Exposure-lag response of surface net solar radiation on lung cancer incidence: a global time-series analysis
Highlight box
Key findings
• Low exposure to solar radiation is one of the risk factors of lung cancer incidence.
What is known and what is new?
• Exposure to solar radiation is a risk factor of skin cancer.
• Exposure to solar radiation is a protective factor of lung cancer and the protective effects of sunlight on lung cancer show hysteresis.
What is the implication, and what should change now?
• This study suggested that proper exposure to sunlight is a potential approach to the prevention of lung cancer and is significant in epidemiological studies because it provides a new pattern to investigate on more potential risk factors for diseases.
Introduction
Today, lung cancer is not only the most common causes of cancer death but a leading cause of death according to World Health Organization. In 2022, there were 2.48 million cases of lung cancer and resulted in 1.82 million deaths, which ranked first among causes of cancer incidence and death (1). Therefore, identification of modifiable risk factors for lung cancer would better inform lung cancer screening and public health prevention work. Recently, in addition to widely studied high-risk factors for lung cancer such as smoking (2,3), unhealthy diet, physical inactivity (4), genetic predisposition (5,6) and hormone effect (7), increasing published research focuses on various environmental risk factors, such as atmospheric particulate matter exposure (8,9), air temperature, relative humidity (10) and wildfire exposure (11). But there are still numerous risk factors remained to be found, for instance, solar radiation (RAD).
Recently, the influence of RAD on non-skin cancer has aroused increasing attention (12,13). A growing body of epidemiologic studies have explored the correlation between a variety of cancers and RAD, such as breast (14), colonic (15,16), prostatic (17), pancreatic (18), ovarian (19) and lung cancer (20,21). And the study of Yoshiharu Fukuda et al. (22) using ecological data in Japan shows that RAD is significantly inversely associated with most gastrointestinal cancers and male lung cancer and this study supports the preventive measures of RAD on several types of cancer. According to Webb et al. (23), Higashimoto et al. (24), Güzey et al. (25), RAD, particularly ultraviolet-B (UVB) radiation (280–320 nm), initiates the first stage of vitamin D synthesis by converting 7-dehydrocholesterol (7-DHC) in the skin to the precursor of vitamin D3. The active form of vitamin D, 1,25-(OH)2-D3, has been shown to significantly inhibit cell proliferation in various lung cancer cell lines, including NCI-H82 and NCI-H209 small cell lung carcinoma and EBC-1 and H520 non-small cell carcinoma cell lines. This suggests that adequate sun exposure, facilitating vitamin D production, may have a protective effect against lung cancer by inhibiting the proliferation of cancer cells. Similarly, Lin et al. (26) also found that RAD was significantly associated with decreased risks of squamous cell lung cancer but no significant association with lung adenocarcinoma, and Grant et al. (27) found that there was a strong inverse correlation between lung carcinoma mortality and RAD, while Lin et al. (28) found a weak positive association of RAD with deaths from lung cancer, but no consensus has been reached, and deep, global learning of RAD with lung cancer mortality is scarce.
One of the reasons why the above research reached different conclusions is that risk factors may have delayed influence on diseases (29), while exposure-lag-response associations reveal the duration of radiation-induced diseases onset (30). Common methods to capture the temporal dynamics and cumulative effects of environmental exposures on health outcomes, providing a deeper understanding of their long-term impacts include distributed lag model (DLM) and time-series models such as autoregressive integrated moving average model (ARIMA) (31,32). However, these methods are often limited by their assumptions of linear relationships or their focus on short-term effects, making them less suitable for capturing complex, non-linear, and delayed effects of environmental exposures, which is why distributed lag non-linear model (DLNM) excels. DLNM, first introduced by Armstrong (33), allows for the consideration of both delayed effects and non-linear relationships in time series data (34), making it particularly suitable for our analysis of the lagged effects of RAD on lung cancer incidence. In 2010, Armstrong and Gasparrini established the foundational family of DLNMs by introducing the definition of a ‘cross-basis’, which led to the creation of new models, including generalized linear models and additive models (35). From then on, DLNM is optimized consistently and obtains extensive acknowledgement in environmental epidemiological investigation which studies the relationship between risk factors and health outcomes (2,29,36).
With rapid development in geographic information sciences, statistical data science and computer science, we are conveniently accessible to continuous high-precision remote sensing data measured by satellite (37) and to public health database (38-40), which makes it possible for us to investigate on the correlation between RAD exposure and lung cancer. For instance, Google Earth Engine is an extensive platform that integrates scientific analysis and the visualization of geographic information data, providing historical images and scientific datasets for more than 40 years to meet users’ needs for large-scale data, ranging from Climate and Weather data, Imagery data to Geophysical data.
It is noteworthy that this study is pioneering in investigating the time-lag exposure-response relationship between RAD and lung cancer incidence on a global scale using DLNM. It provides valuable insights into the association between RAD exposure and lung cancer, setting a methodological precedent for investigating the risk factors of various diseases., though further studies are needed to confirm these findings and apply the methodology to investigate on other potential risk factors for various diseases. We present this article in accordance with the STROBE reporting checklist (available at https://tlcr.amegroups.com/article/view/10.21037/tlcr-24-125/rc).
Methods
Global Burden of Disease (GBD) data
We downloaded the latest data of age-standardized smoking prevalence, lung cancer incidence and socio-demographic index (SDI) of 1992–2019 directly from GBD website: http://ghdx.healthdata.org/gbd-2019. The GBD study, led by the Institute for Health Metrics and Evaluation (IHME), which is a comprehensive worldwide observational epidemiological study tracking health progress within and between 204 countries and territories and selected subnational locations. It offers a comprehensive annual estimation of the diseases, injuries, and risk factors burden, providing valuable insights for clinicians, researchers, and policymakers (41). We used age-standardized estimates of smoking tobacco use prevalence and tracheal, bronchus, and lung cancer (GBD case ID: 426) incidence estimates to describe smoking prevalence with an age group of 15+ years and represent for lung cancer incidence with an age group start at 10–14 years and end at 95+ years. The units of lung cancer incidence were new cases per 100,000 population. In GBD 2019, cancer incidence data were gathered from population-based cancer registries, including “Cancer Incidence in Five Continents (CI5)” (42-51), EUREG (52), and NORDCAN (53). Data were excluded if they lacked representativeness, failed to encompass all malignant neoplasms, did not include data for both sexes and all age groups, or were confined to years prior to 1980. Preference was given to registries with national coverage. Methodological details of GBD 2019 have been presented in previous publications (54-56). As for SDI, it is a composite measure of development status and has a strong correlation with health outcomes developed by GBD researchers and widely used to help make estimates. Additionally, it is the geometric mean calculated from indices ranging from 0 to 1, which include the total fertility rate for individuals under 25 years, the average education level for those aged 15 years and older, and the lag-distributed income per capita. To sum up with, a district with an SDI of 0 represents the theoretical minimum level of development pertinent to health, whereas a district with an SDI of 1 indicates the theoretical maximum level of development (39). Age-standardized smoking prevalence estimates and SDI were used for models adjustment because they are considered the main risk factor for lung cancer incidence. All of the three datasets include all genders. Detailed information about these datasets can be found at http://ghdx.healthdata.org/gbd-2019. The study was conducted in accordance with the Declaration of Helsinki (as revised in 2013).
RAD data
We downloaded raw raster data with a resolution of 11,132 m from Google Earth Engine: (https://developers.google.com/earth-engine/datasets/catalog/ECMWF_ERA5_LAND_MONTHLY) (37). The data we used were a portion of ERA5-Land dataset, which has been post-processed by European Centre for Medium-Range Weather Forecasts (ECMWF). ERA5-Land is a reanalysis dataset that integrates model data with global observations, creating a comprehensive and consistent dataset that adheres to physical laws. In this dataset, we selected the surface net RAD band as our primary radiation data. This measurement represents the amount of RAD, including both direct and diffuse components, that reaches the Earth’s surface, minus the portion reflected by the surface, which is determined by the albedo. The units of this data are expressed in joules per square meter (J/m2). It is noteworthy that this reanalysis data were reprocessed from the raw remote sensing data which is accurate to hours into a data with an accuracy of months and we calculated the average annual value of it. Then, we added this raw raster data as a layer on a country boundaries layer formed by a vector data. Finally, we used the country boundaries to control the raster data and calculated mean radiation value within each country boundaries as our RAD data distributed by countries and years (57,58).
We calculated radiation exposure in 272 countries and territories in total according to the raster data. After eliminated the one which lacked data or the value was equaled to 9,999 (abnormal value) which means the land area of those districts was smaller than the resolution of our raster data, as well Kashmir and Western Sahara which were two disputed districts, 220 countries and territories remained. Countries and territories with missing data on lung cancer prevalence or smoking prevalence for any year were excluded from the study. Finally, there were 186 countries enrolled in the analysis.
Population data
We downloaded the population data updated in March 19th, 2021 from World Development Indicators (WDI): https://datacatalog.worldbank.org/search/dataset/0037712/World-Development-Indicators (59), which is a catalog of the World Bank. The WDI which compiled from officially-recognized international sources are the primary World Bank collection of development indicators and it not only makes the latest and accurate global development data available but also includes national, regional and global estimates. And we next conducted a filter of total population. Total population counts all residents regardless of their legal status or citizenship. The values shown are midyear estimates. However, we lost the population data of Kuwait in 1992, 1993 and 1994.
Statistical analysis
We conducted Spearman’s correlation tests to establish the correlation between RAD and lung cancer and calculated the correlation coefficients. Then, we conducted quasi-Poisson regression analysis with a DLNM which estimated the long-term association between radiation and lung cancer. DLNMs are widely accepted and frequently employed to investigate on the relationship between risk factors and health outcomes. In this study, we utilized the cross-basis function and natural spline (ns) function with 3 degrees of freedom (df) and a maximum lag of 15 years, following the Akaike information criterion (AIC) to construct the basis matrix for the two dimensions of radiation exposure intensity and lag years (60).
Eventually, the model with unadjusted lung cancer incidence can be expressed by the following formula:
The model with adjusted lung cancer incidence can be expressed by the following formula:
In these two formulas, Y represents the actual value of lung cancer prevalence without adjustments for smoking prevalence and SDI, while µ denotes the expected value of lung cancer prevalence. The variable t indicates the observation years. The actual value of lung cancer prevalence adjusted for smoking prevalence and SDI is represented by Z. The term β0 refers to the overall intercept, β1 is the regression coefficient of radiation, and δ stands for the residuals after regressing lung cancer prevalence on smoking prevalence and SD.
We consider P values of less than 0.05 to be statistically significant. All statistical analyses were performed using R (R Core Team, Vienna, Austria), version 4.1.1 and the DLNM models were generated using R package “dlnm” version ‘2.4.7’ and “splines” version ‘4.1.1’.
Results
Figure 1 is the global distribution map of surface net RAD in 2019 and the other global distribution maps of radiation by year (1992–2018) are shown in Figures S1-S27. The countries excluded in our analysis shaded grey. The figures were produced by ArcGIS 10.7.
Table 1 shows the statistical descriptions of RAD, SDI, lung cancer incidence (unadjusted for smoking and SDI and adjusted for smoking and SDI) and smoking prevalence. For all countries dataset, the smoking prevalence was 0.315±0.127, where the minimum prevalence was 0.056 (Guinea-Bissau, 1999, 2000, 2001, 2002), and the maximum prevalence was 0.649 (Timor-Leste, 2014, 2015). The SDI was 0.567±0.197, where the minimum value was 0.052 (Somalia, 1992) and the maximum value was 0.929 (Switzerland, 2019). The lung cancer incidence was 21.918±14.360, where the minimum was 4.735 (Jordan, 2014; Kenya, 1992) and the maximum was 104.156 (Greenland, 1999). The lung cancer incidence which was adjusted for smoking and SDI was 21.945±14.213, where the minimum was 5.095 (Kenya, 2015) and the maximum was 98.455 (Greenland, 2000). The amount of surface net RAD was 12,735,923.05±3,494,273.32, where the minimum was 1,291,606.49 (The Federated States of Micronesia, 2002) and the maximum was 18,440,775.92 (Namibia, 2007). While for the global dataset (we created it by calculating the average value for each country in certain years), the smoking prevalence was 0.315±0.019, where the minimum prevalence was 0.290 (2019), and the maximum prevalence was 0.347 (1992). The SDI was 0.567±0.046, where the minimum value was 0.494 (1992) and the maximum value was 0.640 (2019). The lung cancer incidence was 21.92±0.38, where the minimum was 21.17 (2019) and the maximum was 22.47 (1995). The lung cancer incidence which was adjusted for smoking and SDI was 21.94±0.17, where the minimum was 21.67 (2017) and the maximum was 22.27 (2009). The RAD was 12,735,923.05±67,507.83, where the minimum was 12,571,845.96 (1996) and the maximum was 12,846,318.62 (2019).
Table 1
Item | Min | 1st Qu | Median | Mean | 3rd Qu | Max | SD |
---|---|---|---|---|---|---|---|
Countries | |||||||
Smoking prevalence | 0.056 | 0.216 | 0.306 | 0.315 | 0.418 | 0.649 | 0.127 |
SDI | 0.052 | 0.414 | 0.592 | 0.567 | 0.728 | 0.929 | 0.197 |
Incidence | 4.735 | 10.292 | 17.566 | 21.918 | 31.628 | 104.156 | 14.360 |
Incidence adjusted for smoking and SDI | 5.095 | 10.289 | 17.389 | 21.945 | 32.129 | 98.455 | 14.213 |
RAD (take two decimal places) (J/m2) | 1,291,606.49 | 10,699,905.07 | 13,431,526.54 | 12,735,923.05 | 15,369,323.27 | 18,440,775.92 | 3,494,273.32 |
Global | |||||||
Smoking prevalence | 0.290 | 0.298 | 0.315 | 0.315 | 0.331 | 0.347 | 0.019 |
SDI | 0.494 | 0.529 | 0.567 | 0.567 | 0.606 | 0.640 | 0.046 |
Incidence | 21.17 | 21.76 | 21.97 | 21.92 | 22.15 | 22.47 | 0.38 |
Incidence adjusted for smoking and SDI | 21.67 | 21.83 | 21.93 | 21.94 | 22.10 | 22.27 | 0.17 |
RAD (take two decimal places) (J/m2) | 12,571,845.96 | 12,720,931.75 | 12,742,101.46 | 12,735,923.05 | 12,778,329.76 | 12,846,318.62 | 67,507.83 |
RAD, solar radiation; SDI, socio-demographic index; SD, standard deviation.
Table 2 shows the Spearman’s correlation coefficients and P values between RAD and lung cancer unadjusted or adjusted for smoking and SDI distributed by years. Since that our data show non-normal distribution, we use Spearman’s method to estimate their relevance. As we can see, all the P values are less than 0.05, which means the correlation between these two variables is statistically significant. Moreover, all the values of r are negative so we can say the correlation between them is negative. Compared the value of r, the value of 1996 shows the strongest relevance overall, which is equal to −0.645 with unadjusted lung cancer incidence and −0.630 with adjusted lung cancer incidence.
Table 2
Year | Unadjusted for smoking and SDI | Adjusted for smoking and SDI | |||
---|---|---|---|---|---|
r | P value | r | P value | ||
1992 | −0.646 | <0.001 | −0.613 | <0.001 | |
1993 | −0.641 | <0.001 | −0.615 | <0.001 | |
1994 | −0.637 | <0.001 | −0.615 | <0.001 | |
1995 | −0.639 | <0.001 | −0.622 | <0.001 | |
1996 | −0.645 | <0.001 | −0.630 | <0.001 | |
1997 | −0.615 | <0.001 | −0.601 | <0.001 | |
1998 | −0.608 | <0.001 | −0.595 | <0.001 | |
1999 | −0.626 | <0.001 | −0.620 | <0.001 | |
2000 | −0.615 | <0.001 | −0.610 | <0.001 | |
2001 | −0.623 | <0.001 | −0.621 | <0.001 | |
2002 | −0.605 | <0.001 | −0.606 | <0.001 | |
2003 | −0.581 | <0.001 | −0.586 | <0.001 | |
2004 | −0.595 | <0.001 | −0.595 | <0.001 | |
2005 | −0.601 | <0.001 | −0.604 | <0.001 | |
2006 | −0.606 | <0.001 | −0.611 | <0.001 | |
2007 | −0.606 | <0.001 | −0.613 | <0.001 | |
2008 | −0.607 | <0.001 | −0.619 | <0.001 | |
2009 | −0.598 | <0.001 | −0.612 | <0.001 | |
2010 | −0.597 | <0.001 | −0.610 | <0.001 | |
2011 | −0.581 | <0.001 | −0.602 | <0.001 | |
2012 | −0.583 | <0.001 | −0.606 | <0.001 | |
2013 | −0.583 | <0.001 | −0.609 | <0.001 | |
2014 | −0.586 | <0.001 | −0.612 | <0.001 | |
2015 | −0.564 | <0.001 | −0.593 | <0.001 | |
2016 | −0.578 | <0.001 | −0.611 | <0.001 | |
2017 | −0.572 | <0.001 | −0.604 | <0.001 | |
2018 | −0.580 | <0.001 | −0.613 | <0.001 | |
2019 | −0.549 | <0.001 | −0.581 | <0.001 |
RAD, solar radiation; SDI, socio-demographic index.
Figure 2A is the bubble plot illustrating the correlation between RAD exposure and lung cancer incidence without adjustments for smoking prevalence and SDI. In contrast, Figure 2B displays the correlation between RAD exposure and lung cancer incidence, adjusted for smoking prevalence and SDI, for the year 1996, which shows the strongest correlation between RAD and lung cancer. We can see a robust negative correlation between radiation and lung cancer prevalence. The Spearman’s correlation coefficient between RAD and lung cancer unadjusted for smoking and SDI was −0.645, while the Spearman’s correlation coefficient between RAD and lung cancer adjusted for smoking was −0.630.
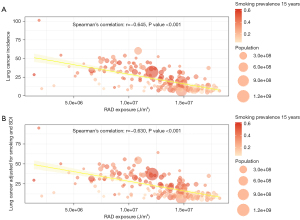
In Figure 3, the three-dimensional exposure-lag response surface illustrates the combined influence of lag time (in years) and RAD (J/m2) on predicted incidence relative risk (RR) of lung cancer. Figure 3A is the plot of lung cancer unadjusted for smoking and SDI while Figure 3B is the plot of lung cancer incidence which is adjusted for smoking and SDI. Across the three-dimensional surface of the model of lung cancer incidence unadjusted for smoking and SDI, the maximum RR is 1.025 [95% confidence interval (CI): 1.023–1.028] at RAD exposure =12,770,000 and lag =5.4 years, while the minimum RR was 0.968 (95% CI: 0.95–0.989) at RAD exposure =12,845,000 and lag =8.4 years. Across the three-dimensional surface of the model of lung cancer adjusted for smoking and SDI, the maximum RR was 1.013 (95% CI: 1.011–1.014) at RAD exposure =12,760,000 and lag =5.8 years, while the minimum RR was 0.973 (95% CI: 0.947–0.992) at RAD exposure =12,845,000 and lag =8.0 years. There was a strong interaction effect between increases in RAD exposure and lag on RR, indicating that the response to changes in RAD exposure depended on the lag, and conversely, the effect of lag was influenced by RAD exposure.

Figure 4A is the cumulative exposure-response curve of lung cancer prevalence unadjusted for smoking and SDI in different RAD exposure and Figure 4B is the curve of lung cancer prevalence adjusted for smoking and SDI. The lag response curves were wave-like. In Figure 4A, we can find that with the increment of radiation, RR decreases first and then increase, at last decrease when lag =1 to 14, with a valley value of RR in about exp =12,670,000 and a peak value of RR in about exp =12,765,000 in the unadjusted model. While in the adjusted model, we find that RR increase first and then decrease to the end, with a peak value of RR in about exp =12,760,000 when lag =1 to 14. As for lag =15, the curve decreases all the time no matter Figure 4A or Figure 4B.
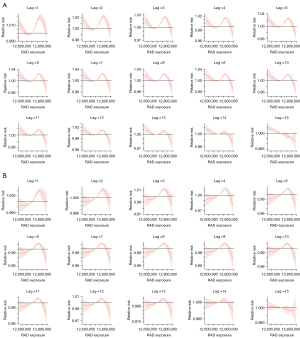
Figure 5 shows the relation between lag and RR of lung cancer incidence (unadjusted for smoking and SDI). As we can see, when exp <12,700,000, there is an approximately U-shaped correlation between them and when exp >12,700,000, there is an approximately inverted U-shaped correlation between them. With the increasing of exposure, we can find that the confident interval is shrinking which means the accuracy of the estimated lag-response is increasing. The other turning points of these two variables are in Figure S28 and Figure S29. Moreover, we also conducted a sectional analysis of lag-response between radiation exposure and RR of lung cancer incidence adjusted for smoking and SDI and found similar trends in this model (see Figure S30 and Figure S31).
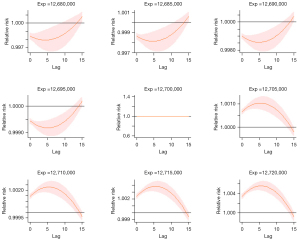
Discussion
To the best of our knowledge, this study is the first research to investigate on the exposure-lag response of RAD exposure on lung cancer using a DLNM and remote sensing data measured by satellite on a global scale, which illustrated the non-linear and delayed associations between them.
The results show complicated nonlinear relationship between RAD and lung cancer incidence. In Figure 4, some might have expected that with the increment of RAD exposure, RR decrease all the way like the lag =15 plot because of the preventive effect of RAD on lung cancer (22). However, from lag =1 to lag =14, the curves decrease first to a valley value of RR, and then increase to a peak value, but decreases at last. In Figure 5, exp =12,700,000 is an important dividing point, we can find an obvious different tendency on the both sides of it. This may suggest a possible hysteresis effect, in which the protective effects of sunlight on lung cancer may manifest differently over varying time lags. However, due to the lack of relevant research on this specific delayed protective effect of sunlight on lung cancer, further studies are needed to understand these dynamics comprehensively. The variations observed might be due to differences in cumulative exposure, biological adaptation, or other intervening environmental or genetic factors that modulate the long-term risk. Further research should focus on these aspects to elucidate the underlying mechanisms driving these complex associations.
It is generally acknowledged that RAD is vital to our life. RAD not only provides us with warmth, energy, food, etc. but also makes it possible for us to see the world with light. However, lots of evidences have shown that high level of RAD may cause some serious diseases, such as skin cancer while low level RAD may cause vitamin D deficiency (61). Vitamin D can be produced by the body with sufficient exposure to sunlight. The work of Webb et al. explained the mechanism of sunlight on vitamin D absorption clearly. It documented that the first stage of vitamin D synthesis is the conversion of the 7-dehydrocholesterol (7-DHC) to the precursor of vitamin D3 within the skin, which requires radiation of wavelengths in the UVB (280–320 nm) portion of the electromagnetic spectrum (23). Furthermore, vitamin D and several analogs of vitamin D have been proved to modulate proliferation and differentiation of cancer cells (21,62,63), which is consistent with our research results that lung cancer incidence is overall negatively associated with RAD. Vitamin D3 is being transformed to 25-(OH)-D3 in liver and then converted to 1,25-(OH)2-D3 in kidney. And 1,25-(OH)2-D3 has been proved to be significant in the inhibition of cell proliferation in the NCI-H82 and NCI-H209 small cell lung carcinoma and the EBC-1 and H520 non-small cell carcinoma cell lines (24,25).
There are several advantages in our study. Firstly, we innovatively explored the global relationship between RAD and lung cancer incidence using precise satellite measurements of RAD and the most recent data from the 2019 GBD study. To our knowledge, this is the first research using continuous high-precision remote sensing data alongside GBD cancer data on a global scale to investigate potential cancer risk factors, which can provide a new paradigm to analyze and identify more unknown or uncertain high-risk factors of diseases recommended by the experts. Compared with the current standard (such as traditional cohort study or questionnaire-based survey) to explore risk factors, our study not only eliminates recall biases, but also enables rapid and extensive screening of potential risk factors at a lower cost and in less time, significantly alleviating the financial and budgetary pressures faced by countries or organizations. This aids policymakers in making quick decisions.
There are also limitations in our study. Firstly, as for the radiation data, we calculated the mean value of the whole country, which means that for a large country with a wide latitude span, the value may just reflect the RAD exposure condition for the center of the country, hence studies with smaller scale are required. Secondly, another potential limitation of our study is the ecological fallacy, which arises from using aggregate data to make inferences about individual-level relationships. While we used country-level data on RAD and lung cancer incidence, this approach does not account for individual variations in exposure and susceptibility. Consequently, our findings may not directly reflect individual risk, further studies with individual-level data are needed to validate these associations. Thirdly, as we all know, approximately, 50% RAD is in the visible spectral region (400–760 nm), 7% is in ultraviolet spectral region (<400 nm), 43% is in infrared spectral region (>760 nm). In addition, the ultraviolet spectral region part could be divided into 3 more parts, long-wave UVA (320–400 nm), medium-wave UVB (280–320 nm) and short-wave UVC (200–280 nm) (64,65). In order to have deeper knowledge into which light with certain range of wavelength has the exact impact on lung cancer, separate studies for each kind of light are required.
Conclusions
This study suggested that the exposure-response varied by lag period, whilst the lag-response varied by RAD exposure. Moreover, low exposure to RAD is one of the risk factors of lung cancer incidence globally while the actual effect of this has a certain lag period. This study is significant because it not only reveals the correlation between RAD and lung cancer incidence, but also provides a possible strategy for preventing lung cancer. Additionally, this study introduces a new pattern for investigating more potential disease risk factors.
Acknowledgments
The authors primarily express their gratitude to National Natural Science Foundation (NNSF) and Guangzhou government for its strong support to our research. Then, the authors show great appreciation to the ECMWF for providing necessary open data on Google Earth Engine for this study and the Institute for Health Metrics and Evaluation (IHME) for coordinating The Global Burden of Disease Study 2019 (GBD 2019) which offered a reliable and comprehensive annual estimation of the burden of diseases, injuries, and risk factors globally. Additionally, the authors also feel grateful to the staff of the World Bank because they strive constantly to provide a global cognition for the world. Parts of this manuscript were presented as a poster at the European Lung Cancer Congress 2023 (ELCC 2023) conference.
Funding: This work was supported by
Footnote
Reporting Checklist: The authors have completed the STROBE reporting checklist. Available at https://tlcr.amegroups.com/article/view/10.21037/tlcr-24-125/rc
Peer Review File: Available at https://tlcr.amegroups.com/article/view/10.21037/tlcr-24-125/prf
Conflicts of Interest: All authors have completed the ICMJE uniform disclosure form (available at https://tlcr.amegroups.com/article/view/10.21037/tlcr-24-125/coif). W.L. serves as an Associate Editors-in-Chief of Translational Lung Cancer Research from May 2024 to April 2025. X.L. reports Special Funds for the Cultivation of Guangdong College Students’ Scientific and Technological Innovation (“Climbing Program” Special Funds) (Grant No. pdjh2024b312). W.L. reports support from Major Project of Guangzhou National Laboratory (Grant No. SRPG22-017); the National Natural Science Foundation of China (Grant No. 82373121); Science and Technology Plan Project of Guangzhou (Grant No. 202206080013); and National Key Research & Development Programme (Grant No. 2022YFC2505105). The other authors have no conflicts of interest to declare.
Ethical Statement: The authors are accountable for all aspects of the work in ensuring that questions related to the accuracy or integrity of any part of the work are appropriately investigated and resolved. The study was conducted in accordance with the Declaration of Helsinki (as revised in 2013).
Open Access Statement: This is an Open Access article distributed in accordance with the Creative Commons Attribution-NonCommercial-NoDerivs 4.0 International License (CC BY-NC-ND 4.0), which permits the non-commercial replication and distribution of the article with the strict proviso that no changes or edits are made and the original work is properly cited (including links to both the formal publication through the relevant DOI and the license). See: https://creativecommons.org/licenses/by-nc-nd/4.0/.
References
- Ferlay J, Ervik M, Lam F, et al. Global Cancer Observatory: Cancer Today. International Agency for Research on Cancer, Lyon, France. Available online: https://gco.iarc.fr/today. Accessed March 2nd 2024.
- Smith DR, Behzadnia A, Imawana RA, et al. Exposure-lag response of smoking prevalence on lung cancer incidence using a distributed lag non-linear model. Sci Rep 2021;11:14478. [Crossref] [PubMed]
- Deros DE, Hagerman CJ, Kramer JA, et al. Change in amount smoked and readiness to quit among patients undergoing lung cancer screening. J Thorac Dis 2021;13:4947-55. [Crossref] [PubMed]
- Khaltaev N, Axelrod S. Global lung cancer mortality trends and lifestyle modifications: preliminary analysis. Chin Med J (Engl) 2020;133:1526-32. [Crossref] [PubMed]
- Liang C, Pan W, Zhou Z, et al. Identification of prognostic biomarkers of smoking-related lung cancer. J Thorac Dis 2024;16:1438-49. [Crossref] [PubMed]
- Hanash S. Lung cancer susceptibility beyond smoking history: opportunities and challenges. Transl Lung Cancer Res 2022;11:1230-2. [Crossref] [PubMed]
- Yang Z, Wang F, Tan F, et al. Menstrual factors, reproductive history, and risk of lung cancer: a multi-center population-based cohort study in Chinese females. Transl Lung Cancer Res 2021;10:3912-28. [Crossref] [PubMed]
- Hamra GB, Guha N, Cohen A, et al. Outdoor particulate matter exposure and lung cancer: a systematic review and meta-analysis. Environ Health Perspect 2014;122:906-11. [Crossref] [PubMed]
- Chen Q, Wang Y, Yang L, et al. PM2.5 promotes NSCLC carcinogenesis through translationally and transcriptionally activating DLAT-mediated glycolysis reprograming. J Exp Clin Cancer Res 2022;41:229. [Crossref] [PubMed]
- Guo H, Li X, Li W, et al. Climatic modification effects on the association between PM1 and lung cancer incidence in China. BMC Public Health 2021;21:880. [Crossref] [PubMed]
- Zhang D, Xi Y, Boffa DJ, et al. Association of Wildfire Exposure While Recovering From Lung Cancer Surgery With Overall Survival. JAMA Oncol 2023;9:1214-20. [Crossref] [PubMed]
- Kricker A, Armstrong B. Does sunlight have a beneficial influence on certain cancers? Prog Biophys Mol Biol 2006;92:132-9. [Crossref] [PubMed]
- Camara AB, Brandao IA. The Role of Vitamin D and Sunlight Incidence in Cancer. Anticancer Agents Med Chem 2019;19:1418-36. [Crossref] [PubMed]
- Gorham ED, Garland FC, Garland CF. Sunlight and breast cancer incidence in the USSR. Int J Epidemiol 1990;19:820-4. [Crossref] [PubMed]
- Garland CF, Garland FC. Do sunlight and vitamin D reduce the likelihood of colon cancer? Int J Epidemiol 2006;35:217-20. [Crossref] [PubMed]
- Vallès X, Alonso MH, López-Caleya JF, et al. Colorectal cancer, sun exposure and dietary vitamin D and calcium intake in the MCC-Spain study. Environ Int 2018;121:428-34. [Crossref] [PubMed]
- Colli JL, Grant WB. Solar ultraviolet B radiation compared with prostate cancer incidence and mortality rates in United States. Urology 2008;71:531-5. [Crossref] [PubMed]
- Boscoe FP, Schymura MJ. Solar ultraviolet-B exposure and cancer incidence and mortality in the United States, 1993-2002. BMC Cancer 2006;6:264. [Crossref] [PubMed]
- Lefkowitz ES, Garland CF. Sunlight, vitamin D, and ovarian cancer mortality rates in US women. Int J Epidemiol 1994;23:1133-6. [Crossref] [PubMed]
- Mohr SB, Garland CF, Gorham ED, et al. Could ultraviolet B irradiance and vitamin D be associated with lower incidence rates of lung cancer? J Epidemiol Community Health 2008;62:69-74. [Crossref] [PubMed]
- Porojnicu AC, Robsahm TE, Dahlback A, et al. Seasonal and geographical variations in lung cancer prognosis in Norway. Does Vitamin D from the sun play a role? Lung Cancer 2007;55:263-70. [Crossref] [PubMed]
- Fukuda Y, Nakaya T, Nakao H, et al. Multilevel analysis of solar radiation and cancer mortality using ecological data in Japan. Biosci Trends 2008;2:235-40.
- Webb AR, Holick MF. The role of sunlight in the cutaneous production of vitamin D3. Annu Rev Nutr 1988;8:375-99. [Crossref] [PubMed]
- Higashimoto Y, Ohata M, Nishio K, et al. 1 alpha, 25-dihydroxyvitamin D3 and all-trans-retinoic acid inhibit the growth of a lung cancer cell line. Anticancer Res 1996;16:2653-9.
- Güzey M, Sattler C, DeLuca HF. Combinational effects of vitamin D3 and retinoic acid (all trans and 9 cis) on proliferation, differentiation, and programmed cell death in two small cell lung carcinoma cell lines. Biochem Biophys Res Commun 1998;249:735-44. [Crossref] [PubMed]
- Lin SW, Wheeler DC, Park Y, et al. Prospective study of ultraviolet radiation exposure and risk of cancer in the United States. Int J Cancer 2012;131:E1015-23. [Crossref] [PubMed]
- Grant WB. An estimate of premature cancer mortality in the U.S. due to inadequate doses of solar ultraviolet-B radiation. Cancer 2002;94:1867-75. [Crossref] [PubMed]
- Lin SW, Wheeler DC, Park Y, et al. Prospective study of ultraviolet radiation exposure and mortality risk in the United States. Am J Epidemiol 2013;178:521-33. [Crossref] [PubMed]
- Wang Z, Peng J, Liu P, et al. Association between short-term exposure to air pollution and ischemic stroke onset: a time-stratified case-crossover analysis using a distributed lag nonlinear model in Shenzhen, China. Environ Health 2020;19:1. [Crossref] [PubMed]
- Aßenmacher M, Kaiser JC, Zaballa I, et al. Exposure-lag-response associations between lung cancer mortality and radon exposure in German uranium miners. Radiat Environ Biophys 2019;58:321-36. [Crossref] [PubMed]
- Ran J, Qiu H, Sun S, et al. Short-term effects of ambient benzene and TEX (toluene, ethylbenzene, and xylene combined) on cardiorespiratory mortality in Hong Kong. Environ Int 2018;117:91-8. [Crossref] [PubMed]
- Zhang J, Nawata K. A comparative study on predicting influenza outbreaks. Biosci Trends 2017;11:533-41. [Crossref] [PubMed]
- Armstrong B. Models for the relationship between ambient temperature and daily mortality. Epidemiology 2006;17:624-31. [Crossref] [PubMed]
- Gasparrini A. Distributed Lag Linear and Non-Linear Models in R: The Package dlnm. J Stat Softw 2011;43:1-20.
- Gasparrini A, Armstrong B, Kenward MG. Distributed lag non-linear models. Stat Med 2010;29:2224-34. [Crossref] [PubMed]
- Gu S, Huang R, Yang J, et al. Exposure-lag-response association between sunlight and schizophrenia in Ningbo, China. Environ Pollut 2019;247:285-92. [Crossref] [PubMed]
- J MS. ERA5-Land monthly averaged data from 1981 to present. Copernicus Climate Change Service (C3S) Climate Data Store (CDS). 2019. Available online: https://cds.climate.copernicus.eu/cdsapp#!/dataset/10.24381/cds.68d2bb30?tab=overview. Accessed November 15th 2021.
- Global Burden of Disease Study 2019 (GBD 2019) Smoking Tobacco Use Prevalence 1990-2019. Institute for Health Metrics and Evaluation (IHME), Seattle, United States of America. 2021. Available online: https://ghdx.healthdata.org/record/ihme-data/gbd-2019-smoking-tobacco-use-prevalence-1990-2019. Accessed November 16th 2021.
- Global Burden of Disease Study 2019 (GBD 2019) Socio-Demographic Index (SDI) 1950–2019. Institute for Health Metrics and Evaluation (IHME), Seattle, United States of America. 2020. Available online: https://ghdx.healthdata.org/record/ihme-data/gbd-2019-socio-demographic-index-sdi-1950-2019. Accessed November 15th 2021.
- GBD Results. IHME, University of Washington, Seattle, WA. 2020. Available online: http://ghdx.healthdata.org/gbd-2019. Accessed November 15th 2021.
- The Lancet. Available online: https://www.thelancet.com/gbd. Accessed July 18th 2024.
- Doll R, Payne P, Waterhouse J. Cancer Incidence in Five Continents I. Geneva: Union Internationale Contre le Cancer; 1966.
- Doll R, Muir C, Waterhouse J. Cancer Incidence in Five Continents II. Geneva: Union Internationale Contre le Cancer; 1970.
- Waterhouse J, Muir C, Correa P, et al. Cancer Incidence in Five Continents III. Lyon: IARC; 1976.
- Waterhouse J, Muir C, Shanmugaratnam K, et al. Cancer Incidence in Five Continents IV. Lyon: IARC; 1982.
- Muir C, Mack T, Powell J, et al. Cancer Incidence in Five Continents V. Lyon: IARC; 1987.
- Parkin D, Muir C, Whelan S, et al. Cancer Incidence in Five Continents VI. Lyon: IARC; 1992.
- Parkin D, Whelan S, Ferlay J, et al. Cancer Incidence in Five Continents VII. Lyon: IARC; 1997.
- Parkin D, Whelan S, Ferlay J, et al. Cancer Incidence in Five Continents VIII. Lyon: IARC; 2002.
- Curado M, Edwards B, Shin H, et al. Cancer Incidence in Five Continents IX. Lyon: IARC; 2007.
- Forman D, Bray F, Brewster D, et al. Cancer Incidence in Five Continents X. Lyon: IARC; 2014.
- Steliarova-Foucher E, O'Callaghan M, Ferlay J, et al. The European Cancer Observatory: A new data resource. Eur J Cancer 2015;51:1131-43. [Crossref] [PubMed]
- Engholm G, Ferlay J, Christensen N, et al. NORDCAN--a Nordic tool for cancer information, planning, quality control and research. Acta Oncol 2010;49:725-36. [Crossref] [PubMed]
- GBD 2019 Respiratory Tract Cancers Collaborators. Global, regional, and national burden of respiratory tract cancers and associated risk factors from 1990 to 2019: a systematic analysis for the Global Burden of Disease Study 2019. Lancet Respir Med 2021;9:1030-49. [Crossref] [PubMed]
- GBD 2019 Risk Factors Collaborators. Global burden of 87 risk factors in 204 countries and territories, 1990-2019: a systematic analysis for the Global Burden of Disease Study 2019. Lancet 2020;396:1223-49. [Crossref] [PubMed]
- Global Burden of Disease Cancer Collaboration. Global, Regional, and National Cancer Incidence, Mortality, Years of Life Lost, Years Lived With Disability, and Disability-Adjusted Life-Years for 29 Cancer Groups, 1990 to 2016: A Systematic Analysis for the Global Burden of Disease Study. JAMA Oncol 2018;4:1553-68. [Crossref] [PubMed]
- Wang R, Wang Q, Li J, et al. Light at night and lung cancer risk: A worldwide interdisciplinary and time-series study. Chin Med J Pulm Crit Care Med 2024;2:56-62. [Crossref] [PubMed]
- Xu Q, Zhou Q, Chen J, et al. The incidence of asthma attributable to temperature variability: An ecological study based on 1990-2019 GBD data. Sci Total Environ 2023;904:166726. [Crossref] [PubMed]
- World Development Indicators. 2021. Available online: https://datacatalog.worldbank.org/search/dataset/0037712/World-Development-Indicators. Accessed November 16th 2021.
- Vrieze SI. Model selection and psychological theory: a discussion of the differences between the Akaike information criterion (AIC) and the Bayesian information criterion (BIC). Psychol Methods 2012;17:228-43. [Crossref] [PubMed]
- Reichrath J. The challenge resulting from positive and negative effects of sunlight: how much solar UV exposure is appropriate to balance between risks of vitamin D deficiency and skin cancer? Prog Biophys Mol Biol 2006;92:9-16. [Crossref] [PubMed]
- Norton R, O'Connell MA. Vitamin D. potential in the prevention and treatment of lung cancer. Anticancer Res 2012;32:211-21.
- Muñoz A, Grant WB. Vitamin D and Cancer: An Historical Overview of the Epidemiology and Mechanisms. Nutrients 2022;14:1448. [Crossref] [PubMed]
- Hölzle E, Hönigsmann H. UV-radiation--sources, wavelength, environment. J Dtsch Dermatol Ges 2005;3:S3-10. [Crossref] [PubMed]
- Muhammad I. Chapter 2 - THERMAL RADIATION. In: Muhammad I, editor. An Introduction to Solar Radiation. Academic Press; 1983:29-42.