thorac Oncol 2015;10:806-14. [Crossref] [PubMed]
- Warth A, Muley T, Kossakowski CA, et al. Prognostic Impact of Intra-alveolar Tumor Spread in Pulmonary Adenocarcinoma. Am J Surg Pathol 2015;39:793-801. [Crossref] [PubMed]
- Lee JS, Kim EK, Kim M, et al. Genetic and clinicopathologic characteristics of lung adenocarcinoma with tumor spread through air spaces. Lung Cancer 2018;123:121-6. [Crossref] [PubMed]
- Lee G, Lee HY, Jeong JY, et al. Clinical impact of minimal micropapillary pattern in invasive lung adenocarcinoma: prognostic significance and survival outcomes. Am J Surg Pathol 2015;39:660-6. [Crossref] [PubMed]
- Chansky K, Detterbeck FC, Nicholson AG, et al. The IASLC Lung Cancer Staging Project: External Validation of the Revision of the TNM Stage Groupings in the Eighth Edition of the TNM Classification of Lung Cancer. J Thorac Oncol 2017;12:1109-21.
- Zhao W, Wu Y, Xu Y, et al. The Potential of Radiomics Nomogram in Non-invasively Prediction of Epidermal Growth Factor Receptor Mutation Status and Subtypes in Lung Adenocarcinoma. Front Oncol 2019;9:1485. [Crossref] [PubMed]
- Zhao W, Xu Y, Yang Z, et al. Development and validation of a radiomics nomogram for identifying invasiveness of pulmonary adenocarcinomas appearing as subcentimeter ground-glass opacity nodules. Eur J Radiol 2019;112:161-8. [Crossref] [PubMed]
- Liu Z, Zhang XY, Shi YJ, et al. Radiomics Analysis for Evaluation of Pathological Complete Response to Neoadjuvant Chemoradiotherapy in Locally Advanced Rectal Cancer. Clin Cancer Res 2017;23:7253-62. [Crossref] [PubMed]
- Chemotherapy in non-small cell lung cancer: a meta-analysis using updated data on individual patients from 52 randomised clinical trials. Non-small Cell Lung Cancer Collaborative Group. BMJ 1995;311:899-909.
- Scagliotti GV, Fossati R, Torri V, et al. Randomized study of adjuvant chemotherapy for completely resected stage I, II, or IIIA non-small-cell Lung cancer. J Natl Cancer Inst 2003;95:1453-61. [Crossref] [PubMed]
- Waller D, Peake MD, Stephens RJ, et al. Chemotherapy for patients with non-small cell lung cancer: the surgical setting of the Big Lung Trial. Eur J Cardiothorac Surg 2004;26:173-82. [Crossref] [PubMed]
- Strauss GM, Herndon JE 2nd, Maddaus MA, et al. Adjuvant paclitaxel plus carboplatin compared with observation in stage IB non-small-cell lung cancer: CALGB 9633 with the Cancer and Leukemia Group B, Radiation Therapy Oncology Group, and North Central Cancer Treatment Group Study Groups. J Clin Oncol 2008;26:5043-51. [Crossref] [PubMed]
- Jeon HW, Kim YD, Sim SB, et al. Significant difference in recurrence according to the proportion of high grade patterns in stage IA lung adenocarcinoma. Thorac Cancer 2021;12:1952-8. [Crossref] [PubMed]
- Choi SH, Jeong JY, Lee SY, et al. Clinical implication of minimal presence of solid or micropapillary subtype in early-stage lung adenocarcinoma. Thorac Cancer 2021;12:235-44. [Crossref] [PubMed]
- Wang W, Hu Z, Zhao J, et al. Both the presence of a micropapillary component and the micropapillary predominant subtype predict poor prognosis after lung adenocarcinoma resection: a meta-analysis. J Cardiothorac Surg 2020;15:154. [Crossref] [PubMed]
- Moreira AL, Ocampo PSS, Xia Y, et al. A Grading System for Invasive Pulmonary Adenocarcinoma: A Proposal From the International Association for the Study of Lung Cancer Pathology Committee. J Thorac Oncol 2020;15:1599-610. [Crossref] [PubMed]
- Hung JJ, Wu YC, Chou TY, et al. Adjuvant Chemotherapy Improves the Probability of Freedom From Recurrence in Patients With Resected Stage IB Lung Adenocarcinoma. Ann Thorac Surg 2016;101:1346-53. [Crossref] [PubMed]
- Qian F, Yang W, Wang R, et al. Prognostic significance and adjuvant chemotherapy survival benefits of a solid or micropapillary pattern in patients with resected stage IB lung adenocarcinoma. J Thorac Cardiovasc Surg 2018;155:1227-1235.e2. [Crossref] [PubMed]
- Luo J, Huang Q, Wang R, et al. Prognostic and predictive value of the novel classification of lung adenocarcinoma in patients with stage IB. J Cancer Res Clin Oncol 2016;142:2031-40. [Crossref] [PubMed]
- Perez-Johnston R, Araujo-Filho JA, Connolly JG, et al. CT-based Radiogenomic Analysis of Clinical Stage I Lung Adenocarcinoma with Histopathologic Features and Oncologic Outcomes. Radiology 2022;303:664-72. [Crossref] [PubMed]
- Nam JG, Park S, Park CM, et al. Histopathologic Basis for a Chest CT Deep Learning Survival Prediction Model in Patients with Lung Adenocarcinoma. Radiology 2022;305:441-51. [Crossref] [PubMed]
- Chang C, Sun X, Zhao W, et al. Minor components of micropapillary and solid subtypes in lung invasive adenocarcinoma (≤ 3 cm): PET/CT findings and correlations with lymph node metastasis. Radiol Med 2020;125:257-64. [Crossref] [PubMed]
- Cao Y, Zhu LZ, Jiang MJ, et al. Clinical impacts of a micropapillary pattern in lung adenocarcinoma: a review. Onco Targets Ther 2015;9:149-58. [Crossref] [PubMed]
- Hattori A, Matsunaga T, Takamochi K, et al. Neither Maximum Tumor Size nor Solid Component Size Is Prognostic in Part-Solid Lung Cancer: Impact of Tumor Size Should Be Applied Exclusively to Solid Lung Cancer. Ann Thorac Surg 2016;102:407-15. [Crossref] [PubMed]
- Hung JJ, Jeng WJ, Hsu WH, et al. Predictors of death, local recurrence, and distant metastasis in completely resected pathological stage-I non-small-cell lung cancer. J Thorac Oncol 2012;7:1115-23. [Crossref] [PubMed]
- Takenaka T, Yamazaki K, Miura N, et al. The Prognostic Impact of Tumor Volume in Patients with Clinical Stage IA Non-Small Cell Lung Cancer. J Thorac Oncol 2016;11:1074-80. [Crossref] [PubMed]
- Huang W, Zhang H, Zhang Z, et al. A prognostic nomogram based on a new classification of combined micropapillary and solid components for stage IA invasive lung adenocarcinoma. J Surg Oncol 2022;125:796-808. [Crossref] [PubMed]
- Tsutani Y, Imai K, Ito H, et al. Adjuvant Chemotherapy for High-risk Pathologic Stage I Non-Small Cell Lung Cancer. Ann Thorac Surg 2022;113:1608-16. [Crossref] [PubMed]
- Travis WD, Brambilla E, Nicholson AG, et al. The 2015 World Health Organization Classification of Lung Tumors: Impact of Genetic, Clinical and Radiologic Advances Since the 2004 Classification. J Thorac Oncol 2015;10:1243-60. [Crossref] [PubMed]
- Shiono S, Yanagawa N. Spread through air spaces is a predictive factor of recurrence and a prognostic factor in stage I lung adenocarcinoma. Interact Cardiovasc Thorac Surg 2016;23:567-72. [Crossref] [PubMed]
- Toyokawa G, Yamada Y, Tagawa T, et al. Significance of Spread Through Air Spaces in Resected Pathological Stage I Lung Adenocarcinoma. Ann Thorac Surg 2018;105:1655-63. [Crossref] [PubMed]
- Song T, Jiang L, Zhuo Z, et al. Impacts of thoracoscopic surgery and high grade histologic subtypes on spread through air spaces in small stage I lung adenocarcinomas. J Cancer Res Clin Oncol 2019;145:2375-82. [Crossref] [PubMed]
- Wang S, Hao J, Qian C, et al. Tumor Spread Through Air Spaces Is a Survival Predictor in Non-Small-Cell Lung Cancer. Clin Lung Cancer 2019;20:e584-91. [Crossref] [PubMed]
- Ding Q, Chen D, Wang X, et al. Characterization of lung adenocarcinoma with a cribriform component reveals its association with spread through air spaces and poor outcomes. Lung Cancer 2019;134:238-44. [Crossref] [PubMed]
- Kamiya K, Hayashi Y, Douguchi J, et al. Histopathological features and prognostic significance of the micropapillary pattern in lung adenocarcinoma. Mod Pathol 2008;21:992-1001. [Crossref] [PubMed]
- Watanabe K, Sakamaki K, Ito H, et al. Impact of the micropapillary component on the timing of recurrence in patients with resected lung adenocarcinoma. Eur J Cardiothorac Surg 2020;58:1010-8. [Crossref] [PubMed]
- Toyokawa G, Yamada Y, Tagawa T, et al. Computed tomography features of resected lung adenocarcinomas with spread through air spaces. J Thorac Cardiovasc Surg 2018;156:1670-1676.e4. [Crossref] [PubMed]
- Romero-Ventosa EY, Blanco-Prieto S, González-Piñeiro AL, et al. Pretreatment levels of the serum biomarkers CEA, CYFRA 21-1, SCC and the soluble EGFR and its ligands EGF, TGF-alpha, HB-EGF in the prediction of outcome in erlotinib treated non-small-cell lung cancer patients. Springerplus 2015;4:171. [Crossref] [PubMed]
- Cedrés S, Nuñez I, Longo M, et al. Serum tumor markers CEA, CYFRA21-1, and CA-125 are associated with worse prognosis in advanced non-small-cell lung cancer (NSCLC). Clin Lung Cancer 2011;12:172-9. [Crossref] [PubMed]
- Grunnet M, Sorensen JB. Carcinoembryonic antigen (CEA) as tumor marker in lung cancer. Lung Cancer 2012;76:138-43. [Crossref] [PubMed]